#Image Denoising Using Deep Learning
Explore tagged Tumblr posts
Text
Advanced Image Denoising Solutions – Takeoff
Let me welcome you to Takeoff, your No.1 option for the most advanced Image Denoising tech. Our specialty at Takeoff are the cutting-edge algorithms and software, which remove unwanted noise and Flaws totally from the images, to make them clear and precise. Our technology in image processing and machine learning enables Takeoff develops the latest solutions for applications such as photography, medical imaging and more. Get to know the power of image denoising in Takeoff and further improve your picture quality.
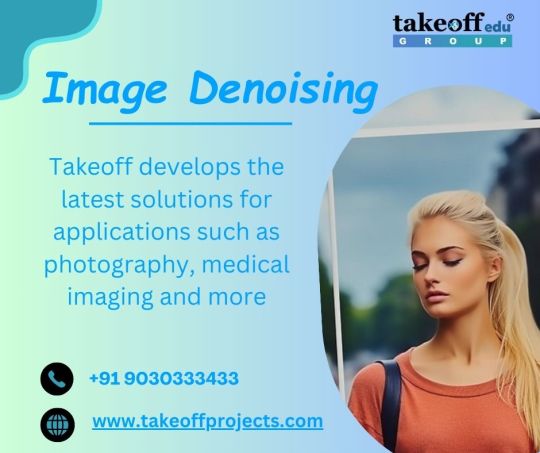
The digital world has the images capable to be distorted or have random dots as the result of bad lighting, camera caliber, or transmission errors, and others. Image denoising algorithms assist in highlighting this noise and discriminating it from other informative details while retaining essential structures of the image.
The algorithms which do this job, recognize different patterns and features of noise in the image. They discern the real image content from the unessential background noise. Lastly, they might use mathematical operations and machine learning techniques to eliminate distortion or disturbance while keeping the important features of the image.
There are a number of denoising approaches, including filters, such as Wiener and Gaussian filters, K-means, and median filters, which smooth out the noise, and more advanced methods, such as wavelet transforms and deep learning-based techniques, which learn how to remove the noise more quickly and effectively.
Conclusion:
Ultimately, Image Denoising that Takeoff provides is an imperative tool for enhancing the quality and the reliability of imageries across several domains. The denoising process is of great importance for photography, medical imaging, surveillance, and satellite imagery. Through the elimination of the noise and the improvement of the clarity, the image will be sharper, more detailed and simpler to perceive. By using the exceptional depixelation technology of Takeoff, the image quality can be improved so that many industries can benefit, thus, creating better viewing experiences for us all.
0 notes
Text

AI model improves 4D STEM imaging for delicate materials
Researchers at Monash University have developed an artificial intelligence (AI) model that significantly improves the accuracy of four-dimensional scanning transmission electron microscopy (4D STEM) images. Called unsupervised deep denoising, this model could be a game-changer for studying materials that are easily damaged during imaging, like those used in batteries and solar cells. The research from Monash University's School of Physics and Astronomy, and the Monash Center of Electron Microscopy, presents a novel machine learning method for denoising large electron microscopy datasets. The study was published in npj Computational Materials. 4D STEM is a powerful tool that allows scientists to observe the atomic structure of materials in unprecedented detail.
Read more.
#Materials Science#Science#Materials Characterization#Computational materials science#Electron microscopy#Monash University
20 notes
·
View notes
Text
This is an excellent summary of the problems with AI Art beyond even the legal issues of copyright- though it indirectly addresses them as well- and especially points out why the comparisons between human learning and the algorithm “learning” only show a deep ignorance of the human process— and why relying on the algorithm will create nothing but stagnation.
From Christopher Doehling:
���I’m often accused of not understanding how gen-AI works. Nahh. I understand. Counter: a lot of tech people don’t understand human creativity or learning. I have backgrounds in all three. But mostly in art and creativity. Check my LinkedIn profile.
Before you come at me with “computers learn/create like peoples do” consider: it may be you who are in a strange land. Not me. Before you go running into the jungle, you might want a guide.
For example, ever wonder why it took us so long to learn how to draw and paint "realistically"(to make images that look like what we see)? It's because by default, our brains learn concepts, not visuals. For most, our eyes are used to recognize, not replicate.
It's like our mind throws away the visual information that is explicit, and exchanges it for understanding. I quickly know a cat from any angle I see it. I know that they are furry quadrupeds that purr when you pet them unless they scratch you. I know a cat when I see one.
My eyes also help me quickly understand the 3d space the cat occupies, so that I know where it is in relationship to my body, so that I can pet it or avoid it. My eyes help me understand "cats". Can I draw one? Not easily, because my brain, by default, doesn't care about that.
Unless... I want to care. I want to make images that represent or communicate my understanding of "Cat". Early art is more abstracted/symbolic because we expressed concepts first before explicit visuals. 4 lines= 4 legs. Shape language tells the story, with an arresting style...
Even if the exact visual (what my eyes saw) is not transmitted. Its not that we didn't want to. We didn't know how, any beginning artist experiences the same problem. Your brain wants to express what it knows conceptually, not what the eye sees.
But over time, our concepts and understanding grew to include things like optics, math, color theory. Tools we could (with great effort) apply to our artistic expressions as well.
Filippo Brunelleschi (re?)discovered linear perspective not just by looking at the world around him or at other art, but by application of those concepts. and then, finally, we could (again?)draw and paint what we saw. We could also make others see what we had only imagined.
So, we draw what we know, about what we see. Even if what we see is other art, even if I do a master copy, It comes from a place of concept. We are seeking to understand technique, another's experience, another's knowledge, not just absorb a visual for later source material.
evidence: If you have not gained at least some of the same conceptual understanding that the master did, you probably will not be able to copy their work, at least not convincingly.
"Generative" Ai (as it is) is not only unlike humans in the way that it learns, it is the polar opposite. It can copy what it sees. it can combine what it sees. But it does so without any understanding at all. About anything. At all.
A computer does not know what "cat" is. It may have some pixels->patterns that are keyworded "cat" but that is all it has. It can denoise from those latent images/parts of images, but it will only do so as instructed by our keyword requests and/or randomly seeded math, etc.
The only concepts delivered into an AI gen image are those given by the original artists or photographers. If you see a cat, its because someone else (or many someones) gave you cats to see. All the Ai did was serve it up, blindly combined.
The uniqueness of each of us, our experiences, and the concepts we learn and teach are what makes art evolve. If Ai had "taken over" for us at, say, the medieval period (in Europe), Art movements would have ended there too. Renniassance, Baroque, Impressionism, Cubism,...
Etc. they never would have happened. because no matter what prompt you gave, all you would get would be remixes of Medieval paintings, or anything previous to that time period. Ai doesn't make anything really new. not the way we do. It only (blindly) combines what's already made.
That's what's at stake here. We are on the brink of handing our creativity over to something that isn't creative. Why would we limit ourselves like that? If you think it makes art easy, it doesn't. It's an illusion. All you have is the art made up to this point. and no more.
If you want to be an artist, be one. No matter what your skill level, it's better than this. You are contributing of yourself to the world. You are contributing. period.
P.S. it’s not that Ai doesn’t have valid uses as a real tool. But when we get the idea that it’s a pet pro artist that “does the dirty work for me”, that’s a dark path. The dirty work is what moves us forward. it’s also the fun part, and we are the only ones who can really do it.”
Original post:
https://twitter.com/dolimac/status/1635286958330224641?s=46&t=MInooHF4e3-CHmlyx2cj8w
12 notes
·
View notes
Text
Intel Open Image Denoise Wins Scientific and Technical Award

Intel Open Image Denoise
Intel Open Image Denoise Wins Scientific and Technical Award
The Academy of Motion Picture Arts and Sciences will honour Intel Open Image Denoise, an open-source library that provides high-performance, AI-based denoising for ray traced images, with a Technical Achievement Award. The Oscar-organizing Academy recognised the library as a modern filmmaking pioneer.
Modern rendering relies on ray tracing. The powerful algorithm creates lifelike pictures, but it demands a lot of computing power. To create noise-free images, ray tracing alone must trace many rays, which is time-consuming and expensive. Adding a good denoiser like Intel Open Image Denoise to the renderer can reduce rendering times and trace fewer rays without affecting image quality.
Intel Open Image Denoise uses AI neural networks to filter out ray tracing noise to speed up rendering and real-time previews during the creative process. Its simple but customisable C/C++ API makes it easy to integrate into most rendering systems. It allows cross-vendor optimisations for most CPU and GPU architectures from Apple, AMD, Nvidia, Arm, and Intel.
Intel Open Image Denoise is part of the Intel Rendering Toolkit and licensed under Apache 2.0. The industry standard for computer-generated images is improved by the widely utilised, highly effective, and detail-preserving U-Net architecture. The library is free and open source, and its training tools lets users train unique denoising models using their own datasets, improving image quality and flexibility. Producers and companies can also retrain integrated denoising neural networks for their renderers, styles, and films.
The Intel Open Image Denoise package relies on deep learning-based denoising filters that can handle 1 spp to virtually converged samples per pixel (spp). This makes it suitable for previews and final frames. To preserve detail, filters can denoise images using only the noisy colour (beauty) buffer or auxiliary feature buffers (e.g. albedo, normal). Most renderers offer buffers as AOVs or make them straightforward to implement.
The library includes pre-trained filter models, however they are optional. With the supplied training toolkit and user-provided photo datasets, it can optimise a filter for a renderer, sample count, content type, scene, etc.
Intel Open Image Denoise supports many CPUs and GPUs from different vendors:
For Intel 64 architecture (SSE4.1 or higher), Apple silicon CPUs use ARM64 (AArch64).
Dedicated and integrated GPUs for the Intel Xe, Xe2, and Xe3 architectures include Intel Arc B-Series Graphics, A-Series Graphics, Pro Series Graphics, Data Centre GPU Flex Series, Data Centre GPU Max Series, Iris Xe Graphics, Intel Core Ultra Processors with Intel Arc Graphics, 11th–14th Gen Intel Core processor graphics, and associated Intel Pentium and Celeron processors.
Volta, Turing, Ampere, Ada Lovelace, Hopper, and Blackwell are NVIDIA GPU architectures.
AMD GPUs with RDNA2 (Navi 21 only), RDNA3 (Navi 3x), and RDNA4 chips
Apple silicon GPUs, like M1
The majority of laptops, workstations, and high-performance computing nodes can run it. Based on the technique, it can be utilized for interactive or real-time ray tracing as well as offline rendering due to its efficiency.
Intel Open Image Denoise uses NVIDIA GPU tensor cores, Intel Xe Matrix Extensions (Intel XMX), and CPU instruction sets SSE4, AVX2, AVX-512, and NEON to denoise well.
Intel Open Image Denoise System Details
Intel Open Image Denoise requires a 64-bit Windows, Linux, or macOS with an Intel 64 (SSE4.1) or ARM64 CPU.
For Intel GPU support, install the latest Intel graphics drivers:
Windows: Intel Graphics Driver 31.0.101.4953+
Intel General Purpose GPU software release 20230323 or newer for Linux
Intel Open Image Denoise may be limited, unreliable, or underperforming if you use outdated drivers. Resizable BAR is required in the BIOS for Intel dedicated GPUs on Linux and recommended on Windows.
For GPU support, install the latest NVIDIA graphics drivers:
Windows: 528.33+
Linux: 525.60.13+
Please also install the latest AMD graphics drivers to support AMD GPUs:
AMD Windows program (Adrenalin Edition 25.3.1+)
Version 24.30.4 of Radeon Software for Linux
Apple GPU compatibility requires macOS Ventura or later.
#IntelOpenImageDenoise#OpenImage#ImageDenoise#AI#CPU#GPU#News#Technews#Technology#Technologynews#Technologytrends#Govindhtech
0 notes
Text
Understanding Generative AI: Key Milestones from GANs to Diffusion Models
Generative AI has become one of the most transformative technologies of the 21st century. It has impacted various sectors, from entertainment to healthcare, design to data science. But as its evolution continues, one might wonder: How did we get here? What are the key milestones that have shaped Generative AI into the powerful tool it is today?
In this article, we will explore the evolution of Generative AI, starting with the introduction of Generative Adversarial Networks (GANs) and advancing to the more recent developments like Diffusion Models. This journey through Generative AI’s evolution will shed light on its capabilities and how it's reshaping industries worldwide. Additionally, we will discuss how you can be part of this technological revolution through Generative AI training online.
The Birth of Generative AI: The GAN Revolution
Generative AI's roots trace back to one of its most iconic developments: Generative Adversarial Networks (GANs), introduced in 2014 by Ian Goodfellow. GANs introduced a novel way of training artificial intelligence to generate data — whether that be images, videos, or even music — that mimics real-world data.
At the heart of GANs is a unique architecture involving two neural networks — the generator and the discriminator. The generator creates synthetic data, while the discriminator evaluates it against real-world data. The two networks "compete" against each other: the generator tries to improve at creating realistic data, and the discriminator gets better at distinguishing between the real and fake. This adversarial process leads to impressive results over time.
Thanks to GANs, we witnessed remarkable advancements in image generation, such as the creation of hyper-realistic faces and landscapes, the production of artwork, and even the deepfake phenomena. The key to GANs' success was the unsupervised learning model, which enabled these networks to learn patterns without being explicitly programmed to do so.
Generative AI training online gained traction during the rise of GANs, as many AI enthusiasts and professionals began to explore how these networks could be applied to various fields. As GANs demonstrated the potential of generative models, they sparked a wave of research into further refining these networks and addressing their inherent challenges.
Refining the Technology: Variational Autoencoders (VAEs)
As GANs made waves in the AI community, another breakthrough in generative models came in the form of Variational Autoencoders (VAEs). Introduced around the same time as GANs, VAEs also served the purpose of generating new data, but their approach was different. While GANs use a confrontational approach, VAEs focus on encoding and decoding data into a latent space.
In a VAE, the input data is compressed into a smaller representation (encoding), and then it is decoded back into data that resembles the original input. The key advantage of VAEs is that they can be more stable and easier to train compared to GANs, though they typically generate less sharp results.
VAEs found great use in tasks like image denoising, anomaly detection, and generating images that share underlying patterns with the original data. This technology also introduced variational inference as a method to optimize generative models. VAEs quickly became a staple in many deep learning applications, offering a more structured approach to generative modeling.
As the interest in generative models grew, Generative AI training online programs became popular, as more AI practitioners sought to understand these cutting-edge models and their applications in various industries.
Diffusion Models: The New Frontier
The latest wave of generative models, which have taken the AI world by storm, is the Diffusion Model. While they may sound complicated, these models work by taking a random noise input and gradually denoising it to form a meaningful output, such as an image, sound, or text. In a sense, Diffusion Models are the reverse of the process used in GANs, which generate data by adding noise to a target.
Diffusion models have gained massive attention in 2022 and beyond, with implementations like DALL·E 2, Stable Diffusion, and MidJourney achieving extraordinary results. These models are capable of generating high-quality images with remarkable attention to detail. For instance, DALL·E 2 can generate photorealistic images from simple textual prompts, showcasing the potential of Diffusion Models to change the way we create visual content.
Unlike GANs, Diffusion Models do not require an adversarial setup and are often simpler to train. They are also more stable, as they do not face the challenges of mode collapse or training instability that GANs are known for. As a result, Diffusion Models are rapidly becoming the go-to generative tool for a variety of applications, from art generation to drug discovery.
With this level of capability, Diffusion Models are reshaping not just the creative industries but also fields like data science, medicine, and biotechnology. The ability to generate high-quality, synthetic data has important implications for training AI models, particularly in fields where data is scarce or expensive to collect.
Where Are We Heading Next?
As we look toward the future of Generative AI, it's clear that we're only scratching the surface of its potential. While GANs and VAEs were foundational, and Diffusion Models are pushing the boundaries, we can expect even more breakthroughs in the coming years. Innovations in transformers, autoregressive models, and even more efficient training methods are on the horizon.
The future of Generative AI is likely to see even more advanced models capable of multimodal generation — that is, generating content that seamlessly integrates multiple forms of media, such as text, images, and audio. We could even see the rise of AI models that not only generate content but also improve the content quality in real-time, offering tools that adapt to our preferences and needs.
For professionals eager to keep up with these rapid advancements, Generative AI training online offers a flexible, accessible way to gain expertise in these cutting-edge technologies. Whether you're looking to explore GANs or master Diffusion Models, enrolling in an online course can give you the knowledge and hands-on experience needed to excel in this field.
Conclusion
Generative AI has come a long way, from the groundbreaking introduction of GANs to the recent success of Diffusion Models. These technologies are revolutionizing industries and offering new possibilities in art, entertainment, healthcare, and beyond. As the field continues to evolve, staying ahead of the curve is essential for anyone interested in AI.
If you're eager to learn more about the latest advancements in Generative AI and explore practical applications for these models, Generative AI training online is the perfect solution. With flexible schedules, expert instructors, and comprehensive course content, online training empowers you to dive deep into this exciting field.
0 notes
Text
Complex and Intelligent Systems, Volume 11, Issue 2, February 2025
1) A low-carbon scheduling method based on improved ant colony algorithm for underground electric transportation vehicles
Author(s): Yizhe Zhang, Yinan Guo, Shirong Ge
2) A survey of security threats in federated learning
Author(s): Yunhao Feng, Yanming Guo, Gang Liu
3) Vehicle positioning systems in tunnel environments: a review
Author(s): Suying Jiang, Qiufeng Xu, Jiachun Li
4) Barriers and enhance strategies for green supply chain management using continuous linear diophantine neural networks
Author(s): Shougi S. Abosuliman, Saleem Abdullah, Nawab Ali
5) XTNSR: Xception-based transformer network for single image super resolution
Author(s): Jagrati Talreja, Supavadee Aramvith, Takao Onoye
6) Efficient guided inpainting of larger hole missing images based on hierarchical decoding network
Author(s): Xiucheng Dong, Yaling Ju, Jinqing He
7) A crossover operator for objective functions defined over graph neighborhoods with interdependent and related variables
Author(s): Jaume Jordan, Javier Palanca, Vicente Julian
8) A multitasking ant system for multi-depot pick-up and delivery location routing problem with time window
Author(s): Haoyuan Lv, Ruochen Liu, Jianxia Li
9) Short-term urban traffic forecasting in smart cities: a dynamic diffusion spatial-temporal graph convolutional network
Author(s): Xiang Yin, Junyang Yu, Xiaoli Liang
10) Vehicle-routing problem for low-carbon cold chain logistics based on the idea of cost–benefit
Author(s): Yan Liu, Fengming Tao, Rui Zhu
11) Enhancing navigation performance in unknown environments using spiking neural networks and reinforcement learning with asymptotic gradient method
Author(s): Xiaode Liu, Yufei Guo, Zhe Ma
12) Mape: defending against transferable adversarial attacks using multi-source adversarial perturbations elimination
Author(s): Xinlei Liu, Jichao Xie, Zhen Zhang
13) Robust underwater object tracking with image enhancement and two-step feature compression
Author(s): Jiaqing Li, Chaocan Xue, Bin Lin
14) DMR: disentangled and denoised learning for multi-behavior recommendation
Author(s): Yijia Zhang, Wanyu Chen, Feng Qi
15) A traffic prediction method for missing data scenarios: graph convolutional recurrent ordinary differential equation network
Author(s): Ming Jiang, Zhiwei Liu, Yan Xu
16) Enhancing zero-shot stance detection via multi-task fine-tuning with debate data and knowledge augmentation
Author(s): Qinlong Fan, Jicang Lu, Shouxin Shang
17) Adaptive temporal-difference learning via deep neural network function approximation: a non-asymptotic analysis
Author(s): Guoyong Wang, Tiange Fu, Mingchuan Zhang
18) A bi-subpopulation coevolutionary immune algorithm for multi-objective combinatorial optimization in multi-UAV task allocation
Author(s): Xi Chen, Yu Wan, Jun Tang
19) Protocol-based set-membership state estimation for linear repetitive processes with uniform quantization: a zonotope-based approach
Author(s): Minghao Gao, Pengfei Yang, Qi Li
20) Optimization of high-dimensional expensive multi-objective problems using multi-mode radial basis functions
Author(s): Jiangtao Shen, Xinjing Wang, Zhiwen Wen
21) Computationally expensive constrained problems via surrogate-assisted dynamic population evolutionary optimization
Author(s): Zan Yang, Chen Jiang, Jiansheng Liu
22) View adaptive multi-object tracking method based on depth relationship cues
Author(s): Haoran Sun, Yang Li, Kexin Luo
23) Preference learning based deep reinforcement learning for flexible job shop scheduling problem
Author(s): Xinning Liu, Li Han, Huadong Miao
24) Microscale search-based algorithm based on time-space transfer for automated test case generation
Author(s): Yinghan Hong, Fangqing Liu, Guizhen Mai
25) A hybrid Framework for plant leaf disease detection and classification using convolutional neural networks and vision transformer
Author(s): Sherihan Aboelenin, Foriaa Ahmed Elbasheer, Khalid M. Hosny
26) A novel group-based framework for nature-inspired optimization algorithms with adaptive movement behavior
Author(s): Adam Robson, Kamlesh Mistry, Wai-Lok Woo
27) A generalized diffusion model for remaining useful life prediction with uncertainty
Author(s): Bincheng Wen, Xin Zhao, Jianfeng Li
28) A joint learning method for low-light facial expression recognition
Author(s): Yuanlun Xie, Jie Ou, Wenhong Tian
29) MKER: multi-modal knowledge extraction and reasoning for future event prediction
Author(s): Chenghang Lai, Shoumeng Qiu
30) RL4CEP: reinforcement learning for updating CEP rules
Author(s): Afef Mdhaffar, Ghassen Baklouti, Bernd Freisleben
31) Practice of an improved many-objective route optimization algorithm in a multimodal transportation case under uncertain demand
Author(s): Tianxu Cui, Ying Shi, Kai Li
32) Sentimentally enhanced conversation recommender system
Author(s): Fengjin Liu, Qiong Cao, Huaiyu Liu
33) New Jensen–Shannon divergence measures for intuitionistic fuzzy sets with the construction of a parametric intuitionistic fuzzy TOPSIS
Author(s): Xinxing Wu, Qian Liu, Xu Zhang
34) TMFN: a text-based multimodal fusion network with multi-scale feature extraction and unsupervised contrastive learning for multimodal sentiment analysis
Author(s): Junsong Fu, Youjia Fu, Zihao Xu
35) Batch-in-Batch: a new adversarial training framework for initial perturbation and sample selection
Author(s): Yinting Wu, Pai Peng, Le Li
36) RenalSegNet: automated segmentation of renal tumor, veins, and arteries in contrast-enhanced CT scans
Author(s): Rashid Khan, Chao Chen, Bingding Huang
37) Light-YOLO: a lightweight detection algorithm based on multi-scale feature enhancement for infrared small ship target
Author(s): Ji Tang, Xiao-Min Hu, Wei-Neng Chen
38) CPP: a path planning method taking into account obstacle shadow hiding
Author(s): Ruixin Zhang, Qing Xu, Guo Zhang
39) A semi-supervised learning technique assisted multi-objective evolutionary algorithm for computationally expensive problems
Author(s): Zijian Jiang, Chaoli Sun, Sisi Wang
40) An adjoint feature-selection-based evolutionary algorithm for sparse large-scale multiobjective optimization
Author(s): Panpan Zhang, Hang Yin, Xingyi Zhang
41) Balanced coarse-to-fine federated learning for noisy heterogeneous clients
Author(s): Longfei Han, Ying Zhai, Xiankai Huang
0 notes
Text
"Image Denoising with Deep Learning: A Practical Approach"
Introduction Image Denoising with Deep Learning: A Practical Approach is a comprehensive tutorial that covers the fundamentals of image denoising using deep learning techniques. This tutorial is designed for practitioners and researchers who want to learn how to implement and optimize image denoising models using popular deep learning frameworks. In this tutorial, we will cover the following…
0 notes
Text
Gen AI Training | Generative AI Course in Hyderabad
Generative AI Training: Essential Terms, Tools, and Techniques
The field of artificial intelligence (AI) is expanding rapidly, and one of the most exciting areas driving this progress is Generative AI. For anyone interested in taking up a Generative AI Course in Hyderabad or diving into Generative AI Training online, this article will help you get familiar with the essentials. As GenAI Training grows in popularity, understanding its key terms, tools, and techniques is crucial for developing the skills needed in the industry. This guide will walk you through foundational terms, introduce popular tools, and explain the techniques behind Generative AI.

Understanding Key Terms in Generative AI
Generative AI, sometimes referred to as GenAI, encompasses algorithms and models that generate new content, such as images, text, audio, or even video. To fully benefit from Gen AI Training and make the most of a Generative AI Course in Hyderabad, you should be comfortable with a few important terms.
Neural Networks: Neural networks are the backbone of Generative AI. They are computational models inspired by the human brain, used to recognize patterns and make predictions. Generative AI typically uses deep neural networks, especially for image, text, and audio generation.
GANs (Generative Adversarial Networks): GANs consist of two networks—a generator and a discriminator. The generator produces new data samples, whereas the discriminator assesses their validity. This interaction results in refined outputs, whether they be realistic images or high-quality audio samples.
Transformers: Transformers are models mainly used in natural language processing tasks, such as generating coherent paragraphs of text. The advent of transformer models, such as GPT (Generative Pre-trained Transformer), has led to breakthroughs in creating text, coding assistance, and more.
Autoencoders: Autoencoders are neural networks used to learn efficient data encodings. For instance, in image processing, an autoencoder compresses an image to understand its structure and then reconstructs it, aiding in applications such as image denoising and colorization.
Diffusion Models: A newer approach in Generative AI, diffusion models create images by progressively denoising a random noise signal. They are gaining popularity for their high quality in image generation and serve as an alternative to GANs.
Exploring Essential Tools for Generative AI
One of the first things you will encounter in a GenAI Training is the variety of tools and platforms used to develop Generative AI models. Here’s a look at some widely-used tools in the field.
TensorFlow and PyTorch: These are two of the most popular open-source libraries for machine learning and AI. TensorFlow, developed by Google, and PyTorch, developed by Facebook, are essential for building neural networks, including GANs and transformer models. Both are widely used for research and production and have extensive communities and resources for beginners.
Hugging Face: This is a popular platform for natural language processing (NLP) models, especially those using transformer-based architectures. Hugging Face hosts a wide range of pre-trained models that can be fine-tuned for various tasks like text generation, sentiment analysis, and question answering.
Google Colab: Google Colab is a free cloud-based tool that provides a Jupyter Notebook environment with GPU and TPU support. It’s widely used in Generative AI Training and is accessible for beginners who want to test and train models without needing a high-performance computer.
Runway ML: Runway ML is a creative tool that allows users to experiment with Generative AI models without needing deep programming knowledge. It’s popular for generating images, videos, and other artistic content.
OpenAI API: With OpenAI’s GPT-3 and DALL-E models, users can quickly integrate advanced Generative AI capabilities into their applications. Many Generative AI Courses in Hyderabad and elsewhere incorporate OpenAI tools for text, image, and other types of data generation.
Key Techniques in Generative AI
The techniques involved in Generative AI are complex, but understanding the basics can help beginners progress effectively. Courses, such as a Generative AI Course in Hyderabad, will usually cover these approaches.
Data Preprocessing: One of the first steps in Generative AI is data preprocessing, which prepares the dataset for training. This might involve data cleaning, normalization, and augmenting the data, which is especially crucial in image and audio generation. Preprocessing sets the foundation for the model to learn effectively.
Model Training and Tuning: Training Generative AI models is a computationally intensive task. With frameworks like TensorFlow and PyTorch, training involves feeding the model with input data and adjusting parameters. Model tuning refers to adjusting hyperparameters to optimize the model's performance, a skill heavily emphasized in Generative AI Training.
Fine-Tuning and Transfer Learning: Transfer learning is a method where pre-trained models are fine-tuned for specific tasks, saving time and resources. In many Generative AI Training programs, you’ll encounter tasks where a model trained on one dataset (e.g., images) is fine-tuned to generate a different dataset (e.g., specific objects in images).
Evaluation Metrics: After training, evaluating the model’s output is essential to ensure its quality and usability. For GANs, metrics like the Inception Score or Fréchet Inception Distance are used. For text models, metrics such as BLEU or ROUGE are common. These metrics help in refining models to meet desired standards.
Ethical Considerations and Safety: As Generative AI capabilities grow, it’s crucial to understand ethical considerations, including biases in generated data and potential misuse. Many training courses, such as a Generative AI Course in Hyderabad, will emphasize the importance of ethical Generative AI practices.
Conclusion
Learning Generative AI can be an exciting journey filled with discovery and innovation. Starting with a Generative AI Course in Hyderabad or participating in online Generative AI Training can open doors to understanding the potential of AI in generating creative content, aiding research, and more. From neural networks to GANs, knowing the key terms, tools, and techniques is essential for success in this field.
Visualpath is the Leading and Best Software Online Training Institute in Hyderabad. Avail complete Generative AI Online Training Worldwide. You will get the best course at an affordable cost.
Attend Free Demo
Call on - +91-9989971070.
Visit Blog: https://visualpathblogs.com/
WhatsApp: https://www.whatsapp.com/catalog/919989971070
Visit https://www.visualpath.in/online-gen-ai-training.html
#GenAI Training#Generative AI Training#Gen AI Training in Hyderabad#Genai Online Training#GenAI Course in Hyderabad#Generative AI Course in Hyderabad#Generative AI Online Training Courses#Generative AI Training Course
1 note
·
View note
Text
3D Scanner System Solution Based on FET3576-C Embedded Board
3D scanning, an integrated technology of optics, mechanics, electronics, and computing, captures 3D coordinates and color information of object surfaces through non-contact measurement, generating high-precision 3D models. In game development, 3D scanners have significantly enhanced the realism and detail richness of game scenes, characters, and objects.
The production team of the popular game "Black Myth: Wukong" spent a year using 3D scanning to create precise 3D models of real Buddhist statues, temples, and rocks across China. These models not only provide rich materials and inspiration for game designers but also ensure the authenticity and detail richness of game scenes. By capturing real-world 3D data, every detail in the game accurately reproduces historical features, enhancing the game's cultural depth and artistic value.
Not only in the gaming field, but 3D scanning systems, with their high precision, non-contact measurement, and digitization characteristics, can also contribute to the preservation and restoration of cultural relics. The fire at Notre-Dame Cathedral in 2019 attracted global attention. Prior to this, Notre-Dame Cathedral had already undergone comprehensive data recording using 3D scanning technology. This allows experts to use this data to carry out precise repair work after the fire. In addition, it can also be widely used in surveying and mapping engineering, industrial manufacturing, architectural design, education and scientific research and many other industries.
The 3D scanner, as the core component, is responsible for data acquisition and conversion. It consists of a probe, lens, and camera. The probe collects surface patterns without contact, the lens adjusts the probe position and viewing angle, and the camera converts captured images into digital signals for further processing like denoising, stitching, and optimization.
Hardware implementation faces several challenges:
Complex data processing: Limited by onsite conditions during data collection, processing can be complex, requiring extended post-processing like noise removal and mesh reconstruction, demanding high-performance hardware.
Compatibility issues: Incompatible hardware and software designs for point cloud data processing across different manufacturers hinder resource sharing and conversion, increasing learning and technical difficulties for users.
Environmental sensitivity: Lasers and optical systems are sensitive to temperature changes, requiring frequent calibration and affecting efficiency. External factors like temperature, humidity, and vibration can also impact measurement accuracy, necessitating strict environmental control.
High cost: The hardware cost of 3D scanners is often high, potentially leading to increased product prices and affecting market competitiveness.
Forlinx Embedded recommends using the FET3576-C embedded board as the main controller for 3D scanners. Based on the RK3576 processor, the FET3576-C is a high-performance, low-power, feature-rich, cost-effective solution tailored for AIoT and industrial markets. It integrates four ARM Cortex-A72 and four ARM Cortex-A53 high-performance cores, with a 6TOPS powerful NPU supporting various operations and deep learning frameworks.

(1)Versatile Interfaces
With GPIO, UART, SPI, and other communication interfaces, the FET3576-C precisely controls scanning parameters (e.g., scanning speed, resolution) and sensor states (e.g., laser emitter, camera shutter), enhancing scanning efficiency and quality
(2)Data Processing Hub
As the system's ''brain,'' the FET3576-C processes raw data like laser point clouds and images, ensuring accurate and complete 3D models through filtering, denoising, registration, and fusion.
(3)Intelligent Optimization
6TOPS NPU enables intelligent analysis of scanned data, automatically identifying and optimizing issues like flaws and holes in models, further enhancing their fineness and realism.
(4)Data Transmission and Storage
Supporting up to 4GB LPDDR4 RAM and 32GB eMMC storage, the FET3576-C securely and efficiently stores scan data locally. Additionally, it supports dual Gigabit Ethernet and Wi-Fi for real-time data transmission and remote access.
(5)Product Stability
With a board-to-board connection design, the FET3576-C facilitates easy installation and maintenance. It has undergone rigorous industrial environment testing, providing excellent stability, and a 10-15 year longevity ensures long-term supply reliability for customer products.
Forlinx Embedded FET3576-C embedded board plays a crucial role in 3D scanning systems. As the main controller, it leverages its powerful data processing capabilities and rich interface resources to enable end-to-end control from data acquisition to model output, providing a solid technical foundation for building high-precision and realistic 3D models.
More info:
0 notes
Text

Image denoising using a diffractive material
While image denoising algorithms have undergone extensive research and advancements in the past decades, classical denoising techniques often necessitate numerous iterations for their inference, making them less suitable for real-time applications. The advent of deep neural networks (DNNs) has ushered in a paradigm shift, enabling the development of non-iterative, feed-forward digital image denoising approaches. These DNN-based methods exhibit remarkable efficacy, achieving real-time performance while maintaining high denoising accuracy. However, these deep learning-based digital denoisers incur a trade-off, demanding high-cost, resource- and power-intensive graphics processing units (GPUs) for operation.
Read more.
12 notes
·
View notes
Text
Online Image Processing Tools
Image processing involves altering the look of an image to improve its aesthetic information for human understanding or enhance its utility for unsupervised computer perception. Digital image processing, a subset of electronics, converts a picture into an array of small integers called pixels. These pixels represent physical quantities such as the brightness of the surroundings, stored in digital memories, and processed by a computer or other digital hardware.
The fascination with digital imaging techniques stems from two key areas of application: enhancing picture information for human comprehension and processing image data for storage, transmission, and display for unsupervised machine vision. This paper introduces several online image processing tools developed and built specifically by Saiwa.

Online Image Denoising
Image denoising is the technique of removing noise from a noisy image to recover the original image. Detecting noise, edges, and texture during the denoising process can be challenging, often resulting in a loss of detail in the denoised image. Therefore, retrieving important data from noisy images while avoiding information loss is a significant issue that must be addressed.
Denoising tools are essential online image processing utilities for removing unwanted noise from images. These tools use complex algorithms to detect and remove noise while maintaining the original image quality. Both digital images and scanned images can benefit from online image noise reduction tools. These tools are generally free, user-friendly, and do not require registration.
Noise can be classified into various types, including Gaussian noise, salt-and-pepper noise, and speckle noise. Gaussian noise, characterized by its normal distribution, often results from poor illumination and high temperatures. Salt-and-pepper noise, which appears as sparse white and black pixels, typically arises from faulty image sensors or transmission errors. Speckle noise, which adds granular noise to images, is common in medical imaging and remote sensing.
Online denoising tools employ various algorithms such as Gaussian filters, median filters, and advanced machine learning techniques. Gaussian filters smooth the image, reducing high-frequency noise, but can also blur fine details. Median filters preserve edges better by replacing each pixel's value with the median of neighboring pixel values. Machine learning-based methods, such as convolutional neural networks (CNNs), have shown significant promise in effectively denoising images while preserving essential details.
Image Deblurring Online
Image deblurring involves removing blur abnormalities from images. This process recovers a sharp latent image from a blurred image caused by camera shake or object motion. The technique has sparked significant interest in the image processing and computer vision fields. Various methods have been developed to address image deblurring, ranging from traditional ones based on mathematical principles to more modern approaches leveraging machine learning and deep learning.
Online image deblurring tools use advanced algorithms to restore clarity to blurred images. These tools are beneficial for both casual users looking to enhance their photos and professionals needing precise image restoration. Like denoising tools, many deblurring tools are free, easy to use, and accessible without registration.
Blur in images can result from several factors, including camera motion, defocus, and object movement. Camera motion blur occurs when the camera moves while capturing the image, leading to a smearing effect. Defocus blur happens when the camera lens is not correctly focused, causing the image to appear out of focus. Object movement blur is caused by the motion of the subject during the exposure time.
Deblurring techniques can be broadly categorized into blind and non-blind deblurring. Blind deblurring methods do not assume any prior knowledge about the blur, making them more versatile but computationally intensive. Non-blind deblurring, on the other hand, assumes some knowledge about the blur kernel, allowing for more efficient processing. Modern approaches often combine traditional deblurring algorithms with deep learning models to achieve superior results.
Image Deraining Online

Image deraining is the process of removing unwanted rain effects from images. This task has gained much attention because rain streaks can reduce image quality and affect the performance of outdoor vision applications, such as surveillance cameras and self-driving cars. Processing images and videos with undesired precipitation artifacts is crucial to maintaining the effectiveness of these applications.
Online image deraining tools employ sophisticated techniques to eliminate rain streaks from images. These tools are particularly valuable for improving the quality of images used in critical applications, ensuring that rain does not hinder the visibility and analysis of important visual information.
Rain in images can obscure essential details, making it challenging to interpret the visual content accurately. The presence of rain streaks can also affect the performance of computer vision algorithms, such as object detection and recognition systems, which are vital for applications like autonomous driving and surveillance.
Deraining methods typically involve detecting rain streaks and removing them while preserving the underlying scene details. Traditional approaches use techniques like median filtering and morphological operations to identify and eliminate rain streaks. However, these methods can struggle with complex scenes and varying rain intensities. Recent advancements leverage deep learning models, such as convolutional neural networks (CNNs) and generative adversarial networks (GANs), to achieve more robust and effective deraining results.
Image Contrast Enhancement Online

Image contrast enhancement increases object visibility in a scene by boosting the brightness difference between objects and their backgrounds. This process is typically achieved through contrast stretching followed by tonal enhancement, although it can also be done in a single step. Contrast stretching evenly enhances brightness differences across the image's dynamic range, while tonal improvements focus on increasing brightness differences in dark, mid-tone (grays), or bright areas at the expense of other areas.
Online image contrast enhancement tools adjust the differential brightness and darkness of objects in an image to improve visibility. These tools are essential for various applications, including medical imaging, photography, and surveillance, where enhanced contrast can reveal critical details otherwise obscured.
Contrast enhancement techniques can be divided into global and local methods. Global methods, such as histogram equalization, adjust the contrast uniformly across the entire image. This approach can effectively enhance contrast but may result in over-enhancement or loss of detail in some regions. Local methods, such as adaptive histogram equalization, adjust the contrast based on local image characteristics, providing more nuanced enhancements.
Histogram equalization redistributes the intensity values of an image, making it easier to distinguish different objects. Adaptive histogram equalization divides the image into smaller regions and applies histogram equalization to each, preserving local details while enhancing overall contrast. Advanced methods, such as contrast-limited adaptive histogram equalization (CLAHE), limit the enhancement in regions with high contrast, preventing over-amplification of noise.
Image Inpainting Online
Image inpainting is one of the most complex tools in online image processing. It involves filling in missing sections of an image. Texture synthesis-based approaches, where gaps are repaired using known surrounding regions, have been one of the primary solutions to this challenge. These methods assume that the missing sections are repeated somewhere in the image. For non-repetitive areas, a general understanding of source images is necessary.
Developments in deep learning and convolutional neural networks have advanced online image inpainting. These tools combine texture synthesis and overall image information in a twin encoder-decoder network to predict missing areas. Two convolutional sections are trained concurrently to achieve accurate inpainting results, making these tools powerful and efficient for restoring incomplete images.
Inpainting applications range from restoring old photographs to removing unwanted objects from images. Traditional inpainting methods use techniques such as patch-based synthesis and variational methods. Patch-based synthesis fills missing regions by copying similar patches from the surrounding area, while variational methods use mathematical models to reconstruct the missing parts.
Deep learning-based inpainting approaches, such as those using generative adversarial networks (GANs) and autoencoders, have shown remarkable results in generating realistic and contextually appropriate content for missing regions. These models learn from large datasets to understand the structure and context of various images, enabling them to predict and fill in missing parts with high accuracy.
Conclusion
The advent of online image processing tools has revolutionized how we enhance and manipulate images. Tools for denoising, deblurring, deraining, contrast enhancement, and inpainting provide accessible, user-friendly solutions for improving image quality. These tools leverage advanced algorithms and machine learning techniques to address various image processing challenges, making them invaluable for both casual users and professionals.
As technology continues to evolve, we can expect further advancements in online image processing tools, offering even more sophisticated and precise capabilities. Whether for personal use, professional photography, or critical applications in fields like medical imaging and autonomous driving, these tools play a crucial role in enhancing our visual experience and expanding the potential of digital imaging.
0 notes
Text
Nvidia DLSS 3.5: Introducing Ray Tracing Reconstruction

Nvidia DLSS 3.5
For intense ray-traced games and applications, DLSS 3.5 with Ray Reconstruction produces ray-traced images of superior quality. This cutting edge AI-powered neural renderer increases ray-traced image quality for all GeForce RTX GPUs by using an NVIDIA supercomputer-trained AI network. Shadows, reflections, and global illumination are improved, making the game more realistic and immersive.
A Light Ray By creating physically accurate reflections, refractions, shadows, and indirect lighting, ray tracing is a rendering technique that can faithfully mimic the illumination of a scene and its objects. By following the path of light from the view camera, which determines the view into the scene through the 2D viewing plane, out into the 3D scene, and back to the light sources, ray tracing creates computer graphics images. For example, reflections are produced when rays hit a mirror.
It is the digital counterpart of real-world objects that are lit up by light beams and the path that light takes from the viewer’s eye to the objects that light interacts with. Ray tracing is that.
It takes a lot of processing power to simulate light in this way shooting rays for each pixel on the screen even for offline renderers that compute scenes over the period of several minutes or hours. Instead, to obtain a representative sample of the illumination, reflectivity, and shadowing of the scene, ray samples fire a small number of rays at different points throughout the picture.
There are restrictions, though. The result is a noisy, pixelated, and gapped image that can be used to determine the desired ray trace of the scene. Hand-tuned denoisers employ two distinct techniques to fill in the missing pixels that weren’t ray traced: temporally gathering pixels over a number of frames and spatially interpolating them to blend adjacent pixels together. This procedure creates a ray-traced image from the noisy raw output.
This makes the development process more complicated and expensive, and also lowers the frame rate in heavily ray-traced games when several denoisers are running at once to produce various lighting effects.
With Nvidia DLSS 3.5 Ray Reconstruction, an AI-powered neural network trained on an NVIDIA supercomputer produces better-quality pixels between the sampled rays. In order to use temporal and spatial data more intelligently, it distinguishes between various ray-traced effects and preserves high frequency information for upscaling of higher quality. Additionally, it recreates in-game lighting patterns it has identified from its training data, such as ambient occlusion or global illumination.
One excellent example of Ray Reconstruction in action is Portal using RTX. The denoiser finds it difficult to recreate the dynamic shadowing with the fan moving while DLSS is turned off.
When Nvidia DLSS 3.5 and Ray Reconstruction are enabled, the AI-trained denoiser learns specific patterns linked to shadows and maintains image stability, collecting precise pixels and combining adjacent pixels to produce reflections of superior quality.
Deep Gaming and Deep Learning DLSS performance is multiplied by AI graphics innovations such as Ray Reconstruction. The main component of DLSS, Super Resolution, reconstructs native-quality images by sampling several lower resolution images and using motion information and feedback from earlier frames. High visual quality is achieved without compromising gameplay.
With the release of DLSS 3, Frame Generation which improves performance by utilising AI to analyse surrounding frames’ data and forecast the appearance of the next generated frame was introduced. After that, these created frames are sandwiched between rendered frames. When DLSS Super Resolution is combined with the frames produced by DLSS, DLSS 3 can use AI to reconstruct seven-eighths of the pixels that are displayed, increasing frame speeds by up to four times when DLSS is not used.
Even in situations where the CPU is the game’s bottleneck, DLSS Frame Generation can increase frame rates since it is post-processed that is, applied to the GPU after the main render.
Ray tracing is a key component of Nvidia DLSS 3.5‘s emphasis on enhancing image quality, and this direction may be pursued in later iterations. It is possible that even at reduced resolutions, DLSS will produce images that are identical to native resolution.
Support for increasingly sophisticated rendering methods As video games incorporate more intricate rendering methods, such as global illumination and ray tracing, DLSS may develop to effectively support these methods. This might enable breathtaking images without compromising functionality.
Integration of AI in game creation AI is becoming more and more significant in the game development process. In order to help creators optimise their games for DLSS and guarantee the optimum speed and visual quality, DLSS may eventually integrate with AI technologies.
DLSS for VR applications VR gaming is growing in popularity, however it might be difficult to render VR games at high resolutions. To enhance performance and resolution, DLSS might be modified for virtual reality, resulting in more immersive and seamless VR experiences.
More precise developer controls With Nvidia DLSS 3.5, game developers have greater influence over how DLSS functions in their creations. If this pattern persists, developers will have much more flexibility to customise DLSS to the unique requirements of their games.
These are merely forecasts; the direction that Nvidia takes in developing the technology and future developments in technology will determine how DLSS develops. But Nvidia DLSS 3.5 bodes well for an era of PC gaming with excellent performance and breathtaking graphics.
FAQS What is DLSS 3.5? Nvidia’s Deep Learning Super Sampling (DLSS) technology was updated in September 2021 with the release of DLSS 3.5. It makes use of machine learning to enhance the graphics quality and performance of games particularly those that employ ray tracing.
Read more on Govindhtech.com
1 note
·
View note
Text
Enhance Your Visual Content with 10 Best AI Image Generator Tools
In today's digital age, the importance of captivating visual content cannot be overstated. Whether it's for marketing purposes, branding, or simply engaging with your audience, compelling visuals play a crucial role in capturing attention and conveying messages effectively. However, creating high-quality visuals can be a time-consuming and resource-intensive process. This is where AI image generator tools come into play, offering innovative solutions to streamline the creation of stunning visuals. In this comprehensive guide, we'll explore the top 10 AI image generator tools that can elevate your visual content to new heights.
Top 10 AI Image Generator Tools
Deep Dream Generator
Description: Deep Dream Generator utilizes neural network algorithms to transform images into dream-like creations.
Features: Customizable filters, easy-to-use interface, cloud-based processing.
Benefits: Add surreal and artistic elements to your visuals, ideal for creative projects and experimental designs.
2. Runway ML
Description: Runway ML is a versatile platform that allows users to create and deploy AI models for various tasks, including image generation.
Features: Access to pre-trained models, real-time collaboration, and support for custom models.
Benefits: Empowers users to generate realistic images across different styles and themes, suitable for professionals and hobbyists alike.
3. Artbreeder
Description: Artbreeder employs a unique breeding technique that enables users to combine and evolve images to create new visual concepts.
Features: Gene manipulation tools, high-resolution outputs, and community sharing.
Benefits: Unlock limitless creative possibilities by blending and refining images, perfect for generating custom artwork and illustrations.
4. DALL-E
Description: Developed by OpenAI, DALL-E is an AI model specifically designed for generating images from textual descriptions.
Features: Natural language input, high-quality image synthesis, fine-grained control over output.
Benefits: Seamlessly translate ideas and concepts into visually stunning images, ideal for storytelling and concept visualization.
5. GANPaint Studio
Description: GANPaint Studio utilizes generative adversarial networks (GANs) to edit and manipulate images with intuitive brush tools.
Features: Object removal and addition, style transfer, interactive editing.
Benefits: Effortlessly edit and enhance images with advanced AI-powered tools, perfect for retouching and photo manipulation tasks.
6. DeepArt
Description: DeepArt employs deep neural networks to transform photos into artworks inspired by famous artists and art styles.
Features: Style transfer presets, customizable parameters, batch processing.
Benefits: Infuse artistic flair into your visuals with ease, great for creating unique and eye-catching designs for various purposes.
7. ImgLab
Description: ImgLabs offers a range of AI-powered tools for image enhancement, restoration, and generation.
Features: Image upscaling, denoising, content-aware fill.
Benefits: Improve the quality of your images and generate realistic visuals with state-of-the-art AI algorithms, perfect for photographers and graphic designers.
8. AI Arta
Description: AI Arta is an innovative platform that harnesses the power of artificial intelligence to revolutionize the world of art creation and curation.
Features: AI Arta boasts a plethora of features tailored to various aspects of art, including painting, drawing, sculpture, and digital art.
Benefits: With AI Arta, users can unlock their creative potential and explore new avenues of artistic expression.
9. DeepAI
Description: DeepAI offers a suite of AI image processing tools, including image generation, recognition, and manipulation.
Features: Image generation APIs, deep learning models, real-time processing.
Benefits: Access cutting-edge AI technologies to generate high-quality visuals and perform advanced image analysis tasks, suitable for developers and researchers.
10. Designify
Description: Designify is a comprehensive AI-driven platform designed to streamline the process of graphic design and visual communication.
Features: Designify offers a wide array of features and tools tailored to the needs of graphic designers, marketers, and content creators.
Benefits: By utilizing Designify, users can significantly enhance their productivity, unleash their creativity, and elevate the quality of their design work.
How AI Image Generator Tools Work
AI image generator tools leverage deep learning algorithms and neural networks to analyze and synthesize visual data. These algorithms are trained on vast amounts of image data to learn patterns, styles, and features, allowing them to generate realistic and visually appealing images. Depending on the specific tool and its capabilities, users can interact with the AI model through various input methods such as textual descriptions, image editing interfaces, or preset parameters. The AI then processes the input data and produces an output image that meets the desired criteria, whether it's mimicking a particular art style, removing unwanted elements, or generating entirely new visuals from scratch.
Benefits of Using AI Image Generator Tools
The utilization of AI image generator tools offers numerous benefits for creators and businesses alike:
● Time Efficiency: AI-powered image generation significantly reduces the time and effort required to create high-quality visuals, allowing users to focus on other aspects of their projects.
● Cost-effectiveness: By automating the image generation process, AI tools can help businesses save on production costs associated with hiring designers or purchasing stock images.
● Versatility and Customization: AI image generator tools offer a wide range of customization options, allowing users to tailor the output to their specific needs and preferences.
● Quality and Consistency: AI algorithms are capable of producing consistently high-quality images with minimal variations, ensuring a professional and polished look across all visuals.
Use Cases and Applications
The versatility of AI image generator tools makes them valuable assets across various industries and applications:
● Marketing and Advertising: AI-generated visuals can be used to create eye-catching advertisements, promotional materials, and social media content that resonate with target audiences.
● Graphic Design and Branding: Designers can leverage AI tools to explore new styles, experiment with different concepts, and create cohesive branding assets for businesses and organizations.
● E-commerce and Product Visualization: AI-generated images enable online retailers to showcase products from multiple angles, customize product variations, and enhance the overall shopping experience for customers.
● Social Media Content Creation: Influencers, content creators, and social media marketers can use AI image generator tools to produce engaging visuals that drive engagement and foster brand awareness.
● Educational Resources: Teachers and educators can leverage AI-generated visuals to create interactive learning materials, illustrate complex concepts, and enhance classroom presentations.
Tips for Effective Utilization of AI Image Generator Tools
To maximize the benefits of AI image generator tools, consider the following tips:
● Understand Your Audience and Brand: Tailor your visuals to resonate with your target audience and align with your brand identity and messaging.
● Experimentation and Iteration: Don't be afraid to explore different styles, techniques, and parameters to discover unique and compelling visual solutions.
● Combine AI-generated Content with Human Creativity: While AI can automate certain aspects of image generation, human creativity, and intuition play a crucial role in refining and enhancing the final output.
● Quality Control and Review Processes: Regularly review and evaluate the output of AI-generated images to ensure accuracy, relevance, and adherence to quality standards.
Potential Challenges and Limitations
Despite their numerous benefits, AI image generator tools also present certain challenges and limitations:
● Over-reliance on AI: Relying too heavily on AI-generated visuals may lead to a lack of originality and creativity, diminishing the authenticity of the content.
● Copyright and Intellectual Property Concerns: Users must be mindful of copyright issues when using AI tools to generate and manipulate images, especially when dealing with copyrighted material or third-party content.
● Learning Curve and Technical Skill Requirements: Some AI image generator tools may have a steep learning curve and require a basic understanding of AI concepts and techniques to effectively utilize them.
Future Trends in AI Image Generation
Looking ahead, several trends are shaping the future of AI image generation:
● Advancements in Generative Adversarial Networks (GANs): Continued research and development in GAN technology are expected to yield more sophisticated and realistic image generation capabilities.
● Integration with Augmented Reality (AR) and Virtual Reality (VR): AI-generated visuals will play a key role in enhancing immersive AR and VR experiences, blurring the lines between virtual and physical reality.
● Ethical Considerations and Regulations: As AI image generation becomes more prevalent, there will be a growing need for ethical guidelines and regulatory frameworks to govern its use and mitigate potential risks and abuses.
Conclusion
AI image generator tools offer unprecedented opportunities for creators, businesses, and industries to elevate their visual content and unlock new creative possibilities. By leveraging the power of AI algorithms and neural networks, users can streamline the image generation process, produce high-quality visuals, and enhance engagement with their target audience. As technology continues to evolve, free AI image generator will undoubtedly play a central role in shaping the future of visual content creation across various domains. So why wait? Explore the top 10 AI image generator tools mentioned in this guide and take your visual content to the next level today!
A Simple Way to Create Better Images
#ai image generator#free ai image generator#ai image generator free#text to image ai#free text to image ai#free ai image generator from text
0 notes
Text
IEEE Transactions on Fuzzy Systems, Volume 33, Issue 1, January 2025
1) Guest Editorial Special Section on Fuzzy-Deep Neural Network Learning in Sentiment Analysis
Author(s): Gautam Srivastava, Chun-Wei Lin
Pages: 1 - 2
2) Fcdnet: Fuzzy Cognition-Based Dynamic Fusion Network for Multimodal Sentiment Analysis
Author(s): Shuai Liu, Zhe Luo, Weina Fu
Pages: 3 - 14
3) Joint Objective and Subjective Fuzziness Denoising for Multimodal Sentiment Analysis
Author(s): Xun Jiang, Xing Xu, Huimin Lu, Lianghua He, Heng Tao Shen
Pages: 15 - 27
4) Exploring Multimodal Multiscale Features for Sentiment Analysis Using Fuzzy-Deep Neural Network Learning
Author(s): Xin Wang, Jianhui Lyu, Byung-Gyu Kim, B. D. Parameshachari, Keqin Li, Qing Li
Pages: 28 - 42
5) Depression Detection From Social Media Posts Using Emotion Aware Encoders and Fuzzy Based Contrastive Networks
Author(s): Sunder Ali Khowaja, Lewis Nkenyereye, Parus Khuwaja, Hussam Al Hamadi, Kapal Dev
Pages: 43 - 53
6) EMSIN: Enhanced Multistream Interaction Network for Vehicle Trajectory Prediction
Author(s): Yilong Ren, Zhengxing Lan, Lingshan Liu, Haiyang Yu
Pages: 54 - 68
7) FICformer: A Multi-factor Fuzzy Bayesian Imputation Cross-former for Big Data-driven Agricultural Decision Support Systems
Author(s): Jianlei Kong, Xiaomeng Fan, Min Zuo, Wenjing Yan, Xuebo Jin
Pages: 69 - 81
8) ViTDFNN: A Vision Transformer Enabled Deep Fuzzy Neural Network for Detecting Sleep Apnea-Hypopnea Syndrome in the Internet of Medical Things
Author(s): Na Ying, Hongyu Li, Zhi Zhang, Yong Zhou, Huahua Chen, Meng Yang
Pages: 82 - 93
9) A Novel Centralized Federated Deep Fuzzy Neural Network with Multi-objectives Neural Architecture Search for Epistatic Detection
Author(s): Xiang Wu, Yong-Ting Zhang, Khin-Wee Lai, Ming-Zhao Yang, Ge-Lan Yang, Huan-Huan Wang
Pages: 94 - 107
10) A Comprehensive Adaptive Interpretable Takagi–Sugeno–Kang Fuzzy Classifier for Fatigue Driving Detection
Author(s): Dongrui Gao, Shihong Liu, Yingxian Gao, Pengrui Li, Haokai Zhang, Manqing Wang, Shen Yan, Lutao Wang, Yongqing Zhang
Pages: 108 - 119
11) A Temporal Multi-View Fuzzy Classifier for Fusion Identification on Epileptic Brain Network
Author(s): Zhengxin Xia, Wei Xue, Jia Zhai, Ta Zhou, Chong Su
Pages: 120 - 130
12) Structured Sparse Regularization-Based Deep Fuzzy Networks for RNA N6-Methyladenosine Sites Prediction
Author(s): Leyao Wang, Yuqing Qian, Hao Xie, Yijie Ding, Fei Guo
Pages: 131 - 144
13) Data-Driven Fuzzy Sliding Mode Observer-Based Control Strategy for Time-Varying Suspension System of 12/14 Bearingless SRM
Author(s): Ye Yuan, Kai Xie, Wen Ji, Yougang Sun, Fan Yang, Yu Nan
Pages: 145 - 155
14) Fusion of Explainable Deep Learning Features Using Fuzzy Integral in Computer Vision
Author(s): Yifan Wang, Witold Pedrycz, Hisao Ishibuchi, Jihua Zhu
Pages: 156 - 167
15) FMFN: A Fuzzy Multimodal Fusion Network for Emotion Recognition in Ensemble Conducting
Author(s): Xiao Han, Fuyang Chen, Junrong Ban
Pages: 168 - 179
16) MFVAE: A Multiscale Fuzzy Variational Autoencoder for Big Data-Based Fault Diagnosis in Gearbox
Author(s): He-xuan Hu, Yicheng Cai, Qing Meng, Han Cui, Qiang Hu, Ye Zhang
Pages: 180 - 191
17) A Dissimilarity Measure Powered Feature Weighted Fuzzy C-Means Algorithm for Gene Expression Data
Author(s): Ning Ma, Qinghua Hu, Kaijun Wu, Yubin Yuan
Pages: 192 - 202
18) End-Edge Collaborative Inference of Convolutional Fuzzy Neural Networks for Big Data-Driven Internet of Things
Author(s): Yuhao Hu, Xiaolong Xu, Li Duan, Muhammad Bilal, Qingyang Wang, Wanchun Dou
Pages: 203 - 217
19) A Hybrid Fuzzy C-Means Heuristic Approach for Two-Echelon Vehicle Routing With Simultaneous Pickup and Delivery of Multicommodity
Author(s): Heng Wang, Sihao Chen, Xiaoyi Yin, Lingxi Meng, Zhanwu Wang, Zhenfeng Wang
Pages: 218 - 230
20) Fuzzy-ViT: A Deep Neuro-Fuzzy System for Cross-Domain Transfer Learning From Large-Scale General Data to Medical Image
Author(s): Qiankun Li, Yimou Wang, Yani Zhang, Zhaoyu Zuo, Junxin Chen, Wei Wang
Pages: 231 - 241
21) Multiobjective Evolution of the Deep Fuzzy Rough Neural Network
Author(s): Jianwei Zhao, Dingjun Chang, Bin Cao, Xin Liu, Zhihan Lyu
Pages: 242 - 254
22) Boosting Robustness in Deep Neuro-Fuzzy Systems: Uncovering Vulnerabilities, Empirical Insights, and a Multiattack Defense Mechanism
Author(s): Jia Wang, Weilong Zhang, Zushu Huang, Jianqiang Li
Pages: 255 - 266
23) A Fuzzy-Operated Convolutional Autoencoder for Classification of Wearable Device-Collected Electrocardiogram
Author(s): Lumin Xing, Xin Li, Wenjian Liu, Xing Wang
Pages: 267 - 277
24) Quantum Fuzzy Federated Learning for Privacy Protection in Intelligent Information Processing
Author(s): Zhiguo Qu, Lailei Zhang, Prayag Tiwari
Pages: 278 - 289
25) Deep Spatio-Temporal Fuzzy Model for NDVI Forecasting
Author(s): Zhao Su, Jun Shen, Yu Sun, Rizhen Hu, Qingguo Zhou, Binbin Yong
Pages: 290 - 301
26) Composite Neuro-Fuzzy System-Guided Cross-Modal Zero-Sample Diagnostic Framework Using Multisource Heterogeneous Noncontact Sensing Data
Author(s): Sheng Li, Jinchen Ji, Ke Feng, Ke Zhang, Qing Ni, Yadong Xu
Pages: 302 - 313
27) Exploring Zadeh's General Type-2 Fuzzy Logic Systems for Uncertainty Quantification
Author(s): Yusuf Güven, Ata Köklü, Tufan Kumbasar
Pages: 314 - 324
28) VL-MFER: A Vision-Language Multimodal Pretrained Model With Multiway-Fuzzy-Experts Bidirectional Retention Network
Author(s): Chen Guo, Xinran Li, Jiaman Ma, Yimeng Li, Yuefan Liu, Haiying Qi, Li Zhang, Yuhan Jin
Pages: 325 - 337
29) Temporal-Spatial Fuzzy Deep Neural Network for the Grazing Behavior Recognition of Herded Sheep in Triaxial Accelerometer Cyber-Physical Systems
Author(s): Shuwei Hou, Tianteng Wang, Di Qiao, David Jingjun Xu, Yuxuan Wang, Xiaochun Feng, Waqar Ahmed Khan, Junhu Ruan
Pages: 338 - 349
30) FRCNN: A Combination of Fuzzy-Rough-Set-Based Feature Discretization and Convolutional Neural Network for Segmenting Subretinal Fluid Lesions
Author(s): Qiong Chen, Lirong Zeng, Weiping Ding
Pages: 350 - 364
31) Learning Fuzzy Label-Distribution-Specific Features for Data Processing
Author(s): Xin Wang, J. Dinesh Peter, Adam Slowik, Fan Zhang, Xingsi Xue
Pages: 365 - 376
32) Deep Reinforcement Learning With Fuzzy Feature Fusion for Cooperative Control in Traffic Light and Connected Autonomous Vehicles
Author(s): Liang Xu, Zhengyang Zhang, Han Jiang, Bin Zhou, Haiyang Yu, Yilong Ren
Pages: 377 - 391
33) A Fuzzy Neural Network Enabled Deep Subspace Domain Adaptive Fusion Approaches for Facial Expression Recognition
Author(s): Wanneng Shu, Feng Zhang, Runze Wan
Pages: 392 - 405
34) Hybrid Model Integrating Fuzzy Systems and Convolutional Factorization Machine for Delivery Time Prediction in Intelligent Logistics
Author(s): Delong Zhu, Zhong Han, Xing Du, Dafa Zuo, Liang Cai, Changchun Xue
Pages: 406 - 417
35) Dual Guidance Enabled Fuzzy Inference for Enhanced Fine-Grained Recognition
Author(s): Qiupu Chen, Feng He, Gang Wang, Xiao Bai, Long Cheng, Xin Ning
Pages: 418 - 430
36) Skeleton-Based Gait Recognition Based on Deep Neuro-Fuzzy Network
Author(s): Jiefan Qiu, Yizhe Jia, Xingyu Chen, Xiangyun Zhao, Hailin Feng, Kai Fang
Pages: 431 - 443
37) A Deep Neuro-Fuzzy Method for ECG Big Data Analysis via Exploring Multimodal Feature Fusion
Author(s): Xiaohong Lyu, Shalli Rani, S. Manimurugan, Yanhong Feng
Pages: 444 - 456
38) A Reconstructed UNet Model With Hybrid Fuzzy Pooling for Gastric Cancer Segmentation in Tissue Pathology Images
Author(s): Junjun Huang, Shier Nee Saw, Yanlin Chen, Dongdong Hu, Xufeng Sun, Ning Chen, Loo Chu Kiong
Pages: 457 - 467
39) Odyssey of Interval Type-2 Fuzzy Logic Systems: Learning Strategies for Uncertainty Quantification
Author(s): Ata Köklü, Yusuf Güven, Tufan Kumbasar
Pages: 468 - 478
40) Ensemble Deep Random Vector Functional Link Neural Network Based on Fuzzy Inference System
Author(s): M. Sajid, M. Tanveer, Ponnuthurai N. Suganthan
Pages: 479 - 490
41) Quantum-Assisted Hierarchical Fuzzy Neural Network for Image Classification
Author(s): Shengyao Wu, Runze Li, Yanqi Song, Sujuan Qin, Qiaoyan Wen, Fei Gao
Pages: 491 - 502
42) Deep Graphical and Temporal Neuro-Fuzzy Methodology for Automatic Modulation Recognition in Cognitive Wireless Big Data
Author(s): Xin Jian, Qing Wang, Yaoyao Li, Abdullah Alharbi, Keping Yu, Victor Leung
Pages: 503 - 513
0 notes
Text
The first neural language model, that's Yoshua Bengio, one of the "Godfathers of Deep Learning"! He is widely regarded as one of the most impactful people in natural language processing and unsupervised learning. Here are his main contributions:
- 1994 - Identified the problem of vanishing and exploding gradients in RNN: https://lnkd.in/gNNWDnGG
- 1995 - He applied with Lecun Convolutional Neural Network to the speech and time series learning task: https://lnkd.in/gSJT7rd4
- 1998 - He suggested with LeCun a new CNN architecture (LeNet - Graph Transformer Networks) for the document recognition learning task: https://lnkd.in/gFyijKin
- 2003 - Proposed the first neural language model: https://lnkd.in/gJgngk_K
- 2006 - Proposed a new greedy training method for Deep Networks: https://lnkd.in/g4TfHwKc
- 2008 - Invented the Denoising Autoencoder model: https://lnkd.in/gUvwXGNN
- 2009 Developed a new method called curriculum learning, which is a form of structured learning that gradually exposes models to more difficult examples: https://lnkd.in/gyBirMxN
- 2010 - Proposed a new unsupervised learning technique, Stacked Denoising Autoencoder architecture: https://lnkd.in/gyH5-JTs
- 2010 - Proposed with Glorot the weight initialization technique used in most modern neural nets: https://lnkd.in/g4nqvxzh
- 2011 - Invented the ReLU activation function: https://lnkd.in/gwJsxHYQ
- 2011 - Proposed Bayesian optimization for hyperparameter optimization: https://lnkd.in/geSTQZWU
- 2012 - Proposed the random search technique for hyperparameter optimization: https://lnkd.in/gtx_ABwi
- 2013 - Proposed a unifying perspective on representation learning: https://lnkd.in/gVFU7iUh
- 2013 - Invented the gradient clipping strategy to prevent the vanishing and exploding gradient problem in RNN: https://lnkd.in/gQWkKMYq
- 2013 - Proposed a new activation function for Maxout networks: https://lnkd.in/gWdB72dH
- 2014 - He proposed the RNN encoder-decoder architecture: https://lnkd.in/gnGFsdJe
- 2014 - He proposed with Ian Goodfellow, the Generative Adversarial Networks, a novel class of deep learning models designed to generate synthetic data that resembles real data: https://arxiv.org/pdf/1406.2661.pdf
- 2014 - He proposed the gated recursive convolutional method for machine translation: https://lnkd.in/g-rRQ6km
- 2014 - He invented the Attention mechanism with Bahdanau: https://lnkd.in/g2C3sHjf
- 2014 - Proposed FitNets, a new distillation method to compress Deep NN: https://lnkd.in/gsQy8f8m
- 2014 - Showed the efficacy of transfer learning: https://lnkd.in/g4vfNNUx
- 2015 - Proposed BinaryConnect, a new method for efficient training with binary weights: https://lnkd.in/g-Pg63RT
- 2015 - Proposed a visual Attention mechanism for image caption generation: https://lnkd.in/gbmGDwA2
- 2017 - Proposed a novel architecture with self-attention layers for graph-structured data: https://lnkd.in/gg8tQBtP
- 2017 - Proposed a new convolutional architecture for brain tumor segmentation: https://lnkd.in/gxFuqr53
#machinelearning #datascience #artificialintelligence

0 notes