#Real World Imaging Datasets
Explore tagged Tumblr posts
Text
Latest Trends in AI-Driven Medical Imaging within The Radiology Industry
Explore Segmed's Imaging Wire Recap from July 10, 2024, highlighting key trends and insights in medical imaging and AI. Stay updated on how real-world imaging data is transforming healthcare, advancing diagnostics, and supporting innovation across clinical and research environments.
#Medical Imaging Datasets#Real World Imaging Datasets#Real World Data#Real World Evidence#Real World Medical Imaging Datasets
0 notes
Text
📚 A List Of Useful Websites When Making An RPG 📚
My timeloop RPG In Stars and Time is done! Which means I can clear all my ISAT gamedev related bookmarks. But I figured I would show them here, in case they can be useful to someone. These range from "useful to write a story/characters/world" to "these are SUPER rpgmaker focused and will help with the terrible math that comes with making a game".
This is what I used to make my RPG game, but it could be useful for writers, game devs of all genres, DMs, artists, what have you. YIPPEE
Writing (Names)
Behind The Name - Why don't you have this bookmarked already. Search for names and their meanings from all over the world!
Medieval Names Archive - Medieval names. Useful. For ME
City and Town Name Generator - Create "fake" names for cities, generated from datasets from any country you desire! I used those for the couple city names in ISAT. I say "fake" in quotes because some of them do end up being actual city names, especially for french generated ones. Don't forget to double check you're not 1. just taking a real city name or 2. using a word that's like, Very Bad, especially if you don't know the country you're taking inspiration from! Don't want to end up with Poopaville, USA
Writing (Words)
Onym - A website full of websites that are full of words. And by that I mean dictionaries, thesauruses, translators, glossaries, ways to mix up words, and way more. HIGHLY recommend checking this website out!!!
Moby Thesaurus - My thesaurus of choice!
Rhyme Zone - Find words that rhyme with others. Perfect for poets, lyricists, punmasters.
In Different Languages - Search for a word, have it translated in MANY different languages in one page.
ASSETS
In general, I will say: just look up what you want on itch.io. There are SO MANY assets for you to buy on itch.io. You want a font? You want a background? You want a sound effect? You want a plugin? A pixel base? An attack animation? A cool UI?!?!?! JUST GO ON ITCH.IO!!!!!!
Visual Assets (General)
Creative Market - Shop for all kinds of assets, from fonts to mockups to templates to brushes to WHATEVER YOU WANT
Velvetyne - Cool and weird fonts
Chevy Ray's Pixel Fonts - They're good fonts.
Contrast Checker - Stop making your text white when your background is lime green no one can read that shit babe!!!!!!
Visual Assets (Game Focused)
Interface In Game - Screenshots of UI (User Interfaces) from SO MANY GAMES. Shows you everything and you can just look at what every single menu in a game looks like. You can also sort them by game genre! GREAT reference!
Game UI Database - Same as above!
Sound Assets
Zapsplat, Freesound - There are many sound effect websites out there but those are the ones I saved. Royalty free!
Shapeforms - Paid packs for music and sounds and stuff.
Other
CloudConvert - Convert files into other files. MAKE THAT .AVI A .MOV
EZGifs - Make those gifs bigger. Smaller. Optimize them. Take a video and make it a gif. The Sky Is The Limit
Marketing
Press Kitty - Did not end up needing this- this will help with creating a press kit! Useful for ANY indie dev. Yes, even if you're making a tiny game, you should have a press kit. You never know!!!
presskit() - Same as above, but a different one.
Itch.io Page Image Guide and Templates - Make your project pages on itch.io look nice.
MOOMANiBE's IGF post - If you're making indie games, you might wanna try and submit your game to the Independent Game Festival at some point. Here are some tips on how, and why you should.
Game Design (General)
An insightful thread where game developers discuss hidden mechanics designed to make games feel more interesting - Title says it all. Check those comments too.
Game Design (RPGs)
Yanfly "Let's Make a Game" Comics - INCREDIBLY useful tips on how to make RPGs, going from dungeons to towns to enemy stats!!!!
Attack Patterns - A nice post on enemy attack patterns, and what attacks you should give your enemies to make them challenging (but not TOO challenging!) A very good starting point.
How To Balance An RPG - Twitter thread on how to balance player stats VS enemy stats.
Nobody Cares About It But It’s The Only Thing That Matters: Pacing And Level Design In JRPGs - a Good Post.
Game Design (Visual Novels)
Feniks Renpy Tutorials - They're good tutorials.
I played over 100 visual novels in one month and here’s my advice to devs. - General VN advice. Also highly recommend this whole blog for help on marketing your games.
I hope that was useful! If it was. Maybe. You'd like to buy me a coffee. Or maybe you could check out my comics and games. Or just my new critically acclaimed game In Stars and Time. If you want. Ok bye
#reference#tutorial#writing#rpgmaker#renpy#video games#game design#i had this in my drafts for a while so you get it now. sorry its so long#long post
8K notes
·
View notes
Text
I saw a post the other day calling criticism of generative AI a moral panic, and while I do think many proprietary AI technologies are being used in deeply unethical ways, I think there is a substantial body of reporting and research on the real-world impacts of the AI boom that would trouble the comparison to a moral panic: while there *are* older cultural fears tied to negative reactions to the perceived newness of AI, many of those warnings are Luddite with a capital L - that is, they're part of a tradition of materialist critique focused on the way the technology is being deployed in the political economy. So (1) starting with the acknowledgement that a variety of machine-learning technologies were being used by researchers before the current "AI" hype cycle, and that there's evidence for the benefit of targeted use of AI techs in settings where they can be used by trained readers - say, spotting patterns in radiology scans - and (2) setting aside the fact that current proprietary LLMs in particular are largely bullshit machines, in that they confidently generate errors, incorrect citations, and falsehoods in ways humans may be less likely to detect than conventional disinformation, and (3) setting aside as well the potential impact of frequent offloading on human cognition and of widespread AI slop on our understanding of human creativity...
What are some of the material effects of the "AI" boom?
Guzzling water and electricity
The data centers needed to support AI technologies require large quantities of water to cool the processors. A to-be-released paper from the University of California Riverside and the University of Texas Arlington finds, for example, that "ChatGPT needs to 'drink' [the equivalent of] a 500 ml bottle of water for a simple conversation of roughly 20-50 questions and answers." Many of these data centers pull water from already water-stressed areas, and the processing needs of big tech companies are expanding rapidly. Microsoft alone increased its water consumption from 4,196,461 cubic meters in 2020 to 7,843,744 cubic meters in 2023. AI applications are also 100 to 1,000 times more computationally intensive than regular search functions, and as a result the electricity needs of data centers are overwhelming local power grids, and many tech giants are abandoning or delaying their plans to become carbon neutral. Google’s greenhouse gas emissions alone have increased at least 48% since 2019. And a recent analysis from The Guardian suggests the actual AI-related increase in resource use by big tech companies may be up to 662%, or 7.62 times, higher than they've officially reported.
Exploiting labor to create its datasets
Like so many other forms of "automation," generative AI technologies actually require loads of human labor to do things like tag millions of images to train computer vision for ImageNet and to filter the texts used to train LLMs to make them less racist, sexist, and homophobic. This work is deeply casualized, underpaid, and often psychologically harmful. It profits from and re-entrenches a stratified global labor market: many of the data workers used to maintain training sets are from the Global South, and one of the platforms used to buy their work is literally called the Mechanical Turk, owned by Amazon.
From an open letter written by content moderators and AI workers in Kenya to Biden: "US Big Tech companies are systemically abusing and exploiting African workers. In Kenya, these US companies are undermining the local labor laws, the country’s justice system and violating international labor standards. Our working conditions amount to modern day slavery."
Deskilling labor and demoralizing workers
The companies, hospitals, production studios, and academic institutions that have signed contracts with providers of proprietary AI have used those technologies to erode labor protections and worsen working conditions for their employees. Even when AI is not used directly to replace human workers, it is deployed as a tool for disciplining labor by deskilling the work humans perform: in other words, employers use AI tech to reduce the value of human labor (labor like grading student papers, providing customer service, consulting with patients, etc.) in order to enable the automation of previously skilled tasks. Deskilling makes it easier for companies and institutions to casualize and gigify what were previously more secure positions. It reduces pay and bargaining power for workers, forcing them into new gigs as adjuncts for its own technologies.
I can't say anything better than Tressie McMillan Cottom, so let me quote her recent piece at length: "A.I. may be a mid technology with limited use cases to justify its financial and environmental costs. But it is a stellar tool for demoralizing workers who can, in the blink of a digital eye, be categorized as waste. Whatever A.I. has the potential to become, in this political environment it is most powerful when it is aimed at demoralizing workers. This sort of mid tech would, in a perfect world, go the way of classroom TVs and MOOCs. It would find its niche, mildly reshape the way white-collar workers work and Americans would mostly forget about its promise to transform our lives. But we now live in a world where political might makes right. DOGE’s monthslong infomercial for A.I. reveals the difference that power can make to a mid technology. It does not have to be transformative to change how we live and work. In the wrong hands, mid tech is an antilabor hammer."
Enclosing knowledge production and destroying open access
OpenAI started as a non-profit, but it has now become one of the most aggressive for-profit companies in Silicon Valley. Alongside the new proprietary AIs developed by Google, Microsoft, Amazon, Meta, X, etc., OpenAI is extracting personal data and scraping copyrighted works to amass the data it needs to train their bots - even offering one-time payouts to authors to buy the rights to frack their work for AI grist - and then (or so they tell investors) they plan to sell the products back at a profit. As many critics have pointed out, proprietary AI thus works on a model of political economy similar to the 15th-19th-century capitalist project of enclosing what was formerly "the commons," or public land, to turn it into private property for the bourgeois class, who then owned the means of agricultural and industrial production. "Open"AI is built on and requires access to collective knowledge and public archives to run, but its promise to investors (the one they use to attract capital) is that it will enclose the profits generated from that knowledge for private gain.
AI companies hungry for good data to train their Large Language Models (LLMs) have also unleashed a new wave of bots that are stretching the digital infrastructure of open-access sites like Wikipedia, Project Gutenberg, and Internet Archive past capacity. As Eric Hellman writes in a recent blog post, these bots "use as many connections as you have room for. If you add capacity, they just ramp up their requests." In the process of scraping the intellectual commons, they're also trampling and trashing its benefits for truly public use.
Enriching tech oligarchs and fueling military imperialism
The names of many of the people and groups who get richer by generating speculative buzz for generative AI - Elon Musk, Mark Zuckerberg, Sam Altman, Larry Ellison - are familiar to the public because those people are currently using their wealth to purchase political influence and to win access to public resources. And it's looking increasingly likely that this political interference is motivated by the probability that the AI hype is a bubble - that the tech can never be made profitable or useful - and that tech oligarchs are hoping to keep it afloat as a speculation scheme through an infusion of public money - a.k.a. an AIG-style bailout.
In the meantime, these companies have found a growing interest from military buyers for their tech, as AI becomes a new front for "national security" imperialist growth wars. From an email written by Microsoft employee Ibtihal Aboussad, who interrupted Microsoft AI CEO Mustafa Suleyman at a live event to call him a war profiteer: "When I moved to AI Platform, I was excited to contribute to cutting-edge AI technology and its applications for the good of humanity: accessibility products, translation services, and tools to 'empower every human and organization to achieve more.' I was not informed that Microsoft would sell my work to the Israeli military and government, with the purpose of spying on and murdering journalists, doctors, aid workers, and entire civilian families. If I knew my work on transcription scenarios would help spy on and transcribe phone calls to better target Palestinians, I would not have joined this organization and contributed to genocide. I did not sign up to write code that violates human rights."
So there's a brief, non-exhaustive digest of some vectors for a critique of proprietary AI's role in the political economy. tl;dr: the first questions of material analysis are "who labors?" and "who profits/to whom does the value of that labor accrue?"
For further (and longer) reading, check out Justin Joque's Revolutionary Mathematics: Artificial Intelligence, Statistics and the Logic of Capitalism and Karen Hao's forthcoming Empire of AI.
25 notes
·
View notes
Note
My apologies for bringing back the AI discourse once more, lol, but while I understand and agree with a lot of the ideas you’ve brought up about AI generated art, I still can’t fully get behind it because, I dunno, isn’t it messed up to have taken someone’s labor to create the datasets capable of making AI art happen? If you don’t have the consent of the people whose content the AI is being trained on, isn’t that wrong too? As far as I know, a lot of these AI generators just scrape the Internet, even copyrighted material (lol) for datasets— that makes me really uncomfortable to know that my work is probably floating around some dataset somewhere, and while in the end it’s just a picture that someone generates for fun, the lack of consent to that is very discomforting. I don’t quite know what I’m asking here, I just feel like that has always been my biggest qualm with AI art. Is the solution just ethical AI generators with the consent of the people whose data is being used? Is that possible?
i guess i just fundamentally disagree with you on how ownership or art should work and how far it should be extended & think that following your stance to its natural conclusion would make the world a worse place. i think someone using art posted online in a collage is cool and good and that seems like much more straightforwardly 'using someone else's art without consented' than using that same piece of art as one datapoint among millions in a training set that teaches AI what things look like. & of course all the practical real methods of increasing that sort of post facto artistic control include strengthening IP laws and by that token strengthening large media corporations -- imagine a world where AI can only be trained on images you have the legal rights to, that would mean getty and disney could train away on their massive catalogues to the detriment of independent artists!
my one contention with AI dataset gathering is that private datasets are precipitating an arms race where platforms try to lay legal claim to their content so they can get a slice of the pie that is appropriating and privatising what should be publicly available.
149 notes
·
View notes
Text
A friendly wizard and style reference.
Midjourney has just released both the version 6 of its niji anime engine and the first version of its "style reference" tool.
Functionally this is a variation of the image prompting system (explained here), in which breaks a submitted image down into the 'token language' the AI uses internally and uses that as a supplement to a text prompt. "Style Reference" (or 'sref') lets you do this with up to three images, only with only the tokens associated with 'style' being drawn upon.
This is not to be confused with style transfer, a much older and very different AI art process.
But what is a style in this context? And how does it affect generation?
Prompt: a blue axolotl-anthro wizard in a red-and-yellow swirl-pattern robe, holding a sheleighleigh made of purple wood and a potion full of glowing green energy drink. A blue-and-green ladybug familiar stands near his feet, white background, fullbody image
Settings: --niji 6, --style raw --s 50 --seed 1762468963
Here, I've tested the same seed and prompt with a number of reference images.
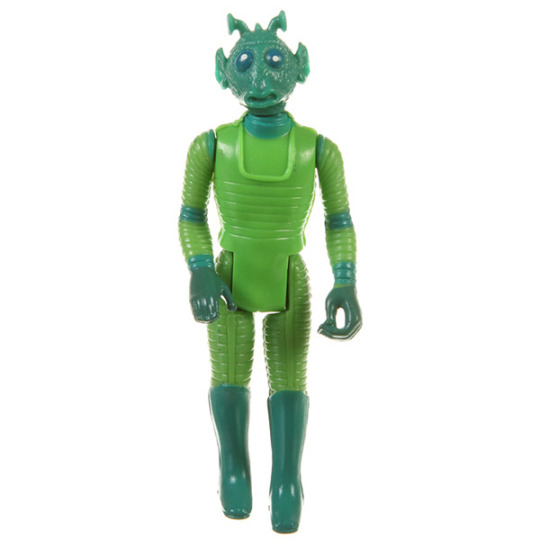

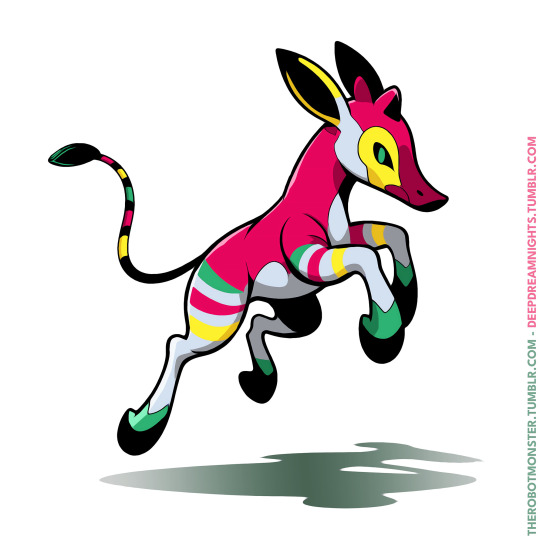
My semiorganized ramblings under the fold
The first thing I note is that style reference affects the gen so much that same-seed/different style ref comparisons are kind of pointless. Way too much of pose, composition and content changes for it to matter, so for future style ref tests, I'm probably going to drop the seeds.
The second thing I note is that there are certain limitations. You need to change up your prompt for things like photography, and the system interprets styles using its own criteria, not ours. If image prompting misinterprets something, so will style ref, but perhaps not in the same way.
This is notable for the one prompted with a scan from the Nuremberg Chronicle (first row). It recognizes that its a woodcut and emulates that general vibe nicely, but MJ is highly tuned for aesthetics, and emulating real world jank and clumsiness is a weak area. This is literally the first printed (european at least) book with illustrations. Every example thereafter is building on that skillset, so the dataset for woodcuts is going to be largely of a higher apparent quality.
In short, with Midjourney, additional prompt work is needed to replicate the look of early jank or intentionally 'ugly' art styles, and even as recent as v6 I've had no luck with things like midcentury Hanna-Barbereesque cheap TV animation styles or shitty 1990s CGI.
Style reference can help, I've gotten some pretty good cheap 80s-90s TV animation looking stuff from v6 niji and style ref in my early tests:
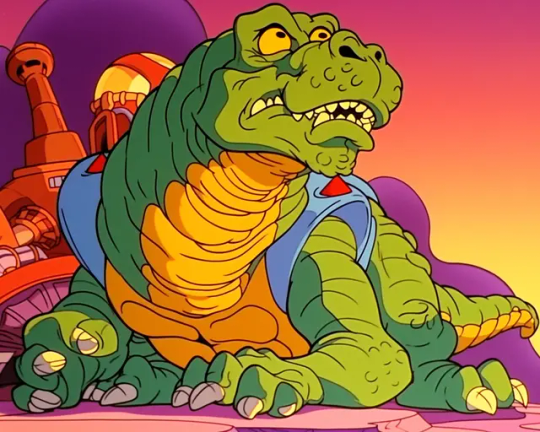
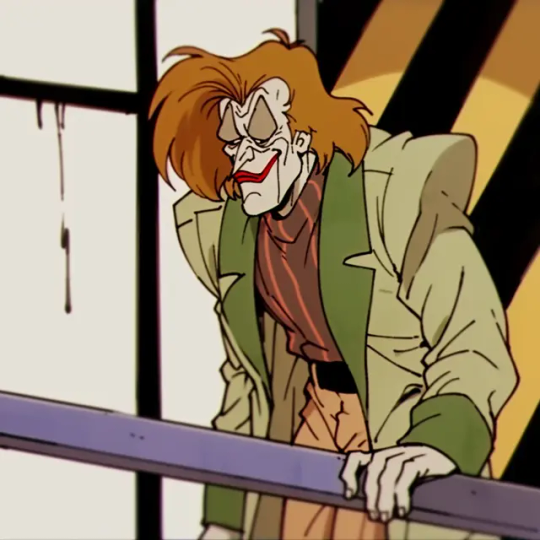
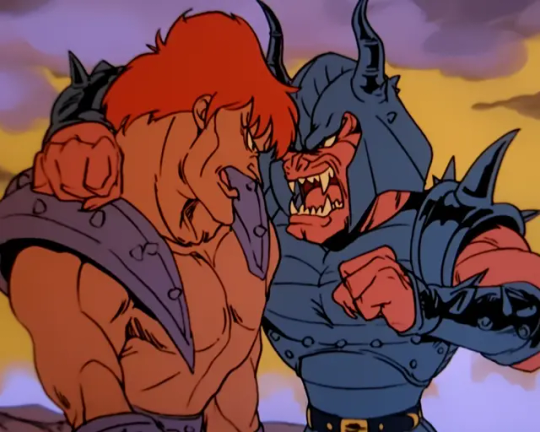
Color observations: Absent specific requests in the prompt, SREF will stick pretty close to the palette and lighting conditions of the referenced image. With such instructions, you get blending, so the one referencing the okapi fakemon (second row from bottom), for instance, has a lot of colors the reference image doesn't have, but they're in similar in vibrancy and saturation.
One limitation, however, is it doesn't apply to the aspects of the gen that come from any image prompts, so it will always blend the style of the style reference with the style aspects inherited from the image prompt, and that is very strong compared to the style ref.
Using the dog as the image prompt, and the TFTM reformatting as the style prompt, and the text prompt: "a cute older yorkie dog sitting on a bedspread", we get the image on the left. Dropping the image prompt weight to .25 gets us the center option, and removing the image prompt entirely produces the one on the right.
I expect this will be patched eventually, or general image prompting may fall out of favor compared to a combination of style ref and the upcoming character reference option, which will be the same thing, but will only reference the tokens associated with the character in the reference image. Depending on how that works that will have a lot of uses.
Stay tuned for more experiments. There's some good potential for freaky, unexplored aesthetics with combinations of multiple style refs and text prompts.
#ai artwork#style reference#midjourney v6#nijijourney v6#generative art#axolotol#wizard#prompt testing#ai experiments
55 notes
·
View notes
Text
Was watching a video yesterday when an argument was made for ai art that rubbed me the wrong way but didn't know how to express my thoughts at the time. (I'm an art student, not an expert. Would love to discuss.)
It was a pro-ai argument for art that discussed how throughout history when new developments in art were made, it was discredited every time by people who didn't see it as "real art" (which for me brought to mind examples of the photograph, conceptual art, the shift from hand-drawn to digitized animation, etc). By being anti-ai, the argument was that people are discrediting a new technology completely that could bring about great change and completely shift the artistic landscape in the future.
Additionally, there was a mention of data usage and how they very much wanted their data used to contribute to the datasets for ai development, and had opted in, specifically mentioning that everyone should get a choice in whether or not their data should be used, which I very much agree with. This part of the argument I'm not concerned with, I think informed and consensual data contribution to companies is so important and if you want to give away your data for that cause that's just fine (... i think you should be PAID with MONEY for that data usage, but that's a whole other thing). It has to be consented to, and if people opt out, you can't use their data. Seemingly easy.
It's the part about historical movements that got to me, lumping together artistic / technological developments as positive jumps forward with a certain group of detractors who are on the "wrong side of history". I think examining what is actually at play now and what makes this situation different from previous examples is what people are so concerned about.
My issues with ai that i can think of off the top of my head:
steals from millions of people without their consent
heavily monetized and intertwined in corporate profit
destabilizes entire industries, creative unions, countless jobs
aids in misinformation and constant plagiarism
disrupts the education of students globally and the jobs of teachers
massive environmental impact and energy requirements to keep the development going
No artistic movement I can think of has had such dire consequences. I don't just hate ai as an art medium because it makes shitty garbage, because it's eliminating jobs I once thought I could have, even because it's stealing from me, actively, right now. I am overwhelmed by the amount terrible results that have emerged from the development of ai, and no matter how interesting art eventually becomes with the aid of that technology, I will never forget what that technology is taking from people across the world. Until those problems are fixed (some of which NEVER will be because exploitations is so engrained in the way ai functions), I can never call myself someone who is pro-ai.
I think of the development of photography, specifically the daguerreotype (a technology that was stolen by Dagguerre from the original inventor Niepce) which came about and changed the landscape of images. Painters who once did the job a photographer could do in a fraction of the time lost jobs, and there was a struggle to determine the legitimacy of photography as an art and a science.
I think of the growth of conceptual art, working beyond the image to create experiences, to use one's body as the canvas, to experiment with performance, perception, endurance. People criticized these movements as non-art movements, but they endure today as really important concepts in the art world.
Even the more recent shift in the 80s-90s, the shift from hand-drawn animation to digital animation. People did lose jobs, industries did shift and that form of animation became much harder as digital animation became the cheaper, quicker alternative which was preferred by companies funding projects.
These cases have never had such vast impacts as we're facing now with the growth of ai, and even though there is work being created today including the use of certain ai algorithms that I do enjoy and find really interesting (ex. Hito Steyerl, Rachel Maclean, Heather Dewey-Hagborg), this doesn't erase the constant advertisement I receive about shitty ai programs, search engines overrun by ai images, the PEERS I have around me who go to university today and write assignments using ai, and the massive water usage of tech centers dedicated to house ai systems.
This topic is exhaustive and I've only barely gotten my thoughts out on this, may or may not add onto this later. The industry is constantly changing and I know things have to get better, but the rock bottom feeling I'm experiencing when I face the subject is sooo strong. Going back to drawing comics now.
9 notes
·
View notes
Text
assuaging my anxieties about machine learning over the last week, I learn that despite there being about ten years of doom-saying about the full automation of radiomics, there's actually a shortage of radiologists now (and, also, the machine learning algorithms that are supposed to be able to detect cancers better than human doctors are very often giving overconfident predictions). truck driving was supposed to be completely automated by now, but my grampa is still truckin' and will probably get to retire as a trucker. companies like GM are now throwing decreasing amounts of money at autonomous vehicle research after throwing billions at cars that can just barely ferry people around san francisco (and sometimes still fails), the most mapped and trained upon set of roads in the world. (imagine the cost to train these things for a city with dilapidated infrastructure, where the lines in the road have faded away, like, say, Shreveport, LA).
we now have transformer-based models that are able to provide contextually relevant responses, but the responses are often wrong, and often in subtle ways that require expertise to needle out. the possibility of giving a wrong response is always there - it's a stochastic next-word prediction algorithm based on statistical inferences gleaned from the training data, with no innate understanding of the symbols its producing. image generators are questionably legal (at least the way they were trained and how that effects the output of essentially copyrighted material). graphic designers, rather than being replaced by them, are already using them as a tool, and I've already seen local designers do this (which I find cheap and ugly - one taco place hired a local designer to make a graphic for them - the tacos looked like taco bell's, not the actual restaurant's, and you could see artefacts from the generation process everywhere). for the most part, what they produce is visually ugly and requires extensive touchups - if the model even gives you an output you can edit. the role of the designer as designer is still there - they are still the arbiter of good taste, and the value of a graphic designer is still based on whether or not they have a well developed aesthetic taste themself.
for the most part, everything is in tech demo phase, and this is after getting trained on nearly the sum total of available human produced data, which is already a problem for generalized performance. while a lot of these systems perform well on older, flawed, benchmarks, newer benchmarks show that these systems (including GPT-4 with plugins) consistently fail to compete with humans equipped with everyday knowledge.
there is also a huge problem with the benchmarks typically used to measure progress in machine learning that impact their real world use (and tell us we should probably be more cautious because the human use of these tools is bound to be reckless given the hype they've received). back to radiomics, some machine learning models barely generalize at all, and only perform slightly better than chance at identifying pneumonia in pediatric cases when it's exposed to external datasets (external to the hospital where the data it was trained on came from). other issues, like data leakage, make popular benchmarks often an overoptimistic measure of success.
very few researchers in machine learning are recognizing these limits. that probably has to do with the academic and commercial incentives towards publishing overconfident results. many papers are not even in principle reproducible, because the code, training data, etc., is simply not provided. "publish or perish", the bias journals have towards positive results, and the desire of tech companies to get continued funding while "AI" is the hot buzzword, all combined this year for the perfect storm of techno-hype.
which is not to say that machine learning is useless. their use as glorified statistical methods has been a boon for scientists, when those scientists understand what's going on under the hood. in a medical context, tempered use of machine learning has definitely saved lives already. some programmers swear that copilot has made them marginally more productive, by autocompleting sometimes tedious boilerplate code (although, hey, we've had code generators doing this for several decades). it's probably marginally faster to ask a service "how do I reverse a string" than to look through the docs (although, if you had read the docs to begin with would you even need to take the risk of the service getting it wrong?) people have a lot of fun with the image generators, because one-off memes don't require high quality aesthetics to get a chuckle before the user scrolls away (only psychopaths like me look at these images for artefacts). doctors will continue to use statistical tools in the wider machine learning tool set to augment their provision of care, if these were designed and implemented carefully, with a mind to their limitations.
anyway, i hope posting this will assuage my anxieties for another quarter at least.
35 notes
·
View notes
Text
youtube
Okay, I'm a data nerd. But this is so interesting?!
She starts out by looking at whether YouTube intense promotion of short-form content is harming long-form content, and ends up looking at how AI models amplify cultural biases.
In one of her examples, Amazon had to stop using AI recruitment software because it was filtering out women. They had to tell it to stop removing resumes with the word "women's" in them.
But even after they did that, the software was filtering out tons of women by doing things like selecting for more "aggressive" language like "executed on" instead of, idk, "helped."
Which is exactly how humans act. In my experience, when people are trying to unlearn bias, the first thing they do is go, "okay, so when I see that someone was president of the women's hockey team or something, I tend to dismiss them, but they could be good! I should try to look at them, instead of immediately dismissing candidates who are women! That makes sense, I can do that!"
And then they don't realize that they can also look at identical resumes, one with a "man's" name and one with a "woman's" name, and come away being more impressed by the "man's" resume.
So then they start having HR remove the names from all resumes. But they don't extrapolate from all this and think about whether the interviewer might also be biased. They don't think about how many different ways you can describe the same exact tasks at the same exact job, and how some of them sound way better.
They don't think about how, the more marginalized someone is, the less access they have to information about what language to use. And the more likely they are to have been "trained," by the way people treat them, to minimize their own skills and achievements.
They don't think about why certain words sound polished to them, and whether that's actually reflecting how good the person using that language will be at their job.
In this How To Cook That video, she talks about the fact that they're training that type of software on, say, ten years of hiring data, and that inherently means it's going to learn the biases reflected in that data... and that what AI models do is EXAGGERATE what they've learned.
Her example is that if you do a Google image search for "doctor," 90% of them will be men, even though in real life only 63% are men.
This is all fascinating to me because this is why representation matters. This is such an extreme, obvious example of why representation matters. OUR brains look at everything around us and learn who the world says is good at what.
We learn what a construction worker looks like, what a general practitioner doctor looks like, what a pediatrician looks like, what a teacher looks like.
We look at the people in our lives and in the media we consume and the ambient media we live through. And we learn what people who matter in our particular society look like.
We learn what a believable, trustworthy person looks like. The kind of person who can be the faux-generic-human talking to you about or illustrating a product.
Unless we also actively learn that other kinds of people matter equally, are equally trustworthy and believable... we don't.
And that affects EVERYTHING.
Also, this seems very easy to undo -- for AI, at least.
Like, instead of giving it a dataset of all the pictures of doctors humans have put out there, they could find people who actively prefer diverse, interesting groups of examples. And give the dataset to a bunch of them, first, to produce something for AI to learn from.
Harvard has a whole slew of really good tests for bias, although I would love to see more. (Note: they say things like "gay - straight, " but it's not testing how you feel about straight people. It's testing whether you have negative associations about gay people, and it uses straight people as a kind of baseline.)
There must be a way for image-recognition AI software to take these tests and reveal how biased a given model is, so it can be tweaked.
A lot of people would probably object that you're biasing the model intentionally if you do that. But we know the models are biased. We know we all have cultural biases. (I mean. Most people know that, I think.)
Anyway, this is already known in the field. There are plenty of studies about the biases in different AI programs, and the biases humans have.
That means we're already choosing to bias models intentionally. Both by knowingly giving them our biases, and by knowing they'll make our biases even bigger. And we already know this has a negative impact on people's lives.
12 notes
·
View notes
Text
The Future of AI: What’s Next in Machine Learning and Deep Learning?
Artificial Intelligence (AI) has rapidly evolved over the past decade, transforming industries and redefining the way businesses operate. With machine learning and deep learning at the core of AI advancements, the future holds groundbreaking innovations that will further revolutionize technology. As machine learning and deep learning continue to advance, they will unlock new opportunities across various industries, from healthcare and finance to cybersecurity and automation. In this blog, we explore the upcoming trends and what lies ahead in the world of machine learning and deep learning.
1. Advancements in Explainable AI (XAI)
As AI models become more complex, understanding their decision-making process remains a challenge. Explainable AI (XAI) aims to make machine learning and deep learning models more transparent and interpretable. Businesses and regulators are pushing for AI systems that provide clear justifications for their outputs, ensuring ethical AI adoption across industries. The growing demand for fairness and accountability in AI-driven decisions is accelerating research into interpretable AI, helping users trust and effectively utilize AI-powered tools.
2. AI-Powered Automation in IT and Business Processes
AI-driven automation is set to revolutionize business operations by minimizing human intervention. Machine learning and deep learning algorithms can predict and automate tasks in various sectors, from IT infrastructure management to customer service and finance. This shift will increase efficiency, reduce costs, and improve decision-making. Businesses that adopt AI-powered automation will gain a competitive advantage by streamlining workflows and enhancing productivity through machine learning and deep learning capabilities.
3. Neural Network Enhancements and Next-Gen Deep Learning Models
Deep learning models are becoming more sophisticated, with innovations like transformer models (e.g., GPT-4, BERT) pushing the boundaries of natural language processing (NLP). The next wave of machine learning and deep learning will focus on improving efficiency, reducing computation costs, and enhancing real-time AI applications. Advancements in neural networks will also lead to better image and speech recognition systems, making AI more accessible and functional in everyday life.
4. AI in Edge Computing for Faster and Smarter Processing
With the rise of IoT and real-time processing needs, AI is shifting toward edge computing. This allows machine learning and deep learning models to process data locally, reducing latency and dependency on cloud services. Industries like healthcare, autonomous vehicles, and smart cities will greatly benefit from edge AI integration. The fusion of edge computing with machine learning and deep learning will enable faster decision-making and improved efficiency in critical applications like medical diagnostics and predictive maintenance.
5. Ethical AI and Bias Mitigation
AI systems are prone to biases due to data limitations and model training inefficiencies. The future of machine learning and deep learning will prioritize ethical AI frameworks to mitigate bias and ensure fairness. Companies and researchers are working towards AI models that are more inclusive and free from discriminatory outputs. Ethical AI development will involve strategies like diverse dataset curation, bias auditing, and transparent AI decision-making processes to build trust in AI-powered systems.
6. Quantum AI: The Next Frontier
Quantum computing is set to revolutionize AI by enabling faster and more powerful computations. Quantum AI will significantly accelerate machine learning and deep learning processes, optimizing complex problem-solving and large-scale simulations beyond the capabilities of classical computing. As quantum AI continues to evolve, it will open new doors for solving problems that were previously considered unsolvable due to computational constraints.
7. AI-Generated Content and Creative Applications
From AI-generated art and music to automated content creation, AI is making strides in the creative industry. Generative AI models like DALL-E and ChatGPT are paving the way for more sophisticated and human-like AI creativity. The future of machine learning and deep learning will push the boundaries of AI-driven content creation, enabling businesses to leverage AI for personalized marketing, video editing, and even storytelling.
8. AI in Cybersecurity: Real-Time Threat Detection
As cyber threats evolve, AI-powered cybersecurity solutions are becoming essential. Machine learning and deep learning models can analyze and predict security vulnerabilities, detecting threats in real time. The future of AI in cybersecurity lies in its ability to autonomously defend against sophisticated cyberattacks. AI-powered security systems will continuously learn from emerging threats, adapting and strengthening defense mechanisms to ensure data privacy and protection.
9. The Role of AI in Personalized Healthcare
One of the most impactful applications of machine learning and deep learning is in healthcare. AI-driven diagnostics, predictive analytics, and drug discovery are transforming patient care. AI models can analyze medical images, detect anomalies, and provide early disease detection, improving treatment outcomes. The integration of machine learning and deep learning in healthcare will enable personalized treatment plans and faster drug development, ultimately saving lives.
10. AI and the Future of Autonomous Systems
From self-driving cars to intelligent robotics, machine learning and deep learning are at the forefront of autonomous technology. The evolution of AI-powered autonomous systems will improve safety, efficiency, and decision-making capabilities. As AI continues to advance, we can expect self-learning robots, smarter logistics systems, and fully automated industrial processes that enhance productivity across various domains.
Conclusion
The future of AI, machine learning and deep learning is brimming with possibilities. From enhancing automation to enabling ethical and explainable AI, the next phase of AI development will drive unprecedented innovation. Businesses and tech leaders must stay ahead of these trends to leverage AI's full potential. With continued advancements in machine learning and deep learning, AI will become more intelligent, efficient, and accessible, shaping the digital world like never before.
Are you ready for the AI-driven future? Stay updated with the latest AI trends and explore how these advancements can shape your business!
#artificial intelligence#machine learning#techinnovation#tech#technology#web developers#ai#web#deep learning#Information and technology#IT#ai future
2 notes
·
View notes
Text
In late July, OpenAI began rolling out an eerily humanlike voice interface for ChatGPT. In a safety analysis released today, the company acknowledges that this anthropomorphic voice may lure some users into becoming emotionally attached to their chatbot.
The warnings are included in a “system card” for GPT-4o, a technical document that lays out what the company believes are the risks associated with the model, plus details surrounding safety testing and the mitigation efforts the company’s taking to reduce potential risk.
OpenAI has faced scrutiny in recent months after a number of employees working on AI’s long-term risks quit the company. Some subsequently accused OpenAI of taking unnecessary chances and muzzling dissenters in its race to commercialize AI. Revealing more details of OpenAI’s safety regime may help mitigate the criticism and reassure the public that the company takes the issue seriously.
The risks explored in the new system card are wide-ranging, and include the potential for GPT-4o to amplify societal biases, spread disinformation, and aid in the development of chemical or biological weapons. It also discloses details of testing designed to ensure that AI models won’t try to break free of their controls, deceive people, or scheme catastrophic plans.
Some outside experts commend OpenAI for its transparency but say it could go further.
Lucie-Aimée Kaffee, an applied policy researcher at Hugging Face, a company that hosts AI tools, notes that OpenAI's system card for GPT-4o does not include extensive details on the model’s training data or who owns that data. "The question of consent in creating such a large dataset spanning multiple modalities, including text, image, and speech, needs to be addressed," Kaffee says.
Others note that risks could change as tools are used in the wild. “Their internal review should only be the first piece of ensuring AI safety,” says Neil Thompson, a professor at MIT who studies AI risk assessments. “Many risks only manifest when AI is used in the real world. It is important that these other risks are cataloged and evaluated as new models emerge.”
The new system card highlights how rapidly AI risks are evolving with the development of powerful new features such as OpenAI’s voice interface. In May, when the company unveiled its voice mode, which can respond swiftly and handle interruptions in a natural back and forth, many users noticed it appeared overly flirtatious in demos. The company later faced criticism from the actress Scarlett Johansson, who accused it of copying her style of speech.
A section of the system card titled “Anthropomorphization and Emotional Reliance” explores problems that arise when users perceive AI in human terms, something apparently exacerbated by the humanlike voice mode. During the red teaming, or stress testing, of GPT-4o, for instance, OpenAI researchers noticed instances of speech from users that conveyed a sense of emotional connection with the model. For example, people used language such as “This is our last day together.”
Anthropomorphism might cause users to place more trust in the output of a model when it “hallucinates” incorrect information, OpenAI says. Over time, it might even affect users’ relationships with other people. “Users might form social relationships with the AI, reducing their need for human interaction—potentially benefiting lonely individuals but possibly affecting healthy relationships,” the document says.
Joaquin Quiñonero Candela, head of preparedness at OpenAI, says that voice mode could evolve into a uniquely powerful interface. He also notes that the kind of emotional effects seen with GPT-4o can be positive—say, by helping those who are lonely or who need to practice social interactions. He adds that the company will study anthropomorphism and the emotional connections closely, including by monitoring how beta testers interact with ChatGPT. “We don’t have results to share at the moment, but it’s on our list of concerns,” he says.
Other problems arising from voice mode include potential new ways of “jailbreaking” OpenAI’s model—by inputting audio that causes the model to break loose of its restrictions, for instance. The jailbroken voice mode could be coaxed into impersonating a particular person or attempting to read a users’ emotions. The voice mode can also malfunction in response to random noise, OpenAI found, and in one instance, testers noticed it adopting a voice similar to that of the user. OpenAI also says it is studying whether the voice interface might be more effective at persuading people to adopt a particular viewpoint.
OpenAI is not alone in recognizing the risk of AI assistants mimicking human interaction. In April, Google DeepMind released a lengthy paper discussing the potential ethical challenges raised by more capable AI assistants. Iason Gabriel, a staff research scientist at the company and a coauthor of the paper, tells WIRED that chatbots’ ability to use language “creates this impression of genuine intimacy,” adding that he himself had found an experimental voice interface for Google DeepMind’s AI to be especially sticky. “There are all these questions about emotional entanglement,” Gabriel says.
Such emotional ties may be more common than many realize. Some users of chatbots like Character AI and Replika report antisocial tensions resulting from their chat habits. A recent TikTok with almost a million views shows one user apparently so addicted to Character AI that they use the app while watching a movie in a theater. Some commenters mentioned that they would have to be alone to use the chatbot because of the intimacy of their interactions. “I’ll never be on [Character AI] unless I’m in my room,” wrote one.
6 notes
·
View notes
Text
Generating Chest X-Rays with AI: Insights from Christian Bluethgen | Episode 24 - Bytes of Innovation
Join Christian Bluethgen in this 32-minute webinar as he delves into RoentGen, an AI model synthesizing chest X-ray images from textual descriptions. Learn about its potential in medical imaging, benefits for rare disease data, and considerations regarding model limitations and ethical concerns.
#AI in Radiology#Real World Data#Real World Evidence#Real World Imaging Datasets#Medical Imaging Datasets#RWiD#AI in Healthcare
0 notes
Text
What is Artificial Intelligence?? A Beginner's Guide to Understand Artificial Intelligence
1) What is Artificial Intelligence (AI)??
Artificial Intelligence (AI) is a set of technologies that enables computer to perform tasks normally performed by humans. This includes the ability to learn (machine learning) reasoning, decision making and even natural language processing from virtual assistants like Siri and Alexa to prediction algorithms on Netflix and Google Maps.
The foundation of the AI lies in its ability to simulate cognitive tasks. Unlike traditional programming where machines follow clear instructions, AI systems use vast algorithms and datasets to recognize patterns, identify trends and automatically improve over time.
2) Many Artificial Intelligence (AI) faces
Artificial Intelligence (AI) isn't one thing but it is a term that combines many different technologies together. Understanding its ramifications can help you understand its versatility:
Machine Learning (ML): At its core, AI focuses on enabling ML machines to learn from data and make improvements without explicit programming. Applications range from spam detection to personalized shopping recommendations.
Computer Vision: This field enables machines to interpret and analyze image data from facial recognition to medical image diagnosis. Computer Vision is revolutionizing many industries.
Robotics: By combining AI with Engineering Robotics focuses on creating intelligent machines that can perform tasks automatically or with minimal human intervention.
Creative AI: Tools like ChatGPT and DALL-E fail into this category. Create human like text or images and opens the door to creative and innovative possibilities.
3) Why is AI so popular now??
The Artificial Intelligence (AI) explosion may be due to a confluence of technological advances:
Big Data: The digital age creates unprecedented amounts of data. Artificial Intelligence (AI) leverages data and uses it to gain insights and improve decision making.
Improved Algorithms: Innovations in algorithms make Artificial Intelligence (AI) models more efficient and accurate.
Computing Power: The rise of cloud computing and GPUs has provided the necessary infrastructure for processing complex AI models.
Access: The proliferation of publicly available datasets (eg: ImageNet, Common Crawl) has provided the basis for training complex AI Systems. Various Industries also collect a huge amount of proprietary data. This makes it possible to deploy domain specific AI applications.
4) Interesting Topics about Artificial Intelligence (AI)
Real World applications of AI shows that AI is revolutionizing industries such as Healthcare (primary diagnosis and personalized machine), finance (fraud detection and robo advisors), education (adaptive learning platforms) and entertainment (adaptive platforms) how??
The role of AI in "Creativity Explore" on how AI tools like DALL-E and ChatGPT are helping artists, writers and designers create incredible work. Debate whether AI can truly be creative or just enhance human creativity.
AI ethics and Bias are an important part of AI decision making, it is important to address issues such as bias, transparency and accountability. Search deeper into the importance of ethical AI and its impact on society.
AI in everyday life about how little known AI is affecting daily life, from increasing energy efficiency in your smart home to reading the forecast on your smartphone.
The future of AI anticipate upcoming advance services like Quantum AI and their potential to solve humanity's biggest challenges like climate change and pandemics.
5) Conclusion
Artificial Intelligence (AI) isn't just a technological milestone but it is a paradigm shift that continues to redefine our future. As you explore the vast world of AI, think outside the box to find nuances, applications and challenges with well researched and engaging content
Whether unpacking how AI works or discussing its transformative potential, this blog can serve as a beacon for those eager to understand this underground branch.
"As we stand on the brink of an AI-powered future, the real question isn't what AI can do for us, but what we dare to imagine next"
"Get Latest News on www.bloggergaurang.com along with Breaking News and Top Headlines from all around the World !!"
2 notes
·
View notes
Text
The Role of Machine Learning Engineer: Combining Technology and Artificial Intelligence
Artificial intelligence has transformed our daily lives in a greater way than we can’t imagine over the past year, Impacting how we work, communicate, and solve problems. Today, Artificial intelligence furiously drives the world in all sectors from daily life to the healthcare industry. In this blog we will learn how machine learning engineer build systems that learn from data and get better over time, playing a huge part in the development of artificial intelligence (AI). Artificial intelligence is an important field, making it more innovative in every industry. In the blog, we will look career in Machine learning in the field of engineering.
What is Machine Learning Engineering?
Machine Learning engineer is a specialist who designs and builds AI models to make complex challenges easy. The role in this field merges data science and software engineering making both fields important in this field. The main role of a Machine learning engineer is to build and design software that can automate AI models. The demand for this field has grown in recent years. As Artificial intelligence is a driving force in our daily needs, it become important to run the AI in a clear and automated way.
A machine learning engineer creates systems that help computers to learn and make decisions, similar to human tasks like recognizing voices, identifying images, or predicting results. Not similar to regular programming, which follows strict rules, machine learning focuses on teaching computers to find patterns in data and improve their predictions over time.
Responsibility of a Machine Learning Engineer:
Collecting and Preparing Data
Machine learning needs a lot of data to work well. These engineers spend a lot of time finding and organizing data. That means looking for useful data sources and fixing any missing information. Good data preparation is essential because it sets the foundation for building successful models.
Building and Training Models
The main task of Machine learning engineer is creating models that learn from data. Using tools like TensorFlow, PyTorch, and many more, they build proper algorithms for specific tasks. Training a model is challenging and requires careful adjustments and monitoring to ensure it’s accurate and useful.
Checking Model Performance
When a model is trained, then it is important to check how well it works. Machine learning engineers use scores like accuracy to see model performance. They usually test the model with separate data to see how it performs in real-world situations and make improvements as needed.
Arranging and Maintaining the Model
After testing, ML engineers put the model into action so it can work with real-time data. They monitor the model to make sure it stays accurate over time, as data can change and affect results. Regular updates help keep the model effective.
Working with Other Teams
ML engineers often work closely with data scientists, software engineers, and experts in the field. This teamwork ensures that the machine learning solution fits the business goals and integrates smoothly with other systems.
Important skill that should have to become Machine Learning Engineer:
Programming Languages
Python and R are popular options in machine learning, also other languages like Java or C++ can also help, especially for projects needing high performance.
Data Handling and Processing
Working with large datasets is necessary in Machine Learning. ML engineers should know how to use SQL and other database tools and be skilled in preparing and cleaning data before using it in models.
Machine Learning Structure
ML engineers need to know structure like TensorFlow, Keras, PyTorch, and sci-kit-learn. Each of these tools has unique strengths for building and training models, so choosing the right one depends on the project.
Mathematics and Statistics
A strong background in math, including calculus, linear algebra, probability, and statistics, helps ML engineers understand how algorithms work and make accurate predictions.
Why to become a Machine Learning engineer?
A career as a machine learning engineer is both challenging and creative, allowing you to work with the latest technology. This field is always changing, with new tools and ideas coming up every year. If you like to enjoy solving complex problems and want to make a real impact, ML engineering offers an exciting path.
Conclusion
Machine learning engineer plays an important role in AI and data science, turning data into useful insights and creating systems that learn on their own. This career is great for people who love technology, enjoy learning, and want to make a difference in their lives. With many opportunities and uses, Artificial intelligence is a growing field that promises exciting innovations that will shape our future. Artificial Intelligence is changing the world and we should also keep updated our knowledge in this field, Read AI related latest blogs here.
2 notes
·
View notes
Text
Mastering Neural Networks: A Deep Dive into Combining Technologies
How Can Two Trained Neural Networks Be Combined?
Introduction
In the ever-evolving world of artificial intelligence (AI), neural networks have emerged as a cornerstone technology, driving advancements across various fields. But have you ever wondered how combining two trained neural networks can enhance their performance and capabilities? Let’s dive deep into the fascinating world of neural networks and explore how combining them can open new horizons in AI.
Basics of Neural Networks
What is a Neural Network?
Neural networks, inspired by the human brain, consist of interconnected nodes or "neurons" that work together to process and analyze data. These networks can identify patterns, recognize images, understand speech, and even generate human-like text. Think of them as a complex web of connections where each neuron contributes to the overall decision-making process.
How Neural Networks Work
Neural networks function by receiving inputs, processing them through hidden layers, and producing outputs. They learn from data by adjusting the weights of connections between neurons, thus improving their ability to predict or classify new data. Imagine a neural network as a black box that continuously refines its understanding based on the information it processes.
Types of Neural Networks
From simple feedforward networks to complex convolutional and recurrent networks, neural networks come in various forms, each designed for specific tasks. Feedforward networks are great for straightforward tasks, while convolutional neural networks (CNNs) excel in image recognition, and recurrent neural networks (RNNs) are ideal for sequential data like text or speech.
Why Combine Neural Networks?
Advantages of Combining Neural Networks
Combining neural networks can significantly enhance their performance, accuracy, and generalization capabilities. By leveraging the strengths of different networks, we can create a more robust and versatile model. Think of it as assembling a team where each member brings unique skills to tackle complex problems.
Applications in Real-World Scenarios
In real-world applications, combining neural networks can lead to breakthroughs in fields like healthcare, finance, and autonomous systems. For example, in medical diagnostics, combining networks can improve the accuracy of disease detection, while in finance, it can enhance the prediction of stock market trends.
Methods of Combining Neural Networks
Ensemble Learning
Ensemble learning involves training multiple neural networks and combining their predictions to improve accuracy. This approach reduces the risk of overfitting and enhances the model's generalization capabilities.
Bagging
Bagging, or Bootstrap Aggregating, trains multiple versions of a model on different subsets of the data and combines their predictions. This method is simple yet effective in reducing variance and improving model stability.
Boosting
Boosting focuses on training sequential models, where each model attempts to correct the errors of its predecessor. This iterative process leads to a powerful combined model that performs well even on difficult tasks.
Stacking
Stacking involves training multiple models and using a "meta-learner" to combine their outputs. This technique leverages the strengths of different models, resulting in superior overall performance.
Transfer Learning
Transfer learning is a method where a pre-trained neural network is fine-tuned on a new task. This approach is particularly useful when data is scarce, allowing us to leverage the knowledge acquired from previous tasks.
Concept of Transfer Learning
In transfer learning, a model trained on a large dataset is adapted to a smaller, related task. For instance, a model trained on millions of images can be fine-tuned to recognize specific objects in a new dataset.
How to Implement Transfer Learning
To implement transfer learning, we start with a pretrained model, freeze some layers to retain their knowledge, and fine-tune the remaining layers on the new task. This method saves time and computational resources while achieving impressive results.
Advantages of Transfer Learning
Transfer learning enables quicker training times and improved performance, especially when dealing with limited data. It’s like standing on the shoulders of giants, leveraging the vast knowledge accumulated from previous tasks.
Neural Network Fusion
Neural network fusion involves merging multiple networks into a single, unified model. This method combines the strengths of different architectures to create a more powerful and versatile network.
Definition of Neural Network Fusion
Neural network fusion integrates different networks at various stages, such as combining their outputs or merging their internal layers. This approach can enhance the model's ability to handle diverse tasks and data types.
Types of Neural Network Fusion
There are several types of neural network fusion, including early fusion, where networks are combined at the input level, and late fusion, where their outputs are merged. Each type has its own advantages depending on the task at hand.
Implementing Fusion Techniques
To implement neural network fusion, we can combine the outputs of different networks using techniques like averaging, weighted voting, or more sophisticated methods like learning a fusion model. The choice of technique depends on the specific requirements of the task.
Cascade Network
Cascade networks involve feeding the output of one neural network as input to another. This approach creates a layered structure where each network focuses on different aspects of the task.
What is a Cascade Network?
A cascade network is a hierarchical structure where multiple networks are connected in series. Each network refines the outputs of the previous one, leading to progressively better performance.
Advantages and Applications of Cascade Networks
Cascade networks are particularly useful in complex tasks where different stages of processing are required. For example, in image processing, a cascade network can progressively enhance image quality, leading to more accurate recognition.
Practical Examples
Image Recognition
In image recognition, combining CNNs with ensemble methods can improve accuracy and robustness. For instance, a network trained on general image data can be combined with a network fine-tuned for specific object recognition, leading to superior performance.
Natural Language Processing
In natural language processing (NLP), combining RNNs with transfer learning can enhance the understanding of text. A pre-trained language model can be fine-tuned for specific tasks like sentiment analysis or text generation, resulting in more accurate and nuanced outputs.
Predictive Analytics
In predictive analytics, combining different types of networks can improve the accuracy of predictions. For example, a network trained on historical data can be combined with a network that analyzes real-time data, leading to more accurate forecasts.
Challenges and Solutions
Technical Challenges
Combining neural networks can be technically challenging, requiring careful tuning and integration. Ensuring compatibility between different networks and avoiding overfitting are critical considerations.
Data Challenges
Data-related challenges include ensuring the availability of diverse and high-quality data for training. Managing data complexity and avoiding biases are essential for achieving accurate and reliable results.
Possible Solutions
To overcome these challenges, it’s crucial to adopt a systematic approach to model integration, including careful preprocessing of data and rigorous validation of models. Utilizing advanced tools and frameworks can also facilitate the process.
Tools and Frameworks
Popular Tools for Combining Neural Networks
Tools like TensorFlow, PyTorch, and Keras provide extensive support for combining neural networks. These platforms offer a wide range of functionalities and ease of use, making them ideal for both beginners and experts.
Frameworks to Use
Frameworks like Scikit-learn, Apache MXNet, and Microsoft Cognitive Toolkit offer specialized support for ensemble learning, transfer learning, and neural network fusion. These frameworks provide robust tools for developing and deploying combined neural network models.
Future of Combining Neural Networks
Emerging Trends
Emerging trends in combining neural networks include the use of advanced ensemble techniques, the integration of neural networks with other AI models, and the development of more sophisticated fusion methods.
Potential Developments
Future developments may include the creation of more powerful and efficient neural network architectures, enhanced transfer learning techniques, and the integration of neural networks with other technologies like quantum computing.
Case Studies
Successful Examples in Industry
In healthcare, combining neural networks has led to significant improvements in disease diagnosis and treatment recommendations. For example, combining CNNs with RNNs has enhanced the accuracy of medical image analysis and patient monitoring.
Lessons Learned from Case Studies
Key lessons from successful case studies include the importance of data quality, the need for careful model tuning, and the benefits of leveraging diverse neural network architectures to address complex problems.
Online Course
I have came across over many online courses. But finally found something very great platform to save your time and money.
1.Prag Robotics_ TBridge
2.Coursera
Best Practices
Strategies for Effective Combination
Effective strategies for combining neural networks include using ensemble methods to enhance performance, leveraging transfer learning to save time and resources, and adopting a systematic approach to model integration.
Avoiding Common Pitfalls
Common pitfalls to avoid include overfitting, ignoring data quality, and underestimating the complexity of model integration. By being aware of these challenges, we can develop more robust and effective combined neural network models.
Conclusion
Combining two trained neural networks can significantly enhance their capabilities, leading to more accurate and versatile AI models. Whether through ensemble learning, transfer learning, or neural network fusion, the potential benefits are immense. By adopting the right strategies and tools, we can unlock new possibilities in AI and drive advancements across various fields.
FAQs
What is the easiest method to combine neural networks?
The easiest method is ensemble learning, where multiple models are combined to improve performance and accuracy.
Can different types of neural networks be combined?
Yes, different types of neural networks, such as CNNs and RNNs, can be combined to leverage their unique strengths.
What are the typical challenges in combining neural networks?
Challenges include technical integration, data quality, and avoiding overfitting. Careful planning and validation are essential.
How does combining neural networks enhance performance?
Combining neural networks enhances performance by leveraging diverse models, reducing errors, and improving generalization.
Is combining neural networks beneficial for small datasets?
Yes, combining neural networks can be beneficial for small datasets, especially when using techniques like transfer learning to leverage knowledge from larger datasets.
#artificialintelligence#coding#raspberrypi#iot#stem#programming#science#arduinoproject#engineer#electricalengineering#robotic#robotica#machinelearning#electrical#diy#arduinouno#education#manufacturing#stemeducation#robotics#robot#technology#engineering#robots#arduino#electronics#automation#tech#innovation#ai
4 notes
·
View notes
Note
Have you found that you’ve been less motivated to create art now that AI has become so good?
I don’t really draw anymore because whenever I start a new drawing, I’m immediately plagued by thoughts like, why even bother? This piece is going to take hours when, theoretically, I could ask Mid-journey to do it for me and it would take about 10 seconds and probably look way better. So like, why should I even try?
I’m at college getting a degree in illustration but I’m afraid that by the time I graduate and get out into the field, I won’t have any job prospects. Human artists are becoming increasingly obsolete in the corporate world and I feel like nobody is going to want to hire me. I mean, from a shitty CEO’s perspective, why hire human artists when AI is right there? It’s faster and cheaper. Many established studio and corporate artists are already being fired in droves. We’re seeing it happen in real time.
I feel like I’m fighting a losing battle. AI has drained me of my creativity and my future job security. I’ve lost interest in one of my dearest hobbies and my degree may end up becoming completely useless. I loathe AI for the way it has stripped me of something I’ve dedicated so many years of my life to. Something that was once so precious to me.
I feel that I’ve spent thousands of hours honing a now useless skill. And that really sucks.
Sorry for ranting in your inbox, I hope you don’t mind… but since you are a working adult and do art and writing (of course writing AI has gotten stupid good as well and I’m bitter about that too) professionally, and as a hobby too, I figured that you would definitely understand.
Hey! This is a great question, and I have what I hope is a very hope-filled answer.
By the way, I don't call image generation "AI." It's not. There's no actual intelligence involved. It's an algorithm that averages images and combines them into something new. I refer to it as GenSlop.
First, the reason you're seeing such a proliferation of image generators attaching their dirty little claws into every website on the internet is due to what I call "just-in-casing." Rather than develop an ACTUAL ethical image generator (which would only use images from creative commons or pay artists for their use) generators like Deviantart's DreamUp and Twitter's Grok (?????? wtf is that name) have just stuffed LAION-5 into their code and called it a day.
Why? Why not wait and create an ethical dataset over several years?
Because it's become more likely than not than image generation is going to become strictly regulated by law, and companies like DA, Stability, Twitter, Adobe, and many others want to profit off it while it's still free and "legal."
I say "legal" in quotes, because at the moment, it's neither legal nor illegal. There are no laws in existence to govern this specific thing because it appeared so fast, there was literally no predicting it. So now it's in a legal grey area where it can't be prosecuted by US courts. (But it can be litigated--more on that in a bit.)
When laws are passed to govern the use of image generators, these companies that opted to use LAION-5 immediately without concern for the artists and communities they were harming will have to stop. but because of precedent, they will likely have their prior use of these generators forgiven, meaning they will not be forced to pay fines on their use before a certain date.
So while it seems they're popping up everywhere and taking over the art market, this is only so they can get in their share of profits from it before it becomes illegal to use them without compensation or consent.
But how do I know the law will support artists on this?
First, litigation. There are several huge lawsuits right now; one notable lawsuit against almost every major company using GenSlop technology with plaintiffs like Karla Ortiz and Grzegorz Rutkowski, among other high-profile artists. This lawsuit was recently """pared down""" or """mostly dismissed""" according to pro-GenSlop users, but what really happened is that the judge in the case asked the plaintiffs to amend their complaint to be more specific, which is generally a positive thing in cases like this. It means that precedent after a decision will be far clearer and have a longer reach than a more generalized complaint.
I don't know what pro-GenSloppers are insisting on spreading the "dismissal" tale on the internet, except to discourage actual artists. What they say has no bearing in the court, and it's looking more and more likely that the plaintiffs will be able to win this case and claim damages.
Getty Images, a huge image stock company, is also suing Stability AI for scraping its database. I'm not as well-versed on the case, though.
The other positive, despite what a lot of artists are saying, is the new SAG-AFTRA contract.
It's not perfect. It still allows GenSlop use. But it does require consent and compensation. Ideally, it would ban the use of artist images and voice entirely, but this contract is far better than what they would have gotten without striking. If you recall, before the strike, the AMPTP wanted to be able to use actor images and voices without any compensation or permission, without limitation.
And you can bet your ass that Hollywood isn't going to allow other organizations to have unregulated GenSlop use if they can't. They might even step in to argue against its use in front of congress, because their outlook is going to be "if we can't make money stealing art, no one else should be able to, either."
TL;DR: the huge proliferation of image generators and GenSlop right now is only because it's neither legal nor illegal. Regulations are coming, and artists will still be necessary and even required. Because the world is essentially built on a backbone or artistry.
I personally can't wait to drink the tears of all the techbros who can't steal art anymore.
7 notes
·
View notes
Text
Getting Machine Learning Accessible to Everyone: Breaking the Complexity Barrier
Machine learning has become an essential part of our daily lives, influencing how we interact with technology and impacting various industries. But, what exactly is machine learning? In simple terms, it's a subset of artificial intelligence (AI) that focuses on teaching computers to learn from data and make decisions without explicit programming. Now, let's delve deeper into this fascinating realm, exploring its core components, advantages, and real-world applications.
Imagine teaching a computer to differentiate between fruits like apples and oranges. Instead of handing it a list of rules, you provide it with numerous pictures of these fruits. The computer then seeks patterns in these images - perhaps noticing that apples are round and come in red or green hues, while oranges are round and orange in colour. After encountering many examples, the computer grasps the ability to distinguish between apples and oranges on its own. So, when shown a new fruit picture, it can decide whether it's an apple or an orange based on its learning. This is the essence of machine learning: computers learn from data and apply that learning to make decisions.
Key Concepts in Machine Learning
Algorithms: At the heart of machine learning are algorithms, mathematical models crafted to process data and provide insights or predictions. These algorithms fall into categories like supervised learning, unsupervised learning, and reinforcement learning, each serving distinct purposes.
Supervised Learning: This type of algorithm learns from labelled data, where inputs are matched with corresponding outputs. It learns the mapping between inputs and desired outputs, enabling accurate predictions on unseen data.
Unsupervised Learning: In contrast, unsupervised learning involves unlabelled data. This algorithm uncovers hidden patterns or relationships within the data, often revealing insights that weren't initially apparent.
Reinforcement Learning: This algorithm focuses on training agents to make sequential decisions by receiving rewards or penalties from the environment. It excels in complex scenarios such as autonomous driving or gaming.
Training and Testing Data: Training a machine learning model requires a substantial amount of data, divided into training and testing sets. The training data teaches the model patterns, while the testing data evaluates its performance and accuracy.
Feature Extraction and Engineering: Machine learning relies on features, specific attributes of data, to make predictions. Feature extraction involves selecting relevant features, while feature engineering creates new features to enhance model performance.
Benefits of Machine Learning
Machine learning brings numerous benefits that contribute to its widespread adoption:
Automation and Efficiency: By automating repetitive tasks and decision-making processes, machine learning boosts efficiency, allowing resources to be allocated strategically.
Accurate Predictions and Insights: Machine learning models analyse vast data sets to uncover patterns and make predictions, empowering businesses with informed decision-making.
Adaptability and Scalability: Machine learning models improve with more data, providing better results over time. They can scale to handle large datasets and complex problems.
Personalization and Customization: Machine learning enables personalized user experiences by analysing preferences and behaviour, fostering customer satisfaction.
Real-World Applications of Machine Learning
Machine learning is transforming various industries, driving innovation:
Healthcare: Machine learning aids in medical image analysis, disease diagnosis, drug discovery, and personalized medicine. It enhances patient outcomes and streamlines healthcare processes.
Finance: In finance, machine learning enhances fraud detection, credit scoring, and risk analysis. It supports data-driven decisions and optimization.
Retail and E-commerce: Machine learning powers recommendations, demand forecasting, and customer behaviour analysis, optimizing sales and enhancing customer experiences.
Transportation: Machine learning contributes to traffic prediction, autonomous vehicles, and supply chain optimization, improving efficiency and safety.
Incorporating machine learning into industries has transformed them. If you're interested in integrating machine learning into your business or learning more, consider expert guidance or specialized training, like that offered by ACTE institute. As technology advances, machine learning will continue shaping our future in unimaginable ways. Get ready to embrace its potential and transformative capabilities.
#machine learning ai#learn machine learning#machine learning#machine learning development company#technology#machine learning services
8 notes
·
View notes