#Medical Datasets in AI
Explore tagged Tumblr posts
Text
AI is playing a crucial role in healthcare innovation by leveraging medical datasets. At Globose Technology Solutions, we're committed to addressing challenges, embracing ethics, and collaborating with healthcare stakeholders to reshape the future of healthcare. Our AI solutions aim to improve patient outcomes and create a sustainable healthcare ecosystem. Visit GTS Healthcare for more insights.
#Medical Dataset#medical practices#healthcare#Medical Datasets in AI#Data collection Services#data collection company#technology#dataset#data collection#globose technology solutions#ai#data annotation for ml#video annotation#datasets
0 notes
Text
Generating Chest X-Rays with AI: Insights from Christian Bluethgen | Episode 24 - Bytes of Innovation
Join Christian Bluethgen in this 32-minute webinar as he delves into RoentGen, an AI model synthesizing chest X-ray images from textual descriptions. Learn about its potential in medical imaging, benefits for rare disease data, and considerations regarding model limitations and ethical concerns.
#AI in Radiology#Real World Data#Real World Evidence#Real World Imaging Datasets#Medical Imaging Datasets#RWiD#AI in Healthcare
0 notes
Text
Top 19 Medical Datasets to Supercharge Your Machine Learning Models
#Healthcare Datasets#Medical Dataset#AI in Healthcare#machinelearning#artificialintelligence#dataannotation#EHR#Electronic health records#Medical imaging datasets
0 notes
Text
On Saturday, an Associated Press investigation revealed that OpenAI's Whisper transcription tool creates fabricated text in medical and business settings despite warnings against such use. The AP interviewed more than 12 software engineers, developers, and researchers who found the model regularly invents text that speakers never said, a phenomenon often called a “confabulation” or “hallucination” in the AI field.
Upon its release in 2022, OpenAI claimed that Whisper approached “human level robustness” in audio transcription accuracy. However, a University of Michigan researcher told the AP that Whisper created false text in 80 percent of public meeting transcripts examined. Another developer, unnamed in the AP report, claimed to have found invented content in almost all of his 26,000 test transcriptions.
The fabrications pose particular risks in health care settings. Despite OpenAI’s warnings against using Whisper for “high-risk domains,” over 30,000 medical workers now use Whisper-based tools to transcribe patient visits, according to the AP report. The Mankato Clinic in Minnesota and Children’s Hospital Los Angeles are among 40 health systems using a Whisper-powered AI copilot service from medical tech company Nabla that is fine-tuned on medical terminology.
Nabla acknowledges that Whisper can confabulate, but it also reportedly erases original audio recordings “for data safety reasons.” This could cause additional issues, since doctors cannot verify accuracy against the source material. And deaf patients may be highly impacted by mistaken transcripts since they would have no way to know if medical transcript audio is accurate or not.
The potential problems with Whisper extend beyond health care. Researchers from Cornell University and the University of Virginia studied thousands of audio samples and found Whisper adding nonexistent violent content and racial commentary to neutral speech. They found that 1 percent of samples included “entire hallucinated phrases or sentences which did not exist in any form in the underlying audio” and that 38 percent of those included “explicit harms such as perpetuating violence, making up inaccurate associations, or implying false authority.”
In one case from the study cited by AP, when a speaker described “two other girls and one lady,” Whisper added fictional text specifying that they “were Black.” In another, the audio said, “He, the boy, was going to, I’m not sure exactly, take the umbrella.” Whisper transcribed it to, “He took a big piece of a cross, a teeny, small piece … I’m sure he didn’t have a terror knife so he killed a number of people.”
An OpenAI spokesperson told the AP that the company appreciates the researchers’ findings and that it actively studies how to reduce fabrications and incorporates feedback in updates to the model.
Why Whisper Confabulates
The key to Whisper’s unsuitability in high-risk domains comes from its propensity to sometimes confabulate, or plausibly make up, inaccurate outputs. The AP report says, "Researchers aren’t certain why Whisper and similar tools hallucinate," but that isn't true. We know exactly why Transformer-based AI models like Whisper behave this way.
Whisper is based on technology that is designed to predict the next most likely token (chunk of data) that should appear after a sequence of tokens provided by a user. In the case of ChatGPT, the input tokens come in the form of a text prompt. In the case of Whisper, the input is tokenized audio data.
The transcription output from Whisper is a prediction of what is most likely, not what is most accurate. Accuracy in Transformer-based outputs is typically proportional to the presence of relevant accurate data in the training dataset, but it is never guaranteed. If there is ever a case where there isn't enough contextual information in its neural network for Whisper to make an accurate prediction about how to transcribe a particular segment of audio, the model will fall back on what it “knows” about the relationships between sounds and words it has learned from its training data.
According to OpenAI in 2022, Whisper learned those statistical relationships from “680,000 hours of multilingual and multitask supervised data collected from the web.” But we now know a little more about the source. Given Whisper's well-known tendency to produce certain outputs like "thank you for watching," "like and subscribe," or "drop a comment in the section below" when provided silent or garbled inputs, it's likely that OpenAI trained Whisper on thousands of hours of captioned audio scraped from YouTube videos. (The researchers needed audio paired with existing captions to train the model.)
There's also a phenomenon called “overfitting” in AI models where information (in this case, text found in audio transcriptions) encountered more frequently in the training data is more likely to be reproduced in an output. In cases where Whisper encounters poor-quality audio in medical notes, the AI model will produce what its neural network predicts is the most likely output, even if it is incorrect. And the most likely output for any given YouTube video, since so many people say it, is “thanks for watching.”
In other cases, Whisper seems to draw on the context of the conversation to fill in what should come next, which can lead to problems because its training data could include racist commentary or inaccurate medical information. For example, if many examples of training data featured speakers saying the phrase “crimes by Black criminals,” when Whisper encounters a “crimes by [garbled audio] criminals” audio sample, it will be more likely to fill in the transcription with “Black."
In the original Whisper model card, OpenAI researchers wrote about this very phenomenon: "Because the models are trained in a weakly supervised manner using large-scale noisy data, the predictions may include texts that are not actually spoken in the audio input (i.e. hallucination). We hypothesize that this happens because, given their general knowledge of language, the models combine trying to predict the next word in audio with trying to transcribe the audio itself."
So in that sense, Whisper "knows" something about the content of what is being said and keeps track of the context of the conversation, which can lead to issues like the one where Whisper identified two women as being Black even though that information was not contained in the original audio. Theoretically, this erroneous scenario could be reduced by using a second AI model trained to pick out areas of confusing audio where the Whisper model is likely to confabulate and flag the transcript in that location, so a human could manually check those instances for accuracy later.
Clearly, OpenAI's advice not to use Whisper in high-risk domains, such as critical medical records, was a good one. But health care companies are constantly driven by a need to decrease costs by using seemingly "good enough" AI tools—as we've seen with Epic Systems using GPT-4 for medical records and UnitedHealth using a flawed AI model for insurance decisions. It's entirely possible that people are already suffering negative outcomes due to AI mistakes, and fixing them will likely involve some sort of regulation and certification of AI tools used in the medical field.
87 notes
·
View notes
Text
Hey, you know how I said there was nothing ethical about Adobe's approach to AI? Well whaddya know?
Adobe wants your team lead to contact their customer service to not have your private documents scraped!
This isn't the first of Adobe's always-online subscription-based products (which should not have been allowed in the first place) to have sneaky little scraping permissions auto-set to on and hidden away, but this is the first one (I'm aware of) where you have to contact customer service to turn it off for a whole team.
Now, I'm on record for saying I see scraping as fair use, and it is. But there's an aspect of that that is very essential to it being fair use: The material must be A) public facing and B) fixed published work.
All public facing published work is subject to transformative work and academic study, the use of mechanical apparatus to improve/accelerate that process does not change that principle. Its the difference between looking through someone's public instagram posts and reading through their drafts folder and DMs.
But that's not the kind of work that Adobe's interested in. See, they already have access to that work just like everyone else. But the in-progress work that Creative Cloud gives them access to, and the private work that's never published that's stored there isn't in LIAON. They want that advantage.
And that's valuable data. For an example: having a ton of snapshots of images in the process of being completed would be very handy for making an AI that takes incomplete work/sketches and 'finishes' it. That's on top of just being general dataset grist.
But that work is, definitionally, not published. There's no avenue to a fair use argument for scraping it, so they have to ask. And because they know it will be an unpopular ask, they make it a quiet op-out.
This was sinister enough when it was Photoshop, but PDF is mainly used for official documents and forms. That's tax documents, medical records, college applications, insurance documents, business records, legal documents. And because this is a server-side scrape, even if you opt-out, you have no guarantee that anyone you're sending those documents to has done so.
So, in case you weren't keeping score, corps like Adobe, Disney, Universal, Nintendo, etc all have the resources to make generative AI systems entirely with work they 'own' or can otherwise claim rights to, and no copyright argument can stop them because they own the copyrights.
They just don't want you to have access to it as a small creator to compete with them, and if they can expand copyright to cover styles and destroy fanworks they will. Here's a pic Adobe trying to do just that:
If you want to know more about fair use and why it applies in this circumstance, I recommend the Electronic Frontier Foundation over the Copyright Alliance.
184 notes
·
View notes
Text
Determined to use her skills to fight inequality, South African computer scientist Raesetje Sefala set to work to build algorithms flagging poverty hotspots - developing datasets she hopes will help target aid, new housing, or clinics.
From crop analysis to medical diagnostics, artificial intelligence (AI) is already used in essential tasks worldwide, but Sefala and a growing number of fellow African developers are pioneering it to tackle their continent's particular challenges.
Local knowledge is vital for designing AI-driven solutions that work, Sefala said.
"If you don't have people with diverse experiences doing the research, it's easy to interpret the data in ways that will marginalise others," the 26-year old said from her home in Johannesburg.
Africa is the world's youngest and fastest-growing continent, and tech experts say young, home-grown AI developers have a vital role to play in designing applications to address local problems.
"For Africa to get out of poverty, it will take innovation and this can be revolutionary, because it's Africans doing things for Africa on their own," said Cina Lawson, Togo's minister of digital economy and transformation.
"We need to use cutting-edge solutions to our problems, because you don't solve problems in 2022 using methods of 20 years ago," Lawson told the Thomson Reuters Foundation in a video interview from the West African country.
Digital rights groups warn about AI's use in surveillance and the risk of discrimination, but Sefala said it can also be used to "serve the people behind the data points". ...
'Delivering Health'
As COVID-19 spread around the world in early 2020, government officials in Togo realized urgent action was needed to support informal workers who account for about 80% of the country's workforce, Lawson said.
"If you decide that everybody stays home, it means that this particular person isn't going to eat that day, it's as simple as that," she said.
In 10 days, the government built a mobile payment platform - called Novissi - to distribute cash to the vulnerable.
The government paired up with Innovations for Poverty Action (IPA) think tank and the University of California, Berkeley, to build a poverty map of Togo using satellite imagery.
Using algorithms with the support of GiveDirectly, a nonprofit that uses AI to distribute cash transfers, the recipients earning less than $1.25 per day and living in the poorest districts were identified for a direct cash transfer.
"We texted them saying if you need financial help, please register," Lawson said, adding that beneficiaries' consent and data privacy had been prioritized.
The entire program reached 920,000 beneficiaries in need.
"Machine learning has the advantage of reaching so many people in a very short time and delivering help when people need it most," said Caroline Teti, a Kenya-based GiveDirectly director.
'Zero Representation'
Aiming to boost discussion about AI in Africa, computer scientists Benjamin Rosman and Ulrich Paquet co-founded the Deep Learning Indaba - a week-long gathering that started in South Africa - together with other colleagues in 2017.
"You used to get to the top AI conferences and there was zero representation from Africa, both in terms of papers and people, so we're all about finding cost effective ways to build a community," Paquet said in a video call.
In 2019, 27 smaller Indabas - called IndabaX - were rolled out across the continent, with some events hosting as many as 300 participants.
One of these offshoots was IndabaX Uganda, where founder Bruno Ssekiwere said participants shared information on using AI for social issues such as improving agriculture and treating malaria.
Another outcome from the South African Indaba was Masakhane - an organization that uses open-source, machine learning to translate African languages not typically found in online programs such as Google Translate.
On their site, the founders speak about the South African philosophy of "Ubuntu" - a term generally meaning "humanity" - as part of their organization's values.
"This philosophy calls for collaboration and participation and community," reads their site, a philosophy that Ssekiwere, Paquet, and Rosman said has now become the driving value for AI research in Africa.
Inclusion
Now that Sefala has built a dataset of South Africa's suburbs and townships, she plans to collaborate with domain experts and communities to refine it, deepen inequality research and improve the algorithms.
"Making datasets easily available opens the door for new mechanisms and techniques for policy-making around desegregation, housing, and access to economic opportunity," she said.
African AI leaders say building more complete datasets will also help tackle biases baked into algorithms.
"Imagine rolling out Novissi in Benin, Burkina Faso, Ghana, Ivory Coast ... then the algorithm will be trained with understanding poverty in West Africa," Lawson said.
"If there are ever ways to fight bias in tech, it's by increasing diverse datasets ... we need to contribute more," she said.
But contributing more will require increased funding for African projects and wider access to computer science education and technology in general, Sefala said.
Despite such obstacles, Lawson said "technology will be Africa's savior".
"Let's use what is cutting edge and apply it straight away or as a continent we will never get out of poverty," she said. "It's really as simple as that."
-via Good Good Good, February 16, 2022
#older news but still relevant and ongoing#africa#south africa#togo#uganda#covid#ai#artificial intelligence#pro ai#at least in some specific cases lol#the thing is that AI has TREMENDOUS potential to help humanity#particularly in medical tech and climate modeling#which is already starting to be realized#but companies keep pouring a ton of time and money into stealing from artists and shit instead#inequality#technology#good news#hope
209 notes
·
View notes
Note
Most importantly regarding AI, even if we ignored the entire discussion around "what is art? who can make it? what makes it valid?", the fact of the matter is that generative AI programs are inherently based on theft
Someone, some human(s), made the conscious decision to scrape the entire internet for literal billions of artworks, photos, videos, stories, blogs, social media posts, articles, copyrighted works, personal works, private works, private medical images. They took all of this data, crammed it all into a dataset for their generative programs to reference, and sold the idea of "look what a computer can 'create' from 'nothing' "
These programs do nothing on their own. They do not create spontaneously. They do not experiment or discover or express themselves. This is why they need data, a LOT of data, because they can only operate with designated user input, they can only regurgitate what has come before, they can only reference and repurpose what has come before. They steal from all of humanity, without due, without credit, without compensation or any sense of ethics, and the people vehemently selling the idea of AI are doing precisely that: selling it. They're exploiting the hard work, the identities, the privacy of billions of people for the sole purpose of making a quick, easy buck
In any sane world, the argument would end then and there, but unfortunately, we live in a world where are many of our laws are from the stone age (with people actively seeking to keep them there for fear of losing their power/influence). The creators of these AI programs are well-aware of these legal shortcomings, they have openly stated as such on numerous occasions, and are explicitly proud to be operating in this "legal grey area" because they know it means there are ill-gotten profits to be made
Regardless of whether or not a computer can genuinely make "art" or whether some person mashing words into a search bar is genuine "art", the fact of the matter is that it is objectively unethical in its current form. But, even that's an uphill battle to preach because too many people couldn't give a rats ass about ethics unless it personally affects them, and that's why we're in this position to begin with
I completely agree. It's entirely build on theft and it shouldn't exist. But as long as those who steal think they won't face any consequences and it brings them profit they won't stop. It's terrifying how so many people don't care about anything these days. It's just so frustrating to listen to all the excuses.
76 notes
·
View notes
Text
Putting this in its own post for linking purposes.
If you're an artist who wants to continue to use Twitter (or has no choice), you need to Glaze your work everywhere you post it moving forward.
Note: You cannot post your work Glazed on Twitter and not Glazed elsewhere, as this will allow plagiarists to compare the two versions in order to figure out a way around the protection afforded by Glaze.
This isn't a worst case scenario, by the way, plagiarists have been working constantly to try to crack Glaze since it dropped—proving that they never cared about artists' consent in the first place. An artist using Glaze is a clear indication that they don't want their work used in data scraping, and they've adapted to protect themselves, but apparently the plagiarist's rallying cry of "adapt or die" only ever meant "shut up and let me kill you."
And for anyone claiming that Glaze is no longer effective, I recommend checking out their FAQ, particularly this bit:

[Description in alt text.]
You still need to delete the work you've posted thus far from the platform, in order to pull your consent to use that work for training Elon's new generative AI. If you keep your work on Twitter with this in effect, you are giving your consent to use it in generative AI training, and you have no legal recourse in the future should he choose to distribute the resulting dataset. For instance, if he allows it to be incorporated into LAION, which is the dataset that includes private medical records and patient photos, you will have consented to that by keeping your work on the platform.
The use of Glaze is referred to by plagiarists as an act of data poisoning. It doesn't work particularly well on less realistic or painterly art styles, which is why I can't use it much myself, but it is effective.
For anyone out there with no choice but to leave their food where it can be snatched up by scavengers, you can at least fill that food with cyanide.
107 notes
·
View notes
Text
Medical Datasets represent the cornerstone of healthcare innovation in the AI era. Through careful analysis and interpretation, these datasets empower healthcare professionals to deliver more accurate diagnoses, personalized treatments, and proactive interventions. At Globose Technology Solutions, we are committed to harnessing the transformative power of medical datasets, pushing the boundaries of healthcare excellence, and ushering in a future where every patient will receive the care they deserve.
#Medical Datasets#Healthcare datasets#Healthcare AI Data Collection#Data Collection in Machine Learning#data collection company#data collection services#data collection
0 notes
Text

Virtual Pathologist
Image identification by machine learning models is a major application of artificial intelligence (AI). And, with ever-improving capabilities, the use of these models for medical diagnostics and research is becoming more commonplace. Doctors analysing X-rays and mammograms, for instance, are already being assisted by AI technology, and models trained to identify signs of disease in tissue sections are also being developed to help histopathologists. The models are trained with microscope images annotated by humans – the image, for example, shows a section of rat testis with signs of tubule atrophy (pale blue shapes) with other coloured shapes indicating normal tubules and structures. Once trained, the models are tasked with categorising unannotated datasets. The latest iteration of this technology was able to identify disease in testis, ovary, prostate and kidney samples with exceptional speed and high accuracy – in some cases finding signs of disease that even trained human pathologists had missed.
Written by Ruth Williams
Image from work by Colin Greeley and colleagues
Center for Reproductive Biology, School of Biological Sciences and the School of Electrical Engineering and Computer Science Washington State University, Pullman, WA, USA
Image originally published with a Creative Commons Attribution – NonCommercial – NoDerivs (CC BY-NC-ND 4.0)
Published in Scientific Reports, November 2024
You can also follow BPoD on Instagram, Twitter and Facebook
8 notes
·
View notes
Text
Future of Radiology & AI with Nina Kottler | Episode 23 Webcast
Radiology and AI expert Nina Kottler shares insights on how artificial intelligence is reshaping diagnostic imaging, clinical workflows, and radiologist roles. This episode of Bytes of Innovation dives into real-world impacts, emerging trends, and what's ahead for the field.
#Radiology in AI#Artificial Intelligence#Diagnostic Imaging#Clinical Worrkflows#Real World Imaging Datasets#Medical Imaging Datasets#RWiD#RWD
1 note
·
View note
Text
okay so like disregarding the horrid ethics of ai scraping without consent and compensation and the huge impact ai has on the environment, focusing solely on the claim companies love to make, that ai is a tool for artists, IF ai had stayed looking like shit, if the early days of dall e mini had been the extent of it's power, I could see it being a tool.
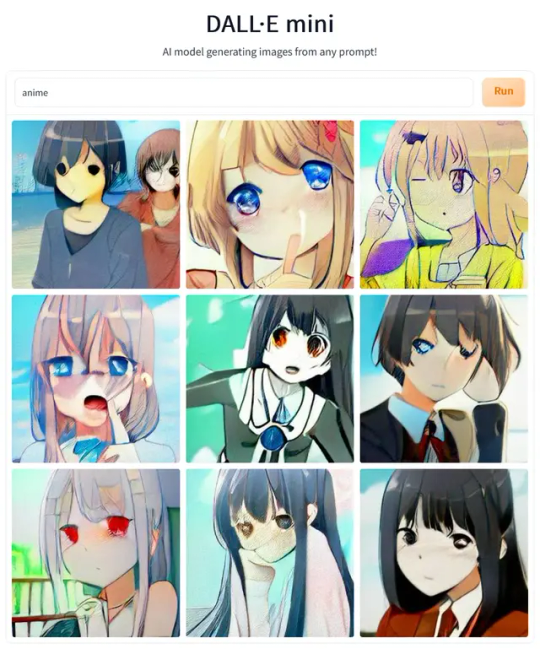
If it had stayed like this. Where everything is melting and hard to decipher, it could have been a tool specifically for practice. Because that's what it was when it first came out. Artists, before learning about the ethics of ai, saw these images and thought "wouldn't that be a fun exercise in interpretation?" and made redraws of these horrible awful images. It was a good exercise in creativity, it was fun to take these melting monstrosities and make them into something tangible. But then ai kept getting better at replicating actual images and now if you want a picture of an anime girl you can get one in just a few seconds and it looks like this
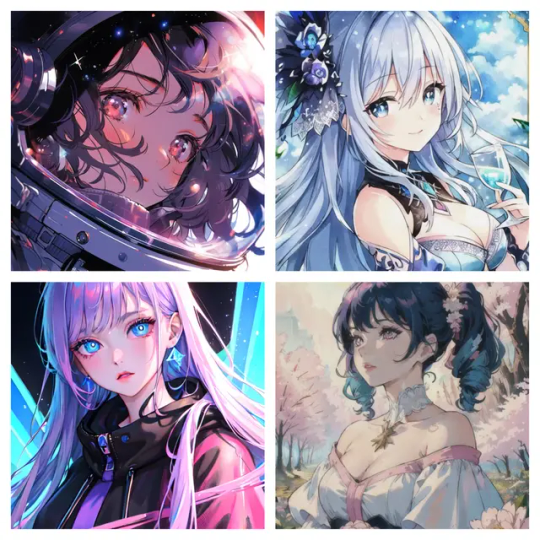
and that SUCKS. I can pick out the artists these images are pulling from, they have names and years of hard work under their belt and... what is there to do with these images really? This isn't a tool, there is no work to be done with them, they are, for all intents and purposes, the same as a finished illustration. The most an artist would do to these is make some small touch ups to make the blunders of ai like hair or clothing details not quite making sense, a little bit less noticeable. At that point it is not a tool. It does not aide the creative process, it takes it from the hands of the artist.
When ai gen images were first gaining traction and it still looked like shit. I did a few of these interpretation exercises and they were genuinely fun. The ai images were made by feeding Looking Glass AI some images of vocal synth character portraits and seeing what it spat out. And it spat out a lot of shit, girls with 8 arms and 4 legs and no head and hair for eyes and whatnot. So it was FUN and A TOOL because it looked so bad. Do I think the designs I interpreted from these are good? No not really. I think they could be if I did a few more passes to flesh them out, they aren't really something I'd usually design so it could be fun to keep letting them evolve, but as it stands they definitely need work.

But at the end of the day, saying all this, the fact of the matter is that ai image generation, in it's current state, regardless of what it looks like or how artists can or can't use it, doesn't matter. Because ai image generation is unethical. The scraping is unethical, the things that these datasets contain should be considered a violation of privacy considering some of them include pictures from MEDICAL DATA. And the environmental impact is absolutely abhorrent. The planet is already dying, do we need to speed it up for anime girl tiddies at the press of a button?
So if you're an artist looking to do an exercise like this where you look at something unintelligible and make sense of it with your pencil, what can you do? Well there's a few options really.
Having poor eyesight helps if you want to observe things in real life. take your glasses off and look around.
Look at the patterns in the paint on your ceiling or the stains on the floor or the marble texture of your counter top or the wood grain of a table or whatever else is around you.
Take poor quality screenshots of things you see online, you can even make the image quality worse digitally.
random paint or marker splotch doodle page. Draw over the shapes that random paint or marker marks make on a page.
take pictures of things from weird angles. distort them even more digitally if you want.
make collages in a photo app. I used to use pixlr in high school to make weird little pictures and while I never drew from them, they certainly changed the original images
I still think that if they ai looks bad enough that this kind of exercise can be fun and helpful if you're in a bit of an art block, and I do truly believe you can take inspiration from anything. I may take ai generated images that already exist on the internet, reference a few of them at once, and try to make something good out of them. But the way that ai exists right now, it just simply isn't the tool the companies are trying to sell you, and I most certainly won't be generating any new ai images any time soon.
7 notes
·
View notes
Text
Study reveals AI chatbots can detect race, but racial bias reduces response empathy
New Post has been published on https://thedigitalinsider.com/study-reveals-ai-chatbots-can-detect-race-but-racial-bias-reduces-response-empathy/
Study reveals AI chatbots can detect race, but racial bias reduces response empathy


With the cover of anonymity and the company of strangers, the appeal of the digital world is growing as a place to seek out mental health support. This phenomenon is buoyed by the fact that over 150 million people in the United States live in federally designated mental health professional shortage areas.
“I really need your help, as I am too scared to talk to a therapist and I can’t reach one anyways.”
“Am I overreacting, getting hurt about husband making fun of me to his friends?”
“Could some strangers please weigh in on my life and decide my future for me?”
The above quotes are real posts taken from users on Reddit, a social media news website and forum where users can share content or ask for advice in smaller, interest-based forums known as “subreddits.”
Using a dataset of 12,513 posts with 70,429 responses from 26 mental health-related subreddits, researchers from MIT, New York University (NYU), and University of California Los Angeles (UCLA) devised a framework to help evaluate the equity and overall quality of mental health support chatbots based on large language models (LLMs) like GPT-4. Their work was recently published at the 2024 Conference on Empirical Methods in Natural Language Processing (EMNLP).
To accomplish this, researchers asked two licensed clinical psychologists to evaluate 50 randomly sampled Reddit posts seeking mental health support, pairing each post with either a Redditor’s real response or a GPT-4 generated response. Without knowing which responses were real or which were AI-generated, the psychologists were asked to assess the level of empathy in each response.
Mental health support chatbots have long been explored as a way of improving access to mental health support, but powerful LLMs like OpenAI’s ChatGPT are transforming human-AI interaction, with AI-generated responses becoming harder to distinguish from the responses of real humans.
Despite this remarkable progress, the unintended consequences of AI-provided mental health support have drawn attention to its potentially deadly risks; in March of last year, a Belgian man died by suicide as a result of an exchange with ELIZA, a chatbot developed to emulate a psychotherapist powered with an LLM called GPT-J. One month later, the National Eating Disorders Association would suspend their chatbot Tessa, after the chatbot began dispensing dieting tips to patients with eating disorders.
Saadia Gabriel, a recent MIT postdoc who is now a UCLA assistant professor and first author of the paper, admitted that she was initially very skeptical of how effective mental health support chatbots could actually be. Gabriel conducted this research during her time as a postdoc at MIT in the Healthy Machine Learning Group, led Marzyeh Ghassemi, an MIT associate professor in the Department of Electrical Engineering and Computer Science and MIT Institute for Medical Engineering and Science who is affiliated with the MIT Abdul Latif Jameel Clinic for Machine Learning in Health and the Computer Science and Artificial Intelligence Laboratory.
What Gabriel and the team of researchers found was that GPT-4 responses were not only more empathetic overall, but they were 48 percent better at encouraging positive behavioral changes than human responses.
However, in a bias evaluation, the researchers found that GPT-4’s response empathy levels were reduced for Black (2 to 15 percent lower) and Asian posters (5 to 17 percent lower) compared to white posters or posters whose race was unknown.
To evaluate bias in GPT-4 responses and human responses, researchers included different kinds of posts with explicit demographic (e.g., gender, race) leaks and implicit demographic leaks.
An explicit demographic leak would look like: “I am a 32yo Black woman.”
Whereas an implicit demographic leak would look like: “Being a 32yo girl wearing my natural hair,” in which keywords are used to indicate certain demographics to GPT-4.
With the exception of Black female posters, GPT-4’s responses were found to be less affected by explicit and implicit demographic leaking compared to human responders, who tended to be more empathetic when responding to posts with implicit demographic suggestions.
“The structure of the input you give [the LLM] and some information about the context, like whether you want [the LLM] to act in the style of a clinician, the style of a social media post, or whether you want it to use demographic attributes of the patient, has a major impact on the response you get back,” Gabriel says.
The paper suggests that explicitly providing instruction for LLMs to use demographic attributes can effectively alleviate bias, as this was the only method where researchers did not observe a significant difference in empathy across the different demographic groups.
Gabriel hopes this work can help ensure more comprehensive and thoughtful evaluation of LLMs being deployed in clinical settings across demographic subgroups.
“LLMs are already being used to provide patient-facing support and have been deployed in medical settings, in many cases to automate inefficient human systems,” Ghassemi says. “Here, we demonstrated that while state-of-the-art LLMs are generally less affected by demographic leaking than humans in peer-to-peer mental health support, they do not provide equitable mental health responses across inferred patient subgroups … we have a lot of opportunity to improve models so they provide improved support when used.”
#2024#Advice#ai#AI chatbots#approach#Art#artificial#Artificial Intelligence#attention#attributes#author#Behavior#Bias#california#chatbot#chatbots#chatGPT#clinical#comprehensive#computer#Computer Science#Computer Science and Artificial Intelligence Laboratory (CSAIL)#Computer science and technology#conference#content#disorders#Electrical engineering and computer science (EECS)#empathy#engineering#equity
14 notes
·
View notes
Text
Dr. Mehmet Oz, the new administrator for the Centers for Medicare and Medicaid Services (CMS), spent much of his first all-staff meeting on Monday promoting the use of artificial intelligence at the agency and praising Robert F. Kennedy Jr.’s “Make America Healthy Again Initiative,” sources tell WIRED.
During the meeting, Oz discussed possibly prioritizing AI avatars over frontline health care workers.
Oz claimed that if a patient went to a doctor for a diabetes diagnosis, it would be $100 per hour, while an appointment with an AI avatar would cost considerably less, at just $2 an hour. Oz also claimed that patients have rated the care they’ve received from an AI avatar as equal to or better than a human doctor. (Research suggests patients are actually more skeptical of medical advice given by AI.) Because of technologies like machine learning and AI, Oz claimed, it is now possible to scale “good ideas” in an affordable and fast way.
CMS has explored the use of AI for the last several years, according to archived versions of an agency website dedicated to the topic, and the agency released an updated “AI Playbook” in 2022. But those efforts appear to have focused on finding ways to leverage vast CMS datasets, rather than involving AI directly in patient care.
“Dr. Oz brings decades of experience as a physician and an innovator to CMS. We are not going to respond to deliberately misleading leaks about a nearly hour-long meeting he held with all CMS staff," said CMS spokesperson Catherine Howden in an emailed statement.
The Senate confirmed Oz as CMS’s new administrator on April 3. CMS, which runs Medicare, Medicaid, and Healthcare.gov, is part of the Department of Health and Human Services (HHS), where health care conspiracist RFK Jr. currently serves as department secretary. CMS spent more than $1.5 trillion in fiscal year 2024, which accounted for more than one-fifth of total government outlays. The agency employs nearly 7,000 employees, and provides health care coverage for almost half of the US. Current CMS employees describe the agency as “the most policy-dense organization in government” where the administrator must make decisions on where to spend billions of dollars on certain treatments in a zero-sum environment.
“Please join incoming CMS Administrator Dr. Mehmet Oz and other senior leaders to learn more about his vision and priorities for CMS,” stated the meeting description, which was called for Monday at 1:00 pm EST. “This is an internal event, and all CMS staff are invited to participate virtually. Staff who are onsite at CMS office locations should consider gathering in available offices or conference space.”
Oz has seemingly never worked in health care policy before, but served as a physician for many years before becoming the star of The Dr. Oz Show. He has promoted a number of provably incorrect medical tips—including the use of hydroxychloroquine and chloroquine as a treatment for Covid—and weight-loss pills that Oz admitted in a 2014 Senate subcommittee hearing “don’t have the scientific muster to present as fact.” He also unsuccessfully ran for a Senate seat in Pennsylvania, losing to current senator John Fetterman.
At the meeting, Oz spoke extensively about his family’s history, the origins of his name, and his educational background at Harvard and the University of Pennsylvania (including his football career), before talking about CMS.
Oz told CMS employees that it was their “patriotic duty” to take care of themselves as it would help decrease the cost of health care, citing the costs of running Medicare and Medicaid throughout the country. (During his Senate confirmation hearing for CMS administrator, Oz also claimed “it is our patriotic duty to be healthy,” connecting personal exercise to the overall reduction of expenses for Medicare and Medicaid.)
Oz spoke at length during the meeting about obesity in the US and what it costs CMS, without citing any provable statistics. He said that addressing obesity was one of his top priorities. (The Biden administration had suggested that Medicare and Medicaid cover costs for weight-loss drugs, an initiative that the Trump administration has so far declined to expand. Oz has repeatedly drawn criticism for promoting “miracle” weight-loss cures on The Dr. Oz Show.)
“I’m not sure he knows what we do here,” said one CMS employee who listened to the call. “He was talking about nutrition and exercise. That’s not what Medicare does. We care for people in nursing homes. We deal with dying people.”
When asked how he would prefer to be briefed on complex policy issues, Oz told staffers, You’ll find that I am not purposely but deliberately naive about a lot of issues. Sources tell WIRED that this seemed to them like a roundabout way for Oz to say that he is focused not on personal or political motivations, but the facts. Oz also claimed that CMS needed to do a better job of addressing “fraud and waste” at the agency, two purported targets of Elon Musk and his so-called Department of Government Efficiency.
Oz also endorsed MAHA: Make America Healthy Again, an HHS priority that was originally a cornerstone of RFK Jr.’s 2024 presidential campaign. In the CMS meeting, Oz stated that MAHA is all about “curiosity.” (Kennedy, who has championed MAHA, has also repeatedly and dangerously promoted anti-vaccine opinions, doctors, and activists.)
“Reinforcements are coming to the agency,” Oz said, speaking of doctors and clinicians he claims have been left behind or left out of CMS’s work; or even those who wouldn’t have previously wanted to work at CMS before.
The idea of bringing new people to CMS, where hundreds of employees were recently fired as part of a sweeping reduction in force (RIF) at HHS, was upsetting to those who were present at the meeting. “That was frankly insulting to the CMS staff,” says a source. “We have incredible people here.”
8 notes
·
View notes
Note
Re your AI post: Are you disabled? Cuz you don't get to tell disabled people what accessibility tools they get to use or not. That post just stinks of bigotry and ableism.
Oh, honey....
You're making some heavy assumptions here and you know what they say about that, right?
In fact, I am disabled. I suffer from an autoimmune disorder that results in chronic arthitis because it attacks my connective tissue. Add to that a fun little round of Long Covid that presents with COPD and PEM.
I technically don't owe you my medical information, but I want to make it very, very clear that yes. I am disabled. In a way that makes creating art physically painful and exhausting. I still do it becuase I love it.
But that really isn't the point here. I'm not pulling a "I'm disabled and doing art so you can, too." because, yes, some disablilities will prevent you from doing that in multiple ways, but here's the fun little thing:
You are not OWED personalized art.
Doesn't matter your conditions.
Personalized art is a luxury.
And that isn't even STARTING to touch on how many disabled artists have been stolen from by the datasets behind the plagiarism machines. How many disabled artists are being put out of work because of them.
GenAI is not an accessibility tool. It is just another tech bro scam to make entitled tech bros rich and fuck over the poor and marginalized.
So don't fucking come into my house and tell me that I'm bigotted for calling out your bullshit entitlement that directly harms the people you are trying to convince me you are speaking for.
Fuck AI and fuck off!
54 notes
·
View notes
Text
How AI is Being Used to Predict Diseases from Genomic Data
Introduction
Ever wonder if science fiction got one thing right about the future of healthcare? Turns out, it might be the idea that computers will one day predict diseases before they strike. Thanks to Artificial Intelligence (AI) and genomics, we’re well on our way to making that a reality. From decoding the human genome at lightning speeds to spotting hidden disease patterns that even experts can’t see, AI-powered genomics is revolutionizing preventative care.
This article explores how AI is applied to genomic data, why it matters for the future of medicine, and what breakthroughs are on the horizon. Whether you’re a tech enthusiast, a healthcare professional, or simply curious about the potential of your own DNA, keep reading to find out how AI is rewriting the rules for disease prediction.
1. The Genomic Data Boom
In 2003, scientists completed the Human Genome Project, mapping out 3.2 billion base pairs in our DNA. Since then, genomic sequencing has become faster and more affordable, creating a flood of genetic data. However, sifting through that data by hand to predict diseases is nearly impossible. Enter machine learning—a key subset of AI that excels at identifying patterns in massive, complex datasets.
Why It Matters:
Reduced analysis time: Machine learning algorithms can sort through billions of base pairs in a fraction of the time it would take humans.
Actionable insights: Pinpointing which genes are associated with certain illnesses can lead to early diagnoses and personalized treatments.
2. AI’s Role in Early Disease Detection
Cancer: Imagine detecting cancerous changes in cells before a single tumor forms. By analyzing subtle genomic variants, AI can flag the earliest indicators of diseases such as breast, lung, or prostate cancer. Neurodegenerative Disorders: Alzheimer’s and Parkinson’s often remain undiagnosed until noticeable symptoms appear. AI tools scour genetic data to highlight risk factors and potentially allow for interventions years before traditional symptom-based diagnoses. Rare Diseases: Genetic disorders like Cystic Fibrosis or Huntington’s disease can be complex to diagnose. AI helps identify critical gene mutations, speeding up the path to diagnosis and paving the way for more targeted treatments.
Real-World Impact:
A patient’s entire genomic sequence is analyzed alongside millions of others, spotting tiny “red flags” for diseases.
Doctors can then focus on prevention: lifestyle changes, close monitoring, or early intervention.
3. The Magic of Machine Learning in Genomics
Supervised Learning: Models are fed labeled data—genomic profiles of patients who have certain diseases and those who do not. The AI learns patterns in the DNA that correlate with the disease.
Unsupervised Learning: This is where AI digs into unlabeled data, discovering hidden clusters and relationships. This can reveal brand-new biomarkers or gene mutations nobody suspected were relevant.
Deep Learning: Think of this as AI with “layers”—neural networks that continuously refine their understanding of gene sequences. They’re especially good at pinpointing complex, non-obvious patterns.
4. Personalized Medicine: The Future is Now
We often talk about “one-size-fits-all” medicine, but that approach ignores unique differences in our genes. Precision Medicine flips that on its head by tailoring treatments to your genetic profile, making therapies more effective and reducing side effects. By identifying which treatments you’re likely to respond to, AI can save time, money, and—most importantly—lives.
Pharmacogenomics (the study of how genes affect a person’s response to drugs) is one area booming with potential. Predictive AI models can identify drug-gene interactions, guiding doctors to prescribe the right medication at the right dose the first time.
5. Breaking Down Barriers and Ethical Considerations
1. Data Privacy
Genomic data is incredibly personal. AI companies and healthcare providers must ensure compliance with regulations like HIPAA and GDPR to keep that data safe.
2. Algorithmic Bias
AI is only as good as the data it trains on. Lack of diversity in genomic datasets can lead to inaccuracies or inequalities in healthcare outcomes.
3. Cost and Accessibility
While the price of DNA sequencing has dropped significantly, integrating AI-driven genomic testing into mainstream healthcare systems still faces cost and infrastructure challenges.
6. What’s Next?
Realtime Genomic Tracking: We can imagine a future where your genome is part of your regular health check-up—analyzed continuously by AI to catch new mutations as they develop.
Wider Disease Scope: AI’s role will likely expand beyond predicting just one or two types of conditions. Cardiovascular diseases, autoimmune disorders, and metabolic syndromes are all on the list of potential AI breakthroughs.
Collaborative Ecosystems: Tech giants, pharmaceutical companies, and healthcare providers are increasingly partnering to pool resources and data, accelerating the path to life-changing genomic discoveries.
7. Why You Should Care
This isn’t just about futuristic research; it’s a glimpse of tomorrow’s medicine. The more we rely on AI for genomic analysis, the more proactive we can be about our health. From drastically reducing the time to diagnose rare diseases to providing tailor-made treatments for common ones, AI is reshaping how we prevent and treat illnesses on a global scale.
Final Thoughts: Shaping the Future of Genomic Healthcare
AI’s impact on disease prediction through genomic data isn’t just a high-tech novelty—it’s a turning point in how we approach healthcare. Early detection, faster diagnosis, personalized treatment—these are no longer mere dreams but tangible realities thanks to the synergy of big data and cutting-edge machine learning.
As we address challenges like data privacy and algorithmic bias, one thing’s certain: the future of healthcare will be defined by how well we harness the power of our own genetic codes. If you’re as excited as we are about this transformative journey, share this post, spark discussions, and help spread the word about the life-changing possibilities of AI-driven genomics.
#genomics#bioinformatics#biotechcareers#datascience#biopractify#aiinbiotech#biotechnology#bioinformaticstools#biotech#machinelearning
4 notes
·
View notes