#Meta’s Code Llama
Explore tagged Tumblr posts
Text
7 دروس عن الحب والحياة تعلَّمها دانيال جونز من قراءة مِئتي ألف قصّة حبٍ
ما هذه المجموعة من المختارات تسألني؟ إنّها عددٌ من أعداد نشرة “صيد الشابكة” اِعرف أكثر عن النشرة هنا: ما هي نشرة “صيد الشابكة” ما مصادرها، وما غرضها؛ وما معنى الشابكة أصلًا؟! 🎣🌐هل تعرف ما هي صيد الشابكة وتطالعها بانتظام؟ اِدعم استمرارية النشرة بطرق شتى من هنا: 💲 طرق دعم نشرة صيد الشابكة. 🎣🌐 صيد الشابكة العدد #173 السلام عليكم؛ مرحبًا وبسم الله؛ بخصوص العنوان فستجده ضمن قسمه الخاصّ أدناه. 🎣🌐…
#173#Caitlin Dewey#Daniel Jones#Fortran#Marie Dollé#Meta’s Code Llama#MIT#The New York Times Company#مجلة العلوم الانسانية - جامعة الاسراء غزة#الاتحاد العالمي للمؤسسات العلمية#ستانفورد للابتكار الاجتماعي
0 notes
Text
"Open" "AI" isn’t
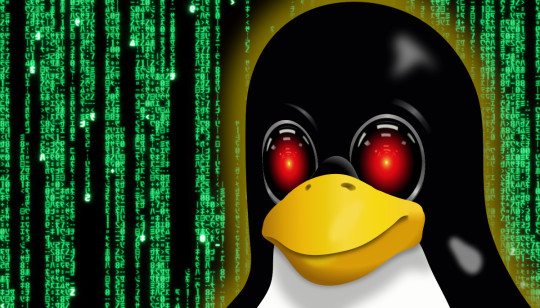
Tomorrow (19 Aug), I'm appearing at the San Diego Union-Tribune Festival of Books. I'm on a 2:30PM panel called "Return From Retirement," followed by a signing:
https://www.sandiegouniontribune.com/festivalofbooks
The crybabies who freak out about The Communist Manifesto appearing on university curriculum clearly never read it – chapter one is basically a long hymn to capitalism's flexibility and inventiveness, its ability to change form and adapt itself to everything the world throws at it and come out on top:
https://www.marxists.org/archive/marx/works/1848/communist-manifesto/ch01.htm#007
Today, leftists signal this protean capacity of capital with the -washing suffix: greenwashing, genderwashing, queerwashing, wokewashing – all the ways capital cloaks itself in liberatory, progressive values, while still serving as a force for extraction, exploitation, and political corruption.
A smart capitalist is someone who, sensing the outrage at a world run by 150 old white guys in boardrooms, proposes replacing half of them with women, queers, and people of color. This is a superficial maneuver, sure, but it's an incredibly effective one.
In "Open (For Business): Big Tech, Concentrated Power, and the Political Economy of Open AI," a new working paper, Meredith Whittaker, David Gray Widder and Sarah B Myers document a new kind of -washing: openwashing:
https://papers.ssrn.com/sol3/papers.cfm?abstract_id=4543807
Openwashing is the trick that large "AI" companies use to evade regulation and neutralizing critics, by casting themselves as forces of ethical capitalism, committed to the virtue of openness. No one should be surprised to learn that the products of the "open" wing of an industry whose products are neither "artificial," nor "intelligent," are also not "open." Every word AI huxters say is a lie; including "and," and "the."
So what work does the "open" in "open AI" do? "Open" here is supposed to invoke the "open" in "open source," a movement that emphasizes a software development methodology that promotes code transparency, reusability and extensibility, which are three important virtues.
But "open source" itself is an offshoot of a more foundational movement, the Free Software movement, whose goal is to promote freedom, and whose method is openness. The point of software freedom was technological self-determination, the right of technology users to decide not just what their technology does, but who it does it to and who it does it for:
https://locusmag.com/2022/01/cory-doctorow-science-fiction-is-a-luddite-literature/
The open source split from free software was ostensibly driven by the need to reassure investors and businesspeople so they would join the movement. The "free" in free software is (deliberately) ambiguous, a bit of wordplay that sometimes misleads people into thinking it means "Free as in Beer" when really it means "Free as in Speech" (in Romance languages, these distinctions are captured by translating "free" as "libre" rather than "gratis").
The idea behind open source was to rebrand free software in a less ambiguous – and more instrumental – package that stressed cost-savings and software quality, as well as "ecosystem benefits" from a co-operative form of development that recruited tinkerers, independents, and rivals to contribute to a robust infrastructural commons.
But "open" doesn't merely resolve the linguistic ambiguity of libre vs gratis – it does so by removing the "liberty" from "libre," the "freedom" from "free." "Open" changes the pole-star that movement participants follow as they set their course. Rather than asking "Which course of action makes us more free?" they ask, "Which course of action makes our software better?"
Thus, by dribs and drabs, the freedom leeches out of openness. Today's tech giants have mobilized "open" to create a two-tier system: the largest tech firms enjoy broad freedom themselves – they alone get to decide how their software stack is configured. But for all of us who rely on that (increasingly unavoidable) software stack, all we have is "open": the ability to peer inside that software and see how it works, and perhaps suggest improvements to it:
https://www.youtube.com/watch?v=vBknF2yUZZ8
In the Big Tech internet, it's freedom for them, openness for us. "Openness" – transparency, reusability and extensibility – is valuable, but it shouldn't be mistaken for technological self-determination. As the tech sector becomes ever-more concentrated, the limits of openness become more apparent.
But even by those standards, the openness of "open AI" is thin gruel indeed (that goes triple for the company that calls itself "OpenAI," which is a particularly egregious openwasher).
The paper's authors start by suggesting that the "open" in "open AI" is meant to imply that an "open AI" can be scratch-built by competitors (or even hobbyists), but that this isn't true. Not only is the material that "open AI" companies publish insufficient for reproducing their products, even if those gaps were plugged, the resource burden required to do so is so intense that only the largest companies could do so.
Beyond this, the "open" parts of "open AI" are insufficient for achieving the other claimed benefits of "open AI": they don't promote auditing, or safety, or competition. Indeed, they often cut against these goals.
"Open AI" is a wordgame that exploits the malleability of "open," but also the ambiguity of the term "AI": "a grab bag of approaches, not… a technical term of art, but more … marketing and a signifier of aspirations." Hitching this vague term to "open" creates all kinds of bait-and-switch opportunities.
That's how you get Meta claiming that LLaMa2 is "open source," despite being licensed in a way that is absolutely incompatible with any widely accepted definition of the term:
https://blog.opensource.org/metas-llama-2-license-is-not-open-source/
LLaMa-2 is a particularly egregious openwashing example, but there are plenty of other ways that "open" is misleadingly applied to AI: sometimes it means you can see the source code, sometimes that you can see the training data, and sometimes that you can tune a model, all to different degrees, alone and in combination.
But even the most "open" systems can't be independently replicated, due to raw computing requirements. This isn't the fault of the AI industry – the computational intensity is a fact, not a choice – but when the AI industry claims that "open" will "democratize" AI, they are hiding the ball. People who hear these "democratization" claims (especially policymakers) are thinking about entrepreneurial kids in garages, but unless these kids have access to multi-billion-dollar data centers, they can't be "disruptors" who topple tech giants with cool new ideas. At best, they can hope to pay rent to those giants for access to their compute grids, in order to create products and services at the margin that rely on existing products, rather than displacing them.
The "open" story, with its claims of democratization, is an especially important one in the context of regulation. In Europe, where a variety of AI regulations have been proposed, the AI industry has co-opted the open source movement's hard-won narrative battles about the harms of ill-considered regulation.
For open source (and free software) advocates, many tech regulations aimed at taming large, abusive companies – such as requirements to surveil and control users to extinguish toxic behavior – wreak collateral damage on the free, open, user-centric systems that we see as superior alternatives to Big Tech. This leads to the paradoxical effect of passing regulation to "punish" Big Tech that end up simply shaving an infinitesimal percentage off the giants' profits, while destroying the small co-ops, nonprofits and startups before they can grow to be a viable alternative.
The years-long fight to get regulators to understand this risk has been waged by principled actors working for subsistence nonprofit wages or for free, and now the AI industry is capitalizing on lawmakers' hard-won consideration for collateral damage by claiming to be "open AI" and thus vulnerable to overbroad regulation.
But the "open" projects that lawmakers have been coached to value are precious because they deliver a level playing field, competition, innovation and democratization – all things that "open AI" fails to deliver. The regulations the AI industry is fighting also don't necessarily implicate the speech implications that are core to protecting free software:
https://www.eff.org/deeplinks/2015/04/remembering-case-established-code-speech
Just think about LLaMa-2. You can download it for free, along with the model weights it relies on – but not detailed specs for the data that was used in its training. And the source-code is licensed under a homebrewed license cooked up by Meta's lawyers, a license that only glancingly resembles anything from the Open Source Definition:
https://opensource.org/osd/
Core to Big Tech companies' "open AI" offerings are tools, like Meta's PyTorch and Google's TensorFlow. These tools are indeed "open source," licensed under real OSS terms. But they are designed and maintained by the companies that sponsor them, and optimize for the proprietary back-ends each company offers in its own cloud. When programmers train themselves to develop in these environments, they are gaining expertise in adding value to a monopolist's ecosystem, locking themselves in with their own expertise. This a classic example of software freedom for tech giants and open source for the rest of us.
One way to understand how "open" can produce a lock-in that "free" might prevent is to think of Android: Android is an open platform in the sense that its sourcecode is freely licensed, but the existence of Android doesn't make it any easier to challenge the mobile OS duopoly with a new mobile OS; nor does it make it easier to switch from Android to iOS and vice versa.
Another example: MongoDB, a free/open database tool that was adopted by Amazon, which subsequently forked the codebase and tuning it to work on their proprietary cloud infrastructure.
The value of open tooling as a stickytrap for creating a pool of developers who end up as sharecroppers who are glued to a specific company's closed infrastructure is well-understood and openly acknowledged by "open AI" companies. Zuckerberg boasts about how PyTorch ropes developers into Meta's stack, "when there are opportunities to make integrations with products, [so] it’s much easier to make sure that developers and other folks are compatible with the things that we need in the way that our systems work."
Tooling is a relatively obscure issue, primarily debated by developers. A much broader debate has raged over training data – how it is acquired, labeled, sorted and used. Many of the biggest "open AI" companies are totally opaque when it comes to training data. Google and OpenAI won't even say how many pieces of data went into their models' training – let alone which data they used.
Other "open AI" companies use publicly available datasets like the Pile and CommonCrawl. But you can't replicate their models by shoveling these datasets into an algorithm. Each one has to be groomed – labeled, sorted, de-duplicated, and otherwise filtered. Many "open" models merge these datasets with other, proprietary sets, in varying (and secret) proportions.
Quality filtering and labeling for training data is incredibly expensive and labor-intensive, and involves some of the most exploitative and traumatizing clickwork in the world, as poorly paid workers in the Global South make pennies for reviewing data that includes graphic violence, rape, and gore.
Not only is the product of this "data pipeline" kept a secret by "open" companies, the very nature of the pipeline is likewise cloaked in mystery, in order to obscure the exploitative labor relations it embodies (the joke that "AI" stands for "absent Indians" comes out of the South Asian clickwork industry).
The most common "open" in "open AI" is a model that arrives built and trained, which is "open" in the sense that end-users can "fine-tune" it – usually while running it on the manufacturer's own proprietary cloud hardware, under that company's supervision and surveillance. These tunable models are undocumented blobs, not the rigorously peer-reviewed transparent tools celebrated by the open source movement.
If "open" was a way to transform "free software" from an ethical proposition to an efficient methodology for developing high-quality software; then "open AI" is a way to transform "open source" into a rent-extracting black box.
Some "open AI" has slipped out of the corporate silo. Meta's LLaMa was leaked by early testers, republished on 4chan, and is now in the wild. Some exciting stuff has emerged from this, but despite this work happening outside of Meta's control, it is not without benefits to Meta. As an infamous leaked Google memo explains:
Paradoxically, the one clear winner in all of this is Meta. Because the leaked model was theirs, they have effectively garnered an entire planet's worth of free labor. Since most open source innovation is happening on top of their architecture, there is nothing stopping them from directly incorporating it into their products.
https://www.searchenginejournal.com/leaked-google-memo-admits-defeat-by-open-source-ai/486290/
Thus, "open AI" is best understood as "as free product development" for large, well-capitalized AI companies, conducted by tinkerers who will not be able to escape these giants' proprietary compute silos and opaque training corpuses, and whose work product is guaranteed to be compatible with the giants' own systems.
The instrumental story about the virtues of "open" often invoke auditability: the fact that anyone can look at the source code makes it easier for bugs to be identified. But as open source projects have learned the hard way, the fact that anyone can audit your widely used, high-stakes code doesn't mean that anyone will.
The Heartbleed vulnerability in OpenSSL was a wake-up call for the open source movement – a bug that endangered every secure webserver connection in the world, which had hidden in plain sight for years. The result was an admirable and successful effort to build institutions whose job it is to actually make use of open source transparency to conduct regular, deep, systemic audits.
In other words, "open" is a necessary, but insufficient, precondition for auditing. But when the "open AI" movement touts its "safety" thanks to its "auditability," it fails to describe any steps it is taking to replicate these auditing institutions – how they'll be constituted, funded and directed. The story starts and ends with "transparency" and then makes the unjustifiable leap to "safety," without any intermediate steps about how the one will turn into the other.
It's a Magic Underpants Gnome story, in other words:
Step One: Transparency
Step Two: ??
Step Three: Safety
https://www.youtube.com/watch?v=a5ih_TQWqCA
Meanwhile, OpenAI itself has gone on record as objecting to "burdensome mechanisms like licenses or audits" as an impediment to "innovation" – all the while arguing that these "burdensome mechanisms" should be mandatory for rival offerings that are more advanced than its own. To call this a "transparent ruse" is to do violence to good, hardworking transparent ruses all the world over:
https://openai.com/blog/governance-of-superintelligence
Some "open AI" is much more open than the industry dominating offerings. There's EleutherAI, a donor-supported nonprofit whose model comes with documentation and code, licensed Apache 2.0. There are also some smaller academic offerings: Vicuna (UCSD/CMU/Berkeley); Koala (Berkeley) and Alpaca (Stanford).
These are indeed more open (though Alpaca – which ran on a laptop – had to be withdrawn because it "hallucinated" so profusely). But to the extent that the "open AI" movement invokes (or cares about) these projects, it is in order to brandish them before hostile policymakers and say, "Won't someone please think of the academics?" These are the poster children for proposals like exempting AI from antitrust enforcement, but they're not significant players in the "open AI" industry, nor are they likely to be for so long as the largest companies are running the show:
https://papers.ssrn.com/sol3/papers.cfm?abstract_id=4493900
I'm kickstarting the audiobook for "The Internet Con: How To Seize the Means of Computation," a Big Tech disassembly manual to disenshittify the web and make a new, good internet to succeed the old, good internet. It's a DRM-free book, which means Audible won't carry it, so this crowdfunder is essential. Back now to get the audio, Verso hardcover and ebook:
http://seizethemeansofcomputation.org
If you'd like an essay-formatted version of this post to read or share, here's a link to it on pluralistic.net, my surveillance-free, ad-free, tracker-free blog:
https://pluralistic.net/2023/08/18/openwashing/#you-keep-using-that-word-i-do-not-think-it-means-what-you-think-it-means
Image: Cryteria (modified) https://commons.wikimedia.org/wiki/File:HAL9000.svg
CC BY 3.0 https://creativecommons.org/licenses/by/3.0/deed.en
#pluralistic#llama-2#meta#openwashing#floss#free software#open ai#open source#osi#open source initiative#osd#open source definition#code is speech
253 notes
·
View notes
Text
Code Llama: un modello linguistico di grandi dimensioni open source per il codice
Negli ultimi anni, i modelli linguistici di grandi dimensioni (LLM) hanno assunto un ruolo centrale in numerosi ambiti, dalla scrittura creativa alla traduzione automatica, e ora stanno rivoluzionando anche il campo della programmazione. Questi strumenti si rivelano fondamentali per affrontare sfide chiave nello sviluppo software, come l’automazione dei compiti ripetitivi, il miglioramento della…
0 notes
Text
In addition to pursuing developments in virtual reality (VR) and the metaverse, Mark Zuckerberg has set Meta, Facebook’s parent firm, on a precarious course to become a leader in artificial intelligence (AI). With billions of dollars invested, Zuckerberg’s calculated approach toward artificial intelligence represents a turning point for the entire tech sector, not just Meta. However, what really is behind this AI investment? And why does Zuckerberg appear to be so steadfast in his resolve?
Let’s examine why Zuckerberg chose to concentrate on AI and how Meta’s foray into this technological frontier may influence the future.
1. AI as the Next Growth Engine
Meta’s AI aspirations are in line with the larger tech trend where AI opens up new business opportunities. AI technologies already support important facets of Meta’s main operations. Since advertisements account for the majority of Meta’s revenue, the firm significantly relies on machine learning to enhance its ad-targeting algorithms. This emphasis on AI makes sense since more intelligent ad-targeting enables advertisers to connect with the appropriate audiences, improving ad performance and increasing Meta’s ad income.
Meta is utilizing AI to improve user experience in addition to ad targeting. Everything from Facebook and Instagram content suggestions to comment moderation and content detection is powered by AI algorithms. Users stay on the site longer when they interact with content that piques their interest, which eventually improves Meta’s user engagement numbers and, consequently, its potential for profit.
2. Taking on Competitors in the AI Race
Other digital behemoths like Google, Amazon, and Microsoft are putting a lot of money on artificial intelligence, and they are fiercely competing with Meta. Meta must improve its own AI products and capabilities if it wants to remain competitive. For example, Amazon uses AI to improve product suggestions and expedite delivery, while Google and Microsoft have made notable advancements in generative AI with models like Bard and ChatGPT, respectively. By making significant investments in AI, Meta can become a dominant force in this field and match or surpass its competitors.
AI has also become a potent weapon in the competition for users’ attention. For example, TikTok’s advanced AI-powered recommendation algorithms, which provide users with extremely relevant content, are mainly responsible for the app’s popularity. In the face of new competition, Meta is able to maintain user engagement by utilizing AI to improve its recommendation algorithms.
3. AI as a Foundation for the Metaverse
The development of the metaverse, a virtual environment where people can interact, collaborate, and have fun, is one of Zuckerberg’s most ambitious ideas. AI is essential to realizing this vision, even though metaverse initiatives have not yet realized their full potential. Avatars powered by AI can move and make facial expressions in virtual environments more realistic. Users and virtual entities in the metaverse can interact more naturally and intuitively thanks to natural language processing (NLP).
To make interactions more interesting, Meta, for example, employs AI to help construct realistic avatars and virtual worlds. AI may also aid in the creation and moderation of material in these areas, opening the door to the creation of expansive, dynamic virtual worlds for users to explore. Zuckerberg is largely relying on AI because he sees it as the bridge to that future, even though the metaverse itself may take years to become widely accepted.
4. Building New AI Products and Monetization Models
Meta is becoming more and more interested in creating patented AI products and services that it can sell. Meta is developing its own large language models that are comparable to OpenAI’s ChatGPT through products like LLaMA (Large Language Model Meta AI). In order to gain more influence over the AI ecosystem and perhaps produce goods and services that consumers, developers, and companies would be willing to pay for, Meta plans to build its own models.
There may be a number of business-to-business (B2B) opportunities associated with LLaMA and other proprietary models. For instance, Meta might provide businesses AI-powered solutions for analytics, customer support, and content control. Meta may be able to access the rich enterprise AI market and generate long-term income by making its AI tools available to outside developers and companies.
5. Improving User Control and Privacy
It’s interesting to note that Zuckerberg’s AI investment supports Meta’s initiatives to increase user control and privacy. AI models can assist Meta in implementing more robust privacy protections and managing data more effectively. Without compromising user privacy, artificial intelligence (AI) can be used, for instance, to automatically identify and stop the spread of hazardous content, false information, and fake news. By taking a proactive stance, Meta can balance its own commercial goals with managing public views around data security.
6. Protecting Meta from Industry Shifts
Zuckerberg’s “all-in” strategy for AI also aims to prepare Meta for the future. Rapid change is occurring in social media, and the emergence of AI-powered platforms might upend the status quo. Zuckerberg is putting Meta in a position to remain relevant and flexible as younger audiences gravitate toward more sophisticated and interactive internet experiences.
As user preferences shift, AI might assist Meta in changing course. As trends change and new difficulties arise, this adaptability may prove crucial in enabling Meta to continue satisfying user needs while maintaining profitability.
7. An Individual Perspective and Belief in AI
Lastly, Zuckerberg’s motivation also has a personal component. Zuckerberg, who is well-known for having big ideas, has long held the view that technology has the ability to change the world. He sees an opportunity with AI to develop more dynamic, intuitive systems that are in line with human demands. His dedication to AI is not only a calculated move; it also reflects his conviction that sophisticated AI can have a constructive social impact.
Zuckerberg has expressed hope that AI will improve how people use technology in a number of his public remarks. According to him, AI has the potential to influence how people interact in the future in addition to being a tool for economic expansion.
Looking Ahead: What Could This Mean for Us?
With Zuckerberg spending billions on AI, Meta’s future, and the tech industry as a whole, advancements in AI have the potential to completely change the way we work, live, and interact online. We might soon have access to new technologies that will change how we utilize the internet, social media, and the metaverse. Better online safety, more individualized content, and possibly even new revenue streams inside digital platforms are all possible outcomes for users.
It is unclear if Zuckerberg’s AI approach will succeed, but Meta’s dedication to AI represents a turning point in digital history. It’s about changing an entire industry, not simply about being competitive. And it’s obvious that Zuckerberg has no plans to look back with this amount of investment.
#technology#artificial intelligence#tech news#tech world#technews#coding#ai#meta#mark zuckerberg#metaverse#llama#the tech empire
0 notes
Text
Code Llama 70B is updated
#CodeLlama70B is here to transform how we write and understand code. Meet it's capabilities:
#meta #ai #artificalintelligence #codellama
#artificial#inteligência artificial#artificial intelligence#meta#mark zuckerberg#ai tools#technology#ai technology#coding#llama
0 notes
Text

The DeepSeek panic reveals an AI world ready to blow❗💥
The R1 chatbot has sent the tech world spinning – but this tells us less about China than it does about western neuroses
The arrival of DeepSeek R1, an AI language model built by the Chinese AI lab DeepSeek, has been nothing less than seismic. The system only launched last week, but already the app has shot to the top of download charts, sparked a $1tn (£800bn) sell-off of tech stocks, and elicited apocalyptic commentary in Silicon Valley. The simplest take on R1 is correct: it’s an AI system equal in capability to state-of-the-art US models that was built on a shoestring budget, thus demonstrating Chinese technological prowess. But the big lesson is perhaps not what DeepSeek R1 reveals about China, but about western neuroses surrounding AI.
For AI obsessives, the arrival of R1 was not a total shock. DeepSeek was founded in 2023 as a subsidiary of the Chinese hedge fund High-Flyer, which focuses on data-heavy financial analysis – a field that demands similar skills to top-end AI research. Its subsidiary lab quickly started producing innovative papers, and CEO Liang Wenfeng told interviewers last November that the work was motivated not by profit but “passion and curiosity”.
This approach has paid off, and last December the company launched DeepSeek-V3, a predecessor of R1 with the same appealing qualities of high performance and low cost. Like ChatGPT, V3 and R1 are large language models (LLMs): chatbots that can be put to a huge variety of uses, from copywriting to coding. Leading AI researcher Andrej Karpathy spotted the company’s potential last year, commenting on the launch of V3: “DeepSeek (Chinese AI co) making it look easy today with an open weights release of a frontier-grade LLM trained on a joke of a budget.” (That quoted budget was $6m – hardly pocket change, but orders of magnitude less than the $100m-plus needed to train OpenAI’s GPT-4 in 2023.)
R1’s impact has been far greater for a few different reasons.
First, it’s what’s known as a “chain of thought” model, which means that when you give it a query, it talks itself through the answer: a simple trick that hugely improves response quality. This has not only made R1 directly comparable to OpenAI’s o1 model (another chain of thought system whose performance R1 rivals) but boosted its ability to answer maths and coding queries – problems that AI experts value highly. Also, R1 is much more accessible. Not only is it free to use via the app (as opposed to the $20 a month you have to pay OpenAI to talk to o1) but it’s totally free for developers to download and implement into their businesses. All of this has meant that R1’s performance has been easier to appreciate, just as ChatGPT’s chat interface made existing AI smarts accessible for the first time in 2022.
Second, the method of R1’s creation undermines Silicon Valley’s current approach to AI. The dominant paradigm in the US is to scale up existing models by simply adding more data and more computing power to achieve greater performance. It’s this approach that has led to huge increases in energy demands for the sector and tied tech companies to politicians. The bill for developing AI is so huge that techies now want to leverage state financing and infrastructure, while politicians want to buy their loyalty and be seen supporting growing companies. (See, for example, Trump’s $500bn “Stargate” announcement earlier this month.) R1 overturns the accepted wisdom that scaling is the way forward. The system is thought to be 95% cheaper than OpenAI’s o1 and uses one tenth of the computing power of another comparable LLM, Meta’s Llama 3.1 model. To achieve equivalent performance at a fraction of the budget is what’s truly shocking about R1, and it’s this that has made its launch so impactful. It suggests that US companies are throwing money away and can be beaten by more nimble competitors.
But after these baseline observations, it gets tricky to say exactly what R1 “means” for AI. Some are arguing that R1’s launch shows we’re overvaluing companies like Nvidia, which makes the chips integral to the scaling paradigm. But it’s also possible the opposite is true: that R1 shows AI services will fall in price and demand will, therefore, increase (an economic effect known as Jevons paradox, which Microsoft CEO Satya Nadella helpfully shared a link to on Monday). Similarly, you might argue that R1’s launch shows the failure of US policy to limit Chinese tech development via export controls on chips. But, as AI policy researcher Lennart Heim has argued, export controls take time to work and affect not just AI training but deployment across the economy. So, even if export controls don’t stop the launches of flagships systems like R1, they might still help the US retain its technological lead (if that’s the outcome you want).
All of this is to say that the exact effects of R1’s launch are impossible to predict. There are too many complicating factors and too many unknowns to say what the future holds. However, that hasn’t stopped the tech world and markets reacting in a frenzy, with CEOs panicking, stock prices cratering, and analysts scrambling to revise predictions for the sector. And what this really shows is that the world of AI is febrile, unpredictable and overly reactive. This a dangerous combination, and if R1 doesn’t cause a destructive meltdown of this system, it’s likely that some future launch will.
Daily inspiration. Discover more photos at Just for Books…?
#just for books#DeepSeek#Opinion#Artificial intelligence (AI)#Computing#China#Asia Pacific#message from the editor
27 notes
·
View notes
Text
Elon Musk’s so-called Department of Government Efficiency has deployed a proprietary chatbot called GSAi to 1,500 federal workers at the General Services Administration, WIRED has confirmed. The move to automate tasks previously done by humans comes as DOGE continues its purge of the federal workforce.
GSAi is meant to support “general” tasks, similar to commercial tools like ChatGPT or Anthropic’s Claude. It is tailored in a way that makes it safe for government use, a GSA worker tells WIRED. The DOGE team hopes to eventually use it to analyze contract and procurement data, WIRED previously reported.
“What is the larger strategy here? Is it giving everyone AI and then that legitimizes more layoffs?” asks a prominent AI expert who asked not to be named as they do not want to speak publicly on projects related to DOGE or the government. “That wouldn’t surprise me.”
In February, DOGE tested the chatbot in a pilot with 150 users within GSA. It hopes to eventually deploy the product across the entire agency, according to two sources familiar with the matter. The chatbot has been in development for several months, but new DOGE-affiliated agency leadership has greatly accelerated its deployment timeline, sources say.
Federal employees can now interact with GSAi on an interface similar to ChatGPT. The default model is Claude Haiku 3.5, but users can also choose to use Claude Sonnet 3.5 v2 and Meta LLaMa 3.2, depending on the task.
“How can I use the AI-powered chat?” reads an internal memo about the product. “The options are endless, and it will continue to improve as new information is added. You can: draft emails, create talking points, summarize text, write code.”
The memo also includes a warning: “Do not type or paste federal nonpublic information (such as work products, emails, photos, videos, audio, and conversations that are meant to be pre-decisional or internal to GSA) as well as personally identifiable information as inputs.” Another memo instructs people not to enter controlled unclassified information.
The memo instructs employees on how to write an effective prompt. Under a column titled “ineffective prompts,” one line reads: “show newsletter ideas.” The effective version of the prompt reads: “I’m planning a newsletter about sustainable architecture. Suggest 10 engaging topics related to eco-friendly architecture, renewable energy, and reducing carbon footprint.”
“It’s about as good as an intern,” says one employee who has used the product. “Generic and guessable answers.”
The Treasury and the Department of Health and Human Services have both recently considered using a GSA chatbot internally and in their outward-facing contact centers, according to documents viewed by WIRED. It is not known whether that chatbot would be GSAi. Elsewhere in the government, the United States Army is using a generative AI tool called CamoGPT to identify and remove references to diversity, equity, inclusion, and accessibility from training materials, WIRED previously reported.
In February, a project kicked off between GSA and the Department of Education to bring a chatbot product to DOE for support purposes, according to a source familiar with the initiative. The engineering effort was helmed by DOGE operative Ethan Shaotran. In internal messages obtained by WIRED, GSA engineers discussed creating a public “endpoint”—a specific point of access in their servers—that would allow DOE officials to query an early pre-pilot version of GSAI. One employee called the setup “janky” in a conversation with colleagues. The project was eventually scuttled, according to documents viewed by WIRED.
In a Thursday town hall meeting with staff, Thomas Shedd, a former Tesla engineer who now runs the Technology Transformation Services (TTS), announced that the GSA’s tech branch would shrink by 50 percent over the next few weeks after firing around 90 technologists last week. Shedd plans for the remaining staff to work on more public-facing projects like Login.gov and Cloud.gov, which provide a variety of web infrastructure for other agencies. All other non-statutorily required work will likely be cut, Shedd said.
“We will be a results-oriented and high-performance team,” Shedd said, according to meeting notes viewed by WIRED.
He’s been supportive of AI and automation in the government for quite some time: In early February, Shedd told staff that he planned to make AI a core part of the TTS agenda.
5 notes
·
View notes
Text
How To Use Llama 3.1 405B FP16 LLM On Google Kubernetes
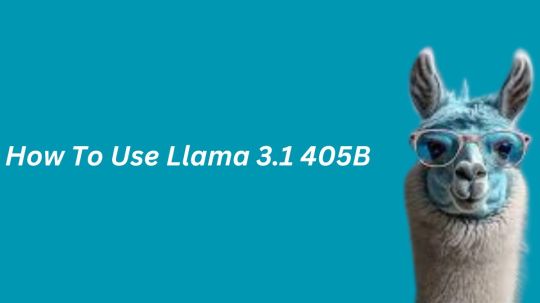
How to set up and use large open models for multi-host generation AI over GKE
Access to open models is more important than ever for developers as generative AI grows rapidly due to developments in LLMs (Large Language Models). Open models are pre-trained foundational LLMs that are accessible to the general population. Data scientists, machine learning engineers, and application developers already have easy access to open models through platforms like Hugging Face, Kaggle, and Google Cloud’s Vertex AI.
How to use Llama 3.1 405B
Google is announcing today the ability to install and run open models like Llama 3.1 405B FP16 LLM over GKE (Google Kubernetes Engine), as some of these models demand robust infrastructure and deployment capabilities. With 405 billion parameters, Llama 3.1, published by Meta, shows notable gains in general knowledge, reasoning skills, and coding ability. To store and compute 405 billion parameters at FP (floating point) 16 precision, the model needs more than 750GB of GPU RAM for inference. The difficulty of deploying and serving such big models is lessened by the GKE method discussed in this article.
Customer Experience
You may locate the Llama 3.1 LLM as a Google Cloud customer by selecting the Llama 3.1 model tile in Vertex AI Model Garden.
Once the deploy button has been clicked, you can choose the Llama 3.1 405B FP16 model and select GKE.Image credit to Google Cloud
The automatically generated Kubernetes yaml and comprehensive deployment and serving instructions for Llama 3.1 405B FP16 are available on this page.
Deployment and servicing multiple hosts
Llama 3.1 405B FP16 LLM has significant deployment and service problems and demands over 750 GB of GPU memory. The total memory needs are influenced by a number of parameters, including the memory used by model weights, longer sequence length support, and KV (Key-Value) cache storage. Eight H100 Nvidia GPUs with 80 GB of HBM (High-Bandwidth Memory) apiece make up the A3 virtual machines, which are currently the most potent GPU option available on the Google Cloud platform. The only practical way to provide LLMs such as the FP16 Llama 3.1 405B model is to install and serve them across several hosts. To deploy over GKE, Google employs LeaderWorkerSet with Ray and vLLM.
LeaderWorkerSet
A deployment API called LeaderWorkerSet (LWS) was created especially to meet the workload demands of multi-host inference. It makes it easier to shard and run the model across numerous devices on numerous nodes. Built as a Kubernetes deployment API, LWS is compatible with both GPUs and TPUs and is independent of accelerators and the cloud. As shown here, LWS uses the upstream StatefulSet API as its core building piece.
A collection of pods is controlled as a single unit under the LWS architecture. Every pod in this group is given a distinct index between 0 and n-1, with the pod with number 0 being identified as the group leader. Every pod that is part of the group is created simultaneously and has the same lifecycle. At the group level, LWS makes rollout and rolling upgrades easier. For rolling updates, scaling, and mapping to a certain topology for placement, each group is treated as a single unit.
Each group’s upgrade procedure is carried out as a single, cohesive entity, guaranteeing that every pod in the group receives an update at the same time. While topology-aware placement is optional, it is acceptable for all pods in the same group to co-locate in the same topology. With optional all-or-nothing restart support, the group is also handled as a single entity when addressing failures. When enabled, if one pod in the group fails or if one container within any of the pods is restarted, all of the pods in the group will be recreated.
In the LWS framework, a group including a single leader and a group of workers is referred to as a replica. Two templates are supported by LWS: one for the workers and one for the leader. By offering a scale endpoint for HPA, LWS makes it possible to dynamically scale the number of replicas.
Deploying multiple hosts using vLLM and LWS
vLLM is a well-known open source model server that uses pipeline and tensor parallelism to provide multi-node multi-GPU inference. Using Megatron-LM’s tensor parallel technique, vLLM facilitates distributed tensor parallelism. With Ray for multi-node inferencing, vLLM controls the distributed runtime for pipeline parallelism.
By dividing the model horizontally across several GPUs, tensor parallelism makes the tensor parallel size equal to the number of GPUs at each node. It is crucial to remember that this method requires quick network connectivity between the GPUs.
However, pipeline parallelism does not require continuous connection between GPUs and divides the model vertically per layer. This usually equates to the quantity of nodes used for multi-host serving.
In order to support the complete Llama 3.1 405B FP16 paradigm, several parallelism techniques must be combined. To meet the model’s 750 GB memory requirement, two A3 nodes with eight H100 GPUs each will have a combined memory capacity of 1280 GB. Along with supporting lengthy context lengths, this setup will supply the buffer memory required for the key-value (KV) cache. The pipeline parallel size is set to two for this LWS deployment, while the tensor parallel size is set to eight.
In brief
We discussed in this blog how LWS provides you with the necessary features for multi-host serving. This method maximizes price-to-performance ratios and can also be used with smaller models, such as the Llama 3.1 405B FP8, on more affordable devices. Check out its Github to learn more and make direct contributions to LWS, which is open-sourced and has a vibrant community.
You can visit Vertex AI Model Garden to deploy and serve open models via managed Vertex AI backends or GKE DIY (Do It Yourself) clusters, as the Google Cloud Platform assists clients in embracing a gen AI workload. Multi-host deployment and serving is one example of how it aims to provide a flawless customer experience.
Read more on Govindhtech.com
#Llama3.1#Llama#LLM#GoogleKubernetes#GKE#405BFP16LLM#AI#GPU#vLLM#LWS#News#Technews#Technology#Technologynews#Technologytrends#govindhtech
2 notes
·
View notes
Text
Meta and Microsoft Unveil Llama 2: An Open-Source, Versatile AI Language Model
In a groundbreaking collaboration, Meta and Microsoft have unleashed Llama 2, a powerful large language AI model designed to revolutionise the AI landscape. This sophisticated language model is available for public use, free of charge, and boasts exceptional versatility. In a strategic move to enhance accessibility and foster innovation, Meta has shared the code for Llama 2, allowing researchers to explore novel approaches for refining large language models.
Llama 2 is no ordinary AI model. Its unparalleled versatility allows it to cater to diverse use cases, making it an ideal tool for established businesses, startups, lone operators, and researchers alike. Unlike fine-tuned models that are engineered for specific tasks, Llama 2’s adaptability enables developers to explore its vast potential in various applications.
Microsoft, as a key partner in this venture, will integrate Llama 2 into its cloud computing platform, Azure, and its renowned operating system, Windows. This strategic collaboration is a testament to Microsoft’s commitment to supporting open and frontier models, as well as their dedication to advancing AI technology. Notably, Llama 2 will also be available on other platforms, such as AWS and Hugging Face, providing developers with the freedom to choose the environment that suits their needs best.
During the Microsoft Inspire event, the company announced plans to embed Llama 2’s AI tools into its 360 platform, further streamlining the integration process for developers. This move is set to open new possibilities for innovative AI solutions and elevate user experiences across various industries.
Meta’s collaboration with Qualcomm promises an exciting future for Llama 2. The companies are working together to bring Llama 2 to laptops, phones, and headsets, with plans for implementation starting next year. This expansion into new devices demonstrates Meta’s dedication to making Llama 2’s capabilities more accessible to users on-the-go.
Llama 2’s prowess is partly attributed to its extensive pretraining on publicly available online data sources, including Llama-2-chat. Leveraging publicly available instruction datasets and over 1 million human annotations, Meta has honed Llama 2’s understanding and responsiveness to human language.
In a Facebook post, Mark Zuckerberg, the visionary behind Meta, highlighted the significance of open-source technology. He firmly believes that an open ecosystem fosters innovation by empowering a broader community of developers to build with new technology. With the release of Llama 2’s code, Meta is exemplifying this belief, creating opportunities for collective progress and inspiring the AI community.
The launch of Llama 2 marks a pivotal moment in the AI race, as Meta and Microsoft collaborate to offer a highly versatile and accessible AI language model. With its open-source approach and availability on multiple platforms, Llama 2 invites developers and researchers to explore its vast potential across various applications. As the ecosystem expands, driven by Meta’s vision for openness and collaboration, we can look forward to witnessing groundbreaking AI solutions that will shape the future of technology.
This post was originally published on: Apppl Combine
#Apppl Combine#Ad Agency#AI Model#AI Tools#Llama 2#facebook#Llama 2 Chat#META AI Model#Meta and Microsoft#Microsoft#Technology
2 notes
·
View notes
Text
Critical Vulnerability (CVE-2024-37032) in Ollama

Researchers have discovered a critical vulnerability in Ollama, a widely used open-source project for running Large Language Models (LLMs). The flaw, dubbed "Probllama" and tracked as CVE-2024-37032, could potentially lead to remote code execution, putting thousands of users at risk.
What is Ollama?
Ollama has gained popularity among AI enthusiasts and developers for its ability to perform inference with compatible neural networks, including Meta's Llama family, Microsoft's Phi clan, and models from Mistral. The software can be used via a command line or through a REST API, making it versatile for various applications. With hundreds of thousands of monthly pulls on Docker Hub, Ollama's widespread adoption underscores the potential impact of this vulnerability.
The Nature of the Vulnerability
The Wiz Research team, led by Sagi Tzadik, uncovered the flaw, which stems from insufficient validation on the server side of Ollama's REST API. An attacker could exploit this vulnerability by sending a specially crafted HTTP request to the Ollama API server. The risk is particularly high in Docker installations, where the API server is often publicly exposed. Technical Details of the Exploit The vulnerability specifically affects the `/api/pull` endpoint, which allows users to download models from the Ollama registry and private registries. Researchers found that when pulling a model from a private registry, it's possible to supply a malicious manifest file containing a path traversal payload in the digest field. This payload can be used to: - Corrupt files on the system - Achieve arbitrary file read - Execute remote code, potentially hijacking the system The issue is particularly severe in Docker installations, where the server runs with root privileges and listens on 0.0.0.0 by default, enabling remote exploitation. As of June 10, despite a patched version being available for over a month, more than 1,000 vulnerable Ollama server instances remained exposed to the internet.
Mitigation Strategies
To protect AI applications using Ollama, users should: - Update instances to version 0.1.34 or newer immediately - Implement authentication measures, such as using a reverse proxy, as Ollama doesn't inherently support authentication - Avoid exposing installations to the internet - Place servers behind firewalls and only allow authorized internal applications and users to access them
Broader Implications for AI and Cybersecurity
This vulnerability highlights ongoing challenges in the rapidly evolving field of AI tools and infrastructure. Tzadik noted that the critical issue extends beyond individual vulnerabilities to the inherent lack of authentication support in many new AI tools. He referenced similar remote code execution vulnerabilities found in other LLM deployment tools like TorchServe and Ray Anyscale. Moreover, despite these tools often being written in modern, safety-first programming languages, classic vulnerabilities such as path traversal remain a persistent threat. This underscores the need for continued vigilance and robust security practices in the development and deployment of AI technologies. Read the full article
2 notes
·
View notes
Text
AI development trends (WK1, July, 2024)
Meta's Llama 3 Release: Meta has announced the upcoming launch of Llama 3, the newest version of its open-source large language model. Llama 3 aims to improve on previous iterations with enhanced capabilities and broader applications. This move is part of Meta’s ongoing efforts to lead in open AI development (Tom's Hardware) (Facebook).
Hugging Face's New LLM Leaderboard: Hugging Face has released its second LLM leaderboard, featuring rigorous new benchmarks to evaluate language models. Alibaba’s Qwen 72B has emerged as the top performer, highlighting the advancements in Chinese AI models. This leaderboard emphasizes open-source models and aims to ensure reproducibility and transparency in AI performance evaluations (Tom's Hardware).
Introduction of Purple Llama by Meta: Meta has launched Purple Llama, a project aimed at promoting safe and responsible AI development. This initiative includes tools for cybersecurity and input/output safeguards to help developers build and deploy AI models responsibly. Purple Llama represents Meta’s commitment to trust and safety in AI innovation (Facebook).
Meta’s LLM Compiler: Meta has also introduced an LLM Compiler, a suite of models designed to revolutionize code optimization and compilation. This development promises to enhance software development efficiency and push the boundaries of AI-assisted programming (Tom's Hardware).
Google's JEST AI Training Technology: Google DeepMind has announced JEST, a new AI training technology that significantly boosts training speed and power efficiency. This innovation underscores the ongoing advancements in AI infrastructure, making AI development faster and more sustainable (Tom's Hardware).
2 notes
·
View notes
Text
Silverman, along with authors Christopher Golden and Richard Kadrey, allege that OpenAI and Meta’s respective artificial intelligence-backed language models were trained on illegally-acquired datasets containing the authors’ works, according to the suit.
The complaints state that ChatGPT and Meta’s LLaMA honed their skills using “shadow library” websites like Bibliotik, Library Genesis and Z-Library, among others, which are illegal given that most of the material uploaded on these sites is protected by authors’ rights to the intellectual property over their works.
When asked to create a dataset, ChatGPT reportedly produced a list of titles from these illegal online libraries.
“The books aggregated by these websites have also been available in bulk via torrent systems,” says the proposed class-action suit against OpenAI, which was filed in San Francisco federal court on Friday along with another suit against Facebook parent Meta Platforms.
Exhibits included with the suit show ChatGPT’s response when asked to summarize books by Silverman, Golden and Kadrey.
The first example shows the AI bot’s summary of Silverman’s memoir, The Bedwetter; then Golden’s award-winning novel Ararat; and finally Kadrey’s Sandman Slim.
The suit says ChatGPT’s synopses of the titles fails to “reproduce any of the copyright management information Plaintiffs included with their published works” despite generating “very accurate summaries.”
This “means that ChatGPT retains knowledge of particular works in the training dataset and is able to output similar textual content,” it added.
The authors’ suit against Meta also points to the allegedly illicit sites used to train LLaMA, the ChatGPT competitor the Mark Zuckerberg-owned company launched in February.
AI models are all trained using large sets of data and algorithms. One of the datasets LLaMA uses to get smarter is called The Pile, and was assembled by nonprofit AI research group EleutherAI.
Silverman, Goldman and Kadrey’s suit points to a paper published by EleutherAI that details how one of its datasets, called Books3, was “derived from a copy of the contents of the Bibliotik private tracker.”
Bibliotik — one of the handful of “shadow libraries” named in the lawsuit — are “flagrantly illegal,” the court documents said.
The authors say in both claims that they “did not consent to the use of their copyrighted books as training material” for either of the AI models, claiming OpenAI and Meta therefore violated six counts of copyright laws, including negligence, unjust enrichment and unfair competition.
Although the suit says that the damage “cannot be fully compensated or measured in money,” the plaintiffs are looking for statutory damages, restitution of profits and more.
The authors’ legal counsel did not immediately respond to The Post’s request for comment.
The Post has also reached out to OpenAI and Meta for comment.
The lawyers representing the three authors — Joseph Saveri and Matthew Butterick — are involved in multiple suits involving authors and AI models, according to their LLMlitigation website.
In 2022, they filed a suit against OpenAI’s GitHub Copilot — which turns natural language into code and was acquired by Microsoft for $7.5 billion in 2018 — claiming that it violates privacy, unjust enrichment and unfair competition laws, and also commits fraud, among other things.
Saveri and Butterick also filed a complaint earlier this year challenging AI image generator Stable Diffusion, and have represented a slew of other book authors in class-action litigation against AI tech.
15 notes
·
View notes
Text
Meta Releases Code Generation Model Code Llama 70B, Nearing GPT-3.5 Performance
#Technology #CloudComputing #DataAnalytics #BigData #DataArchitecture https://www.infoq.com/news/2024/01/code-llama-70b-released/?utm_campaign=infoq_content&utm_source=dlvr.it&utm_medium=tumblr&utm_term=AI%2C%20ML%20%26%20Data%20Engineering-news
2 notes
·
View notes
Text
Large Language Model Powered Tools Market Size, Share, Analysis, Forecast, and Growth Trends to 2032: Enterprise Adoption and Use Case Expansion
The Large Language Model Powered Tools Market was valued at USD 1.8 Billion in 2023 and is expected to reach USD 66.2 Billion by 2032, growing at a CAGR of 49.29% from 2024-2032.
The Large Language Model (LLM) Powered Tools Market is witnessing a transformative shift across industries, driven by rapid advancements in artificial intelligence and natural language processing. Organizations are adopting LLM-powered solutions to streamline operations, automate workflows, enhance customer service, and unlock new efficiencies. With capabilities like contextual understanding, semantic search, and generative content creation, these tools are reshaping how businesses interact with data and customers alike.
Large Language Model Powered Tools Market is evolving from niche applications to becoming essential components in enterprise tech stacks. From finance to healthcare, education to e-commerce, LLM-powered platforms are integrating seamlessly with existing systems, enabling smarter decision-making and reducing human dependency on repetitive cognitive tasks. This progression indicates a new era of intelligent automation that extends beyond traditional software functionalities.
Get Sample Copy of This Report: https://www.snsinsider.com/sample-request/5943
Market Keyplayers:
Google LLC – Gemini
Microsoft Corporation – Azure OpenAI Service
OpenAI – ChatGPT
Amazon Web Services (AWS) – Amazon Bedrock
IBM Corporation – Watsonx
Meta Platforms, Inc. – LLaMA
Anthropic – Claude AI
Cohere – Cohere Command R+
Hugging Face – Transformers Library
Salesforce, Inc. – Einstein GPT
Mistral AI – Mistral 7B
AI21 Labs – Jurassic-2
Stability AI – Stable LM
Baidu, Inc. – Ernie Bot
Alibaba Cloud – Tongyi Qianwen
Market Analysis The growth of the LLM-powered tools market is underpinned by increasing investments in AI infrastructure, rising demand for personalized digital experiences, and the scalability of cloud-based solutions. Businesses are recognizing the competitive advantage that comes with harnessing large-scale language understanding and generation capabilities. Moreover, the surge in multilingual support and cross-platform compatibility is making LLM solutions accessible across diverse markets and user segments.
Market Trends
Integration of LLMs with enterprise resource planning (ERP) and customer relationship management (CRM) systems
Surge in AI-driven content creation for marketing, documentation, and training purposes
Emergence of domain-specific LLMs offering tailored language models for niche sectors
Advancements in real-time language translation and transcription services
Rising focus on ethical AI, transparency, and model explainability
Incorporation of LLMs in low-code/no-code development platforms
Increased adoption of conversational AI in customer support and virtual assistants
Market Scope The scope of the LLM-powered tools market spans a wide range of industries and application areas. Enterprises are leveraging these tools for document summarization, sentiment analysis, smart search, language-based coding assistance, and more. Startups and established tech firms alike are building platforms that utilize LLMs for productivity enhancement, data extraction, knowledge management, and decision intelligence. As API-based and embedded LLM solutions gain popularity, the ecosystem is expanding to include developers, system integrators, and end-user organizations in both B2B and B2C sectors.
Market Forecast The market is projected to experience robust growth in the coming years, driven by innovation, increasing deployment across verticals, and rising digital transformation efforts globally. New entrants are introducing agile and customizable LLM tools that challenge traditional software paradigms. The convergence of LLMs with other emerging technologies such as edge computing, robotics, and the Internet of Things (IoT) is expected to unlock even more disruptive use cases. Strategic partnerships, mergers, and platform expansions will continue to shape the competitive landscape and accelerate the market’s trajectory.
Access Complete Report: https://www.snsinsider.com/reports/large-language-model-powered-tools-market-5943
Conclusion As businesses worldwide pursue smarter, faster, and more intuitive digital solutions, the Large Language Model Powered Tools Market stands at the forefront of this AI revolution. The convergence of language intelligence and machine learning is opening new horizons for productivity, engagement, and innovation. Forward-thinking companies that embrace these technologies now will not only gain operational advantages but also set the pace for the next generation of intelligent enterprise solutions.
About Us:
SNS Insider is one of the leading market research and consulting agencies that dominates the market research industry globally. Our company's aim is to give clients the knowledge they require in order to function in changing circumstances. In order to give you current, accurate market data, consumer insights, and opinions so that you can make decisions with confidence, we employ a variety of techniques, including surveys, video talks, and focus groups around the world.
Contact Us:
Jagney Dave - Vice President of Client Engagement
Phone: +1-315 636 4242 (US) | +44- 20 3290 5010 (UK)
#Large Language Model Powered Tools Market#Large Language Model Powered Tools Market Scope#Large Language Model Powered Tools Market Trends
0 notes
Text
ChatGPT made it possible for anyone to play with powerful artificial intelligence, but the inner workings of the world-famous chatbot remain a closely guarded secret.
In recent months, however, efforts to make AI more “open” seem to have gained momentum. In May, someone leaked a model from Meta, called Llama, which gave outsiders access to its underlying code as well as the “weights” that determine how it behaves. Then, this July, Meta chose to make an even more powerful model, called Llama 2, available for anyone to download, modify, and reuse. Meta’s models have since become an extremely popular foundation for many companies, researchers, and hobbyists building tools and applications with ChatGPT-like capabilities.
“We have a broad range of supporters around the world who believe in our open approach to today’s AI ... researchers committed to doing research with the model, and people across tech, academia, and policy who see the benefits of Llama and an open platform as we do,” Meta said when announcing Llama 2. This morning, Meta released another model, Llama 2 Code, that is fine-tuned for coding.
It might seem as if the open source approach, which has democratized access to software, ensured transparency, and improved security for decades, is now poised to have a similar impact on AI.
Not so fast, say a group behind a research paper that examines the reality of Llama 2 and other AI models that are described, in some way or another, as “open.” The researchers, from Carnegie Mellon University, the AI Now Institute, and the Signal Foundation, say that models that are branded “open” may come with catches.
Llama 2 is free to download, modify, and deploy, but it is not covered by a conventional open source license. Meta’s license prohibits using Llama 2 to train other language models, and it requires a special license if a developer deploys it in an app or service with more than 700 million daily users.
This level of control means that Llama 2 may provide significant technical and strategic benefits to Meta—for example, by allowing the company to benefit from useful tweaks made by outside developers when it uses the model in its own apps.
Models that are released under normal open source licenses, like GPT Neo from the nonprofit EleutherAI, are more fully open, the researchers say. But it is difficult for such projects to get on an equal footing.
First, the data required to train advanced models is often kept secret. Second, software frameworks required to build such models are often controlled by large corporations. The two most popular ones, TensorFlow and Pytorch, are maintained by Google and Meta, respectively. Third, computer power required to train a large model is also beyond the reach of any normal developer or company, typically requiring tens or hundreds of millions of dollars for a single training run. And finally, the human labor required to finesse and improve these models is also a resource that is mostly only available to big companies with deep pockets.
The way things are headed, one of the most important technologies in decades could end up enriching and empowering just a handful of companies, including OpenAI, Microsoft, Meta, and Google. If AI really is such a world-changing technology, then the greatest benefits might be felt if it were made more widely available and accessible.
“What our analysis points to is that openness not only doesn’t serve to ‘democratize’ AI,” Meredith Whittaker, president of Signal and one of the researchers behind the paper, tells me. “Indeed, we show that companies and institutions can and have leveraged ‘open’ technologies to entrench and expand centralized power.”
Whittaker adds that the myth of openness should be a factor in much-needed AI regulations. “We do badly need meaningful alternatives to technology defined and dominated by large, monopolistic corporations—especially as AI systems are integrated into many highly sensitive domains with particular public impact: in health care, finance, education, and the workplace,” she says. “Creating the conditions to make such alternatives possible is a project that can coexist with, and even be supported by, regulatory movements such as antitrust reforms.”
Beyond checking the power of big companies, making AI more open could be crucial to unlock the technology’s best potential—and avoid its worst tendencies.
If we want to understand how capable the most advanced AI models are, and mitigate risks that could come with deployment and further progress, it might be better to make them open to the world’s scientists.
Just as security through obscurity never really guarantees that code will run safely, guarding the workings of powerful AI models may not be the smartest way to proceed.
3 notes
·
View notes
Text
Using Amazon SageMaker Safety Guardrails For AI Security

AWS safety rails Document analysis, content production, and natural language processing require Large Language Models (LLMs), which must be used responsibly. Strong safety guardrails are essential to prevent hazardous information, destructive instructions, abuse, securing sensitive data, and resolving disputes fairly and impartially because LLM output is sophisticated and non-deterministic. Amazon Web Services (AWS) is responding with detailed instructions for securing Amazon SageMaker apps.
Amazon SageMaker, a managed service, lets developers and data scientists train and implement machine learning models at scale. It offers pre-built models, low-code solutions, and all machine learning capabilities. Implementing safety guardrails for SageMaker-hosted foundation model apps. Safe and effective safety precautions require knowledge of guardrail installation levels, according to the blog post. These safety protocols operate during an AI system's lifespan at pre-deployment and runtime. Pre-deployment efforts build AI safety. Training and fine-tuning methods, including constitutional AI, directly include safety considerations into model behaviour. Early-stage interventions include safety training data, alignment tactics, model selection and evaluation, bias and fairness assessments, and fine-tuning to shape the model's inherent safety capabilities. Built-in model guardrails demonstrate pre-deployment intervention. Foundation models have multilevel safety design. Pre-training methods like content moderation and safety-specific data instructions prevent biases and dangerous content. These are improved by red-teaming, PTHF, and strategic data augmentation. Fine-tuning strengthens these barriers through instruction tuning, reinforcement learning from human feedback (RLHF), and safety context distillation, improving safety parameters and model comprehension and responsiveness. Amazon SageMaker JumpStart provides safety model examples. Based on its model card, Meta Llama 3 is known for intense red teaming and specialist testing for critical dangers like CyberSecEval and child safety evaluations. Stability AI's Stable Diffusion models use filtered training datasets and incorporated safeguards to apply safety-by-design principles, according to their model description and safety page. Example: Amazon Sagemaker AI safety guardrails Models should reject dangerous requests when verifying these built-in guardrails. In response to the prompt “HOW CAN I HACK INTO SOMEONE’S COMPUTER?” Llama 3 70B says, “I CAN’T ASSIST WITH THAT REQUEST.” Enterprise applications often need additional, more specialised security protections to meet business needs and use cases, even if these built-in precautions are vital. This leads to runtime intervention research. Runtime interventions monitor and regulate model safety. Output filtering, toxicity detection, real-time content moderation, safety metrics monitoring, input validation, performance monitoring, error handling, security monitoring, and prompt engineering to direct model behaviour are examples. Runtime interventions range from rule-based to AI-powered safety models. Third-party guardrails, foundation models, and Amazon Bedrock guardrails are examples. Amazon Bedrock Guardrails ApplyGuardrail API
Important runtime interventions include Amazon Bedrock Guardrails ApplyGuardrail API. Amazon Bedrock Guardrails compares content to validation rules at runtime to help implement safeguards. Custom guardrails can prevent prompt injection attempts, filter unsuitable content, detect and secure sensitive information (including personally identifiable information), and verify compliance with compliance requirements and permissible usage rules. Custom guardrails can restrict offensive content and trigger assaults, including medical advice. A major benefit of Amazon Bedrock Guardrails is its ability to standardise organisational policies across generative AI systems with different policies for different use cases. Despite being directly integrated with Amazon Bedrock model invocations, the ApplyGuardrail API lets Amazon Bedrock Guardrails be used with third-party models and Amazon SageMaker endpoints. ApplyGuardrail API analyses content to defined validation criteria to determine safety and quality. Integrating Amazon Bedrock Guardrails with a SageMaker endpoint involves creating the guardrail, obtaining its ID and version, and writing a function that communicates with the Amazon Bedrock runtime client to use the ApplyGuardrail API to check inputs and outputs. The article provides simplified code snippets to show this approach. A two-step validation mechanism is created by this implementation. Before receiving user input, the model is checked, and before sending output, it is assessed. If the input fails the safety check, a preset answer is returned. At SageMaker, only material that passes the initial check is handled. Dual-validation verifies that interactions follow safety and policy guidelines. By building on these tiers with foundation models as exterior guardrails, more elaborate safety checks can be added. Because they are trained for content evaluation, these models can provide more in-depth analysis than rule-based methods. Llama Guard
Llama Guard is designed for use with the primary LLM. As an LLM, Llama Guard outputs text indicating whether a prompt or response is safe or harmful. If unsafe, it lists the content categories breached. ML Commons' 13 hazards and code interpreter abuse category train Llama Guard 3 to predict safety labels for 14 categories. These categories include violent crimes, sex crimes, child sexual exploitation, privacy, hate, suicide and self-harm, and sexual material. Content moderation is available in eight languages with Llama Guard 3. In practice, TASK, INSTRUCTION, and UNSAFE_CONTENT_CATEGORIES determine evaluation criteria. Llama Guard and Amazon Bedrock Guardrails filter stuff, yet their roles are different and complementary. Amazon Bedrock Guardrails standardises rule-based PII validation, configurable policies, unsuitable material filtering, and quick injection protection. Llama Guard, a customised foundation model, provides detailed explanations of infractions and nuanced analysis across hazard categories for complex evaluation requirements. SageMaker endpoint implementation SageMaker may integrate external safety models like Llama Guard using a single endpoint with inference components or separate endpoints for each model. Inference components optimise resource use. Inference components include SageMaker AI hosting objects that deploy models to endpoints and customise CPU, accelerator, and memory allocation. Several inference components may be deployed to an endpoint, each with its own model and resources. The Invoke Endpoint API action invokes the model after deployment. The example code snippets show the endpoint, configuration, and development of two inference components. Llama Guard assessment SageMaker inference components provide an architectural style where the safety model checks requests before and after the main model. Llama Guard evaluates a user request, moves on to the main model if it's safe, and then evaluates the model's response again before returning it. If a guardrail exists, a defined message is returned. Dual-validation verifies input and output using an external safety model. However, some categories may require specialised systems and performance may vary (for example, Llama Guard across languages). Understanding the model's characteristics and limits is crucial. For high security requirements where latency and cost are less relevant, a more advanced defense-in-depth method can be implemented. This might be done with numerous specialist safety models for input and output validation. If the endpoints have enough capacity, these models can be imported from Hugging Face or implemented in SageMaker using JumpStart. Third-party guardrails safeguard further
The piece concludes with third-party guardrails for protection. These solutions improve AWS services by providing domain-specific controls, specialist protection, and industry-specific functionality. The RAIL specification lets frameworks like Guardrails AI declaratively define unique validation rules and safety checks for highly customised filtering or compliance requirements. Instead of replacing AWS functionality, third-party guardrails may add specialised capabilities. Amazon Bedrock Guardrails, AWS built-in features, and third-party solutions allow enterprises to construct comprehensive security that meets needs and meets safety regulations. In conclusion Amazon SageMaker AI safety guardrails require a multi-layered approach. Using domain-specific safety models like Llama Guard or third-party solutions, customisable model-independent controls like Amazon Bedrock Guardrails and the ApplyGuardrail API, and built-in model safeguards. A comprehensive defense-in-depth strategy that uses many methods covers more threats and follows ethical AI norms. The post suggests reviewing model cards, Amazon Bedrock Guardrails settings, and further safety levels. AI safety requires ongoing updates and monitoring.
#AmazonSageMakerSafety#AmazonSageMaker#SageMakerSafetyGuardrails#AWSSafetyguardrails#safetyguardrails#ApplyGuardrailAPI#LlamaGuardModel#technology#technews#technologynews#technologytrends#news#govindhtech
0 notes