#Operations Research And Statistics Techniques
Explore tagged Tumblr posts
Text
Elon Musk has pledged that the work of his so-called Department of Government Efficiency, or DOGE, would be “maximally transparent.” DOGE’s website is proof of that, the Tesla and SpaceX CEO, and now White House adviser, has repeatedly said. There, the group maintains a list of slashed grants and budgets, a running tally of its work.
But in recent weeks, The New York Times reported that DOGE has not only posted major mistakes to the website—crediting DOGE, for example, with saving $8 billion when the contract canceled was for $8 million and had already paid out $2.5 million—but also worked to obfuscate those mistakes after the fact, deleting identifying details about DOGE’s cuts from the website, and later even from its code, that made them easy for the public to verify and track.
For road-safety researchers who have been following Musk for years, the modus operandi feels familiar. DOGE “put out some numbers, they didn’t smell good, they switched things around,” alleges Noah Goodall, an independent transportation researcher. “That screamed Tesla. You get the feeling they’re not really interested in the truth.”
For nearly a decade, Goodall and others have been tracking Tesla’s public releases on its Autopilot and Full Self-Driving features, advanced driver-assistance systems designed to make driving less stressful and more safe. Over the years, researchers claim, Tesla has released safety statistics without proper context; promoted numbers that are impossible for outside experts to verify; touted favorable safety statistics that were later proved misleading; and even changed already-released safety statistics retroactively. The numbers have been so inconsistent that Tesla Full Self-Driving fans have taken to crowdsourcing performance data themselves.
Instead of public data releases, “what we have is these little snippets that, when researchers look into them in context, seem really suspicious,” alleges Bryant Walker Smith, a law professor and engineer who studies autonomous vehicles at the University of South Carolina.
Government-Aided Whoopsie
Tesla’s first and most public number mix-up came in 2018, when it released its first Autopilot safety figures after the first known death of a driver using Autopilot. Immediately, researchers noted that while the numbers seemed to show that drivers using Autopilot were much less likely to crash than other Americans on the road, the figures lacked critical context.
At the time, Autopilot combined adaptive cruise control, which maintains a set distance between the Tesla and the vehicle in front of it, and steering assistance, which keeps the car centered between lane markings. But the comparison didn’t control for type of car (luxury vehicles, the only kind Tesla made at the time, are less likely to crash than others), the person driving the car (Tesla owners were more likely to be affluent and older, and thus less likely to crash), or the types of roads where Teslas were driving (Autopilot operated only on divided highways, but crashes are more likely to occur on rural roads, and especially connector and local ones).
The confusion didn’t stop there. In response to the fatal Autopilot crash, Tesla did hand over some safety numbers to the National Highway Traffic Safety Administration, the nation’s road safety regulator. Using those figures, the NHTSA published a report indicating that Autopilot led to a 40 percent reduction in crashes. Tesla promoted the favorable statistic, even citing it when, in 2018, another person died while using Autopilot.
But by spring of 2018, the NHTSA had copped to the number being off. The agency did not wholly evaluate the effectiveness of the technology in comparison to Teslas not using the feature—using, for example, air bag deployment as an inexact proxy for crash rates. (The airbags did not deploy in the 2018 Autopilot death.)
Because Tesla does not release Autopilot or Full Self-Driving safety data to independent, third-party researchers, it’s difficult to tell exactly how safe the features are. (Independent crash tests by the NHTSA and other auto regulators have found that Tesla cars are very safe, but these don’t evaluate driver assistance tech.) Researchers contrast this approach with the self-driving vehicle developer Waymo, which often publishes peer-reviewed papers on its technology’s performance.
Still, the unknown safety numbers did not prevent Musk from criticizing anyone who questioned Autopilot’s safety record. “It's really incredibly irresponsible of any journalists with integrity to write an article that would lead people to believe that autonomy is less safe,” he said in 2018, around the time the NHTSA figure publicly fell apart. “Because people might actually turn it off, and then die.”
Number Questions
More recently, Tesla has continued to shift its Autopilot safety figures, leading to further questions about its methods. Without explanation, the automaker stopped putting out quarterly Autopilot safety reports in the fall of 2022. Then, in January 2023, it revised all of its safety numbers.
Tesla said it had belatedly discovered that it had erroneously included in its crash numbers events where no airbags nor active restraints were deployed and that it had found that some events were counted more than once. Now, instead of dividing its crash rates into three categories, "Autopilot engaged,” “without Autopilot but with our active safety features,” and “without Autopilot and without our active safety features,” it would report just two: with and without Autopilot. It applied those new categories, retroactively, to its old safety numbers and said it would use them going forward.
That discrepancy allowed Goodall, the researcher, to peer more closely into the specifics of Tesla’s crash reporting. He noticed something in the data. He expected the “without Autopilot” number to just be an average of the two old “without Auptilot” categories. It wasn’t. Instead, the new figure looked much more like the old “without Autopilot and without our active safety features” number. That’s weird, he thought. It’s not easy—or, according to studies that also include other car makes, common—for drivers to turn off all their active safety features, which include lane departure and forward collision warnings and automatic emergency braking.
Goodall calculated that even if Tesla drivers were going through the burdensome and complicated steps of turning off their EV’s safety features, they’d need to drive way more miles than other Tesla drivers to create a sensible baseline. The upshot: Goodall wonders if Tesla is allegedly making its non-Autopilot crash rate look higher than it is—and so the Autopilot crash rate allegedly looks much better by comparison.
The discrepancy is still puzzling to the researcher, who published a peer-reviewed note on the topic last summer. Tesla “put out this data that looks questionable on first glance—and then you look at it, and it is questionable,” he claims. “Instead of taking it down and acknowledging it, they change the numbers to something that is even weirder and flawed in a more complicated way. I feel like I’m doing their homework at this point.” The researcher calls for more transparency. So far, Tesla has not put out more specific safety figures.
Tesla, which disbanded its public relations team in 2021, did not reply to WIRED’s questions about the study or its other public safety data.
Direct Reports
Tesla is not a total outlier in the auto industry when it comes to clamming up about the performance of its advanced technology. Automakers are not required to make public many of their safety numbers. But where tech developers are required to submit public accounting on their crashes, Tesla is still less transparent than most. One prominent national data submission requirement, first instituted by the NHTSA in 2021, requires makers of both advanced driver assistance and automated driving tech to submit public data about its crashes. Tesla redacts nearly every detail about its Autopilot-related crashes in its public submissions.
“The specifics of all 2,819 crash reports have been redacted from publicly available data at Tesla's request,” says Philip Koopman, an engineering professor at Carnegie Mellon University whose research includes self-driving-car safety. “No other company is so blatantly opaque about their crash data.”
The federal government likely has access to details on these crashes, but the public doesn’t. But even that is at risk. Late last year, Reuters reported that the crash-reporting requirement appeared to be a focus of the Trump transition team.
In many ways, Tesla—and perhaps DOGE—is distinctive. “Tesla also uniquely engages with the public and is such a cause célèbre that they don’t have to do their own marketing. I think that also entails some special responsibility. Lots of claims are made on behalf of Tesla,” says Walker Smith, the law professor. “I think it engages selectively and opportunistically and does not correct sufficiently.”
Proponents of DOGE, like those of Tesla, engage enthusiastically on Musk’s platform, X, applauded by Musk himself. The two entities have at least one other thing in common: ProPublica recently reported that there is a new employee at the US Department of Transportation—a former Tesla senior counsel.
22 notes
·
View notes
Text



New DESI results strengthen hints that dark energy may evolve
The Dark Energy Spectroscopic Instrument used millions of galaxies and quasars to build the largest 3D map of our universe to date. Combining the DESI data with other experiments shows signs that the impact of dark energy may be weakening over time
The fate of the universe hinges on the balance between matter and dark energy: the fundamental ingredient that drives its accelerating expansion. New results from the Dark Energy Spectroscopic Instrument (DESI) collaboration use the largest 3D map of our universe ever made to track dark energy’s influence over the past 11 billion years. Researchers see hints that dark energy, widely thought to be a “cosmological constant,” might be evolving over time in unexpected ways.
DESI is an international experiment with more than 900 researchers from over 70 institutions around the world and is managed by the U.S. Department of Energy’s Lawrence Berkeley National Laboratory (Berkeley Lab). The collaboration shared their findings today in multiple papers that will be posted on the online repository arXiv and in a presentation at the American Physical Society’s Global Physics Summit in Anaheim, California.
“What we are seeing is deeply intriguing,” said Alexie Leauthaud-Harnett, co-spokesperson for DESI and a professor at UC Santa Cruz. “It is exciting to think that we may be on the cusp of a major discovery about dark energy and the fundamental nature of our universe.”
Taken alone, DESI’s data are consistent with our standard model of the universe: Lambda CDM (where CDM is cold dark matter and Lambda represents the simplest case of dark energy, where it acts as a cosmological constant). However, when paired with other measurements, there are mounting indications that the impact of dark energy may be weakening over time and that other models may be a better fit. Those other measurements include the light leftover from the dawn of the universe (the cosmic microwave background or CMB), exploding stars (supernovae), and how light from distant galaxies is warped by gravity (weak lensing).
“We’re guided by Occam’s razor, and the simplest explanation for what we see is shifting,” said Will Percival, co-spokesperson for DESI and a professor at the University of Waterloo. “It’s looking more and more like we may need to modify our standard model of cosmology to make these different datasets make sense together — and evolving dark energy seems promising.”
So far, the preference for an evolving dark energy has not risen to “5 sigma,” the gold standard in physics that represents the threshold for a discovery. However, different combinations of DESI data with the CMB, weak lensing, and supernovae datasets range from 2.8 to 4.2 sigma. (A 3-sigma event has a 0.3% chance of being a statistical fluke, but many 3-sigma events in physics have faded away with more data.) The analysis used a technique to hide the results from the scientists until the end, mitigating any unconscious bias about the data.
“We're in the business of letting the universe tell us how it works, and maybe the universe is telling us it's more complicated than we thought it was,” said Andrei Cuceu, a postdoctoral researcher at Berkeley Lab and co-chair of DESI’s Lyman-alpha working group, which uses the distribution of intergalactic hydrogen gas to map the distant universe. “It's interesting and gives us more confidence to see that many different lines of evidence are pointing in the same direction.”
DESI is one of the most extensive surveys of the cosmos ever conducted. The state-of-the-art instrument, which capture light from 5,000 galaxies simultaneously, was constructed and is operated with funding from the DOE Office of Science. DESI is mounted on the U.S. National Science Foundation’s Nicholas U. Mayall 4-meter Telescope at Kitt Peak National Observatory (a program of NSF NOIRLab) in Arizona. The experiment is now in its fourth of five years surveying the sky, with plans to measure roughly 50 million galaxies and quasars (extremely distant yet bright objects with black holes at their cores) by the time the project ends.
The new analysis uses data from the first three years of observations and includes nearly 15 million of the best measured galaxies and quasars. It’s a major leap forward, improving the experiment’s precision with a dataset that is more than double what was used in DESI’s first analysis, which also hinted at an evolving dark energy.
“It’s not just that the data continue to show a preference for evolving dark energy, but that the evidence is stronger now than it was,” said Seshadri Nadathur, professor at the University of Portsmouth and co-chair of DESI’s Galaxy and Quasar Clustering working group. “We’ve also performed many additional tests compared to the first year, and they’re making us confident that the results aren't driven by some unknown effect in the data that we haven't accounted for.”
DESI tracks dark energy’s influence by studying how matter is spread across the universe. Events in the very early universe left subtle patterns in how matter is distributed, a feature called baryon acoustic oscillations (BAO). That BAO pattern acts as a standard ruler, with its size at different times directly affected by how the universe was expanding. Measuring the ruler at different distances shows researchers the strength of dark energy throughout history. DESI’s precision with this approach is the best in the world.
“For a couple of decades, we’ve had this standard model of cosmology that is really impressive,” said Willem Elbers, a postdoctoral researcher at Durham University and co-chair of DESI’s Cosmological Parameter Estimation working group, which works out the numbers that describe our universe. “As our data are getting more and more precise, we’re finding potential cracks in the model and realizing we may need something new to explain all the results together.”
The collaboration will soon begin work on additional analyses to extract even more information from the current dataset, and DESI will continue collecting data. Other experiments coming online over the next several years will also provide complementary datasets for future analyses.
“Our results are fertile ground for our theory colleagues as they look at new and existing models, and we’re excited to see what they come up with,” said Michael Levi, DESI director and a scientist at Berkeley Lab. "Whatever the nature of dark energy is, it will shape the future of our universe. It's pretty remarkable that we can look up at the sky with our telescopes and try to answer one of the biggest questions that humanity has ever asked.”
TOP IMAGE: DESI maps distant objects to study dark energy. The instrument is installed on the Mayall Telescope, shown here beneath star trails. Credit KPNO/NOIRLab/NSF/AURA/B. Tafreshi
CENTRE IMAGE: From its mountaintop location in Arizona, DESI maps the universe. Credit Marilyn Sargent/Berkeley Lab
LOWER IMAGE: DESI is a state-of-the-art instrument and can capture light from up to 5,000 celestial objects simultaneously. Credit Marilyn Sargent/Berkeley Lab
8 notes
·
View notes
Text
PSYCHO-PASS: Providence - According to Japanese Twitter
After a long wait (or maybe it just felt long to me), PSYCHO-PASS: Providence finally hits North American theaters this week (14 July 2023), before becoming more widely internationally available in early August.
Unfortunately, as I'm not currently in Japan, I've not yet seen it. Fortunately, I speak Japanese, so I've read pretty much everything I could find about what happens. If you're like me and can't wait to see it in cinemas/don't mind major spoilers, this post is for you.
What follows is a compilation of everything my sister and I know about PPP -- drawing from fan talk on Twitter, director and writer interviews and tweets, and other official promotional materials only available in Japanese -- without actually having seen it.
We also explain some of the major plot points and go into detail on the real-life works referenced in the film, so if you watched it but feel like you could still use some clarification (as many Japanese fans did), this post might be for you too.
Once again, this post is nothing but spoilers (to be taken with several grains of salt as there is a certain amount of guesswork involved), so read on at your own risk.
*Note: "SN" denotes tweets/quotes by director Shiotani Naoyoshi.
We open on a snowy, stormy night, January 2118 (2 months post-SS Case.3).
A team of armed mercenaries board a transport ship off the coast of Kanagawa, Japan and set about killing the Ministry of Foreign Affairs (MOFA) Suppressing Action Department (SAD) agents on board.
Among them is Kai Mikhaylov, a Russian agent with a large burn scar on the left half of his face.
Kai Mikhaylov (VA: Kase Yasuyuki): A member of the “Peacebreakers.” In order to obtain the Stronskaya Document, he launches an attack on the ship Milcia is on.
Leading the mercenaries is fellow mercenary Bokamoso Murray, who sports distinctive red dreadlocks.
Bokamoso Murray (VA: Shirokuma Hiroshi): A combatant affiliated with the “Peacebreakers.” He operates in tandem with Kai Mikhaylov; beginning with the assault on the Grootslang, he works to seize the Stronskaya Document.
For the record, the Grootslang (the ship’s name) is a mythical giant snake rumoured to dwell deep in a cave in the Richtersveld, South Africa. It’s said that anyone who encounters it will meet with misfortune. Well then.
Indoors on the same ship, we find Dr Milicia Stronskaya, who has been invited to Tokyo from Russia to participate in an important political conference.
Milcia Stronskaya (VA: Tsuda Shōko): A researcher and global authority on behavioural economics and statistics. She establishes the basic theory simulation referred to as the “Stronskaya Document.”
Realising the ship is under attack, she hurriedly sends a communication to someone, apologising under her breath as she does so.
She pulls out a gun just as a helmeted mercenary bursts into the room, and she shoots him dead. You can tell from how she handles it that she’s competent.
Kai charges in next, dodging her shots and pinning her down.
Leaning over her, Kai calls her “professor,” at which she startles. He then says to her, “There’s nowhere left to run.”
Kai shoots Dr Stronskaya, killing her.
Bokamoso shows up then and says to Kai, “You screwed up, huh, Kai,” and “We’re switching to Plan B.”
Meanwhile, Kogami Shinya, one of our two main protagonists, heads to her rescue.
Kōgami Shinya (VA: Seki Tomokazu): Special Investigator, Suppressing Action Department, Overseas Coordination Bureau, Ministry of Foreign Affairs. Age 33. He was living a nomadic life abroad acting as a mercenary but was recruited by Frederica and returned to Japan; currently, he’s pursuing international incidents. He prides himself on his advanced combat techniques and honed physique.
Kogami makes an insane jump from an aircraft wearing a wingsuit. (I’ve seen him described alternately as Batman, Captain America, and a flying squirrel here lol)
SN: What colour suits a man who flies... Thinking about it.
Kogami proceeds to fight his way through the enemy soldiers with his typical efficiency.
Unfortunately, he arrives too late to save the professor, and the mercenaries have already absconded with her head. The reason for this is explained later.
On deck, Bokamoso and his team board their aircraft and make their escape.
Kogami, who has followed them out, takes aim at the aircraft but is tackled to the deck by a reanimated SAD agent. The man’s mouth doesn’t move but we hear a voice quoting what appears to be a passage from a religious text.
An explosion goes off and Kogami breaks free of his attacker and escapes the conflagration by jumping into the ocean.
Backlit by the flames and treading water, Kogami — vexed but composed as usual — reports on the situation via his device.
<<Opening Credits>>
OP: 「アレキシサイミアスペア」 (alexithymiaspare) ~ 凛として時雨 (Ling tosite sigure)
We cut to the opening credits, set to Ling tosite sigure’s “Alexthymiaspare.” The group also contributed to the soundtracks for PP1, PP2, and PP: The Movie (M1), so this is one of many ways in which the film “returns to its roots.”
The credits are then followed by a brief shot of the Sibyl System accompanied by the following text: 《"The Sibyl System," a vast surveillance network that assigns numeric values to and governs human beings’ mental states. Detectives who carry "Dominators" — guns that measure "crime coefficients" — pursue "latent criminals" before they commit crimes.》

The next morning, our other main protagonist, Tsunemori Akane, now Chief Inspector of the CID, attends a meeting of senior bureaucrats to discuss the proposed abolishment of the Ministry of Justice and the old system of law.
Tsunemori Akane (VA: Hanazawa Kana): Chief Inspector of the Ministry of Health and Welfare’s Public Safety Bureau. Age 25. She commands the Public Safety Bureau’s Criminal Investigation Department. She possesses an incontrovertible sense of justice and a stalwart mentality that makes it difficult for her Hue to cloud; she makes an appeal for maintaining the law under the Sibyl System.
The official name of the conference, which is being held at Nona Tower (i.e. the Ministry of Welfare’s HQ), is “Review Meeting on the Topic of the Overseas Expansion of Industry RE: the Sibyl System.”
Shindo Atsushi — father to PP3 protagonist Shindo Arata — is also in attendance, alongside officials from the Ministry of Health and Welfare, the Ministry of Public Management, Home Affairs, Posts and Telecommunications, the Ministry of Justice, and the Ministry of Foreign Affairs.
Shindō Atsushi (VA: Sugō Takayuki): Director-General of the Statistics Department, Minister’s Secretariat, Ministry of Health and Welfare. One of the elite who started his career as an Inspector [at the CID] and entered the MHW. He’s involved in the exportation of the Sibyl System, immigration policy, etc.
For the record, this is the same conference that Dr Stronskaya was originally scheduled to attend at Atsushi’s invitation.
Akane is the only woman and by far the youngest person present, but she doesn’t hesitate to say her piece. When it’s her turn to speak, she opens by saying, “‘Under the Sibyl System, the law is unnecessary.’ Is that truly the case?”
Akane is basically the sole voice of dissent, while Atsushi assumes a more neutral position.
During the meeting, Atsushi receives a text message, which he checks covertly before stashing his device in an inner pocket of his suit jacket.
Moments later, Akane receives a red alert on her device and excuses herself.
Atsushi calls a break in the meeting while Akane steps out to take a call from Mika.
Shimotsuki Mika (VA: Sakura Ayane): Inspector, Division 1, Criminal Investigation Department, Public Safety Bureau, Ministry of Health and Welfare. Age 21. The youngest Inspector ever inducted. At the time, she took a negative stance towards Akane’s way of thinking, but the two have a good working relationship now. She’s competitive but possesses both presence of mind and rational judgement.
Director Shiotani tweeted a quote by Rousseau that I saw someone identify as having been in reference to this scene. It’s not clear to me though whether a character quotes it aloud, or if Shiotani just meant it as an overarching theme:
SN: “Keep this truth ever before you—Ignorance never did any one any harm, error alone is fatal, and we do not lose our way through ignorance but through self-confidence.” by.Rousseau
from Rousseau’s Emile (On Education), Book III
SN: “Real knowledge is knowing the extent of one’s ignorance.”〈matcha emoji〉
from Confucius’ Analects II, Political Philosophy
Keep reading here.
#psycho pass#psycho pass: providence#PPP#akane tsunemori#tsunemori akane#kogami shinya#ginoza nobuchika#spoilers#foundintranslation#サイコパス#常守朱#狡噛信也#宜野座伸元#塩谷直義#Sugou Teppei#Kunizuka Yayoi#Karanomori Shion#Hinakawa Sho#Shimotsuki Mika#SaigaJouji#Hanashiro Frederica#Shindo Arata#Kei Mikhail Ignatov#PP1#PP2#PP3
82 notes
·
View notes
Text
Data Analysis: Turning Information into Insight
In nowadays’s digital age, statistics has come to be a vital asset for businesses, researchers, governments, and people alike. However, raw facts on its personal holds little value till it's far interpreted and understood. This is wherein records evaluation comes into play. Data analysis is the systematic manner of inspecting, cleansing, remodeling, and modeling facts with the objective of coming across beneficial information, drawing conclusions, and helping selection-making.
What Is Data Analysis In Research

What is Data Analysis?
At its middle, records analysis includes extracting meaningful insights from datasets. These datasets can variety from small and based spreadsheets to large and unstructured facts lakes. The primary aim is to make sense of data to reply questions, resolve issues, or become aware of traits and styles that are not without delay apparent.
Data evaluation is used in truely every enterprise—from healthcare and finance to marketing and education. It enables groups to make proof-based choices, improve operational efficiency, and advantage aggressive advantages.
Types of Data Analysis
There are several kinds of information evaluation, every serving a completely unique purpose:
1. Descriptive Analysis
Descriptive analysis answers the question: “What happened?” It summarizes raw facts into digestible codecs like averages, probabilities, or counts. For instance, a store might analyze last month’s sales to decide which merchandise achieved satisfactory.
2. Diagnostic Analysis
This form of evaluation explores the reasons behind beyond outcomes. It answers: “Why did it occur?” For example, if a agency sees a surprising drop in internet site visitors, diagnostic evaluation can assist pinpoint whether or not it changed into because of a technical problem, adjustments in search engine marketing rating, or competitor movements.
3. Predictive Analysis
Predictive analysis makes use of historical information to forecast destiny consequences. It solutions: “What is probable to occur?” This includes statistical models and system getting to know algorithms to pick out styles and expect destiny trends, such as customer churn or product demand.
4. Prescriptive Analysis
Prescriptive analysis provides recommendations primarily based on facts. It solutions: “What have to we do?” This is the maximum advanced type of analysis and often combines insights from predictive analysis with optimization and simulation techniques to manual selection-making.
The Data Analysis Process
The technique of information analysis commonly follows those steps:
1. Define the Objective
Before diving into statistics, it’s essential to without a doubt recognize the question or trouble at hand. A well-defined goal guides the entire analysis and ensures that efforts are aligned with the preferred outcome.
2. Collect Data
Data can come from numerous sources which includes databases, surveys, sensors, APIs, or social media. It’s important to make certain that the records is relevant, timely, and of sufficient high-quality.
3. Clean and Prepare Data
Raw information is regularly messy—it may comprise missing values, duplicates, inconsistencies, or mistakes. Data cleansing involves addressing these problems. Preparation may include formatting, normalization, or growing new variables.
Four. Analyze the Data
Tools like Excel, SQL, Python, R, or specialized software consisting of Tableau, Power BI, and SAS are typically used.
5. Interpret Results
Analysis isn't pretty much numbers; it’s about meaning. Interpreting effects involves drawing conclusions, explaining findings, and linking insights lower back to the authentic goal.
6. Communicate Findings
Insights have to be communicated effectively to stakeholders. Visualization tools including charts, graphs, dashboards, and reports play a vital position in telling the story behind the statistics.
7. Make Decisions and Take Action
The last aim of statistics analysis is to tell selections. Whether it’s optimizing a advertising marketing campaign, improving customer support, or refining a product, actionable insights flip data into real-global effects.
Tools and Technologies for Data Analysis
A big selection of gear is available for facts analysis, each suited to distinct tasks and talent levels:
Excel: Great for small datasets and short analysis. Offers capabilities, pivot tables, and charts.
Python: Powerful for complicated facts manipulation and modeling. Popular libraries consist of Pandas, NumPy, Matplotlib, and Scikit-learn.
R: A statistical programming language extensively used for statistical analysis and statistics visualization.
SQL: Essential for querying and handling information saved in relational databases.
Tableau & Power BI: User-friendly enterprise intelligence equipment that flip facts into interactive visualizations and dashboards.
Healthcare: Analyzing affected person statistics to enhance treatment plans, predict outbreaks, and control resources.
Finance: Detecting fraud, coping with threat, and guiding investment techniques.
Retail: Personalizing advertising campaigns, managing inventory, and optimizing pricing.
Sports: Enhancing performance through participant records and game analysis.
Public Policy: Informing choices on schooling, transportation, and financial improvement.
Challenges in Data Analysis
Data Quality: Incomplete, old, or incorrect information can lead to deceptive conclusions.
Data Privacy: Handling sensitive records requires strict adherence to privacy guidelines like GDPR.
Skill Gaps: There's a developing demand for skilled information analysts who can interpret complicated facts sets.
Integration: Combining facts from disparate resources may be technically hard.
Bias and Misinterpretation: Poorly designed analysis can introduce bias or lead to wrong assumptions.
The Future of Data Analysis
As facts keeps to grow exponentially, the sector of facts analysis is evolving rapidly. Emerging developments include:
Artificial Intelligence (AI) & Machine Learning: Automating evaluation and producing predictive fashions at scale.
Real-Time Analytics: Enabling decisions based totally on live data streams for faster reaction.
Data Democratization: Making records handy and understandable to everybody in an business enterprise
2 notes
·
View notes
Text
India’s Tech Sector to Create 1.2 Lakh AI Job Vacancies in Two Years
India’s technology sector is set to experience a hiring boom with job vacancies for artificial intelligence (AI) roles projected to reach 1.2 lakh over the next two years. As the demand for AI latest technology increases across industries, companies are rapidly adopting advanced tools to stay competitive. These new roles will span across tech services, Global Capability Centres (GCCs), pure-play AI and analytics firms, startups, and product companies.
Following a slowdown in tech hiring, the focus is shifting toward the development of AI. Market analysts estimate that Indian companies are moving beyond Proof of Concept (PoC) and deploying large-scale AI systems, generating high demand for roles such as AI researchers, product managers, and data application specialists. “We foresee about 120,000 to 150,000 AI-related job vacancies emerging as Indian IT services ramp up AI applications,” noted Gaurav Vasu, CEO of UnearthInsight.
India currently has 4 lakh AI professionals, but the gap between demand and supply is widening, with job requirements expected to reach 6 lakh soon. By 2026, experts predict the number of AI specialists required will hit 1 million, reflecting the deep integration of AI latest technology into industries like healthcare, e-commerce, and manufacturing.
The transition to AI-driven operations is also altering the nature of job vacancies. Unlike traditional software engineering roles, artificial intelligence positions focus on advanced algorithms, automation, and machine learning. Companies are recruiting experts in fields like deep learning, robotics, and natural language processing to meet the growing demand for innovative AI solutions. The development of AI has led to the rise of specialised roles such as Machine Learning Engineers, Data Scientists, and Prompt Engineers.
Krishna Vij, Vice President of TeamLease Digital, remarked that new AI roles are evolving across industries as AI latest technology becomes an essential tool for product development, operations, and consulting. “We expect close to 120,000 new job vacancies in AI across different sectors like finance, healthcare, and autonomous systems,” he said.
AI professionals also enjoy higher compensation compared to their traditional tech counterparts. Around 80% of AI-related job vacancies offer premium salaries, with packages 40%-80% higher due to the limited pool of trained talent. “The low availability of experienced AI professionals ensures that artificial intelligence roles will command attractive pay for the next 2-3 years,” noted Krishna Gautam, Business Head of Xpheno.
Candidates aiming for AI roles need to master key competencies. Proficiency in programming languages like Python, R, Java, or C++ is essential, along with knowledge of AI latest technology such as large language models (LLMs). Expertise in statistics, machine learning algorithms, and cloud computing platforms adds value to applicants. As companies adopt AI latest technology across domains, candidates with critical thinking and AI adaptability will stay ahead so it is important to learn and stay updated with AI informative blogs & news.
Although companies are prioritising experienced professionals for mid-to-senior roles, entry-level job vacancies are also rising, driven by the increased use of AI in enterprises. Bootcamps, certifications, and academic programs are helping freshers gain the skills required for artificial intelligence roles. As AI development progresses, entry-level roles are expected to expand in the near future. AI is reshaping the industries providing automation & the techniques to save time , to increase work efficiency.
India’s tech sector is entering a transformative phase, with a surge in job vacancies linked to AI latest technology adoption. The next two years will witness fierce competition for AI talent, reshaping hiring trends across industries and unlocking new growth opportunities in artificial intelligence. Both startups and established companies are racing to secure talent, fostering a dynamic landscape where artificial intelligence expertise will be help in innovation and growth. AI will help organizations and businesses to actively participate in new trends.
#aionlinemoney.com
2 notes
·
View notes
Text
How Do Market Research and Competitive Analysis? – Types with Examples
Products that do not satisfy customer needs and wants fail to perform well in market dynamics, affecting your sales revenue. However, market research and analytics help you estimate consumer behavior. Corporate leaders also create competitive strategies using customer insights discovered by market research consulting partners. So, this post will explain how to do market research and competitive analysis.
What is Market Research?
Market research involves interviews, surveys, social listening, and media coverage analytics to acquire valuable customer insights. Therefore, businesses employ market research consulting firms to improve their understanding of consumer preferences.
The obtained insights allow companies to revise their pricing strategies and marketing efforts to attract new customers and retain existing ones. Besides, such data-driven pricing, marketing, and innovation strategies are less vulnerable to human errors, a significant drawback of empirical business development methods.
Enterprises use market research to minimize product launch risks. A marketing analytics company also delivers transparent and flexible reports to research what promotional strategies drive the most engagement from target customer profiles.
What is Competitive Analytics?
Competitive analytics leverages statistical modeling and automation technologies to identify methods to help you overcome your competition and increase your market share. For example, marketing research and analytics firms can guide you in optimizing your internal operations to compete more aggressively.
Consider how inefficient allocation of resources affects all enterprises. If two companies target the same customer segment, the more efficient company will succeed. After all, corporate competitiveness improves when a business reduces the irresponsible use of company resources. Later, it can transfer the related financial benefits to the customers, i.e., rationalizing prices.
Simultaneously, you want to know how your competitors plan to increase their market position. However, they will not share such confidential intelligence on public platforms.
Therefore, market research consulting teams will develop machine learning (ML) models to process your competitors’ press releases. ML facilitates modern predictive analytics and helps companies forecast how competitors plan to grow their business.
How to Conduct Market Research and Competitive Analysis?
Goal determination is the first step in conducting market research or competitive analysis. If a business invests in market research consulting without clearly communicating its envisioned objectives, it will experience disappointment due to directionless competitive analysis or macroeconomic surveys.
Later, study the available technologies and how implementing them will affect the company financially. For example, standard marketing analytics tools benefit a regional company. Similarly, a global business firm will require scalable, automated analytics software to generate high-quality reports.
Finally, you want to specify a timeframe. Otherwise, monitoring the progress of your market research efforts will become daunting. Moreover, the risk of scheduling conflicts increases without time-bound activities. Financial planning also depends on the time factor for interest estimations associated with borrowed capital resources.
Organizations have distinct business objectives, risk dynamics, and data processing requirements. Therefore, study the following market research and competitive analysis techniques.
Part 1 – Types of Market Research Services
1| Primary Research
It is primary market research when a marketing analytics company interviews customers, suppliers, and employees. After all, the collected customer insights originate at the source, enhancing the quality of your competitive analytics operations in market research. You also get ownership rights to the resulting databases.
Such original research helps you create thought leadership content, establish authority, and acquire unique strategic foresight. Sometimes, primary research integrates into whitepapers, case studies, and investment relations (IR) disclosures, increasing the trust in the brand among stakeholders.
2| Secondary Research
Finding customer insights through social listening and media coverage analytics for secondary research primarily concentrates on publicly available intelligence gathered by somebody else. Also, the scope of market research consulting teams revolves around magazines, social media platforms, consumer discussion forums, and global news publications.
Secondary market research relies on already available intelligence resources. Therefore, most data in a secondary research project will have third-party owners. The hired marketing analytics company might use the editorial reproduction freedoms often governed by fair use or educational intent principles to help you in your marketing efforts.
Still, organizations must practice proper caution since different secondary data sources can be prone to manipulative content and misinterpreted perspectives on business-critical ideas. Assessing the authoritative qualities and historical reputation of each data source can become easier with the help of a market research consulting firm.
3| Manual Research
Small businesses and young social media accounts can evaluate their growth, revenue, and competitiveness using simple analytics for customer insights. Remember, they generate fewer data points, eliminating any necessity for extensive database processing.
Nevertheless, manual market research suffers from a more prominent risk of exposure to human errors. For example, psychological issues and physical limits often prevent your team members from developing holistic data models efficiently. So, manual research efforts are no longer relevant. Besides, enterprises have adopted advanced marketing analytics.
4| Automated Research
Machine learning allows for self-learning software applications, i.e., they can learn multiple tasks that usually require human intervention. Likewise, artificial intelligence (AI) enables automated marketing research and analytics through abilities similar to idea synthesis.
Market research consulting will offer data gathering, validation, and cleaning automation. You will have access to more extensive data throughout the day and night.
Corporations save a lot of time and human effort when ML models extract customer insights via analytics. Additionally, such technologies eliminate ambiguity in competitive analysis and market research by facilitating accelerated data validation.
5| Qualitative Research
Customers might complain about a product feature in their social media posts or consumer discussion forums. Some users will also give you meaningful feedback using highly descriptive texts. Additionally, you want to analyze product ratings and reviews if you operate an e-commerce business division.
However, software applications need more help understanding meaning and emotions when processing qualitative consumer responses. Qualitative marketing research implements natural language processing (NLP) algorithms for sentiment analytics. Therefore, categorizing unstructured data becomes seamless.
6| Quantitative Research
The customer rating system varies from website to website. Still, it contains numerical data manageable using straightforward mathematical programs. So, quantitative market research gathers more structured data.
Analyzing properly structured data does not require extensive computing resources. Businesses utilize quantitative research in financial modeling and total quality management (TQM) instead of sentiment analytics. They prioritize the quantitative methods for these two operations because the core reporting systems are well-structured and standardized.
Moreover, it does not make any business sense to use a lot of computing power when the marginal gains in performance contribute little to ultimate goals, like revenue enhancement and market share increment. Therefore, professional consulting firms specializing in market research technologies assist enterprises in deciding when to use quantitative or qualitative analytics for customer insights.
Part 2 – Types of Competitive Analytics
1| Internal Competitive Research and Analysis
Every established marketing analytics company treats an organization’s competitiveness using a systems approach. So, internal competitive analytics investigates how an enterprise manages its supply chain, professional networks, business units, and investor relations.
For example, a business might suffer above-average employee attrition during a specific financial year. It can ask a competitive analytics company to inspect how such problematic events in retaining talent affect its performance.
The consulting analysts will then reveal the impact on the company through statistical modeling. Later, the business can revise its talent acquisition processes, employment contracts, and workplace environment to counter the adverse effect of employee attention using the consultants’ insights.
2| External Competitive Analytics
A company’s performance relies on factors outside its direct control, and consulting firms research these external market forces. It is external competitive analytics with a broad scope of data gathering, validation, modeling, and reporting global customer insights.
Consider how currency fluctuations influence the financial planning done by import-export businesses. Likewise, natural disasters introduce systemic issues across transportation, communication, and healthcare infrastructure.
How can an organization become more resilient against the losses resulting from earthquakes, avalanches, tsunamis, landslides, or other catastrophes caused by malicious actors? Competitive analysis and market research can give you the data necessary to evaluate such business queries.
Most market research consulting teams consider the socioeconomic and political stability indicators for such inquiries. After all, enterprises of all scales must be attentive to external competitive risks.
3| Competitor Analytics
Competitor analysis has a narrower scope since it concentrates all the marketing research and analytics activities on your top business rivals. It is a subset of a more holistic competitive analysis. Therefore, it takes less time, consumes a few computing resources, and delivers reports fast.
You can utilize computer analytics for peer benchmarking in a target industry. This activity allows enterprises to compare their performance with how their business rivals perform in the same industry. However, computer analytics becomes more complex if a company serves multiple customer segments, leading to the application of advanced tools to acquire insights.
5| Descriptive and Diagnostic Analysis for Competitive Intelligence
Descriptive analytics explains a company’s past performance so that the leadership, management, marketing, and sales teams can learn how their strategies have contributed to business objectives.
Diagnostic analytics adds value to historical performance records by identifying methods to improve productivity, capital efficiency, and risk assessment. It helps companies solve the problems encountered in the preceding business quarters.
6| Predictive and Prescriptive Analytics
Predictive analytics utilizes machine learning to estimate how market forces, consumer preferences, regulatory policies, and competitor strategies will evolve. Corporations also use it to eliminate the gaps in market research and competitive analysis databases.
Prescriptive analytics offers practical solutions to combat business risks identified by predictive ML models. It is vital to preventing or mitigating the potential losses attributed to market volatility, the introduction of new laws, and macroeconomic events.
Conclusion
Leveraging analytics to identify customer insights is the most prominent advantage of marketing research. Besides, enterprises utilize primary research in authoritative content. Additionally, secondary market research finds valuable trends across social media platforms and review sites.
Qualitative research differs from quantitative analytics since the raw datasets vary in structure. Meanwhile, automated aggregation tools have replaced manual data collection procedures. If you want to do market research and competitive analysis, consider these developments before hiring a consultant.
A leader in market research consulting, SG Analytics supports enterprises in extracting customer insights by performing analytics on primary and secondary data sources. Contact us today if you want outcome-oriented technological assistance with automated aggregation capabilities.
2 notes
·
View notes
Text
MBA Specializations in Bangalore
Top MBA Specializations
An MBA degree offers a plethora of specializations, allowing students to tailor their education to their career aspirations and interests. In Bangalore, a hub of educational excellence, several MBA colleges offer a wide range of specializations to cater to the diverse needs of management professionals. Let’s explore some of the popular MBA specializations available in Bangalore, providing students with the opportunity to gain specialized knowledge and skills in their chosen field.
Colleges Offering MBA Specialization:
Indian Institute of Management Bangalore (IIMB)
Symbiosis Institute of Business Management (SIBM)
Xavier Institute of Management and Entrepreneurship (XIME)
IFIM Business School
Alliance School of Business, Alliance University
International Institute of Business Studies (IIBS)
1. Marketing Management
Marketing Management is one of the most sought-after MBA specializations in Bangalore, focusing on understanding consumer behavior, market trends, and strategic marketing techniques. Students pursuing this specialization learn how to develop effective marketing strategies, conduct market research, and launch successful marketing campaigns to promote products and services.
2. Finance
Finance is another popular MBA specialization in Bangalore, focusing on financial management, investment analysis, and risk assessment. Students pursuing this specialization learn how to analyze financial data, make informed investment decisions, and manage financial resources effectively to maximize profitability and shareholder value.
3. Human Resource Management (HRM)
Human Resource Management (HRM) specialization focuses on managing human capital, employee relations, and organizational development. Students pursuing this specialization learn how to recruit and retain talent, design employee training programs, and create a positive work culture conducive to employee engagement and productivity.
4. Operations Management
Operations Management specialization focuses on streamlining business operations, optimizing processes, and improving efficiency and productivity. Students pursuing this specialization learn how to manage supply chains, inventory, and logistics effectively to meet customer demands and enhance organizational performance.
5. Information Technology (IT) Management
Information Technology (IT) Management specialization focuses on leveraging technology to drive business innovation and transformation. Students pursuing this specialization learn how to align IT strategies with business goals, manage IT projects effectively, and leverage emerging technologies to gain a competitive edge in the digital era.
6. International Business
International Business focuses on understanding global markets, cross-border transactions, and international trade policies. Students learn to navigate cultural differences, manage international operations, and develop global business strategies for market expansion and organizational growth.
7. Entrepreneurship
Entrepreneurship specialization fosters innovation, creativity, and entrepreneurial mindset among students. Students learn to identify business opportunities, develop business plans, and launch successful ventures in dynamic and competitive business environments.
8. Business Analytics
Business Analytics focuses on leveraging data analysis and statistical techniques to drive informed business decisions. Students learn to analyze complex data sets, derive actionable insights, and make strategic recommendations to optimize business processes and enhance performance.
Conclusion
In conclusion, Bangalore offers a wide range of MBA specializations, catering to the diverse interests and career goals of management professionals. Whether it’s Marketing Management, Finance, Human Resource Management, Operations Management, or Information Technology Management, aspiring MBA students can choose from a plethora of options to gain specialized knowledge and skills in their chosen field. With top-notch faculty, state-of-the-art infrastructure, and strong industry connections, MBA colleges in Bangalore provide the perfect platform for students to embark on a successful career journey in the dynamic world of business.
#mba#mbacollege#business#businessanalysis#placement#management#marketing#finance#internationalbusiness#entrepreneurship#specializations#bangalore#education#educationcollege#bestcollege#businesscollege#college
4 notes
·
View notes
Text
Predictive vs Prescriptive vs Descriptive Analytics Explained
Business analytics leveraging data patterns for strategic moves comes in three key approaches – descriptive identifying “what has occurred", predictive forecasting “what could occur” and prescriptive recommending “what should occur” to optimize decisions. We decode the science behind each for aspiring analytics professionals.
Descriptive analytics convert volumes of historical data into insightful summaries around metrics revealing business health, customer trends, operational efficiencies etc. using direct analysis, aggregation and mining techniques producing current reports.
Predictive analytics forecast unknown future probabilities applying statistical, econometric and machine learning models over existing data to minimize uncertainties and capture emerging behaviors early for mitigation actions. Risk models simulate scenarios balancing upside/downside tradeoffs.
Prescriptive analytics take guidance one step further by dynamically recommending best decision options factoring in key performance indicators for business objective improvements after predicting multiple futures using bell curve simulations. Optimization algorithms deliver preferred actions.
While foundational data comprehension and wrangling abilities fuel all models – pursuing analytics specializations focused on statistical, computational or operational excellence boosts career-readiness filling different priorities global employers seek!
Posted By:
Aditi Borade, 4th year Barch,
Ls Raheja School of architecture
Disclaimer: The perspectives shared in this blog are not intended to be prescriptive. They should act merely as viewpoints to aid overseas aspirants with helpful guidance. Readers are encouraged to conduct their own research before availing the services of a consultant.
#analytics#types#predictive#prescriptive#descriptive#PrescriptiveAnalytics#StrategicMoves#AnalyticsProfessionals#DataScience#HistoricalData#Metrics#BusinessHealth#CustomerTrends#OperationalEfficiencies#StatisticalModels#EconometricModels#MachineLearningModels#EnvoyOverseas#EthicalCounselling#EnvoyInternationalStudents#EnvoyCounselling
4 notes
·
View notes
Text
Crafting a Data-Driven Destiny: Your Ultimate Guide to Becoming a Data Scientist
Embarking on the journey to become a data scientist is an exhilarating endeavor, blending education, skill development, and hands-on experience. In a landscape driven by data, the role of a data scientist has become pivotal across industries. This blog aims to provide a detailed step-by-step guide, offering insights into the educational, technical, and practical aspects that shape a successful career in data science. For individuals aspiring to master the art and science of data science, enrolling in the best data science institute becomes pivotal. This ensures a comprehensive learning experience, equipping learners with the skills and knowledge required to excel in the dynamic field of data science.
Here's a step-by-step guide to help you navigate this rewarding career path:
1. Acquire the Necessary Educational Background:
The foundation of a data scientist's journey often begins with a robust educational background. A strong grasp of mathematics, statistics, and computer science is paramount. Many individuals kickstart their path with a bachelor's degree in a relevant field, providing a solid footing for the challenges ahead.
2. Develop Programming Skills:
Programming is the language of data science, and proficiency in languages such as Python or R is essential. This section explores the importance of familiarizing oneself with tools like Jupyter Notebooks and version control systems like Git, which streamline the coding process and collaboration in a data science environment.
3. Gain Proficiency in Data Manipulation and Analysis:
Mastering the art of data manipulation and analysis is a cornerstone of data science. This segment delves into the significance of becoming adept with data manipulation libraries like Pandas and data visualization tools such as Matplotlib and Seaborn. These skills are crucial for interpreting and presenting data effectively.
4. Dive into Machine Learning and Statistics:
Understanding the intricacies of machine learning algorithms, statistical modeling, and data mining techniques is central to a data scientist's skill set. The blog explores platforms like Kaggle, which offer practical challenges, allowing aspiring data scientists to apply and refine their skills in real-world scenarios.
5. Acquire Database and Big Data Skills:
As data sets grow larger and more complex, proficiency in handling databases (SQL) and big data technologies like Hadoop and Spark becomes indispensable. This section outlines the importance of acquiring these skills for tackling the challenges posed by real-world data science tasks.
6. Cultivate Business Acumen:
Beyond technical expertise, a data scientist must cultivate a deep understanding of the business domain they operate in. This segment discusses the significance of aligning data insights with organizational goals, emphasizing the role of a data scientist as a strategic contributor to business success.
7. Stay Updated with Industry Trends:
In the rapidly evolving field of data science, staying abreast of industry trends is crucial. The blog underscores the importance of continuous learning through avenues such as reading research papers, following industry blogs, and active participation in relevant forums.
8. Build a Strong Portfolio:
A compelling portfolio is the tangible evidence of a data scientist's capabilities. This section explores the significance of showcasing practical abilities through a diverse range of projects. A robust portfolio not only reflects technical proficiency but also serves as a testament to problem-solving prowess.
9. Networking and Professional Development:
Connecting with professionals in the field is a valuable aspect of a data scientist's journey. Attendances at conferences, webinars, and meetups provide opportunities for networking and staying informed about the latest developments. This section also emphasizes the importance of continuous learning through online courses and workshops.
Embarking on a career as a data scientist requires dedication, continuous learning, and practical experience. With a commitment to excellence and industry relevance, ACTE Technologies offers a comprehensive data science course in Chennai, ensuring that learners not only grasp the fundamentals but also gain practical insights and hands-on experience. Embrace the possibilities, equip yourself with the right skills, and embark on a fulfilling data science career with ACTE Technologies.
4 notes
·
View notes
Text
Unveiling the Power of Market Research Analytics: A Strategic Imperative for Business Success
Introduction
In today's fast-paced and hyper-competitive business landscape, gaining a competitive edge requires more than just intuition and guesswork. Enter market research analytics – an essential approach that empowers businesses to make informed decisions, uncover hidden insights, and navigate the complex maze of consumer preferences and market trends. In this blog, we take a deep dive into the world of Market Research Analytics, exploring its significance, methodologies, and the transformative impact it can have on your business.
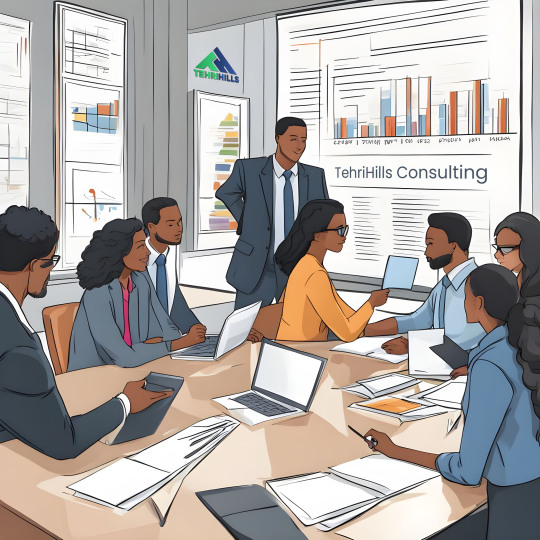
https://www.tehrihills.com/
The Significance of Market Research Analytics -
Market research analytics is the art and science of extracting actionable insights from raw data to drive strategic decision-making. It provides a structured approach to understanding consumer behavior, market dynamics, and industry trends.By leveraging data-driven insights, businesses can:
Enhance Customer Understanding: By analyzing consumer preferences, buying patterns, and sentiment, businesses can tailor their products and services to meet customer needs more effectively.
Competitor Analysis: Market research analytics enables companies to assess competitor strengths and weaknesses, identify gaps in the market, and formulate strategies to gain a competitive advantage.
Optimize Marketing Efforts: Precise data analysis allows businesses to target their marketing campaigns with laser-like precision, reducing costs and increasing conversion rates.
Product Innovation: Uncovers latent customer needs and pain points through data analysis, fuels the creation of innovative products that resonate with the target audience.
Methodologies in Market Research Analytics –
In the domain of Market Research Analytics, diverse methodologies play a pivotal role in facilitating informed and sound decision-making. These methodologies empower businesses with the tools to untangle complex market dynamics, cultivate a deeper understanding of consumer preferences and enable the formulation of impactful strategies.
Quantitative Analysis: This approach involves the use of numerical data to measure, quantify, and analyze various aspects of the market. Surveys, polls, and structured questionnaires are common tools used to gather data for quantitative analysis.
Qualitative Analysis: Qualitative research delves into the subjective aspects of consumer behavior, focusing on insights that are not easily quantifiable. Techniques such as focus groups, in-depth interviews, and content analysis provide valuable context and depth to numerical data.
Predictive Analytics: Using historical data and statistical algorithms, predictive analytics helps forecast future trends, customer behavior, and market shifts. This enables businesses to proactively adapt and strategize.
Text and Sentiment Analysis: With the proliferation of online reviews, social media, and user-generated content, extracting insights from text data has become crucial. Text and sentiment analysis tools decipher consumer sentiment, helping businesses gauge public opinion and adjust strategies accordingly.
Transformative Impact on Business-
Market research analytics has different impacts which transforms business into more successful entity. Brands can improve their bottom line and build stronger relationships with their customers by providing high quality products/services. Embracing market research analytics can usher in a myriad of benefits for businesses:
Informed Decision-Making: Accurate data-driven insights provide a solid foundation, reducing the element of risk and uncertainty in strategic decision-making.
Cost Efficiency: By focusing resources on targeted strategies and campaigns, businesses can optimize their marketing budgets and operational expenditures.
Agility and Adaptability: Real-time data analysis equips businesses to swiftly respond to changing market conditions, ensuring they remain relevant and adaptable.
Customer-Centric Approach: By understanding consumer preferences and pain points, businesses can align their offerings with customer needs, thereby fostering brand loyalty and customer satisfaction.
Innovation Catalyst: Market research analytics can uncover untapped opportunities, enabling businesses to innovate and stay ahead of the curve.
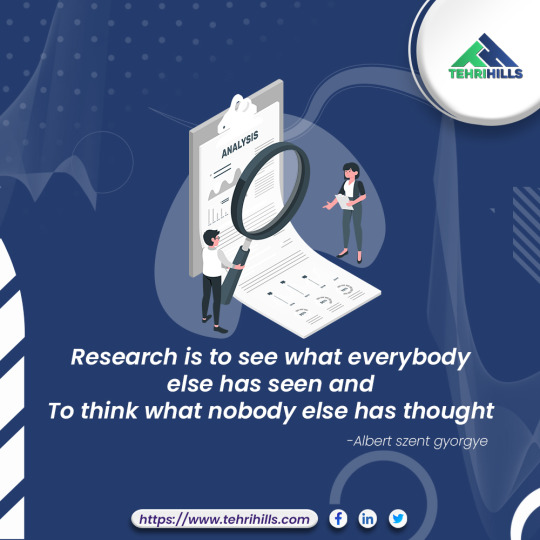
https://www.tehrihills.com/
Conclusion
In a business landscape driven by data and insights, market research analytics emerges as a strategic imperative for sustainable success. By deciphering the intricate web of consumer behavior, market trends, and competition dynamics, businesses can chart a course towards informed decision-making, innovation, and customer-centricity. Embracing market research analytics isn't just an option; it's a powerful tool that can unlock the doors to unparalleled growth and prosperity in today's dynamic marketplace.
#consulting#survey programming#market research#marketresearchreport#marketanalysis#tehrihills#tehrihillsconsulting
3 notes
·
View notes
Text

Astronomy’s dirty window to space
Researchers reconstruct detailed map of dust in the Milky Way
When we observe distant celestial objects, there is a possible catch: Is that star I am observing really as reddish as it appears? Or does the star merely look reddish, since its light has had to travel through a cloud of cosmic dust to reach our telescope? For accurate observations, astronomers need to know the amount of dust between them and their distant targets. Not only does dust make objects appear reddish (“reddening”), it also makes them appear fainter than they really are (“extinction”). It’s like we are looking out into space through a dirty window. Now, two astronomers have published a 3D map that documents the properties of dust all around us in unprecedented detail, helping us make sense of what we observe.
Behind this is the fact that, fortunately, when looking at stars, there is a way of reconstructing the effect of dust. Cosmic dust particles do not absorb and scatter light evenly across all wavelengths. Instead, they absorb light more strongly at shorter wavelengths (towards the blue end of the spectrum), and less strongly at longer wavelengths (towards the red end). The wavelength-dependence can be plotted as an “extinction curve,” and its shape provides information not only about the composition of the dust, but also about its local environment, such as the amount and properties of radiation in the various regions of interstellar space.
Retrieving dust information from 130 million spectra
This is the kind of information used by Xiangyu Zhang, a PhD student at the Max Planck Institute for Astronomy (MPIA), and Gregory Green, an independent research group leader (Sofia Kovalevskaja Group) at MPIA and Zhang’s PhD advisor, to construct the most detailed 3D map yet of the properties of dust in the Milky Way galaxy. Zhang and Green turned to data from ESA’s Gaia mission, which was a 10.5-year-effort to obtain extremely accurate measurements of positions, motions and additional properties for more than a billion stars in our Milky Way and in our nearest galactic neighbours, the Magellanic Clouds. The third data release (DR3) of the Gaia mission, published in June 2022, provides 220 million spectra, and a quality check told Zhang and Green that about 130 million of those would be suitable for their search for dust.
The Gaia spectra are low-resolution, that is, the way that they separate light into different wavelength regions is comparatively coarse. The two astronomers found a way around that limitation: For 1% of their chosen stars, there is high-resolution spectroscopy from the LAMOST survey operated by the National Astronomical Observatories of China. This provides reliable information about the basic properties of the stars in question, such as their surface temperatures, which determines what astronomers call a star’s “spectral type.”
Reconstructing a 3D map
Zhang and Green trained a neural network to generate model spectra based on a star’s properties and the properties of the intervening dust. They compared the results to 130 million suitable spectra from Gaia, and used statistical (“Bayesian”) techniques to deduce the properties of the dust between us and those 130 million stars.
The results allowed the astronomers to reconstruct the first detailed, three-dimensional map of the extinction curve of dust in the Milky Way. This map was made possible by Zhang and Green’s measurement of the extinction curve towards an unprecedented number of stars – 130 million, compared to previous works, which contained approximately 1 million measurements.
But dust is not just a nuisance for astronomers. It is important for star formation, which occurs in giant gas clouds shielded by their dust from the surrounding radiation. When stars form, they are surrounded by disks of gas and dust, which are the birthplaces of planets. The dust grains themselves are the building blocks for what will eventually become the solid bodies of planets like our Earth. In fact, within the interstellar medium of our galaxy, most of the elements heavier than hydrogen and helium are locked up in interstellar dust grains.
Unexpected properties of cosmic dust
The new results not only produce an accurate 3D map. They have also turned up a surprising property of interstellar dust clouds. Previously, it had been expected that the extinction curve should become flatter (less dependent on wavelength) for regions with a higher dust density. “Higher density,” of course, is in this case still very little: approximately ten billionth billionth grams of dust per cubic meter, equivalent to just 10 kg of dust in a sphere with Earth’s radius. In such regions, dust grains tend to grow in size, which changes the overall absorption properties.
Instead, the astronomers found that in areas of intermediate density, the extinction curve actually becomes steeper, with smaller wavelengths absorbed much more effectively than longer ones. Zhang and Green surmise that the steepening might be caused by the growth not of dust, but of a class of molecules called polycyclic aromatic hydrocarbons (PAHs), the most abundant hydrocarbons in the interstellar medium, which may even have played a role in the origin of life. They have already set out to test their hypothesis with future observations.
Background information
The results reported here have been published as Xiangyu Zhang and Gregory M. Green, “Three-dimensional maps of the interstellar dust extinction curve within the Milky Way galaxy,” in the journal Science. Both authors work at the Max Planck Institute for Astronomy.
IMAGE: Red indicates regions where extinction falls off more rapidly at long wavelengths (the red end of the spectrum), while blue indicates that extinction is less dependent on wavelength. Regions with insufficient data are shown in white. The gray contours enclose regions of high dust density. Credit X. Zhang/G. Green, MPIA
5 notes
·
View notes
Text
How to learn Data Science and AI from Scratch
1. Make a Strong Foundation in Mathematics and Statistics
In order to have a stable foundation in math and statistics, one must understand basic ideas, hone problem-solving techniques, and use these ideas in actual situations. Victory in a variety of domains, including data science, depends on it.
2. Learn Programming with Python
Python is a famous and flexible programming language that's frequently used for data analysis, web development, and different tasks. It is renowned for being comfortable to understand and operate, which makes it a superb option for novices. To learn Python, feel free to focus on a single topic, practice continually, work on actual projects, participate in society, and not hurry the learning process.
3. Master Data Analysis and Visualization Tools
Using a range of tools is vital for mastering data analysis and visualization. In addition to specialized platforms like Looker and Qlik, famous options include Tableau, Power BI, Excel, and Python. These technologies provide a variety of features, such as strong data manipulation abilities, interactive dashboards, and perceptive visualizations.
4. Understand Databases and SQL
A relational database, often known as a SQL database, is a set of highly structured tables with each column defining a particular information field and each row representing a data item. The structured query language (SQL) is used in relational databases to develop, save, update, and retrieve data.
5. Engage in Projects and Competitions
By boosting teamwork, developing abilities, and growing motivation, projects and contests can greatly accelerate both professional and personal progress. Research contests, for instance, can enable innovative and scientific thinking, whereas hackathons encourage teamwork and problem-solving.
6. Continuous Learning and Networking
Networking and ongoing education are vital for job progression and professional development. While networking focuses on establishing and maintaining connections with specialists in your sector, continuous learning entails the constant acquisition of new capabilities and information. Both improve job happiness, expand career opportunities, and enable both professional and personal development.
How to Learn AI from Scratch
1. Understand the Basics of AI
Making machines, mainly computers, do tasks that ordinarily need human intelligence, which is known as artificial intelligence. This covers tasks like design recognition, language comprehension, decision-making, and data-driven learning. Basically, artificial intelligence aims to replicate human cognitive abilities in machines.
2. Learn Machine Learning
The objective of the artificial intelligence area of machine learning is to make it possible for computers to understand data without explicit programming. It entails creating algorithms with the ability to recognize patterns, forecast outcomes, and slowly enhance their performance through experience.
3. Explore Deep Learning Frameworks
Neural network construction and performance require deep learning frameworks. They reduce the model-building and training method by delivering pre-built layers, processes, and APIs. PyTorch, Keras, and TensorFlow are famous frameworks.
4. Work on Real-World AI Projects
Explore different AI applications in industries, including healthcare, finance, education, and transportation, to work on real-world AI projects. Start by clearly identifying the problem, collecting and preparing data, choosing the right AI framework, creating and training a prototype, and then assessing and refining it for practical implementation.
5. Stay Updated and Network
Maintaining current networking technology and building a strong network needs a multifaceted strategy. By taking classes, engaging in online groups, and keeping up with industry information, you can stay informed. Building connections and relationships with somebody in your area is the importance of networking.
learning. Essentially, artificial intelligence seeks to replicate human cognitive capacities in machines.
To learn data science and AI from scratch, consider registering for an online course like "Artificial Intelligence with Data Science Course" offered by platforms like 1StepGrow. These courses offer a comprehensive curriculum that covers the fundamentals of data analysis, machine learning, deep learning, and artificial intelligence. Via hands-on projects and practical examples, you’ll gain practical skills in AI and data science, training you for real-world challenges. Whether you're interested in data science and artificial intelligence or pursuing a specialized AI and advanced data science course, these online programs deliver a structured pathway to mastering these in-demand fields.
0 notes
Text
Quantitative vs. Qualitative Research: Which is Right for Your Business?
In today’s data-driven world, businesses cannot afford to make decisions based on guesswork. Effective decision-making starts with robust market research. But when choosing a research approach, many business owners face a common dilemma: Quantitative vs. Qualitative Research—which is right for your business?
Understanding the differences, strengths, and suitable applications of each method is essential to crafting an accurate feasibility plan, validating new product ideas, and improving customer experience through techniques like mystery shopping.
What is Quantitative Research?
Quantitative research focuses on numerical data and statistical analysis. It’s ideal for measuring market sizes, tracking performance, or identifying trends. This method relies on large sample sizes and structured tools such as surveys, polls, and analytics platforms.
Key benefits of quantitative research:
Delivers measurable, reliable data
Enables forecasting based on past trends
Ideal for large-scale market research company operations
Forms the backbone of any solid feasibility study report
For example, if you want to determine how many potential customers are willing to pay for a new service, quantitative research can give you statistically valid results that can feed directly into your feasibility plan.
What is Qualitative Research?
In contrast, qualitative research dives into the “why” behind customer behaviors. It uses open-ended methods such as focus groups, in-depth interviews, and observations. This approach is more exploratory and helps uncover insights that numbers alone can't explain.
Key benefits of qualitative research:
Provides deep understanding of customer motivations
Identifies emotional and cultural drivers
Supports strategic decisions with nuanced insights
Often used in mystery shopping programs to explore customer service quality
A market research company might recommend qualitative research when you're launching a new brand and want to understand how your target audience emotionally connects with your product.
Which One Should You Choose?
Choosing between quantitative and qualitative research depends on your business objectives. Here’s a quick guide: Business Need Recommended Method Measuring customer satisfaction levels Quantitative Exploring new market opportunities Qualitative Testing product pricing or demand Quantitative Understanding brand perception Qualitative Creating a data-backed feasibility study report Quantitative Enhancing customer service via mystery shopping Both
In many cases, the best approach is a mix of both—this is called mixed-method research. For instance, a market research company may start with qualitative research to generate hypotheses and then use quantitative research to test them at scale.
Final Thoughts
Whether you're developing a new product, entering a new market, or validating an idea with a feasibility plan, the choice between quantitative and qualitative research is crucial. Each offers unique insights that can help drive your business forward.
Partnering with a professional market research company can help you strike the right balance and ensure your findings are actionable. With the right data, even tools like mystery shopping can evolve from simple evaluations to strategic assets.
0 notes
Text
How to Choose the Best Surgeon for Your Nephrectomy
When facing the prospect of a nephrectomy—a surgical procedure to remove all or part of a kidney—choosing the right surgeon is one of the most important decisions you’ll make. Whether it's due to kidney cancer, severe kidney damage, or complications from kidney stones, selecting a skilled and experienced surgeon can significantly impact the success of the procedure and your recovery.
If you're looking for the best nephrectomy doctor in Jaipur, here are key factors to help you make the right choice:

1. Check Qualifications and Experience
Look for a urologist or surgeon who is board-certified and has significant experience performing nephrectomies. Surgeons who regularly perform kidney surgeries are more familiar with potential complications and advanced techniques like laparoscopic or robotic-assisted nephrectomy. Ask about their specialization—some may focus solely on complex kidney procedures.
2. Assess Hospital Reputation and Technology
The hospital where the surgeon operates plays a vital role. Choose a facility with advanced surgical infrastructure, a strong nephrology department, and post-operative care units. Many of the top hospitals in Jaipur offer state-of-the-art technology for both nephrectomy and the best kidney stone treatment in Jaipur—an indicator of comprehensive kidney care.
3. Ask About Surgical Techniques
Nephrectomy can be done using different methods—open surgery, laparoscopic, or robotic-assisted. The best surgeons will evaluate your condition and recommend the safest and most effective technique. Less invasive options often result in quicker recovery, smaller incisions, and reduced hospital stays.
4. Review Patient Testimonials and Success Rates
Look for patient reviews and success stories online or through referrals. A good surgeon will have positive feedback, clear communication skills, and high success rates. Don't hesitate to ask for references or statistics about past nephrectomy procedures.
5. Evaluate Pre- and Post-Operative Support
Top surgeons work with a team that provides thorough preoperative preparation and excellent postoperative care. This includes managing pain, preventing infection, and ensuring kidney function monitoring. If you’re also dealing with stones, ask if the surgeon or hospital also provides the best kidney stone treatment in Jaipur for a more integrated approach.
6. Consider Location and Accessibility
Choosing a surgeon close to your home, especially in a medical hub like Jaipur, can make follow-ups and emergency visits easier. Jaipur is home to several highly rated kidney specialists and hospitals offering world-class care.
7. Get a Second Opinion
Before committing to surgery, it's wise to consult at least two nephrologists or urologists. A second opinion can provide clarity on whether nephrectomy is necessary and which approach is best suited to your condition.
Final Thoughts
Your health and peace of mind are paramount. Take the time to research and meet with potential surgeons. If you're searching for the best nephrectomy doctor in Jaipur, prioritize expertise, technology, hospital quality, and patient care.
Additionally, if you're managing kidney stones alongside other issues, it’s beneficial to consult a center known for offering the best kidney stone treatment in Jaipur to ensure complete and continuous care.
Also Read : Best Kidney Stone Specialist: How to Choose the Right Urologist
0 notes
Text
Exploring Dividend Yields in the London Stock Exchange Cannabis Sector
Highlights
Companies listed within the LSE Cannabis stocks category exhibit varied yield patterns.
Certain producers and licensors secure standings among peers.
Yield sustainability linked to revenue diversification and cost structures.
The cannabis sector on the London Stock Exchange has grown steadily as cultivation, processing and distribution firms gain regulatory approvals and commercial partnerships. Within this environment, LSE Cannabis stocks encompass cultivators, biopharmaceutical developers and intellectual-property licensors. This expansion reflects broader acceptance of therapeutic applications and consumer-oriented products across European markets.
Market Landscape A range of small-cap and mid-cap entities populates the LSE Cannabis stocks segment. Cultivation specialists focus on greenhouse operations, while research-based companies develop extraction techniques for botanicals. Licensing outfits secure patent portfolios, generating royalty streams. This diversity shapes the overall risk profile and influences the selection of firms noted for performance.
Dividend Yield Overview Dividend yields among LSE Cannabis stocks vary widely, with some issuers offering double-digit percentages based on last reported distributions and current share prices. Entities aiming for stable payout ratios emphasize established revenue streams from licensing agreements. Those securing the often maintain conservative payout policies, balancing reinvestment in research with distribution to equity holders.
Factors Driving Yield Levels Several elements contribute to the pursuit of status within the LSE Cannabis stocks cohort:
Revenue Diversification: Firms combining cultivation revenue with licensing royalties can allocate funds toward sustained dividend programs.
Cost Management: Entities optimizing indoor-farming and processing expenses preserve cash flow for distribution.
Regulatory Positioning: Companies with early market authorizations benefit from first-mover revenue streams, supporting yield consistency.
Comparative Metrics Key metrics for evaluating dividend performance include payout ratio, free-cash-flow coverage and yield relative to sector averages. Several firms report payout ratios below industry averages, indicating capacity for yield maintenance. Licensors often display higher yields due to low capital-expenditure requirements, positioning them among those with statistics.
Call to Action Explore the latest tables of LSE Cannabis stocks to identify entities offering metrics and review quarterly distribution reports on the official exchange platform.
0 notes
Text
Get Placed Faster with These Best Job Oriented Classes in Bangalore
Best Coaching Centres in Bangalore (2025), Senfine Academy | Call - 9845412266
Best Job-Oriented Classes in Bangalore for IT, Marketing, Finance & More
In today’s fast-paced professional landscape, upskilling is no longer a luxury—it’s a necessity. As industries evolve and new technologies emerge, job seekers and professionals alike are turning to short-term, job-oriented certification courses to stay ahead. Bangalore, known as India’s Silicon Valley, continues to be a hub for career development, offering a wide array of career-focused courses in 2025.
Whether you're a fresh graduate or a working professional looking to pivot or advance in your career, Bangalore offers diverse certification programs that cater to current market needs. Here’s a look at some of the most sought-after job-oriented courses in 2025:
1. Digital Marketing Certification
Digital marketing remains a booming sector, and with businesses expanding their online presence, demand for skilled digital marketers is at an all-time high. Courses typically cover SEO, social media marketing, Google Ads, content strategy, analytics, and email marketing. These programs are ideal for those eyeing careers in brand management, online advertising, or freelance marketing services.
2. Full Stack Development Course
Tech roles continue to dominate the job market, and full stack development is a versatile skillset highly sought after by startups and tech giants alike. Certification courses train learners in both front-end and back-end technologies, covering programming languages, database handling, and deployment. This is a great pick for anyone looking to become a software developer or tech entrepreneur.
3. Data Science and Analytics Program
Data is the new oil, and professionals who can interpret it are in demand. A certification in data science typically includes Python programming, statistics, data visualization, machine learning, and real-world project exposure. This course is ideal for individuals aiming to work as data analysts, data scientists, or AI professionals.
4. UI/UX Design Certification
As companies aim to improve user experience, UI/UX design skills are growing in demand. These courses focus on user research, wireframing, prototyping, and interaction design. Learners also gain exposure to industry-standard tools like Figma, Sketch, and Adobe XD. This creative yet strategic career path is perfect for aspiring designers and product thinkers.
5. Cybersecurity Training
With the rise of online transactions and digital businesses, cybersecurity has become critical. Certification programs in this field focus on ethical hacking, network security, malware analysis, and risk management. This course is recommended for IT professionals, network engineers, and anyone interested in protecting digital infrastructures.
6. Business Analytics Certification
Business analytics bridges the gap between IT and business strategy. These programs teach Excel modeling, SQL, business intelligence tools, and decision-making techniques. It’s a powerful course for professionals in finance, operations, or strategic planning roles.
7. Cloud Computing Certification
Cloud computing skills are essential in today’s remote-first tech world. This course teaches cloud architecture, deployment models, virtualization, and platforms like AWS, Microsoft Azure, and Google Cloud. Ideal for IT professionals looking to boost their infrastructure or DevOps credentials.
Final Thoughts
The job market in 2025 is evolving rapidly, and Bangalore continues to lead as a center for professional advancement. Choosing a certification course that aligns with your interests and career goals can give you the competitive edge needed to succeed.
These job-oriented courses not only enhance your technical and soft skills but also boost your employability in top industries such as IT, marketing, finance, and design. Whether you're looking to transition careers or climb the ladder in your current role, now is the perfect time to invest in yourself.
0 notes