#Machine Learning Training
Explore tagged Tumblr posts
Text
Machine Learning Training for Non-Tech Professionals: How to Get Started
For non-tech professionals, venturing into machine learning can seem intimidating. Yet, this field is becoming more accessible, presenting exciting opportunities for those ready to tackle new challenges. This guide will help you navigate the transition into machine learning roles, providing you with the essential knowledge and resources to start your journey with confidence. By following these steps, you'll be well on your way to harnessing the power of machine learning to enhance your career.
Understanding the Basics
Before you jump into the deep end, it's essential to grasp the fundamentals of machine learning. Start with understanding what machine learning is: it's a branch of artificial intelligence (AI) that involves training algorithms to learn from data and make predictions or decisions without being explicitly programmed. The core concepts include supervised learning, unsupervised learning, and reinforcement learning. Familiarizing yourself with these basics will provide a solid foundation for further exploration.
Identify Your Goals
Next, define why you want to learn machine learning. Are you looking to enhance your current role, switch careers, or start a new project? Knowing your goals will help you choose the right learning path. For instance, if you're in marketing, you might focus on predictive analytics. If you're in finance, you might be more interested in risk modeling.
Leverage Online Resources
There is a wealth of online resources designed to make machine learning accessible to non-tech professionals. Platforms like Coursera, edX, and Udacity offer introductory courses that cover the basics of machine learning without requiring a deep technical background. Look for courses that offer practical exercises and real-world applications, which can help bridge the gap between theory and practice.
Start with Data Analysis
One of the most crucial aspects of machine learning is data analysis. Learning how to handle and analyze data will make the transition smoother. Tools like Excel, Google Sheets, and basic statistical software are great starting points. Once you're comfortable with data handling, you can move on to more advanced tools like Python or R, which are commonly used in machine learning.
Choose the Right Training Program
For non-tech professionals, choosing a comprehensive and supportive training program is vital. Look for programs that offer structured learning paths, hands-on projects, and personalized support. Accelebrate is a renowned provider in this space, offering a wide range of courses designed to cater to different levels of expertise and industry needs. Their courses are known for their practical approach and expert instructors, making them an excellent choice for those new to the field.
Stay Updated and Keep Learning
Machine learning is a rapidly evolving field, and continuous learning is essential. Stay updated with the latest trends, tools, and technologies by following industry blogs, subscribing to relevant journals, and experimenting with new techniques and algorithms.
In Conclusion
Transitioning into a machine learning role as a non-tech professional is entirely achievable with the right approach and resources. To further accelerate your learning journey, consider enrolling in a course with Accelebrate. Their extensive range of machine learning courses is tailored to help professionals from all backgrounds gain the skills they need to succeed in the world of data and AI.
Embrace the challenge, and you'll find that the world of machine learning is not just for techies—it's for anyone willing to learn and innovate. Happy learning!
For more details, visit: https://www.accelebrate.com/machine-learning-training
2 notes
·
View notes
Text
TOP 10 courses that have generally been in high demand in 2024-
Data Science and Machine Learning: Skills in data analysis, machine learning, and artificial intelligence are highly sought after in various industries.
Cybersecurity: With the increasing frequency of cyber threats, cybersecurity skills are crucial to protect sensitive information.
Cloud Computing: As businesses transition to cloud-based solutions, professionals with expertise in cloud computing, like AWS or Azure, are in high demand.
Digital Marketing: In the age of online businesses, digital marketing skills, including SEO, social media marketing, and content marketing, are highly valued.
Programming and Software Development: Proficiency in programming languages and software development skills continue to be in high demand across industries.
Healthcare and Nursing: Courses related to healthcare and nursing, especially those addressing specific needs like telemedicine, have seen increased demand.
Project Management: Project management skills are crucial in various sectors, and certifications like PMP (Project Management Professional) are highly valued.
Artificial Intelligence (AI) and Robotics: AI and robotics courses are sought after as businesses explore automation and intelligent technologies.
Blockchain Technology: With applications beyond cryptocurrencies, blockchain technology courses are gaining popularity in various sectors, including finance and supply chain.
Environmental Science and Sustainability: Courses focusing on environmental sustainability and green technologies are increasingly relevant in addressing global challenges.
Join Now
learn more -
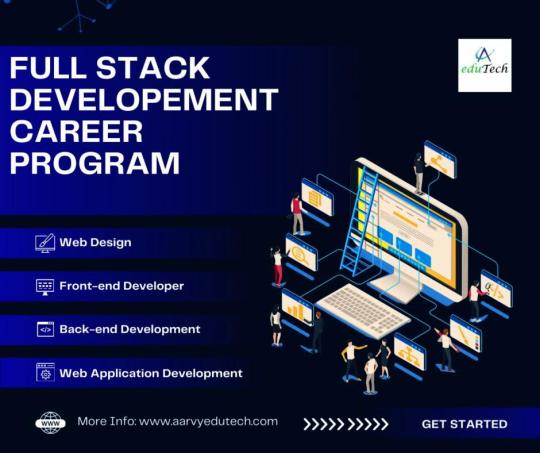
#artificial intelligence#html#coding#machine learning#python#programming#indiedev#rpg maker#devlog#linux#digital marketing#top 10 high demand course#Data Science courses#Machine Learning training#Cybersecurity certifications#Cloud Computing courses#Digital Marketing classes#Programming languages tutorials#Software Development courses#Healthcare and Nursing programs#Project Management certification#Artificial Intelligence courses#Robotics training#Blockchain Technology classes#Environmental Science education#Sustainability courses
2 notes
·
View notes
Text
Machine Learning Projects at Takeoff Projects
Machine Learning is a powerful technology that helps computers learn from data and make intelligent decisions. It is widely used in industries like healthcare, finance, e-commerce, and robotics to improve efficiency and accuracy. As the demand for machine learning experts grows, working on real-world projects has become essential for students and professionals.

At Takeoff Projects, we provide hands-on machine learning projects that help you gain practical experience. Our projects cover various fields such as image recognition, natural language processing, fraud detection, and recommendation systems. By working on these projects, you will learn how to collect and process data, build predictive models, and fine-tune algorithms for better performance.
We offer step-by-step guidance, making it easy for beginners to understand the concepts and implement them. Our projects are designed to give you a deep understanding of machine learning tools and techniques. Whether you want to develop a chatbot, detect fake news, or improve customer recommendations, we have the right project for you.
With the increasing demand for AI and machine learning professionals, having real-world project experience can give you a competitive advantage. At Takeoff Projects, we ensure that you not only learn theoretical concepts but also apply them to solve real problems. Our team provides support at every stage, helping you complete your projects successfully.
If you are looking to build a strong career in machine learning, explore the exciting opportunities at Takeoff Projects today!
#machine learning projects#machine learning training#Takeoff Projects#machine learning internship#ML project ideas
0 notes
Text
Best Coding Bootcamps with AI Curriculum in 2025
The demand for AI skills is skyrocketing, and coding bootcamps have adapted by integrating AI-focused courses into their curricula. Whether you’re a beginner or an experienced developer looking to upskill, enrolling in a bootcamp with AI training can accelerate your career in tech. This article explores the best coding bootcamps that emphasize artificial intelligence, machine learning, and data…
#AI coding bootcamp#best AI bootcamp 2025#learn artificial intelligence#machine learning training#tech bootcamps
0 notes
Text
The Impact of Data Science on AI-Powered Content Platforms Like FanTV

In the ever-evolving digital world, AI-driven content platforms like FanTV are reshaping how audiences discover and engage with media. These platforms rely heavily on data science to enhance user experience, optimize content delivery, and drive engagement. By combining artificial intelligence (AI) with advanced data analytics, FanTV and similar platforms can process massive amounts of data to offer personalized recommendations, refine content discovery, and maximize advertising effectiveness.
What Are AI-Driven Content Platforms?
AI-powered content platforms leverage machine learning (ML), natural language processing (NLP), and deep learning to automate content curation and create immersive experiences. FanTV, for instance, uses AI-based recommendation engines to suggest movies, TV shows, and videos tailored to individual users. By analyzing factors such as watch history, search behaviour, and interaction data, these platforms deliver highly personalized content.
How Data Science Enhances AI-Powered Content Platforms
Data science serves as the backbone of AI-driven content platforms, transforming raw data into meaningful insights. Here’s how it contributes to platforms like FanTV:
1. Personalized Content Recommendations
With the help of data science, platforms create personalized viewing experiences. Techniques like collaborative filtering and content-based filtering analyze user preferences and behavioural patterns to recommend relevant content. AI-powered recommendation engines significantly boost user satisfaction and retention by offering suggestions that align with individual tastes.
2. Understanding User Behaviour
AI-driven content platforms track user interactions, such as viewing history, click-through rates, and time spent on content. Data science helps interpret this data to understand content preferences and optimize user engagement. This insight allows platforms to refine their recommendation models and improve overall user experience.
3. Content Curation and Optimization
Data science plays a key role in dynamically curating content. Machine learning algorithms detect trends, analyze sentiment, and process user-generated content to ensure users see the most relevant media. NLP techniques enable content categorization, tagging, and metadata enhancement, improving discoverability.
4. Audience Sentiment Analysis
Sentiment analysis, powered by data science, allows platforms to gauge audience reactions to specific content by analyzing reviews, comments, and social media discussions. AI algorithms identify patterns in sentiment data, enabling content creators and distributors to align their offerings with audience expectations.
5. Detecting Fraud and Managing Content Moderation
AI-powered platforms are often targeted by fraudulent activities, including fake reviews, bot-driven traffic, and inappropriate content uploads. Data science helps detect and mitigate such risks using anomaly detection and predictive analytics, ensuring a safer and more reliable platform experience.
6. Optimized Advertising and Monetization Strategies
Advertisers rely on AI-driven platforms to reach the right audience. By analyzing user demographics, browsing habits, and interaction trends, data science enables precision-targeted advertisements. This approach enhances user engagement with ads and increases advertising revenue for platforms.
7. Forecasting Content Popularity
Predictive analytics powered by data science helps content platforms anticipate which movies, TV shows, or videos are likely to trend. By analyzing historical performance data, social media buzz, and audience engagement metrics, platforms can make data-driven decisions about content acquisition and promotion.
Upskill with the Boston Institute of Analytics’ Online Data Science Course
For those looking to dive into the world of AI-driven content platforms, mastering data science is essential. The Boston Institute of Analytics (BIA) offers an industry-leading Online Data Science Course designed to equip learners with practical knowledge in:
Data analysis and pre-processing
Machine learning and AI applications
Natural language processing (NLP) for content platforms
Developing recommendation systems
Predictive analytics and audience behaviour modeling
Through hands-on training and real-world case studies, BIA’s program prepares professionals to excel in AI-powered content platforms like FanTV. Whether you're an aspiring data scientist, AI engineer, or digital media expert, this course provides the skills needed to thrive in the evolving digital entertainment space.
READ MORE: FanTV Secures $3 Million Funding to Revolutionize AI-Driven Content Creation
Conclusion
Data science is at the core of AI-driven content platforms like FanTV, enhancing personalized recommendations, user engagement, and monetization strategies. As AI continues to transform digital entertainment, professionals with data science expertise are increasingly in demand. The Boston Institute of Analytics' Online Data Science Course is an excellent stepping stone for those looking to build a career in this space. By developing data-driven skills, individuals can contribute to the future of AI-powered content platforms and revolutionize the way people consume digital media.
#best data science institute#data science course#data science training#AI Training Program#Online Data Science Course#Best Data Science Course#Data Science Program#machine learning training#python training
0 notes
Text
Unlock the potential of your AI models with accurate video transcription services. From precise annotations to seamless data preparation, transcription is essential for scalable AI training.
#video transcription services#video transcription#video data transcription#AI Training#Data Annotation#Accurate Transcription#Dataset Quality#AI Data Preparation#Machine Learning Training#Scalable AI Solutions
0 notes
Text

Looking to master Python and kickstart a career in programming? Enroll in the best Python training in Rajpura with Erginous Technologies, where innovation meets expertise.
You’ll learn Python with hands-on projects and real-world applications, ensuring a practical understanding of concepts. Guided by experienced mentors, our training emphasizes problem-solving, coding efficiency, and industry-relevant skills.
#python training#Python Training in India#machine learning training#industrial training#erginous technologies#professional course#internships#training
0 notes
Text
Data Analytics Training In Marathahalli
Techyse Education in Marathahalli, Bangalore, offers specialized Data Analytics Training in Marathahalli for individuals looking to build expertise in Python, Power BI, and data analysis techniques. Their industry-aligned courses focus on practical learning through real-world projects, ensuring students gain hands-on experience in data manipulation, visualization, and dashboard creation. Whether you are a beginner or an experienced professional, Techyse’s programs are designed to enhance your skill set, making you job-ready for roles in data analytics.
Comprehensive Data Analytics Training in Marathahalli Techyse Education takes pride in delivering high-quality Data Analytics Training in Marathahalli, backed by experienced instructors with deep industry knowledge. The curriculum covers essential tools and techniques, from data wrangling with Python to creating interactive dashboards using Power BI, ensuring students are prepared to meet industry demands. With personalized mentorship, career support, and placement assistance, Techyse provides a well-rounded learning experience. Whether aiming for career growth or a fresh start in data analytics, Techyse Education equips learners with the skills to excel in a competitive job market.
Techyse Education | Data Analyst, Python, Power BI Training in Marathahalli, Bangalore
18, Krishna Summit, 307, 3rd Floor, Aswath Nagar, Next to Canara Bank, Marathahalli, Bangalore, Karnataka 560037
Phone: 098445 14333 Website : https://techyse.in/
Our Google Map Location is : https://maps.app.goo.gl/dLsBM669nKHTutxu9
Follow us: Facebook : https://www.facebook.com/techyse.education/ Twitter: https://x.com/techyse_edu/ Instagram : https://www.instagram.com/techyeseducation/ LinkedIn : https://www.linkedin.com/company/techyse-education/ Youtube: https://www.youtube.com/@TechyseEducation
#Data Analytics Marathahalli#Analytics Training#Data Science Marathahalli#Big Data Training#Data Analytics Course#Learn Data Analytics#Marathahalli Training#Data Science Bangalore#Analytics Experts#Data Analyst Training#Data Skills#Marathahalli Courses#Data Analytics India#Bangalore Training#Data Visualization#SQL Training#Python for Data Analytics#Machine Learning Training#Data Science Bootcamp#Business Intelligence#AI and Analytics#Learn Data Science#Career in Analytics#Analytics Certification#Marathahalli Academy#Data Driven#Advanced Analytics#Real World Analytics#Data Analytics Hub#Data Science Courses Bangalore
0 notes
Text
Machine Learning Fundamentals for Data Analysis
An Overview of Machine Learning and Its Application to Data Analytics
Machine learning (ML) has developed as a key component of data analytics, providing powerful tools and approaches for extracting meaningful patterns and insights from massive volumes of data. At its core, machine learning is a subset of artificial intelligence (AI) that focuses on creating algorithms that can learn from and predict data. This capacity is becoming increasingly important as organizations from diverse industries strive to use data-driven decision-making processes to improve efficiency, optimize operations, and gain a competitive advantage.
Machine learning's importance to data analytics stems from its capacity to automate and increase the accuracy of data analysis processes. Traditional statistical methods, while effective, frequently require pre existing models and assumptions about the data. In contrast, machine learning algorithms can adapt and evolve as they are exposed to new data, revealing previously unknown patterns and relationships. This versatility makes machine learning especially useful for dealing with complicated and high-dimensional datasets that are common in modern data analytics.
Introduction to Supervised and Unsupervised Learning
Machine learning spans a wide range of learning paradigms, with supervised and unsupervised learning being two of the most fundamental.
Supervised learning
Supervised learning entails training a model using a labeled dataset, where each training example is associated with an output label. The goal of supervised learning is to create a mapping from inputs to outputs that can accurately predict labels for new, previously unseen data. This paradigm is analogous to learning with a teacher, in which the model is given the correct responses during training.
Typical supervised learning activities include:
Classification: Assigning inputs to pre-established groups. Ascertaining whether or not an email is spam, for example.
Regression: Making continuous value predictions. For instance, estimating home values depending on attributes like size and location.
In supervised learning, popular methods include neural networks, support vector machines (SVM), decision trees, logistic regression, and linear regression. From illness diagnosis in healthcare to fraud detection in finance, these algorithms have many uses.
Unsupervised Learning
On the other hand, unsupervised learning works with unlabeled information. The objective is to deduce the inherent organization found in a collection of data points. The model analyzes the inherent qualities of the data in order to find patterns and relationships without the need for predefined labels.
The key tasks in unsupervised learning include:
Clustering: Combining related data points together. Market segmentation, in marketing, is the grouping of clients with similar purchase behaviors.
Dimensionality Reduction: Reducing the amount of random variables being considered. Principal Component Analysis (PCA) is a technique for visualizing high-dimensional data and enhancing computational performance.
Unsupervised learning algorithms include k-means clustering, hierarchical clustering, and Gaussian Mixture Models. These techniques are critical in exploratory data analysis, allowing analysts to find trends and patterns without prior understanding of the data structure.
Conclusion
Understanding the fundamentals of machine learning is critical for anyone working in data analytics. Supervised and unsupervised learning are effective frameworks for modeling and analyzing complicated information, with each having distinct capabilities adapted to specific sorts of challenges. As the volume and complexity of data increases, analysts will need to understand these machine learning approaches in order to glean useful insights and make informed judgements. Data professionals can improve their ability to harness the full potential of their data by incorporating machine learning into their analytical toolkit, resulting in increased creativity and efficiency throughout their organizations.
Are you ready to improve your data analytics skills using machine learning? CACMS Institute in Amritsar offers complete data analytics training. We provide hands-on practical training, flexible scheduling, and an industry-specific curriculum to guarantee that you obtain the information and expertise required to flourish in your career.
Enroll in one of our next batches today to begin your journey to understanding data analytics and machine learning. Contact us at +91 8288040281 or visit CACMS Institute for more information. Don't pass up this opportunity to boost your career with the greatest data analytics training in Amritsar!
#cacms institute#techeducation#machine learning#machine learning course in Amritsar#Machine Learning Training#learn programming#machine learning institute in amritsar#big data analytics#data analysis#data analytics course in Amritsarmachine#machine learning fundamentals#machine learning algorithms
0 notes
Text
What are the Benefits of Using Machine Learning?
Machine Learning is useful for Natural language processing and these algorithms can detect the tone of a message and its topic to understand customer requirements. This technology is capable of recognizing images and classifying them into different categories. Along with this, it can recognize different kinds of objects in an image and even recognize a face. In addition, Machine Learning…

View On WordPress
#machine learning#Machine Learning Course#machine learning online training#machine learning training
0 notes
Text
Supercharge Your Career with Machine Learning Training

Accelebrate’s machine learning training is your path to career success—start today! Learn from experts and gain hands-on experience to unlock your full potential.
For more information visit: https://www.accelebrate.com/machine-learning-training
0 notes
Text
Understanding Machine Learning and Its Importance
Machine learning is a subset of artificial intelligence that empowers computers to learn from data and make predictions or decisions without explicit programming. In this blog post, we'll delve into the basics of machine learning, its significance in today's world, and the importance of machine learning Training in mastering this transformative technology.Machine learning is a branch of artificial intelligence where computers learn from data patterns and make decisions or predictions without explicit programming. It involves algorithms that improve their performance over time through experience and exposure to data. Instead of being explicitly programmed, machine learning algorithms identify patterns and relationships within data to make informed decisions or predictions. This technology is widely used in various applications, including predictive analytics, recommendation systems, and autonomous vehicles, revolutionizing industries and driving innovation.
Understanding Machine Learning:
Machine learning training involves algorithms and models that learn from data patterns to make predictions or decisions. Instead of being explicitly programmed, these algorithms improve their performance over time through experience and exposure to data.
Types of Machine Learning
Supervised Learning: Algorithms learn from labeled data, making predictions or decisions based on input-output pairs.
Unsupervised Learning: Algorithms identify patterns and structures in unlabeled data without explicit guidance.
Reinforcement Learning: Agents learn to make sequential decisions by interacting with an environment and receiving feedback.
How Machine Learning Works
Data Collection: Relevant data is gathered from various sources.
Data Preprocessing: The data is cleaned, normalized, and prepared for analysis.
Model Training: Algorithms are trained on the preprocessed data to learn patterns and relationships.
Model Evaluation: The trained models are evaluated to assess their performance and generalization ability.
Model Deployment: Successful models are deployed in real-world applications to make predictions or decisions.
Importance of Machine Learning Training
Machine learning course is essential for understanding the underlying concepts, algorithms, and techniques used in machine learning. Training programs provide individuals with the knowledge and skills needed to develop and deploy machine learning solutions effectively. Hands-on experience with real-world datasets and projects allows trainees to apply their learning in practical scenarios.
Applications of Machine Learning
Machine learning has diverse applications across industries, including healthcare, finance, e-commerce, transportation, and marketing. Examples include predictive analytics, recommendation systems, fraud detection, autonomous vehicles, and personalized medicine. Machine learning algorithms drive innovation, improve efficiency, and enhance decision-making in various domains.
Why Machine Learning Matters
Machine learning matters because it enables organizations to extract valuable insights from data, automate processes, and make data-driven decisions. By leveraging machine learning, businesses can gain a competitive edge, improve customer experiences, and optimize operations. Moreover, machine learning contributes to scientific research, healthcare advancements, and societal progress. With the growing volume of data and the increasing demand for intelligent systems, machine learning continues to play a pivotal role in shaping the future.
Machine learning is a transformative technology with widespread applications and implications across industries. Understanding the basics of machine learning and undergoing machine learning Training is essential for individuals and organizations seeking to harness its potential. As machine learning continues to evolve and advance, its impact on society, economy, and technology will only continue to grow, making it a critical skill for the future.
youtube
0 notes
Text
HMS: Solving One Healthcare Administrators’ Challenge At A Time
Healthcare administrators play a crucial role in the efficient functioning of healthcare facilities, but they often grapple with challenges that impact patient care and organizational effectiveness. One of the primary hurdles is the overwhelming influx of patients, especially when relying on outdated paper-based systems.
The COVID-19 pandemic had a significant effect on the industry globally and altered the market environment. Nearly half of healthcare administrators’s time is consumed by paperwork, significantly impacting patient care and overall efficiency. Since the manual management of tasks consumes valuable time and increases the risk of errors, the use of an Hospital Management System has saved hospitals on a whole new level.
What is a Hospital Management System (HMS)?
A Hospital Management System is a computer-based solution designed to streamline and enhance healthcare operations, mitigating the burden of manual paperwork for healthcare administrators. HMS facilitates the collection, secure storage, retrieval, and sharing of patient information across the entire hospital network.
A hospital management system can manage a variety of functions to optimize operations, including inventory control, billing, and appointment scheduling in addition to patient registration. Healthcare administrators, including doctors, nurses, technicians, and lab personnel, can quickly access critical data with this integrated ecosystem, which empowers them to make well-informed decisions.
By automating processes, HMS not only reduces administrative tasks but also ensures seamless management of medical records, ultimately improving patient care. The adoption of such systems marks a significant step towards enhancing overall hospital efficiency and delivering optimal healthcare services.
Addressing Challenges Faced By Healthcare Administrators through HMS
A hospital management system can help healthcare administrators overcome various challenges through its modules to improve the overall efficiency and effectiveness of healthcare delivery. Below are the healthcare challenges that can be addressed through the implementation of a robust HMS include:
1. Appointment Management
Manually managing appointments can be error-prone and time-consuming. An HMS simplifies the process by offering online appointment scheduling, meeting the preferences of 68% of patients who prefer digital booking. The system efficiently matches patients with relevant specialists, updates real-time slot availability, and facilitates the collection of essential medical documents through a patient portal.
2. Patient Management
The patient management module caters to both inpatient and outpatient needs. It stores comprehensive patient information, including medical history, treatment plans, upcoming appointments, and insurance details. The HMS frees healthcare administrators from having to spend as much time on the tedious paperwork of patients by automating administrative duties.
3. Staff Management
The staff management module provides a centralized solution for HR departments, offering records of staff details, job descriptions, and service domains. This streamlined approach allows hospitals to efficiently plan their hiring processes, ultimately enhancing staff management and organizational efficiency.
4. Supply Management
Timely access to medical supplies is critical for hospitals. The supply management component of the HMS tracks stock availability, records purchase details, and facilitates effective inventory management. This ensures that hospitals can anticipate and address supply needs, preventing shortages that could impact patient care.
5. Financial Management
The financial management component calculates, stores, and presents billing information to patients. Additionally, it records hospital expenses, revenue data, and other financial details. This consolidated approach simplifies financial analysis, saving time and effort by eliminating the need to sift through extensive record books.
6. Insurance Management
The HMS’s insurance management component records and stores patient insurance details, streamlining the hospital insurance validation process. Providing easy access to policy numbers and associated information, this feature ensures a smoother experience for both patients and hospital staff.
7. Laboratory Management
The laboratory management feature of the HMS details various lab tests, furnishing reports promptly, and maintaining comprehensive records. This accessibility allows doctors easy and quick access to relevant information, improving overall efficiency in patient care.
8. Report Management
The report management module records and stores all reports generated by the hospital. Financial reports help analyze performance metrics and business profitability, providing a comparative view over different years. Healthcare dashboards can present this data in a user-friendly format for easy analysis.
9. Vaccination Management
The vaccination management module keeps track of completed or upcoming vaccinations. The system sends timely reminders, books appointments with doctors, and provides parents with all necessary information, ensuring a systematic and organized approach to vaccination schedules.
10. Support Management
Patient satisfaction is a priority, and the support management segment records inquiries, complaints, requests, and feedback. Automating the feedback collection process reduces staff workload, ensuring prompt and appropriate handling of patient concerns.
In conclusion, healthcare administrators face numerous challenges in managing the dynamic environment of healthcare facilities. The adoption of a Hospital Management System emerges as a pivotal solution to overcome these healthcare challenges, streamlining processes, and ultimately delivering better patient care.
Nebel Tech, with its expertise in the healthcare industry, can assist healthcare administrators in developing secure and scalable HMS tailored to their specific needs. Reach out to us for a complimentary assessment and unleash the possibilities of cutting-edge healthcare administration solutions.
#cloud migration services#cloud migration tools#cloud migration solutions#llms#artificial intelligence#healthcare#mobile app development#software apps#software development#machine learning course#machine learning development company#machine learning solutions#machine learning certification#machine learning training#NEW MOBILE APP
0 notes
Text
Machine Learning In Production Bridging Better Tech Worlds

Integration of Machine Learning in Production: The focus is on integrating machine learning into production environments, ensuring seamless deployment and continuous monitoring1.
Development, Training, and Deployment: The process covers development, training, deployment, and continuous monitoring in production environments.
Testing and Integration: Testing and integration of various parts, such as data preparation, feature selection, and model predictions, are essential for ensuring correct functionality.
Performance Testing: Evaluating the speed, scalability, and efficiency of the machine learning model in different scenarios helps fine-tune the model for various use cases.
Containerization and Orchestration: Containerization methods, like Docker, and orchestration tools, such as Kubernetes, facilitate deployment across environments and automate management.
Continuous Deployment: CI/CD pipelines automate the deployment process, enabling efficient and reliable changes to the production environment.
Monitoring and Management: Implementing logging, alerting, and model registry systems promotes transparency, reproducibility, and efficient model management.
https://aitech.studio/aie/machine-learning-in-production/
#machine learning enganeer#machine learning course#machine learning training#machine learning certification#machine learning solutions#mlops
0 notes
Text
Machine Learning Training In Zirakpur at CADL
0 notes
Text
The Beginner's Guide to Machine Learning Training: Everything You Need to Know
Welcome to the exciting world of machine learning! Whether you're a curious beginner or someone looking to dive into the realm of artificial intelligence, understanding the basics of machine learning training is a crucial first step. In this beginner's guide, we'll break down the essentials to help you navigate the complex landscape of machine learning.
Understanding Machine Learning: Machine learning is a subset of artificial intelligence that enables computers to learn and make predictions or decisions without explicit programming. At its core, machine learning involves the use of algorithms and statistical models that allow systems to improve their performance over time.
Types of Machine Learning: There are three main types of machine learning: supervised learning, unsupervised learning, and reinforcement learning. Supervised learning involves training a model on a labeled dataset, unsupervised learning deals with unlabeled data, and reinforcement learning focuses on training models through a system of rewards and punishments.
The Training Process: Machine learning models "learn" through a training process where they are exposed to data, make predictions, and adjust their parameters to minimize errors. This iterative process continues until the model achieves the desired level of accuracy.
Data is King: The quality of the data you feed into your machine learning model is paramount. Clean, relevant, and representative datasets are essential for training accurate models. Preprocessing steps such as cleaning, normalization, and feature engineering play a crucial role in shaping the success of your model.
Choosing the Right Algorithm: Different machine learning algorithms serve various purposes. Choosing the right algorithm depends on the nature of your problem—whether it's a classification, regression, clustering, or other tasks. Popular algorithms include linear regression, decision trees, support vector machines, and neural networks.
Validation and Testing: To ensure the generalization ability of your model, it's essential to split your dataset into training, validation, and testing sets. Training data is used to teach the model, the validation set helps fine-tune parameters, and the testing set evaluates the model's performance on new, unseen data.
Model Evaluation Metrics: Determining the success of your model involves using appropriate evaluation metrics. Accuracy, precision, recall, F1 score, and area under the ROC curve are common metrics used to assess model performance, depending on the type of problem you are solving.
Conclusion:
Embarking on the journey of machine learning training is an exhilarating venture. Equipped with a solid foundation in the fundamentals, you are now better prepared to delve into the expansive realm of artificial intelligence. Enter SkillIQ's Machine Learning Training – your dedicated pathway to mastering the art of AI. Specifically designed for beginners and those aspiring to become adept data scientists, this course encompasses the essential elements of machine learning.
#artificial intelligence course in ahmedabad#artificial intelligence institute in india#machine learning course#machine learning certification#machine learning courses#machine learning training#ml course#machine learning syllabus#machine learning certification course
0 notes