#Medical Imaging Datasets
Explore tagged Tumblr posts
Text
Generating Chest X-Rays with AI: Insights from Christian Bluethgen | Episode 24 - Bytes of Innovation
Join Christian Bluethgen in this 32-minute webinar as he delves into RoentGen, an AI model synthesizing chest X-ray images from textual descriptions. Learn about its potential in medical imaging, benefits for rare disease data, and considerations regarding model limitations and ethical concerns.
#AI in Radiology#Real World Data#Real World Evidence#Real World Imaging Datasets#Medical Imaging Datasets#RWiD#AI in Healthcare
0 notes
Text
Top 19 Medical Datasets to Supercharge Your Machine Learning Models
#Healthcare Datasets#Medical Dataset#AI in Healthcare#machinelearning#artificialintelligence#dataannotation#EHR#Electronic health records#Medical imaging datasets
0 notes
Text
Hey, you know how I said there was nothing ethical about Adobe's approach to AI? Well whaddya know?
Adobe wants your team lead to contact their customer service to not have your private documents scraped!
This isn't the first of Adobe's always-online subscription-based products (which should not have been allowed in the first place) to have sneaky little scraping permissions auto-set to on and hidden away, but this is the first one (I'm aware of) where you have to contact customer service to turn it off for a whole team.
Now, I'm on record for saying I see scraping as fair use, and it is. But there's an aspect of that that is very essential to it being fair use: The material must be A) public facing and B) fixed published work.
All public facing published work is subject to transformative work and academic study, the use of mechanical apparatus to improve/accelerate that process does not change that principle. Its the difference between looking through someone's public instagram posts and reading through their drafts folder and DMs.
But that's not the kind of work that Adobe's interested in. See, they already have access to that work just like everyone else. But the in-progress work that Creative Cloud gives them access to, and the private work that's never published that's stored there isn't in LIAON. They want that advantage.
And that's valuable data. For an example: having a ton of snapshots of images in the process of being completed would be very handy for making an AI that takes incomplete work/sketches and 'finishes' it. That's on top of just being general dataset grist.
But that work is, definitionally, not published. There's no avenue to a fair use argument for scraping it, so they have to ask. And because they know it will be an unpopular ask, they make it a quiet op-out.
This was sinister enough when it was Photoshop, but PDF is mainly used for official documents and forms. That's tax documents, medical records, college applications, insurance documents, business records, legal documents. And because this is a server-side scrape, even if you opt-out, you have no guarantee that anyone you're sending those documents to has done so.
So, in case you weren't keeping score, corps like Adobe, Disney, Universal, Nintendo, etc all have the resources to make generative AI systems entirely with work they 'own' or can otherwise claim rights to, and no copyright argument can stop them because they own the copyrights.
They just don't want you to have access to it as a small creator to compete with them, and if they can expand copyright to cover styles and destroy fanworks they will. Here's a pic Adobe trying to do just that:
If you want to know more about fair use and why it applies in this circumstance, I recommend the Electronic Frontier Foundation over the Copyright Alliance.
184 notes
·
View notes
Note
whenever tumblr has a viral post about a "good" project or idea related to ai I just assume it's not going to work.
Yeah, mostly the ones I've seen have to do with medical stuff, and generally medical domains make it very hard to get ML to work. There's just so many barriers. You have HIPAA making it hard to get large datasets. For labelled datasets you also need to pay a doctor to label images, which is expensive because doctors are expensive. And unlike other domains, where it's perfectly okay to have a "good enough" answer, with medical stuff generally it has to be really accurate. So all these papers are trying to get 95% accuracy+ generalization on like 500 samples, when realistically they need 100x as much, minimum. So to be honest, I find these diagnostic applications way more morally suspect than any image gen network. Maybe in the future if they pass legislation to allow ML training on anonymized medical scans, it'll get a lot better, but until then I don't expect much.
The few tumblr-approved applications outside of medical stuff I can think of are a pretty bad idea as well but for different reasons. Mostly because they expect it to actually be accurate
11 notes
·
View notes
Note
Most importantly regarding AI, even if we ignored the entire discussion around "what is art? who can make it? what makes it valid?", the fact of the matter is that generative AI programs are inherently based on theft
Someone, some human(s), made the conscious decision to scrape the entire internet for literal billions of artworks, photos, videos, stories, blogs, social media posts, articles, copyrighted works, personal works, private works, private medical images. They took all of this data, crammed it all into a dataset for their generative programs to reference, and sold the idea of "look what a computer can 'create' from 'nothing' "
These programs do nothing on their own. They do not create spontaneously. They do not experiment or discover or express themselves. This is why they need data, a LOT of data, because they can only operate with designated user input, they can only regurgitate what has come before, they can only reference and repurpose what has come before. They steal from all of humanity, without due, without credit, without compensation or any sense of ethics, and the people vehemently selling the idea of AI are doing precisely that: selling it. They're exploiting the hard work, the identities, the privacy of billions of people for the sole purpose of making a quick, easy buck
In any sane world, the argument would end then and there, but unfortunately, we live in a world where are many of our laws are from the stone age (with people actively seeking to keep them there for fear of losing their power/influence). The creators of these AI programs are well-aware of these legal shortcomings, they have openly stated as such on numerous occasions, and are explicitly proud to be operating in this "legal grey area" because they know it means there are ill-gotten profits to be made
Regardless of whether or not a computer can genuinely make "art" or whether some person mashing words into a search bar is genuine "art", the fact of the matter is that it is objectively unethical in its current form. But, even that's an uphill battle to preach because too many people couldn't give a rats ass about ethics unless it personally affects them, and that's why we're in this position to begin with
I completely agree. It's entirely build on theft and it shouldn't exist. But as long as those who steal think they won't face any consequences and it brings them profit they won't stop. It's terrifying how so many people don't care about anything these days. It's just so frustrating to listen to all the excuses.
76 notes
·
View notes
Text

Virtual Pathologist
Image identification by machine learning models is a major application of artificial intelligence (AI). And, with ever-improving capabilities, the use of these models for medical diagnostics and research is becoming more commonplace. Doctors analysing X-rays and mammograms, for instance, are already being assisted by AI technology, and models trained to identify signs of disease in tissue sections are also being developed to help histopathologists. The models are trained with microscope images annotated by humans – the image, for example, shows a section of rat testis with signs of tubule atrophy (pale blue shapes) with other coloured shapes indicating normal tubules and structures. Once trained, the models are tasked with categorising unannotated datasets. The latest iteration of this technology was able to identify disease in testis, ovary, prostate and kidney samples with exceptional speed and high accuracy – in some cases finding signs of disease that even trained human pathologists had missed.
Written by Ruth Williams
Image from work by Colin Greeley and colleagues
Center for Reproductive Biology, School of Biological Sciences and the School of Electrical Engineering and Computer Science Washington State University, Pullman, WA, USA
Image originally published with a Creative Commons Attribution – NonCommercial – NoDerivs (CC BY-NC-ND 4.0)
Published in Scientific Reports, November 2024
You can also follow BPoD on Instagram, Twitter and Facebook
8 notes
·
View notes
Text
okay so like disregarding the horrid ethics of ai scraping without consent and compensation and the huge impact ai has on the environment, focusing solely on the claim companies love to make, that ai is a tool for artists, IF ai had stayed looking like shit, if the early days of dall e mini had been the extent of it's power, I could see it being a tool.
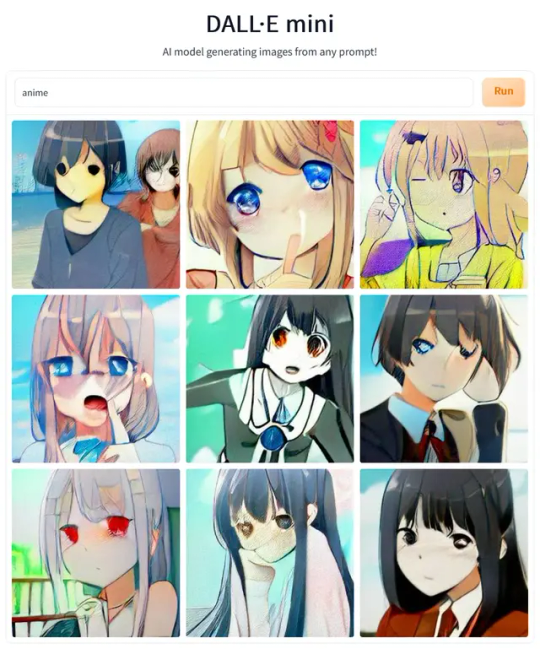
If it had stayed like this. Where everything is melting and hard to decipher, it could have been a tool specifically for practice. Because that's what it was when it first came out. Artists, before learning about the ethics of ai, saw these images and thought "wouldn't that be a fun exercise in interpretation?" and made redraws of these horrible awful images. It was a good exercise in creativity, it was fun to take these melting monstrosities and make them into something tangible. But then ai kept getting better at replicating actual images and now if you want a picture of an anime girl you can get one in just a few seconds and it looks like this
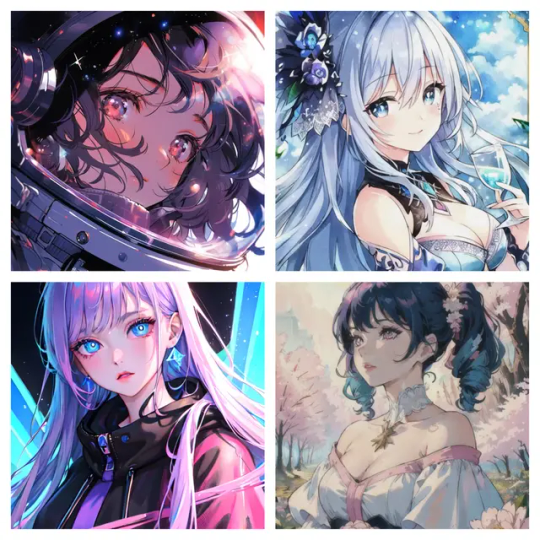
and that SUCKS. I can pick out the artists these images are pulling from, they have names and years of hard work under their belt and... what is there to do with these images really? This isn't a tool, there is no work to be done with them, they are, for all intents and purposes, the same as a finished illustration. The most an artist would do to these is make some small touch ups to make the blunders of ai like hair or clothing details not quite making sense, a little bit less noticeable. At that point it is not a tool. It does not aide the creative process, it takes it from the hands of the artist.
When ai gen images were first gaining traction and it still looked like shit. I did a few of these interpretation exercises and they were genuinely fun. The ai images were made by feeding Looking Glass AI some images of vocal synth character portraits and seeing what it spat out. And it spat out a lot of shit, girls with 8 arms and 4 legs and no head and hair for eyes and whatnot. So it was FUN and A TOOL because it looked so bad. Do I think the designs I interpreted from these are good? No not really. I think they could be if I did a few more passes to flesh them out, they aren't really something I'd usually design so it could be fun to keep letting them evolve, but as it stands they definitely need work.

But at the end of the day, saying all this, the fact of the matter is that ai image generation, in it's current state, regardless of what it looks like or how artists can or can't use it, doesn't matter. Because ai image generation is unethical. The scraping is unethical, the things that these datasets contain should be considered a violation of privacy considering some of them include pictures from MEDICAL DATA. And the environmental impact is absolutely abhorrent. The planet is already dying, do we need to speed it up for anime girl tiddies at the press of a button?
So if you're an artist looking to do an exercise like this where you look at something unintelligible and make sense of it with your pencil, what can you do? Well there's a few options really.
Having poor eyesight helps if you want to observe things in real life. take your glasses off and look around.
Look at the patterns in the paint on your ceiling or the stains on the floor or the marble texture of your counter top or the wood grain of a table or whatever else is around you.
Take poor quality screenshots of things you see online, you can even make the image quality worse digitally.
random paint or marker splotch doodle page. Draw over the shapes that random paint or marker marks make on a page.
take pictures of things from weird angles. distort them even more digitally if you want.
make collages in a photo app. I used to use pixlr in high school to make weird little pictures and while I never drew from them, they certainly changed the original images
I still think that if they ai looks bad enough that this kind of exercise can be fun and helpful if you're in a bit of an art block, and I do truly believe you can take inspiration from anything. I may take ai generated images that already exist on the internet, reference a few of them at once, and try to make something good out of them. But the way that ai exists right now, it just simply isn't the tool the companies are trying to sell you, and I most certainly won't be generating any new ai images any time soon.
7 notes
·
View notes
Text
had to do a presentation on an ai application for my final project (idk why? It wasn’t an ai class). Made it bearable by focusing on medical imaging which does actually have potential benefits and is done with closed datasets, so not copyrighted data (though there are still privacy concerns as with any medical research) and I even managed to find a good fibromyalgia study to squeeze in there and that gave me a few opportunities to talk about the value of self-reported pain levels and I even got to explain fibromyalgia to someone!
Had to listen to other people talk about generative ai but all in all it wasn’t too awful
Still wish that professor had just let us do a computational em project (the topic of the class) instead of making us do an ai project because then I could have used my research topic that I’m already working, but that would have been logical
#Generative ai is so stupid#But there are some real benefits to other types of AI in specific fields#Grad school#Gradblr#Electrical engineering#Women in stem
3 notes
·
View notes
Text
Future of Radiology & AI with Nina Kottler | Episode 23 Webcast
Radiology and AI expert Nina Kottler shares insights on how artificial intelligence is reshaping diagnostic imaging, clinical workflows, and radiologist roles. This episode of Bytes of Innovation dives into real-world impacts, emerging trends, and what's ahead for the field.
#Radiology in AI#Artificial Intelligence#Diagnostic Imaging#Clinical Worrkflows#Real World Imaging Datasets#Medical Imaging Datasets#RWiD#RWD
1 note
·
View note
Note
Genuine question: how does AI destroy the environment?
I'm totally with you on not using AI and that it harms artists but I've not heard the environment angle before.
I think a lot of people aren't aware of that because they don't think about how AI works.
While there are builds that can run on a local machine and even on phones, the way most people use generative machine learning systems like SD and ChatGPT is through a client (often a web client) where the actual processing takes place on remote servers. Those servers need power and cooling.
The processing power needed to generate millions and billions of images and text replies a day is immense, which means the power draw is horrendous. And all those servers need to be cooled. Often with water.
I think you see where there might be a severe environmental impact just from these factors alone. And for what?
Is "haha, funny image of my blorbo" really worth all that?
And then we're not even touching on the whole metric shitton of other ethical issues like the racism, sexism, ableism and mysoginy presented in the output or the medical data that's been scraped and put into the datasets or the fact that people are producing p*rn of real people and children or the potential legal ramifications of not being able to use photo evidence in legal procedings anymore or....
You know.
AI just all-round bad.
But there's still people justifying the use of it because "I'm just doing it for fun."
55 notes
·
View notes
Text
The Future of AI: What’s Next in Machine Learning and Deep Learning?
Artificial Intelligence (AI) has rapidly evolved over the past decade, transforming industries and redefining the way businesses operate. With machine learning and deep learning at the core of AI advancements, the future holds groundbreaking innovations that will further revolutionize technology. As machine learning and deep learning continue to advance, they will unlock new opportunities across various industries, from healthcare and finance to cybersecurity and automation. In this blog, we explore the upcoming trends and what lies ahead in the world of machine learning and deep learning.
1. Advancements in Explainable AI (XAI)
As AI models become more complex, understanding their decision-making process remains a challenge. Explainable AI (XAI) aims to make machine learning and deep learning models more transparent and interpretable. Businesses and regulators are pushing for AI systems that provide clear justifications for their outputs, ensuring ethical AI adoption across industries. The growing demand for fairness and accountability in AI-driven decisions is accelerating research into interpretable AI, helping users trust and effectively utilize AI-powered tools.
2. AI-Powered Automation in IT and Business Processes
AI-driven automation is set to revolutionize business operations by minimizing human intervention. Machine learning and deep learning algorithms can predict and automate tasks in various sectors, from IT infrastructure management to customer service and finance. This shift will increase efficiency, reduce costs, and improve decision-making. Businesses that adopt AI-powered automation will gain a competitive advantage by streamlining workflows and enhancing productivity through machine learning and deep learning capabilities.
3. Neural Network Enhancements and Next-Gen Deep Learning Models
Deep learning models are becoming more sophisticated, with innovations like transformer models (e.g., GPT-4, BERT) pushing the boundaries of natural language processing (NLP). The next wave of machine learning and deep learning will focus on improving efficiency, reducing computation costs, and enhancing real-time AI applications. Advancements in neural networks will also lead to better image and speech recognition systems, making AI more accessible and functional in everyday life.
4. AI in Edge Computing for Faster and Smarter Processing
With the rise of IoT and real-time processing needs, AI is shifting toward edge computing. This allows machine learning and deep learning models to process data locally, reducing latency and dependency on cloud services. Industries like healthcare, autonomous vehicles, and smart cities will greatly benefit from edge AI integration. The fusion of edge computing with machine learning and deep learning will enable faster decision-making and improved efficiency in critical applications like medical diagnostics and predictive maintenance.
5. Ethical AI and Bias Mitigation
AI systems are prone to biases due to data limitations and model training inefficiencies. The future of machine learning and deep learning will prioritize ethical AI frameworks to mitigate bias and ensure fairness. Companies and researchers are working towards AI models that are more inclusive and free from discriminatory outputs. Ethical AI development will involve strategies like diverse dataset curation, bias auditing, and transparent AI decision-making processes to build trust in AI-powered systems.
6. Quantum AI: The Next Frontier
Quantum computing is set to revolutionize AI by enabling faster and more powerful computations. Quantum AI will significantly accelerate machine learning and deep learning processes, optimizing complex problem-solving and large-scale simulations beyond the capabilities of classical computing. As quantum AI continues to evolve, it will open new doors for solving problems that were previously considered unsolvable due to computational constraints.
7. AI-Generated Content and Creative Applications
From AI-generated art and music to automated content creation, AI is making strides in the creative industry. Generative AI models like DALL-E and ChatGPT are paving the way for more sophisticated and human-like AI creativity. The future of machine learning and deep learning will push the boundaries of AI-driven content creation, enabling businesses to leverage AI for personalized marketing, video editing, and even storytelling.
8. AI in Cybersecurity: Real-Time Threat Detection
As cyber threats evolve, AI-powered cybersecurity solutions are becoming essential. Machine learning and deep learning models can analyze and predict security vulnerabilities, detecting threats in real time. The future of AI in cybersecurity lies in its ability to autonomously defend against sophisticated cyberattacks. AI-powered security systems will continuously learn from emerging threats, adapting and strengthening defense mechanisms to ensure data privacy and protection.
9. The Role of AI in Personalized Healthcare
One of the most impactful applications of machine learning and deep learning is in healthcare. AI-driven diagnostics, predictive analytics, and drug discovery are transforming patient care. AI models can analyze medical images, detect anomalies, and provide early disease detection, improving treatment outcomes. The integration of machine learning and deep learning in healthcare will enable personalized treatment plans and faster drug development, ultimately saving lives.
10. AI and the Future of Autonomous Systems
From self-driving cars to intelligent robotics, machine learning and deep learning are at the forefront of autonomous technology. The evolution of AI-powered autonomous systems will improve safety, efficiency, and decision-making capabilities. As AI continues to advance, we can expect self-learning robots, smarter logistics systems, and fully automated industrial processes that enhance productivity across various domains.
Conclusion
The future of AI, machine learning and deep learning is brimming with possibilities. From enhancing automation to enabling ethical and explainable AI, the next phase of AI development will drive unprecedented innovation. Businesses and tech leaders must stay ahead of these trends to leverage AI's full potential. With continued advancements in machine learning and deep learning, AI will become more intelligent, efficient, and accessible, shaping the digital world like never before.
Are you ready for the AI-driven future? Stay updated with the latest AI trends and explore how these advancements can shape your business!
#artificial intelligence#machine learning#techinnovation#tech#technology#web developers#ai#web#deep learning#Information and technology#IT#ai future
2 notes
·
View notes
Text
Harrison.ai raises $112 million to expand AI-powered medical diagnostics globally

- By InnoNurse Staff -
Harrison.ai, a Sydney, Australia-based startup, develops AI-powered diagnostic software for radiology and pathology to enhance disease detection and streamline workflows for healthcare professionals.
The company recently secured $112 million in Series C funding to expand internationally, with plans to establish a presence in Boston.
Founded in 2018 by brothers Dr. Aengus Tran and Dimitry Tran, Harrison.ai has launched Annalise.ai for radiology and Franklin.ai for pathology, aiming to address global clinician shortages and improve patient outcomes. Its products are deployed in over 1,000 healthcare facilities across 15 countries, with regulatory clearance in 40 markets, including 12 FDA approvals in the U.S.
The startup differentiates itself from competitors like Aidoc and Rad AI through its broader diagnostic capabilities and extensive datasets. Its AI models detect lung cancer earlier and outperform standard radiology exams. With its latest funding, Harrison.ai plans to expand AI automation beyond radiology and pathology.
Read more at TechCrunch
///
Other recent news and insights
Advanced imaging and AI detect smoking-related toxins in placenta samples (Rice University/Medical Xpress)
Study suggests AI may outperform humans in analyzing long-term ECG recordings (Lund University)
#ai#radiology#medtech#harrison ai#imaging#medical imaging#australia#health tech#pathology#automation#diagnostics
5 notes
·
View notes
Text
What is Artificial Intelligence?? A Beginner's Guide to Understand Artificial Intelligence
1) What is Artificial Intelligence (AI)??
Artificial Intelligence (AI) is a set of technologies that enables computer to perform tasks normally performed by humans. This includes the ability to learn (machine learning) reasoning, decision making and even natural language processing from virtual assistants like Siri and Alexa to prediction algorithms on Netflix and Google Maps.
The foundation of the AI lies in its ability to simulate cognitive tasks. Unlike traditional programming where machines follow clear instructions, AI systems use vast algorithms and datasets to recognize patterns, identify trends and automatically improve over time.
2) Many Artificial Intelligence (AI) faces
Artificial Intelligence (AI) isn't one thing but it is a term that combines many different technologies together. Understanding its ramifications can help you understand its versatility:
Machine Learning (ML): At its core, AI focuses on enabling ML machines to learn from data and make improvements without explicit programming. Applications range from spam detection to personalized shopping recommendations.
Computer Vision: This field enables machines to interpret and analyze image data from facial recognition to medical image diagnosis. Computer Vision is revolutionizing many industries.
Robotics: By combining AI with Engineering Robotics focuses on creating intelligent machines that can perform tasks automatically or with minimal human intervention.
Creative AI: Tools like ChatGPT and DALL-E fail into this category. Create human like text or images and opens the door to creative and innovative possibilities.
3) Why is AI so popular now??
The Artificial Intelligence (AI) explosion may be due to a confluence of technological advances:
Big Data: The digital age creates unprecedented amounts of data. Artificial Intelligence (AI) leverages data and uses it to gain insights and improve decision making.
Improved Algorithms: Innovations in algorithms make Artificial Intelligence (AI) models more efficient and accurate.
Computing Power: The rise of cloud computing and GPUs has provided the necessary infrastructure for processing complex AI models.
Access: The proliferation of publicly available datasets (eg: ImageNet, Common Crawl) has provided the basis for training complex AI Systems. Various Industries also collect a huge amount of proprietary data. This makes it possible to deploy domain specific AI applications.
4) Interesting Topics about Artificial Intelligence (AI)
Real World applications of AI shows that AI is revolutionizing industries such as Healthcare (primary diagnosis and personalized machine), finance (fraud detection and robo advisors), education (adaptive learning platforms) and entertainment (adaptive platforms) how??
The role of AI in "Creativity Explore" on how AI tools like DALL-E and ChatGPT are helping artists, writers and designers create incredible work. Debate whether AI can truly be creative or just enhance human creativity.
AI ethics and Bias are an important part of AI decision making, it is important to address issues such as bias, transparency and accountability. Search deeper into the importance of ethical AI and its impact on society.
AI in everyday life about how little known AI is affecting daily life, from increasing energy efficiency in your smart home to reading the forecast on your smartphone.
The future of AI anticipate upcoming advance services like Quantum AI and their potential to solve humanity's biggest challenges like climate change and pandemics.
5) Conclusion
Artificial Intelligence (AI) isn't just a technological milestone but it is a paradigm shift that continues to redefine our future. As you explore the vast world of AI, think outside the box to find nuances, applications and challenges with well researched and engaging content
Whether unpacking how AI works or discussing its transformative potential, this blog can serve as a beacon for those eager to understand this underground branch.
"As we stand on the brink of an AI-powered future, the real question isn't what AI can do for us, but what we dare to imagine next"
"Get Latest News on www.bloggergaurang.com along with Breaking News and Top Headlines from all around the World !!"
2 notes
·
View notes
Text
Despite uncovering widespread AI errors in healthcare, Ziad remained optimistic about how algorithms might help to care better for all patients. He felt they could be particularly useful in improving diagnostics that doctors tended to get wrong, but also in improving our current medical knowledge by discovering new patterns in medical data. Most modern healthcare AI is trained on doctors’ diagnoses, which Ziad felt wasn’t enough. ‘If we want AI algorithms to teach us new things,’ he said, ‘that means we can’t train them to learn just from doctors, because then it sets a very low ceiling – they can only teach us what we already know, possibly more cheaply and more efficiently.’ Rather than use AI as an alternative to human doctors – who weren’t as scarce as in rural India – he wanted to use the technology to augment what the best doctors could do.
[….]
To solve the mystery, Ziad had to return to first principles. He wanted to build a software that could predict a patient’s pain levels based on their X-ray scans. But rather than training the machine-learning algorithms to learn from doctors with their own intrinsic biases and blind spots, he trained them on patients’ self-reports. To do this, he acquired a training dataset from the US National Institutes of Health, a set of knee X-rays annotated with patients’ own descriptions of their pain levels, rather than simply a radiologist’s classification. The arthritis pain model he built found correlations between X-ray images and pain descriptions. He then used it to predict how severe a new patient’s knee pain was, from their X-ray. His goal wasn’t to build a commercial app, but to carry out a scientific experiment.
It turned out that the algorithms trained on patients’ own reported pain did a far better job than a human radiologist in predicting which knees were more painful.
The most striking outcome was that Ziad’s pain model outperformed human radiologists at predicting pain in African American patients. ‘The algorithms were seeing signals in the knee X-ray that the radiologist was missing, and those signals were disproportionately present in black patients and not white patients,’ he said. The research was published in 2021, and concluded: ‘Because algorithmic severity measures better capture underserved patients’ pain, and severity measures influence treatment decisions, algorithmic predictions could potentially redress disparities in access to treatments like arthroplasty.’
Meanwhile, Ziad plans to dig deeper to decode what those signals are. He is using machine-learning techniques to investigate what is causing excess pain using MRIs and samples of cartilage or bone in the lab. If he finds explanations, AI may have helped to discover something new about human physiology and neuroscience that would have otherwise been ignored.
— Madhumita Murgia, Code Dependent: Living in the Shadow of AI
2 notes
·
View notes
Text
The Role of Photon Insights in Helps In Academic Research
In recent times, the integration of Artificial Intelligence (AI) with academic study has been gaining significant momentum that offers transformative opportunities across different areas. One area in which AI has a significant impact is in the field of photonics, the science of producing as well as manipulating and sensing photos that can be used in medical, telecommunications, and materials sciences. It also reveals its ability to enhance the analysis of data, encourage collaboration, and propel the development of new technologies.
Understanding the Landscape of Photonics
Photonics covers a broad range of technologies, ranging from fibre optics and lasers to sensors and imaging systems. As research in this field gets more complicated and complex, the need for sophisticated analytical tools becomes essential. The traditional methods of data processing and interpretation could be slow and inefficient and often slow the pace of discovery. This is where AI is emerging as a game changer with robust solutions that improve research processes and reveal new knowledge.
Researchers can, for instance, use deep learning methods to enhance image processing in applications such as biomedical imaging. AI-driven algorithms can improve the image’s resolution, cut down on noise, and even automate feature extraction, which leads to more precise diagnosis. Through automation of this process, experts are able to concentrate on understanding results, instead of getting caught up with managing data.
Accelerating Material Discovery
Research in the field of photonics often involves investigation of new materials, like photonic crystals, or metamaterials that can drastically alter the propagation of light. Methods of discovery for materials are time-consuming and laborious and often require extensive experiments and testing. AI can speed up the process through the use of predictive models and simulations.
Facilitating Collaboration
In a time when interdisciplinary collaboration is vital, AI tools are bridging the gap between researchers from various disciplines. The research conducted in the field of photonics typically connects with fields like engineering, computer science, and biology. AI-powered platforms aid in this collaboration by providing central databases and sharing information, making it easier for researchers to gain access to relevant data and tools.
Cloud-based AI solutions are able to provide shared datasets, which allows researchers to collaborate with no limitations of geographic limitations. Collaboration is essential in photonics, where the combination of diverse knowledge can result in revolutionary advances in technology and its applications.
Automating Experimental Procedures
Automation is a third area in which AI is becoming a major factor in the field of academic research in the field of photonics. The automated labs equipped with AI-driven technology can carry out experiments with no human involvement. The systems can alter parameters continuously based on feedback, adjusting conditions for experiments to produce the highest quality outcomes.
Furthermore, robotic systems that are integrated with AI can perform routine tasks like sampling preparation and measurement. This is not just more efficient but also decreases errors made by humans, which results in more accurate results. Through automation researchers can devote greater time for analysis as well as development which will speed up the overall research process.
Predictive Analytics for Research Trends
The predictive capabilities of AI are crucial for analyzing and predicting research trends in the field of photonics. By studying the literature that is already in use as well as research outputs, AI algorithms can pinpoint new themes and areas of research. This insight can assist researchers to prioritize their work and identify emerging trends that could be destined to be highly impactful.
For organizations and funding bodies These insights are essential to allocate resources as well as strategic plans. If they can understand where research is heading, they are able to help support research projects that are in line with future requirements, ultimately leading to improvements that benefit the entire society.
Ethical Considerations and Challenges
While the advantages of AI in speeding up research in photonics are evident however, ethical considerations need to be taken into consideration. Questions like privacy of data and bias in algorithmic computation, as well as the possibility of misuse by AI technology warrant careful consideration. Institutions and researchers must adopt responsible AI practices to ensure that the applications they use enhance human decision-making and not substitute it.
In addition, the incorporation in the use of AI into academic studies calls for the level of digital literacy which not every researcher are able to attain. Therefore, investing in education and education about AI methods and tools is vital to reap the maximum potential advantages.
Conclusion
The significance of AI in speeding up research at universities, especially in the field of photonics, is extensive and multifaceted. Through improving data analysis and speeding up the discovery of materials, encouraging collaboration, facilitating experimental procedures and providing insights that are predictive, AI is reshaping the research landscape. As the area of photonics continues to grow, the integration of AI technologies is certain to be a key factor in fostering innovation and expanding our knowledge of applications based on light.
Through embracing these developments scientists can open up new possibilities for research, which ultimately lead to significant scientific and technological advancements. As we move forward on this new frontier, interaction with AI as well as academic researchers will prove essential to address the challenges and opportunities ahead. The synergy between these two disciplines will not only speed up discovery in photonics, but also has the potential to change our understanding of and interaction with the world that surrounds us.
2 notes
·
View notes
Text
Mastering Neural Networks: A Deep Dive into Combining Technologies
How Can Two Trained Neural Networks Be Combined?
Introduction
In the ever-evolving world of artificial intelligence (AI), neural networks have emerged as a cornerstone technology, driving advancements across various fields. But have you ever wondered how combining two trained neural networks can enhance their performance and capabilities? Let’s dive deep into the fascinating world of neural networks and explore how combining them can open new horizons in AI.
Basics of Neural Networks
What is a Neural Network?
Neural networks, inspired by the human brain, consist of interconnected nodes or "neurons" that work together to process and analyze data. These networks can identify patterns, recognize images, understand speech, and even generate human-like text. Think of them as a complex web of connections where each neuron contributes to the overall decision-making process.
How Neural Networks Work
Neural networks function by receiving inputs, processing them through hidden layers, and producing outputs. They learn from data by adjusting the weights of connections between neurons, thus improving their ability to predict or classify new data. Imagine a neural network as a black box that continuously refines its understanding based on the information it processes.
Types of Neural Networks
From simple feedforward networks to complex convolutional and recurrent networks, neural networks come in various forms, each designed for specific tasks. Feedforward networks are great for straightforward tasks, while convolutional neural networks (CNNs) excel in image recognition, and recurrent neural networks (RNNs) are ideal for sequential data like text or speech.
Why Combine Neural Networks?
Advantages of Combining Neural Networks
Combining neural networks can significantly enhance their performance, accuracy, and generalization capabilities. By leveraging the strengths of different networks, we can create a more robust and versatile model. Think of it as assembling a team where each member brings unique skills to tackle complex problems.
Applications in Real-World Scenarios
In real-world applications, combining neural networks can lead to breakthroughs in fields like healthcare, finance, and autonomous systems. For example, in medical diagnostics, combining networks can improve the accuracy of disease detection, while in finance, it can enhance the prediction of stock market trends.
Methods of Combining Neural Networks
Ensemble Learning
Ensemble learning involves training multiple neural networks and combining their predictions to improve accuracy. This approach reduces the risk of overfitting and enhances the model's generalization capabilities.
Bagging
Bagging, or Bootstrap Aggregating, trains multiple versions of a model on different subsets of the data and combines their predictions. This method is simple yet effective in reducing variance and improving model stability.
Boosting
Boosting focuses on training sequential models, where each model attempts to correct the errors of its predecessor. This iterative process leads to a powerful combined model that performs well even on difficult tasks.
Stacking
Stacking involves training multiple models and using a "meta-learner" to combine their outputs. This technique leverages the strengths of different models, resulting in superior overall performance.
Transfer Learning
Transfer learning is a method where a pre-trained neural network is fine-tuned on a new task. This approach is particularly useful when data is scarce, allowing us to leverage the knowledge acquired from previous tasks.
Concept of Transfer Learning
In transfer learning, a model trained on a large dataset is adapted to a smaller, related task. For instance, a model trained on millions of images can be fine-tuned to recognize specific objects in a new dataset.
How to Implement Transfer Learning
To implement transfer learning, we start with a pretrained model, freeze some layers to retain their knowledge, and fine-tune the remaining layers on the new task. This method saves time and computational resources while achieving impressive results.
Advantages of Transfer Learning
Transfer learning enables quicker training times and improved performance, especially when dealing with limited data. It’s like standing on the shoulders of giants, leveraging the vast knowledge accumulated from previous tasks.
Neural Network Fusion
Neural network fusion involves merging multiple networks into a single, unified model. This method combines the strengths of different architectures to create a more powerful and versatile network.
Definition of Neural Network Fusion
Neural network fusion integrates different networks at various stages, such as combining their outputs or merging their internal layers. This approach can enhance the model's ability to handle diverse tasks and data types.
Types of Neural Network Fusion
There are several types of neural network fusion, including early fusion, where networks are combined at the input level, and late fusion, where their outputs are merged. Each type has its own advantages depending on the task at hand.
Implementing Fusion Techniques
To implement neural network fusion, we can combine the outputs of different networks using techniques like averaging, weighted voting, or more sophisticated methods like learning a fusion model. The choice of technique depends on the specific requirements of the task.
Cascade Network
Cascade networks involve feeding the output of one neural network as input to another. This approach creates a layered structure where each network focuses on different aspects of the task.
What is a Cascade Network?
A cascade network is a hierarchical structure where multiple networks are connected in series. Each network refines the outputs of the previous one, leading to progressively better performance.
Advantages and Applications of Cascade Networks
Cascade networks are particularly useful in complex tasks where different stages of processing are required. For example, in image processing, a cascade network can progressively enhance image quality, leading to more accurate recognition.
Practical Examples
Image Recognition
In image recognition, combining CNNs with ensemble methods can improve accuracy and robustness. For instance, a network trained on general image data can be combined with a network fine-tuned for specific object recognition, leading to superior performance.
Natural Language Processing
In natural language processing (NLP), combining RNNs with transfer learning can enhance the understanding of text. A pre-trained language model can be fine-tuned for specific tasks like sentiment analysis or text generation, resulting in more accurate and nuanced outputs.
Predictive Analytics
In predictive analytics, combining different types of networks can improve the accuracy of predictions. For example, a network trained on historical data can be combined with a network that analyzes real-time data, leading to more accurate forecasts.
Challenges and Solutions
Technical Challenges
Combining neural networks can be technically challenging, requiring careful tuning and integration. Ensuring compatibility between different networks and avoiding overfitting are critical considerations.
Data Challenges
Data-related challenges include ensuring the availability of diverse and high-quality data for training. Managing data complexity and avoiding biases are essential for achieving accurate and reliable results.
Possible Solutions
To overcome these challenges, it’s crucial to adopt a systematic approach to model integration, including careful preprocessing of data and rigorous validation of models. Utilizing advanced tools and frameworks can also facilitate the process.
Tools and Frameworks
Popular Tools for Combining Neural Networks
Tools like TensorFlow, PyTorch, and Keras provide extensive support for combining neural networks. These platforms offer a wide range of functionalities and ease of use, making them ideal for both beginners and experts.
Frameworks to Use
Frameworks like Scikit-learn, Apache MXNet, and Microsoft Cognitive Toolkit offer specialized support for ensemble learning, transfer learning, and neural network fusion. These frameworks provide robust tools for developing and deploying combined neural network models.
Future of Combining Neural Networks
Emerging Trends
Emerging trends in combining neural networks include the use of advanced ensemble techniques, the integration of neural networks with other AI models, and the development of more sophisticated fusion methods.
Potential Developments
Future developments may include the creation of more powerful and efficient neural network architectures, enhanced transfer learning techniques, and the integration of neural networks with other technologies like quantum computing.
Case Studies
Successful Examples in Industry
In healthcare, combining neural networks has led to significant improvements in disease diagnosis and treatment recommendations. For example, combining CNNs with RNNs has enhanced the accuracy of medical image analysis and patient monitoring.
Lessons Learned from Case Studies
Key lessons from successful case studies include the importance of data quality, the need for careful model tuning, and the benefits of leveraging diverse neural network architectures to address complex problems.
Online Course
I have came across over many online courses. But finally found something very great platform to save your time and money.
1.Prag Robotics_ TBridge
2.Coursera
Best Practices
Strategies for Effective Combination
Effective strategies for combining neural networks include using ensemble methods to enhance performance, leveraging transfer learning to save time and resources, and adopting a systematic approach to model integration.
Avoiding Common Pitfalls
Common pitfalls to avoid include overfitting, ignoring data quality, and underestimating the complexity of model integration. By being aware of these challenges, we can develop more robust and effective combined neural network models.
Conclusion
Combining two trained neural networks can significantly enhance their capabilities, leading to more accurate and versatile AI models. Whether through ensemble learning, transfer learning, or neural network fusion, the potential benefits are immense. By adopting the right strategies and tools, we can unlock new possibilities in AI and drive advancements across various fields.
FAQs
What is the easiest method to combine neural networks?
The easiest method is ensemble learning, where multiple models are combined to improve performance and accuracy.
Can different types of neural networks be combined?
Yes, different types of neural networks, such as CNNs and RNNs, can be combined to leverage their unique strengths.
What are the typical challenges in combining neural networks?
Challenges include technical integration, data quality, and avoiding overfitting. Careful planning and validation are essential.
How does combining neural networks enhance performance?
Combining neural networks enhances performance by leveraging diverse models, reducing errors, and improving generalization.
Is combining neural networks beneficial for small datasets?
Yes, combining neural networks can be beneficial for small datasets, especially when using techniques like transfer learning to leverage knowledge from larger datasets.
#artificialintelligence#coding#raspberrypi#iot#stem#programming#science#arduinoproject#engineer#electricalengineering#robotic#robotica#machinelearning#electrical#diy#arduinouno#education#manufacturing#stemeducation#robotics#robot#technology#engineering#robots#arduino#electronics#automation#tech#innovation#ai
4 notes
·
View notes