#DistributedComputing
Explore tagged Tumblr posts
Text
Cloud Computing Interview Questions . . . . for more interview questions https://bit.ly/3E1ywHp check the above link
0 notes
Text
💡 Did you know? 📊 The rise of big data has led to the development of technologies like Apache Hadoop 🐘 and Spark 🔥, which can process vast amounts of data quickly across distributed systems 🌐💻. . . 👉For more information, please visit our website: https://zoofinc.com/ ➡Your Success Story Begins Here. Let's Grow Your Business with us! 👉Do not forget to share with someone whom it is needed.
➡️Let us know your opinion in the comment below 👉Follow Zoof Software Solutions for more information ✓ Feel free to ask any query at [email protected] ✓ For more detail visit: https://zoof.co.in/ . . .
#BigData#DataProcessing#ApacheHadoop#ApacheSpark#DistributedComputing#DataScience#TechInnovation#MachineLearning#DataAnalytics#TechTrends#DataEngineering#DigitalTransformation#ScalableSolutions#AI#WebappSoftwareDevelopment#BestITservice#ZoofUnitedStates#ZoofIndia#SoftwareCompany#StartUpTechnology#Mobilefriendlywebsite#TechnologyConsulting#GrowBusiness#ZoofSoftwareSolutions#Zoof#Zoofinc#MobileAppDevelopment#AwardWinningCompany#BestSoftwareCompany#digitalmarketingtips
0 notes
Text
Edge Computing: Revolutionizing Connectivity and Data Management Across Industries
The global edge computing market size is expected to reach USD 155.90 billion by 2030, and is expected to expand at 36.9% CAGR from 2024 to 2030, according to a new report by Grand View Research, Inc. Artificial Intelligence (AI) integration into the edge environment is projected to drive market growth. An edge AI system is estimated to help businesses make decisions in real time in milliseconds. The need to minimize privacy concerns associated while transmitting large amounts of data, as well as latency and bandwidth issues that limit an organization's data transmission capabilities, are factors projected to fuel market growth in the coming years.
Edge Computing Market Report Highlights
Over the projected period, the edge server segment is expected to be the booming hardware segment. The increased demand for edge servers throughout several industrial verticals accounts for the segment's promising growth prospects
In terms of application, the AR/VR segment is projected to progress at a substantial CAGR because of the developing cellular network, which offers potential development for edge computing. For instance, to provide a high-quality VR experience to users, Ericsson has improved its radio infrastructure and 5G core
In terms of industry vertical, the data center segment is projected to experience the highest CAGR over the estimated period. This can be ascribed to the fact that edge data centers overcome inconsistent connections and compute and store data close to the end-user
The Asia Pacific region is anticipated to expand at the highest CAGR over the estimated period due to the advent of 5G in the region and the increasing number of IoT-incorporated devices. The evolution of telco edge infrastructure to support 5G-enabled applications is expected to be accelerated by the launch of 5G networks
For More Details or Sample Copy please visit link @: Edge Computing Market Report
Machinery control and precision monitoring are a few use cases that are well-suited for AI on the edge. The latency requirement for a fast-running production line must be maintained to a bare minimum, which can be accomplished by using edge computing. Bringing data processing closer to the manufacturing facility can prove to be extremely important, which can be accomplished using AI. Artificial intelligence-based edge devices can be utilized in a wide range of end-point devices, including sensors, cameras, smartphones, and other IoT devices.
Moreover, the telecom edge is estimated to grow exponentially over the projected period. The telecom edge executes computing adjacent to the telco's mini-data centers, which are operated on the telco-owned property. Several telecom operators, including Telstra and Telefonica, are developing prototypes and pilot projects of open-access networks integrated with edge computing. Edge will be at the forefront of the telecom industry once 5G technology is fully deployed. The telecom industry is in a great position to enhance edge computing, but telecom businesses risk being abridged by irrelevant edge suppliers if they do not move up the value chain.
Presently, Edge computing use cases have outpaced initial infrastructure deployments, and are projected to provide momentum to edge computing infrastructure and use case investments. Edge computing is predicted to become more ubiquitous and evolve toward platform-centric solutions over the projection period. With this development, edge platforms can reduce the infrastructure intricacy using orchestration software and sophisticated management, and provide user-friendly environments for programmers to implement innovative edge services and applications.
#EdgeComputing#DecentralizedData#IoT#CloudComputing#EdgeDevices#DataAnalytics#InternetOfThings#DistributedComputing#DigitalTransformation#RealTimeProcessing#EdgeInfrastructure#EdgeSecurity#NetworkOptimization#EdgeApplications#Industry40#CyberPhysicalSystems#EdgeDeployment#EdgeSolutions#EdgeNetworking#EdgeAI
0 notes
Text

Lambda architecture combines both and processing techniques.
a) Online, real-time b) Batch, offline c) Batch, online d) Parallel, distribute
#Software#SoftwareQuiz#followme#followforfollow#instadaily#follow4follow#like4like#letsconnect#amigowayspoll#amigoways#SoftwareTechnology#LambdaArchitecture#RealTimeProcessing#BatchProcessing#BigData#DataProcessing#DistributedComputing#StreamingAnalytics#DataArchitecture#TechInnovation#DataScience
1 note
·
View note
Text
Big Data Hadoop
Big Data Hadoop Online Training program is designed to equip you with the knowledge and skills you need to become a proficient Big Data and Hadoop practitioner. Whether you’re an IT professional looking to unlock the potential of massive datasets or a data enthusiast eager to dive into the world of Big Data analytics, our in-depth training offers you the perfect opportunity to enhance your career and excel in the era of data-driven decision-making.
#magistersign#onlinetraining#BigData#Hadoop#DataScience#DataAnalytics#BigDataAnalytics#ApacheHadoop#DistributedComputing#DataProcessing#HadoopEcosystem
0 notes
Text
#BigData#DataProcessing#InMemory#DistributedComputing#Analytics#MachineLearning#StreamProcessing#ApacheSpark#OpenSource#DataScience#Hadoop#BigDataTools
0 notes
Text
Data Engineering User Guide
Data Engineering User Guide #sql #database #language #query #schema #ddl #dml#analytics #engineering #distributedcomputing #dataengineering #science #news #technology #data #trends #tech #hadoop #spark #hdfs #bigdata
Even though learning about Data engineering is a daunting task, one can have a clear understanding of this filed by following a step-by-step approach. In this blog post, we will go over each of the steps and relevant steps you can follow through as a tutorial to understand Data Engineering and related topics. Concepts on Data In this section, we will learn about data and its quality before…
0 notes
Text
Is Python Ray the Fast Lane to Distributed Computing?
🚀 Is Python Ray the Fast Lane to Distributed Computing? Python Ray, developed by UC Berkeley's RISELab, is revolutionizing distributed computing. This dynamic framework simplifies parallel and distributed Python applications, making complex tasks easier for ML engineers, data scientists, and developers. Discover Ray's layers, core concepts, installation, and versatility in data processing and model training. Read the full article here: [Is Python Ray the Fast Lane to Distributed Computing?](https://ift.tt/BsEQMmb) #distributedcomputing #pythonray #datascience #machinelearning #developers #RISELab List of Useful Links: AI Scrum Bot - ask about AI scrum and agile Our Telegram @itinai Twitter - @itinaicom
#itinai.com#AI#News#Is Python Ray the Fast Lane to Distributed Computing?#AI News#AI tools#Analytics Vidhya#Innovation#itinai#LLM#Productivity#Yana Khare Is Python Ray the Fast Lane to Distributed Computing?
0 notes
Text
Federated Learning: Privacy-Preserving Machine Learning
In today's data-driven world, machine learning has become an essential tool for many industries. However, traditional machine learning approaches often require large amounts of centralized data, which can pose a risk to privacy and security. Federated learning is an innovative approach to machine learning that allows for collaborative model training without the need to centralize data. In this article, we will explore what federated learning is, how it works, and its potential benefits and challenges.
Federated Learning for Data Privacy
Federated learning is a machine learning approach that enables multiple devices or servers to collaboratively train a model without sharing raw data. This approach is particularly useful in situations where data privacy is a concern, as it allows for the distributed training of a model without the need to centralize data. In federated learning, each device locally trains a model on its own data and sends only the updated model parameters to a centralized server. The server then aggregates these updates from multiple devices to create an improved model, which is then sent back to each device for further training. One of the primary benefits of federated learning is data privacy. With traditional machine learning approaches, data needs to be centralized in order to train a model, which can pose a risk to privacy and security. With federated learning, data remains on the local devices and is not shared, which reduces the risk of data breaches and other privacy violations. This is particularly important in industries such as healthcare and finance, where data privacy is of utmost importance. Federated learning also has potential benefits for efficiency and scalability. With traditional machine learning approaches, large amounts of data need to be centralized and processed on a single server, which can be time-consuming and resource-intensive. Federated learning distributes the computation across multiple devices, making it possible to train models on large-scale datasets without the need for a centralized infrastructure. This can result in significant time and cost savings. However, there are also some challenges associated with federated learning. One of the main challenges is ensuring that the local models are accurate and representative of the overall dataset. Since each device only trains on its own data, it is important to ensure that the local models are not biased towards certain types of data or users. This can be addressed through techniques such as stratified sampling and weighted averaging. Another challenge is ensuring that the model updates sent by each device are secure and reliable. Since these updates are sent over a network, they are vulnerable to attacks such as eavesdropping and tampering. This can be addressed through techniques such as secure aggregation and encryption. Federated learning offers a promising approach to privacy-preserving machine learning. As this technology continues to evolve, it is likely that we will see an increasing number of applications in various industries, and it will be important for both researchers and industry professionals to continue to explore its potential benefits and limitations.
Architectures
There are several types of federated learning architecture, including Federated Averaging, Federated Stochastic Gradient Descent, Split Learning, Hybrid Federated Learning, and Collaborative Learning. Each architecture has its own unique features and advantages, making it more suitable for specific types of datasets and applications. Understanding the different federated learning architectures is important for developing efficient and effective machine learning models that can be trained on decentralized data without compromising data privacy or security.
Federated Averaging
Federated Averaging is a popular federated learning algorithm for training machine learning models on decentralized data. In Federated Averaging, the training process is distributed across multiple devices, and the model is updated through a process of aggregation and averaging. The Federated Averaging algorithm works as follows: - A central server distributes the initial model to a set of client devices. - Each client device trains the model locally on its own data, using a stochastic gradient descent algorithm. - After each local training iteration, the client device computes a model update and sends it back to the central server. - The central server aggregates the model updates received from the client devices, by taking a weighted average of the updates. - The central server then computes a new model using the aggregated update, and sends it back to the client devices for further training. - The process is repeated for a set number of rounds or until a convergence criteria is met. - The key advantage of Federated Averaging is that it allows the training of a machine learning model on decentralized data, without the need to centralize the data in one location. This is particularly important when dealing with sensitive or private data, as it allows the data to remain on the client devices and be protected by the clients themselves. Additionally, the Federated Averaging algorithm has been shown to be efficient and effective, particularly for large-scale datasets.
Federated Stochastic Gradient Descent
Federated Stochastic Gradient Descent (FSGD) is a type of federated learning algorithm that enables the training of machine learning models on decentralized data, without the need to centralize the data in one location. In FSGD, each device or node in a decentralized network computes a gradient of the model on its own local data, and sends the gradient to a central server, which aggregates the gradients and updates the model. The FSGD algorithm works as follows: - A central server distributes the initial model to a set of client devices. - Each client device computes a gradient of the model on its own local data, using a stochastic gradient descent algorithm. - The client device sends the gradient to the central server. - The central server aggregates the gradients received from the client devices, by taking the average of the gradients. - The central server updates the model using the aggregated gradient, and sends the updated model back to the client devices. - The process is repeated for a set number of rounds or until a convergence criteria is met. FSGD is particularly useful when dealing with large-scale datasets or when the client devices have limited computing resources or bandwidth. It allows the model to be trained on decentralized data while still being updated centrally, which can help improve the efficiency of the training process. Additionally, FSGD can provide better privacy guarantees than other federated learning algorithms, as the client devices do not need to share their local data with the central server.
Split Learning
Split Learning is a type of federated learning algorithm that allows the training of machine learning models on decentralized data, without the need to transfer the data to a central server for processing. Instead, in Split Learning, a portion of the model is stored on a client device, while the rest of the model is stored on a central server. The client device trains its portion of the model on its own data and sends the results to the central server, which aggregates the results and updates the central portion of the model. The Split Learning algorithm works as follows: - A central server distributes a partially trained model to a set of client devices. - Each client device trains its portion of the model on its own local data, using the partial model as a starting point. - The client device sends the results of its local training to the central server. - The central server aggregates the results received from the client devices, by taking the average of the results. - The central server updates the central portion of the model using the aggregated results, and sends the updated model back to the client devices. - The process is repeated for a set number of rounds or until a convergence criteria is met. Split Learning is particularly useful when dealing with highly sensitive or private data, where it is important to keep the data on the client devices and protect the privacy of the data. Additionally, Split Learning can be more efficient than other federated learning algorithms, as it reduces the amount of data that needs to be transferred between the client devices and the central server.
Hybrid Federated Learning
Hybrid Federated Learning is a type of federated learning algorithm that combines elements of both Federated Averaging and Split Learning. In Hybrid Federated Learning, some layers of the model are stored on the client devices, while other layers are stored on the central server. The client devices train their layers on their own local data and send the results to the central server, which aggregates the results and updates the central layers of the model. The Hybrid Federated Learning algorithm works as follows: - A central server distributes a partially trained model to a set of client devices. - Each client device trains its portion of the model on its own local data, using the local layers and the partially trained model as starting points. - The client device sends the results of its local training to the central server. - The central server aggregates the results received from the client devices, by taking the average of the results. - The central server updates the central layers of the model using the aggregated results, and sends the updated model back to the client devices. - The process is repeated for a set number of rounds or until a convergence criteria is met. Hybrid Federated Learning is particularly useful when dealing with datasets that have both structured and unstructured data, or when different layers of the model require different amounts of processing power. By storing some layers on the client devices and others on the central server, Hybrid Federated Learning can help optimize the training process and improve the efficiency of the algorithm.
Collaborative Learning
Collaborative Learning is a type of federated learning algorithm that enables multiple clients to work together to train a shared machine learning model. In Collaborative Learning, the clients exchange information and collaborate to improve the model, which is then updated centrally. The Collaborative Learning algorithm works as follows: - A central server distributes an initial model to a set of client devices. - Each client device trains the model locally on its own data and sends the results of its training to the other client devices. - The client devices exchange information and collaborate to improve the model, using techniques such as model averaging, model ensembling, and transfer learning. - The client devices then send the updated model to the central server. - The central server aggregates the updated models and computes a new model, which is sent back to the client devices for further training. - The process is repeated for a set number of rounds or until a convergence criteria is met. Collaborative Learning is particularly useful when dealing with datasets that have diverse characteristics, or when the client devices have different computing resources or processing power. By allowing multiple clients to work together to train a shared model, Collaborative Learning can help improve the accuracy and robustness of the model, while also reducing the amount of time and resources required for training.
Conclusion
Federated learning is an innovative machine learning approach that offers a way to train models collaboratively without the need to centralize data. While it offers many benefits, including improved data privacy and efficiency, it also comes with its own set of challenges. As this technology continues to evolve, it is important for both researchers and industry professionals to be aware of its potential benefits and limitations. Read the full article
#biaseddata#collaborativelearning#dataprivacy#distributedcomputing#federatedlearning#machinelearning#modeltraining#privacy#secureaggregation#security
0 notes
Photo

Using boinc again to crunch numbers to fight COVID. I use Rosetta as well but it's currently not working on a project but will be again soon. It just was a couple of days ago. #boinc #worldcommunitygrid #covidvacccine #covi̇d19 #distributedcomputing (at North Ogden, Utah) https://www.instagram.com/p/CaIFliGuPJU/?utm_medium=tumblr
1 note
·
View note
Text
What does the Relictum PRO blockchain platform offer its users?

Today we’ll talk about what Relictum PRO, a latest generation blockchain platform, looks like from the inside, and who the audience for its products is.
We will talk about this with the CTO of the Relictum PRO project, Alexander Strigin, and Nikolai Osipenko, Relictum PRO CMO, and Relictum StartUp Laboratory CEO, Ilnur Rakhmetov. Let’s go!
Read more
#relictum#blockchain#relictumpro#ecosystem#relictumecosystem#gtn#token#cryptocurrency#startup#lab#relictumlab#laboratory#alexanderstrigin#nikolaiosipenko#blockchaintechnology#distributedcomputing#networking#technology#informationtechnology#infrastructure#datamanagement#ceo#like#project
0 notes
Text
Cloud Computing Interview Questions . . . . for more interview questions https://bit.ly/3E1ywHp check the above link
0 notes
Link
Graphics processing unit (GPU) maker Nvidia has asked users of gaming PCs to help in the effort to fight the COVID-19 coronavirus by joining a distributed computing project. The company urged PC gamers to donate unused GPU clock cycles on their computers in a distributed computing project for simulating protein dynamics to help improve knowledge of the coronavirus.
#distributedcomputing#powercomputing#dataprocessing#DistributedComputingProject#GPUclockCycles#COVID19#gaming#gamers#coronavirus#protein#dynamics
0 notes
Text
What is Cloud Computing and Its Benefits?
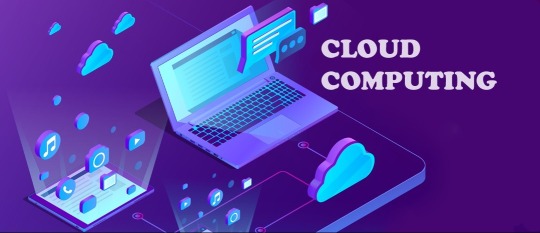
What is Cloud Computing, Its Use, and Benefits You might have heard these words many times, but do you know what this is ultimately Cloud Computing? As we know, computer network technologies have made a lot of progress in the last few years. Since the Internet (the most popular computer network) has expressed its existence, there has been a lot of advancement in the field of Computer Network and there has been considerable research in the fields of technologies like Distributed Computing and Cloud Computing. The concept of Cloud Computing These technical terms The concept of both Distributed Computing and Cloud Computing is often the same. There are some inequalities in both of them. So if you have to understand about Cloud Computing, then it is important for you to have an understanding of Distributed Computing. The Global Industry Analyst has to say that these global cloud computing service market will become a business of up to $ 327 billion by 2020. Almost all the companies are using Cloud Computing service in today's door, directly or indirectly. If we talk about the example, whenever we use the service of Amazon or Google, we are storing all our data in the cloud. If you use Twitter, then you use the indirect cloud computing service. Both Distributed Computing and Cloud Computing are so popular because we needed better computing networks so that our data could be processed faster. So what is cloud computing today? You will learn about this article completely. Then what is the delay, let's start and know what is Cloud? And why is this happening so popular?
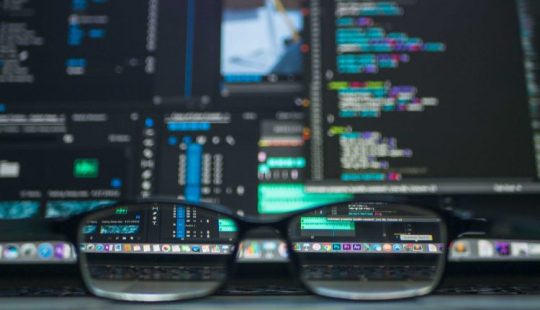
Concept of Distributed Computing and Cloud Computing Service What is the cloud? Talking about Cloud, it is the design of large interconnected networks of servers are designed to deliver computer resources. And there is no real idea of where the data is coming from and where it is going. If I say in a simple language, if a user uses it, then he will feel that he is using a vast formless computing power in which the user can do everything from his email to the mapping of the mobile application according to his need. There is nothing like saying "The Cloud" in the language of business. Computing is a collection of licensed service that is provided by different vendors. Cloud Service technology replaces technology acquisition and replaces them with different products and these products are managed from elsewhere and one thing remains active only when it is needed. What is Cloud Computing? The services provide through the internet called Cloud Computing. This service can be anything such as Off-Site Storage or computing resources. Or so, Cloud Computing is a computing style that provides massively scalable and flexible IT-related capabilities as a service with the help of Internet Technologies. Facilities like infrastructure, platform, application and storage space are available in these services. In this, users use services according to their need and pay the same amount of services they use. For this, they do not need to build their own infrastructure. Nowadays there is a lot of competition in the world and in such a way. People should be served on the Internet at any given time without any delay. When application frozen, there is a lot of dissatisfaction among people. People need their 24/7 service To meet this requirement. We can not emphasize the old mainframe computing so that people used Cloud distributed computing technology to solve the same problem. The big business started to do all of their work very easily. For example, Facebook, which has 757 million active users, who see nearly 2 million photos daily, photos of 3 billion are uploaded every month; 1 million websites use Facebook to make 50 million operations per second. In such a way, the traditional computing system cannot solve these problems. But we need something better that can do this work. Therefore, Cloud Distributed Computing required only to do such computing at this time. Example of Cloud Computing YouTube is a great example of cloud storage that casts millions of video files for users. Picasa and Flickr, which host the digital photographs of millions of users in their server. Google Docs is another example of cloud computing that allows users to upload their presentations, word documents, and spreadsheets into their data servers. Along with this, it also gives the option to edit and publish those documents. Cloud Computing Characteristics and Benefits Related Information Xiaomi India Number One in India’s Smartphone Market Xiaomi Launch Mi Home Security Camera How Does the Internet Actually Work? TRAI NEW RULES extend the deadline for TV users Understand Internet Speeds Sharing Internet Connection With Neighbor is Illegal Google Chrome Latest Version with security and great features Why 4G Is Not A Replacement For Your Home Broadband Jio GigaFiber broadband service TRAI’s Channel Selector and Channel Price List Read the full article
#cloud#cloudcomputing#cloudcomputingservice#computing#computingandcloudcomputing#distributedcomputing#Internet#InternetTechnology#InternetWorks
0 notes
Photo

#cloudstorage #distributedcomputing #cloudcomouting #abstract #abstractart #abstractdesign #graphicdesign #graphicart https://www.instagram.com/stirolak/p/BvUET3rADRa/?utm_source=ig_tumblr_share&igshid=1s20dzg87ihq3
#cloudstorage#distributedcomputing#cloudcomouting#abstract#abstractart#abstractdesign#graphicdesign#graphicart
0 notes