#Python roadmap for machine learning
Explore tagged Tumblr posts
Text
Complete Roadmap To Become Python Developer-2023
If you are aspiring to learn Python programming but don’t know where to start. today’s podcast episode is dedicated to a step-by-step roadmap to becoming a Python developer.
#Roadmap to become Python developer#steps to become a Python developer#Python roadmap for machine learning#Python roadmap for data science#guide to become a python developer#python course in delhi#python training in delhi
0 notes
Text
The Next Wave: Exploring AI and Machine Learning Roadmap in 2024
What is AI and Machine Learning?
Artificial Intelligence (AI):
AI is a broad area of computer science that focuses on creating systems or machines capable of performing tasks that typically require human intelligence. These tasks include problem-solving, learning, perception, understanding natural language, and even decision-making. AI can be categorized into two types:
Narrow or Weak AI: This type of AI is designed to perform a specific task, such as speech recognition or image classification. It operates within a limited domain and doesn't possess generalized intelligence.
General or Strong AI: This is the hypothetical idea of AI possessing the ability to understand, learn, and apply knowledge across various domains, similar to human intelligence. Strong AI, however, is more a concept than a current reality.
Machine Learning (ML):
ML is a subset of AI that focuses on developing algorithms and statistical models that enable computers to perform a task without explicit programming. Instead of being explicitly programmed to perform a task, a machine learning system learns from data and improves its performance over time. ML can be categorized into three main types:
Supervised Learning: The model is trained on a labeled dataset, where the algorithm is provided with input-output pairs. It learns to map inputs to outputs, allowing it to make predictions or classifications on new, unseen data.
Unsupervised Learning: The model is given unlabeled data and is tasked with finding patterns or structures within it. Clustering and dimensionality reduction are common applications of unsupervised learning.
Reinforcement Learning: The algorithm learns by interacting with an environment. It receives feedback in the form of rewards or penalties, allowing it to learn optimal strategies for decision-making.
Edge of AI Machine Learning Roadmap
We stand at the threshold of 2024, and the landscape of Artificial Intelligence (AI) and Machine Learning (ML) unfurls a roadmap teeming with possibilities and innovation. This blog endeavors to elucidate the key trends, advancements, and transformative shifts that mark the AI and ML journey in the year 2024.
Continued Advancements in Deep Learning:
The year 2024 sees Deep Learning, a vanguard in ML, continuing its relentless march forward. Expect breakthroughs in neural network architectures, optimization algorithms, and training techniques, propelling the boundaries of what's achievable in AI applications.
AI for Edge Computing and Federated Learning:
Edge AI and Federated Learning take center stage as the paradigm shifts toward decentralized computing. In 2024, we witness a surge in the development of AI models capable of operating on edge devices, fostering real-time processing, and reducing reliance on centralized cloud servers.
Explainable AI (XAI) Reaches Maturity:
Explainability becomes a paramount concern in AI systems. In 2024, Explainable AI (XAI) matures, providing a clearer lens into the decision-making processes of complex algorithms. This transparency is crucial, especially in fields like healthcare, finance, and autonomous systems.
AI Ethics Takes Center Stage:
The ethical dimensions of AI gain prominence. With increasing societal reliance on AI, 2024 emphasizes the need for responsible AI development. Stricter ethical guidelines, frameworks, and regulations come into play to ensure fairness, transparency, and accountability in AI applications.
AI in Healthcare Revolutionized:
The intersection of AI and healthcare will witness a revolution in 2024. Advanced diagnostic tools, personalized medicine, and predictive analytics powered by machine learning algorithms redefine patient care. AI has become an indispensable ally in the quest for improved healthcare outcomes.
Quantum Computing Impact on ML:
The emergence of quantum computing leaves an indelible mark on ML. In 2024, we witness the exploration of quantum algorithms for machine learning tasks, promising exponential speedups in solving complex problems that were once computationally intractable.
AI Democratization and Accessibility:
The democratization of AI tools and technologies reaches new heights. In 2024, user-friendly platforms, open-source frameworks, and simplified interfaces empower a broader demographic to harness the capabilities of AI and ML, fostering innovation across diverse domains.
AI-powered Cybersecurity:
As cyber threats become more sophisticated, AI steps up as a formidable ally in cybersecurity. Machine learning models in 2024 demonstrate enhanced capabilities in detecting and thwarting cyberattacks, fortifying digital landscapes against evolving threats.
Hybrid Models and Interdisciplinary Collaboration:
The synergy between AI, ML, and other disciplines gains traction. In 2024, hybrid models that amalgamate different AI approaches find applications in diverse fields. Interdisciplinary collaboration becomes the norm, as AI professionals work alongside experts in various domains to solve complex problems.
Continuous Learning and Skill Evolution:
The pace of innovation in AI demands a commitment to continuous learning. In 2024, professionals in the field prioritize ongoing skill development, staying abreast of the latest advancements, and engaging in collaborative communities to foster a culture of knowledge exchange.
Conclusion:
The AI and Machine Learning roadmap for 2024 is a journey marked by innovation, ethical considerations, and the democratization of technology. As we navigate this dynamic landscape, the convergence of technological advancements and societal responsibility will define the narrative of progress. Whether in healthcare, cybersecurity, or interdisciplinary collaboration, the year 2024 unfolds a tapestry of possibilities, inviting professionals and enthusiasts alike to contribute to the ongoing evolution of AI and ML, shaping a future where intelligent systems coalesce seamlessly with the aspirations of a rapidly advancing world.
#machine learning#artificial intelligence#ai#technology#computer science#science#deep science#techinnovation#tech industry#technically#programming#software development#deep learning#datascience#data analytics#python#roadmap#future#futuristic#2024
0 notes
Text
instagram
Hey there! 🚀 Becoming a data analyst is an awesome journey! Here’s a roadmap for you:
1. Start with the Basics 📚:
- Dive into the basics of data analysis and statistics. 📊
- Platforms like Learnbay (Data Analytics Certification Program For Non-Tech Professionals), Edx, and Intellipaat offer fantastic courses. Check them out! 🎓
2. Master Excel 📈:
- Excel is your best friend! Learn to crunch numbers and create killer spreadsheets. 📊🔢
3. Get Hands-on with Tools 🛠️:
- Familiarize yourself with data analysis tools like SQL, Python, and R. Pluralsight has some great courses to level up your skills! 🐍📊
4. Data Visualization 📊:
- Learn to tell a story with your data. Tools like Tableau and Power BI can be game-changers! 📈📉
5. Build a Solid Foundation 🏗️:
- Understand databases, data cleaning, and data wrangling. It’s the backbone of effective analysis! 💪🔍
6. Machine Learning Basics 🤖:
- Get a taste of machine learning concepts. It’s not mandatory but can be a huge plus! 🤓🤖
7. Projects, Projects, Projects! 🚀:
- Apply your skills to real-world projects. It’s the best way to learn and showcase your abilities! 🌐💻
8. Networking is Key 👥:
- Connect with fellow data enthusiasts on LinkedIn, attend meetups, and join relevant communities. Networking opens doors! 🌐👋
9. Certifications 📜:
- Consider getting certified. It adds credibility to your profile. 🎓💼
10. Stay Updated 🔄:
- The data world evolves fast. Keep learning and stay up-to-date with the latest trends and technologies. 📆🚀
. . .
#programming#programmers#developers#mobiledeveloper#softwaredeveloper#devlife#coding.#setup#icelatte#iceamericano#data analyst road map#data scientist#data#big data#data engineer#data management#machinelearning#technology#data analytics#Instagram
8 notes
·
View notes
Text
How to Become a Data Scientist in 2025 (Roadmap for Absolute Beginners)
Want to become a data scientist in 2025 but don’t know where to start? You’re not alone. With job roles, tech stacks, and buzzwords changing rapidly, it’s easy to feel lost.
But here’s the good news: you don’t need a PhD or years of coding experience to get started. You just need the right roadmap.
Let’s break down the beginner-friendly path to becoming a data scientist in 2025.
✈️ Step 1: Get Comfortable with Python
Python is the most beginner-friendly programming language in data science.
What to learn:
Variables, loops, functions
Libraries like NumPy, Pandas, and Matplotlib
Why: It’s the backbone of everything you’ll do in data analysis and machine learning.
🔢 Step 2: Learn Basic Math & Stats
You don’t need to be a math genius. But you do need to understand:
Descriptive statistics
Probability
Linear algebra basics
Hypothesis testing
These concepts help you interpret data and build reliable models.
📊 Step 3: Master Data Handling
You’ll spend 70% of your time cleaning and preparing data.
Skills to focus on:
Working with CSV/Excel files
Cleaning missing data
Data transformation with Pandas
Visualizing data with Seaborn/Matplotlib
This is the “real work” most data scientists do daily.
🧬 Step 4: Learn Machine Learning (ML)
Once you’re solid with data handling, dive into ML.
Start with:
Supervised learning (Linear Regression, Decision Trees, KNN)
Unsupervised learning (Clustering)
Model evaluation metrics (accuracy, recall, precision)
Toolkits: Scikit-learn, XGBoost
🚀 Step 5: Work on Real Projects
Projects are what make your resume pop.
Try solving:
Customer churn
Sales forecasting
Sentiment analysis
Fraud detection
Pro tip: Document everything on GitHub and write blogs about your process.
✏️ Step 6: Learn SQL and Databases
Data lives in databases. Knowing how to query it with SQL is a must-have skill.
Focus on:
SELECT, JOIN, GROUP BY
Creating and updating tables
Writing nested queries
🌍 Step 7: Understand the Business Side
Data science isn’t just tech. You need to translate insights into decisions.
Learn to:
Tell stories with data (data storytelling)
Build dashboards with tools like Power BI or Tableau
Align your analysis with business goals
🎥 Want a Structured Way to Learn All This?
Instead of guessing what to learn next, check out Intellipaat’s full Data Science course on YouTube. It covers Python, ML, real projects, and everything you need to build job-ready skills.
https://www.youtube.com/watch?v=rxNDw68XcE4
🔄 Final Thoughts
Becoming a data scientist in 2025 is 100% possible — even for beginners. All you need is consistency, a good learning path, and a little curiosity.
Start simple. Build as you go. And let your projects speak louder than your resume.
Drop a comment if you’re starting your journey. And don’t forget to check out the free Intellipaat course to speed up your progress!
2 notes
·
View notes
Text
Mastering the Dig: Your Roadmap to the Top 10 Data Mining Courses
In the digital age, where data is a valuable currency, the field of data mining has emerged as a critical discipline for extracting meaningful insights from vast datasets. Whether you're a student looking to ace your data mining assignments or a professional seeking to enhance your skills, a solid education is paramount. In this blog, we'll guide you through the top 10 data mining courses, with a special emphasis on the exceptional resource – DatabaseHomeworkHelp.com – known for its expertise in providing help with data mining homework.
DatabaseHomeworkHelp.com: Your Expert Companion in Data Mining When it comes to mastering the intricacies of data mining, DatabaseHomeworkHelp.com takes the lead. This website specializes in offering comprehensive solutions for data mining assignments, ensuring that students grasp the concepts and techniques involved. With a team of experienced tutors, DatabaseHomeworkHelp.com is your go-to resource for personalized assistance and in-depth understanding of data mining principles.Why Choose DatabaseHomeworkHelp.com?
Expert Tutors: Benefit from the guidance of experienced tutors who have a deep understanding of data mining concepts and practical applications.
Customized Solutions: Get tailor-made solutions for your data mining homework, addressing your specific requirements and ensuring a clear understanding of the subject.
Timely Delivery: DatabaseHomeworkHelp.com is committed to delivering solutions within deadlines, allowing you to stay on track with your academic schedule.
Affordable Pricing: Enjoy cost-effective solutions without compromising on the quality of assistance you receive.
Now, let's explore other noteworthy resources offering top-notch data mining courses to further enrich your learning experience.
"Data Mining Specialization" by University of Illinois (Coursera) This specialization covers the fundamentals of data mining, including techniques for pattern discovery, clustering, and predictive modeling. It is a comprehensive program suitable for beginners and intermediate learners.
"Practical Machine Learning for Computer Vision" by Stanford University (Coursera) Delve into the intersection of data mining and computer vision with this course. Stanford University's offering focuses on practical applications, making it an excellent choice for those interested in extracting insights from visual data.
"Data Science and Machine Learning Bootcamp with R and Python" by Udemy Led by industry experts, this Udemy bootcamp provides a broad overview of data science and machine learning, making it an ideal choice for individuals seeking a holistic understanding of these fields.
"Text Mining and Analytics" by University of Illinois (Coursera) Explore the world of text mining with this Coursera specialization. The course covers techniques for extracting valuable information from textual data, an essential skill in the data mining domain.
"Advanced Machine Learning Specialization" by National Research University Higher School of Economics (Coursera) This specialization delves into advanced machine learning concepts, providing a solid foundation for those interested in leveraging machine learning algorithms for data mining purposes.
"Data Mining and Analysis" by Columbia University (edX) Columbia University's edX course explores the principles of data mining and analysis, emphasizing real-world applications. It's suitable for learners seeking a practical approach to data mining.
"Introduction to Data Science" by Microsoft (edX) Offered by Microsoft on edX, this course introduces learners to the essentials of data science, including data mining techniques. It's a great starting point for beginners in the field.
"Mining Massive Datasets" by Stanford University (Coursera) For those looking to tackle large datasets, this Stanford University course covers the challenges and solutions associated with mining massive amounts of data, preparing learners for real-world scenarios.
"Data Mining and Machine Learning in Python" by Udemy This Udemy course focuses on data mining and machine learning using Python. With hands-on exercises, it's a practical resource for learners looking to apply data mining techniques using Python programming.
Conclusion: Embarking on a journey to master data mining requires access to high-quality resources and expert guidance. The top 10 data mining courses mentioned in this blog, with a special nod to DatabaseHomeworkHelp.com, cater to a variety of skill levels and interests. Whether you're a student seeking homework assistance or a professional looking to enhance your data mining skills, these courses provide a robust foundation for success in the dynamic field of data mining. Start your learning journey today and unlock the potential of data mining expertise.
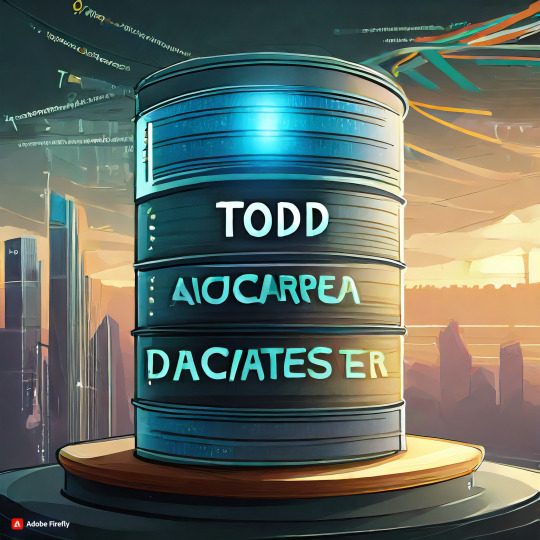
12 notes
·
View notes
Text
Embarking on the Odyssey of Data Science Mastery: A Comprehensive 8-Step Guide
Navigating the dynamic waters of data science requires a combination of technical acumen and analytical finesse. This comprehensive guide unfolds an 8-step roadmap, not just to become a data scientist but to master the intricate art and science that defines this dynamic field.
1. Building a Sturdy Mathematical Foundation: The Pillars of Understanding
Embark on your journey with a laser focus on constructing a formidable foundation in fundamental mathematical principles, probability theory, and statistics. This foundational knowledge serves as the bedrock upon which you'll interpret data and seamlessly implement statistical models with precision.
2. Mastering Programming Languages: The Data Scientist's Linguistic Proficiency
In the vast toolkit of a data scientist, mastery in a programming language is key. Delve into the intricacies of Python or R, navigating the landscape of data manipulation libraries like Pandas or data.table. These tools become your allies in handling large datasets with finesse.
3. Navigating Data Analysis Libraries: Unleashing the Power of Manipulation
With programming proficiency comes the need to manipulate and analyze data efficiently. This is where libraries like Pandas (Python) and dplyr (R) shine. Pandas, known for its ease of use, provides data structures and functions needed to manipulate structured data seamlessly, while dplyr simplifies data manipulation in R.
4. Crafting Visual Narratives: The Art of Data Visualization
Transforming complex insights into compelling visual narratives is the hallmark of a skilled data scientist. Immerse yourself in the world of data visualization tools such as Matplotlib, Seaborn, or ggplot2. Through mastery of these tools, your analytical findings metamorphose into visually striking stories.
5. Navigating the Landscape of Machine Learning Basics: Algorithms as Your Guide
Machine learning (ML) is the heart of data science. Start with the basics – linear regression, logistic regression, and classification algorithms. Platforms like Scikit-Learn (Python) and caret (R) provide practical implementations. A solid grasp of the fundamentals empowers you to tackle a myriad of real-world problems.
6. Bridging Theory and Practice: The Power of Hands-On Projects
Theoretical knowledge gains potency when applied. Platforms like Kaggle provide datasets and competitions, allowing you to test your skills in a real-world context. Building a portfolio of projects showcases your skills to potential employers and collaborators.
7. Structured Learning Paths: The Guiding Compass of Online Courses
The world of data science is vast, and structured courses can act as your compass. Platforms like Coursera, edX, and Udacity offer courses tailored to various aspects of data science. These courses provide not just theoretical knowledge but hands-on experience, ensuring a well-rounded education.
8. Learning Together: The Vibrant Tapestry of Community Engagement
Data science is not a solitary pursuit. Engage with the community. Platforms like Stack Overflow, Reddit (r/datascience), and GitHub offer spaces to ask questions, share insights, and learn from experienced practitioners. Actively participating in the data science community exposes you to the latest trends, tools, and best practices.
Conclusion: A Journey of Continuous Discovery Embarking on the data science odyssey is not a sprint; it's a marathon of continuous learning. Each step in this guide is a stepping stone, guiding you through the intricate landscape of data science. As you navigate through the sea of data, may your curiosity be your compass, and may the insights you unearth become beacons of knowledge in the vast realm of data science. Best of luck on your journey!
#data science course#datascience#technology#data science certification#data science training#tech#education
4 notes
·
View notes
Text
Why Chennai Is Home to the Best Software Development Company in India

Chennai has emerged as one of Asia’s leading IT hubs, earning its reputation as the go‑to destination for businesses seeking premier software development services. When you search for the best software development company in Chennai, you’re tapping into a pool of skilled developers, innovative methodologies, and cutting‑edge technologies that power digital transformation across industries.
1. What Makes a “Best Software Development Company in Chennai”?
Technical Expertise
Proficiency across multiple stacks: Java/.NET, Python/Django, JavaScript (React, Angular, Vue), and mobile frameworks (Flutter, React Native).
In‑house specialists in emerging fields: AI/ML, blockchain, IoT, and cloud‑native architectures.
Proven Delivery Model
Agile and DevOps practices ensure iterative delivery, rapid feedback, and continuous integration/continuous deployment (CI/CD).
Strong project governance, transparency, and clear communication channels.
Domain Experience
Track record in key verticals: finance, healthcare, e‑commerce, education, and logistics.
Case studies showcasing measurable ROI, performance uplift, and scalability.
Customer‑Centric Culture
Dedicated account managers and cross‑functional teams focused on aligning with your business goals.
Post‑launch support, maintenance, and strategic roadmap planning.
2. Why Chennai? Advantages of Partnering with a Software Development Company in Chennai
Cost‑Effectiveness Salaries and operational costs in Chennai remain competitive compared to many Western and other Asian IT centers—without compromising on talent quality.
Rich Talent Pool Chennai’s tech ecosystem is fueled by premier engineering colleges (like IIT Madras and Anna University) and institutes that produce thousands of skilled graduates yearly.
Time‑Zone Compatibility Working with teams in IST (UTC +5:30) enables convenient overlap with Europe and even partial hours with the Americas, fostering real‑time collaboration and faster turnaround.
Robust IT Infrastructure State‑of‑the‑art tech parks, reliable power supply, and extensive broadband connectivity make it easy to scale projects up or down.
3. Core Services Offered by a Top Software Development Company in Chennai
Custom Software Development
Tailor‑made solutions that fit your unique workflows and user requirements.
End‑to‑end services from requirement gathering and prototyping to deployment and beyond.
Web & Mobile App Development
Responsive, progressive web applications (PWAs) built on modern JavaScript frameworks.
Native and cross‑platform mobile apps with focus on performance, security, and user experience.
Enterprise Solutions & ERP/CRM
Scalable enterprise resource planning (ERP) and customer relationship management (CRM) systems.
Seamless integration with existing databases, third‑party APIs, and legacy systems.
Cloud Services & DevOps
Architecting cloud‑native applications on AWS, Azure, or Google Cloud Platform.
Automated CI/CD pipelines, containerization (Docker, Kubernetes), and infrastructure as code (Terraform).
Data Analytics & AI/ML
Data warehousing, BI dashboards, and predictive analytics to turn raw data into actionable insights.
Custom machine learning models for recommendation engines, fraud detection, and image/text processing.
Quality Assurance & Testing
Comprehensive testing services, including functional, performance, security, and usability testing.
Test automation frameworks to accelerate release cycles.
4. How to Choose the Best Software Development Company in Chennai
Portfolio & Case Studies Review past projects, industry verticals, and client testimonials to gauge diversity and depth of expertise.
Technical Assessment Conduct small pilot projects or technical evaluations to verify coding standards, architecture clarity, and responsiveness.
Communication & Cultural Fit Ensure the team communicates clearly, respects deadlines, and aligns with your company culture and values.
Pricing Model & Engagement Terms Compare fixed‑price, time‑and‑materials, and dedicated‑team models to find the best fit for budget flexibility and long‑term collaboration.
Post‑Launch Support Clarify SLAs for maintenance, bug fixes, and feature enhancements to keep your application evolving smoothly.
5. Spotlight: Success Stories
E‑Commerce Transformation A mid‑sized retailer partnered with a leading Software Development Company in Chennai to migrate from a monolithic platform to microservices on AWS. Results: 40% faster page loads, 99.9% uptime, and a 25% uplift in conversion rate.
Healthcare Analytics Platform A healthcare startup tapped Chennai’s AI/ML talent to build a predictive analytics engine for patient risk scoring. After deployment, the platform improved early intervention rates by 30%.
6. Conclusion & Next Steps
Choosing the best software development company in Chennai means more than just engaging a vendor—it’s about forging a strategic partnership that accelerates innovation, optimizes costs, and delivers tangible business outcomes.
Define Your Goals: Outline your project scope, objectives, and KPIs.
Shortlist Vendors: Look for proven expertise, domain knowledge, and cultural alignment.
Engage & Evaluate: Run a pilot, validate technical capabilities, and confirm communication processes.
Scale & Innovate: Once chosen, leverage Chennai’s talent ecosystem to continuously evolve your digital product.
Ready to elevate your software journey? Partner with a premier Software Development Company in Chennai and turn your vision into reality.
0 notes
Text
Mastering AI on Kubernetes: A Deep Dive into the Red Hat Certified Specialist in OpenShift AI
Artificial Intelligence (AI) is no longer a buzzword—it's a foundational technology across industries. From powering recommendation engines to enabling self-healing infrastructure, AI is changing the way we build and scale digital experiences. For professionals looking to validate their ability to run AI/ML workloads on Kubernetes, the Red Hat Certified Specialist in OpenShift AI certification is a game-changer.
What is the OpenShift AI Certification?
The Red Hat Certified Specialist in OpenShift AI certification (EX480) is designed for professionals who want to demonstrate their skills in deploying, managing, and scaling AI and machine learning (ML) workloads on Red Hat OpenShift AI (formerly OpenShift Data Science).
This hands-on exam tests real-world capabilities rather than rote memorization, making it ideal for data scientists, ML engineers, DevOps engineers, and platform administrators who want to bridge the gap between AI/ML and cloud-native operations.
Why This Certification Matters
In a world where ML models are only as useful as the infrastructure they run on, OpenShift AI offers a powerful platform for deploying and monitoring models in production. Here’s why this certification is valuable:
🔧 Infrastructure + AI: It merges the best of Kubernetes, containers, and MLOps.
📈 Enterprise-Ready: Red Hat is trusted by thousands of companies worldwide—OpenShift AI is production-grade.
💼 Career Boost: Certifications remain a proven way to stand out in a crowded job market.
🔐 Security and Governance: Demonstrates your understanding of secure, governed ML workflows.
Skills You’ll Gain
Preparing for the Red Hat OpenShift AI certification gives you hands-on expertise in areas like:
Deploying and managing OpenShift AI clusters
Using Jupyter notebooks and Python for model development
Managing GPU workloads
Integrating with Git repositories
Running pipelines for model training and deployment
Monitoring model performance with tools like Prometheus and Grafana
Understanding OpenShift concepts like pods, deployments, and persistent storage
Who Should Take the EX267 Exam?
This certification is ideal for:
Data Scientists who want to operationalize their models
ML Engineers working in hybrid cloud environments
DevOps Engineers bridging infrastructure and AI workflows
Platform Engineers supporting AI workloads at scale
Prerequisites: While there’s no formal prerequisite, it’s recommended you have:
A Red Hat Certified System Administrator (RHCSA) or equivalent knowledge
Basic Python and machine learning experience
Familiarity with OpenShift or Kubernetes
How to Prepare
Here’s a quick roadmap to help you prep for the exam:
Take the RHODS Training: Red Hat offers a course—Red Hat OpenShift AI (EX267)—which maps directly to the exam.
Set Up a Lab: Practice on OpenShift using Red Hat’s Developer Sandbox or install OpenShift locally.
Learn the Tools: Get comfortable with Jupyter, PyTorch, TensorFlow, Git, S2I builds, Tekton pipelines, and Prometheus.
Explore Real-World Use Cases: Try deploying a sample model and serving it via an API.
Mock Exams: Practice managing user permissions, setting up notebook servers, and tuning ML workflows under time constraints.
Final Thoughts
The Red Hat Certified Specialist in OpenShift AI certification is a strong endorsement of your ability to bring AI into the real world—securely, efficiently, and at scale. If you're serious about blending data science and DevOps, this credential is worth pursuing.
🎯 Whether you're a data scientist moving closer to DevOps, or a platform engineer supporting data teams, this certification puts you at the forefront of MLOps in enterprise environments.
Ready to certify your AI skills in the cloud-native era? Let OpenShift AI be your launchpad.
For more details www.hawkstack.com
0 notes
Text
Mastering Data Science: A Roadmap for Beginners and Aspiring Professionals
Understanding the Foundation of Data Science
Data science has emerged as one of the most sought-after career paths in today’s digital economy. It combines statistics, computer science, and domain knowledge to extract meaningful insights from data. Before diving deep into complex topics, it’s crucial to understand the foundational concepts that shape this field. From data cleaning to basic data visualization techniques, beginners must grasp these essential skills. Additionally, programming languages like Python and R are the primary tools used by data scientists worldwide. Building a strong base in these languages can set the stage for more advanced learning. It’s also important to familiarize yourself with databases, as querying and manipulating data efficiently is a key skill in any data-driven role. Solidifying these basics ensures a smoother transition to more complex areas such as artificial intelligence and machine learning.
Machine Learning for Beginners: The Essential Guide
Once you have a solid foundation, the next logical step is to explore machine learning. Machine Learning for Beginners is an exciting journey filled with numerous algorithms and techniques designed to help computers learn from data. Beginners should start with supervised learning models like linear regression and decision trees before progressing to unsupervised learning and reinforcement learning. Understanding the mathematical intuition behind algorithms such as k-nearest neighbors (KNN) and support vector machines (SVM) can enhance your analytical skills significantly. Online resources, workshops, and hands-on projects are excellent ways to strengthen your knowledge. It’s also vital to practice with real-world datasets, as this will expose you to the challenges and nuances faced in actual data science projects. Remember, mastering machine learning is not just about memorizing algorithms but about understanding when and why to use them.
Interview Preparation for Data Scientists: Key Strategies
Entering the job market as a data scientist can be both thrilling and intimidating. Effective interview preparation for data scientists requires more than just technical knowledge; it demands strategic planning and soft skill development. Candidates should be prepared to tackle technical interviews that test their understanding of statistics, machine learning, and programming. Additionally, behavioral interviews are equally important, as companies seek individuals who can collaborate and communicate complex ideas clearly. Mock interviews, coding challenges, and portfolio projects can significantly boost your confidence. It is beneficial to review common interview questions, such as explaining the bias-variance tradeoff or detailing a machine learning project you have worked on. Networking with professionals and seeking mentorship opportunities can also open doors to valuable insights and career advice. A strong preparation strategy combines technical mastery with effective storytelling about your experiences.
Advancing Your Data Science Career Through Specialization
After entering the field, data scientists often find themselves gravitating towards specialized roles like machine learning engineer, data analyst, or AI researcher. Specializing allows professionals to deepen their expertise and stand out in a competitive job market. Those passionate about prediction models might specialize in machine learning, while others who enjoy working with big data might lean towards data engineering. Continuous learning is essential in this rapidly evolving field. Enrolling in advanced courses, attending industry conferences, and contributing to open-source projects can all accelerate your career growth. Furthermore, staying updated with the latest tools and technologies, such as cloud-based machine learning platforms and advanced data visualization libraries, can give you an edge. A proactive approach to career development ensures you remain adaptable and competitive, regardless of how the industry changes.
Conclusion: Your Gateway to Success in Data Science
The journey to becoming a successful data scientist is both challenging and rewarding. It requires a balance of technical knowledge, practical experience, and continuous learning. Building a strong foundation, mastering machine learning basics, strategically preparing for interviews, and eventually specializing in a niche area are all key steps toward achieving your career goals. For those seeking comprehensive resources to guide them through every phase of their journey, visiting finzebra.com offers access to valuable tools and insights tailored for aspiring data science professionals. By following a structured learning path and leveraging the right resources, anyone can transform their passion for data into a fulfilling career.
0 notes
Text
Stream episode Roadmap To Become A Python Developer- 2023 by Deepak Garhwal
If you are aspiring to learn Python programming but don’t know where to start. today’s podcast episode is dedicated to a step-by-step roadmap to becoming a Python developer.
#Roadmap to become Python developer#steps to become a Python developer#Python roadmap for machine learning#Python roadmap for data science#guide to become a python developer#python course in delhi#python training in delhi#path to become a python developer#how to become a python developer#Python developer roadmap#Python programmer roadmap#Python roadmap for beginners 2023#SoundCloud
0 notes
Text
Best Python Training Institute in Kerala: A Roadmap to Building a Successful Tech Career
Python has taken the tech world by storm. Its simplicity, readability, and versatility make it one of the most sought-after programming languages today. From web development and automation to data science and artificial intelligence, Python plays a central role across various domains. For aspiring developers and working professionals alike, mastering Python is no longer optional—it’s essential.
If you’re in Kerala and planning to begin or upskill your programming journey, finding the Best Python Training Institute in Kerala can be the stepping stone to your success. This guide explores the importance of Python, what a high-quality training program should include, and why Zoople Technologies stands out as a top choice.
Why Python is a Must-Learn Language in 2025
1. Beginner-Friendly Yet Powerful
Python’s syntax is intuitive and close to natural English, making it ideal for beginners. At the same time, it is robust enough to power applications used by giants like Google, Netflix, and NASA.
2. Wide Range of Applications
Python is widely used in:
Web development (using frameworks like Django and Flask)
Data science and analytics
Machine learning and AI
Game development
Internet of Things (IoT)
Scripting and automation
This versatility ensures that learning Python opens doors to various career paths.
3. High Market Demand
Companies across the globe are hiring Python developers for diverse roles. In India, especially in Kerala’s growing IT hubs like Kochi, Trivandrum, and Calicut, Python-skilled professionals are in strong demand.
4. Thriving Community and Resources
Python boasts a massive global community. Learners can access an abundance of tutorials, open-source projects, and peer support forums to accelerate their growth.
What Should You Expect from the Best Python Training Institute in Kerala?
To ensure your time, money, and effort are well invested, here’s what the Best Python Training Institute in Kerala should offer:
1. Comprehensive Curriculum
A top-tier training program should cover:
Core concepts: Variables, data types, operators, control structures
Functions and object-oriented programming
Error handling and file operations
Python libraries: NumPy, Pandas, Matplotlib
Web frameworks: Django and Flask
Real-world project development
Basics of APIs, version control (Git), and deployment techniques
2. Hands-On Learning
Theory alone won’t make you job-ready. Look for institutes that emphasize:
Live coding sessions
Real-time project implementation
Capstone projects
Coding challenges and assessments
3. Qualified and Experienced Trainers
Learning under experienced mentors can fast-track your understanding and confidence. Trainers should:
Have industry experience
Offer personalized mentorship
Be updated with current tech trends
4. Flexible Learning Modes
The institute should cater to diverse learners—students, professionals, and career switchers—offering:
Online and offline batches
Weekday and weekend classes
Access to recorded sessions
5. Certification and Placement Support
A recognized certificate from a reputed institute adds value to your resume. Placement assistance, resume building, and interview prep are key offerings of a leading training institute.
Career Prospects After Learning Python
Python’s wide application spectrum means you can pursue multiple in-demand roles such as:
Python Developer
Full Stack Web Developer
Data Analyst
Machine Learning Engineer
Backend Developer
Automation Tester
Kerala’s tech ecosystem is expanding rapidly, especially in cities like Kochi, Technopark Trivandrum, and Kozhikode Cyberpark. Companies here are constantly scouting for skilled Python professionals. Additionally, with remote work opportunities increasing, Kerala-based professionals can now work for global companies from the comfort of their hometown.
Why Kerala is an Ideal Destination for Python Training
Kerala, known for its high literacy rate and educational infrastructure, is quickly transforming into a technological education hub. Here’s why Choosing The Best Python Training Institute in Kerala makes sense:
Growing IT Hubs: Cities like Kochi and Trivandrum are witnessing exponential growth in IT infrastructure.
Affordable Education: High-quality education at lower costs compared to metros like Bangalore or Hyderabad.
Excellent Connectivity: With a strong internet presence and robust digital infrastructure, learners in Kerala can easily access online resources and hybrid learning options.
Supportive Government Policies: The Kerala Startup Mission and other government initiatives are pushing tech education and employment aggressively.
Whether you're a student or a working professional, Kerala offers the right ecosystem to build and grow your tech skills.
Zoople Technologies – The Best Python Training Institute in Kerala
When it comes to high-quality, job-oriented training, Zoople Technologies stands out as the Best Python Training Institute in Kerala. With a proven track record of creating skilled developers and a student-first approach, Zoople has emerged as a trusted name in the tech training landscape.
Why Zoople Technologies?
Industry-Relevant Curriculum: Zoople’s course content is designed by experienced developers and is regularly updated to match industry trends.
Project-Based Learning: Students work on live projects that mimic real-world challenges, giving them hands-on experience that employers value.
Experienced Trainers: The instructors are not just teachers—they are professionals who bring industry insights directly into the classroom.
Flexible Batches: Whether you’re a student or a working professional, Zoople offers batch timings that suit your schedule, including online options.
Placement Support: Zoople has a dedicated placement team that assists students with job applications, interview preparation, and resume building.
Supportive Learning Environment: With one-on-one mentoring, practical labs, and open forums, Zoople ensures every student gets the attention they need to succeed.
Zoople Technologies has earned its reputation by consistently producing industry-ready professionals who land jobs in top companies across India and abroad.
Final Thoughts
In the world of programming, Python is your gateway language—and choosing the Best Python Training Institute in Kerala is the first step toward a rewarding tech career. Kerala offers the right environment to learn and grow, and Zoople Technologies provides the quality, support, and expertise needed to turn your ambition into a profession.
If you're serious about a future in tech and want to learn from the best, Zoople Technologies is where your journey should begin.
0 notes
Text
In-House vs. Remote: What’s the Best Way to Hire a Computer Vision Engineer?

Computer vision has been reshaping industries from facial recognition, autonomous vehicles, to medical imaging and retail analysis. As demand proliferates, so does the challenge to find the right experts. Whether a startup or a growing tech company investing in AI, the key question in the picture is: should you opt to hire remote developers or in-house professionals for computer vision roles.
Both options offer their own pros and cons, but the best choice depends on your project scope, budget, and team structure. To understand this in more detail let’s read furthermore.
In-House vs. Remote: What’s the Best Way to Hire a Computer Vision Engineer?
Let’s first explore the traditional and most-preferred hiring approach of in-house developers. Hiring computer vision engineers in-house brings obvious advantages, especially when your project involves ongoing development, close collaboration, or sensitive data.
Having a dedicated engineer on-site will make it easier to coordinate with product managers, data scientists, and developers. You must opt for in-house when:
You must work closely with cross-functional teams.
The project is crucial to your roadmap for products.
Working with sensitive or private datasets.
You intend to make long-term innovation investments.
An internal computer vision specialist can fully own the design of the vision system, contribute continuously to the tech stack, and become a part of the corporate culture.
Why Remote Hiring Is Gaining Ground
Unlike advancements in technology, hiring approaches have also evolved, especially post-pandemic. Hiring remotely from a global talent pool has become a preferred approach among several tech companies and startups.
Many tech companies now prefer to hire AI engineers remotely - especially when looking for rare or specialized skills. Remote hiring offers you access to a global talent network, growing your chances of finding the exact expertise you need. Go remote when:
For a particular use case, such as object tracking or picture segmentation, you require a specialist.
Hiring time is crucial or limited.
You're operating on a tight budget.
You desire flexibility without committing to anything long-term.
Hiring remote developers lowers overhead expenses, particularly in the fields of AI and machine learning. In many areas, pay can be lower without compromising quality, and there's no need to move staff or offer office space.
Consider Hybrid or Project-Based Models
Sometimes combining the two is the best course of action. As you gradually assemble an internal team, you may hire a remote engineer for temporary project or consulting work. This enables you to make rapid progress while developing long-term skills.
Depending on the size of the project, many teams also employ ML or AI engineers to collaborate with computer vision experts. A flexible model keeps your core staff small and concentrated while filling in gaps.
Tips for Hiring the Right Talent
Hiring the best candidate, whether in-house or remote, necessitates having a thorough grasp of your requirements. Identify the precise issues you wish to resolve, such as video analytics, facial recognition, or image classification, and compare them to the engineer's background.
Look for the following when hiring computer vision engineers:
A solid foundation in PyTorch, TensorFlow, OpenCV, or Python.
Knowledge of practical applications (not simply scholarly research).
Case studies or a portfolio demonstrating quantifiable impact.
Excellent communication abilities, particularly for jobs requiring remote work.
Screening for collaborative style is also beneficial. Working across time zones with platforms like Slack, GitHub, and project boards requires self-motivation and comfort on the part of remote engineers.
0 notes
Text
Breaking into Data Science: Skills and Roadmap for 2025
With data becoming the backbone of decision-making across industries, many are turning to data science as a promising career. If you're planning to start your journey in this field, enrolling in the best data science training in Hyderabad can be your first step toward success. This rapidly evolving domain offers countless opportunities for those equipped with the right knowledge and practical experience.
Must-Have Skills for Aspiring Data Scientists
To succeed in data science, you need a mix of programming, analytics, and problem-solving skills. Begin by learning programming languages like Python, R, and SQL, which are essential for data handling and analysis. A strong understanding of statistical concepts and mathematical reasoning helps in building accurate models. Additionally, knowing how to create clear and impactful visuals using tools like Tableau or Power BI will set you apart.
Why Machine Learning Matters
Machine learning is a key part of data science, enabling systems to learn and improve without being explicitly programmed. Understand concepts such as classification, clustering, and regression. Learn how to choose and evaluate algorithms for different types of problems. Gaining experience by working on practical ML projects is one of the best ways to sharpen your skills.
A Simple Roadmap to Get Started
Master the Basics – Learn core programming, statistics, and data handling.
Work with Real Data – Practice cleaning and analyzing datasets.
Explore Machine Learning – Understand algorithms and apply them.
Build Real Projects – Create your portfolio to showcase your work.
Stay Current – Keep learning new tools and industry practices.
Conclusion
Starting a career in data science is both exciting and rewarding. For those seeking expert guidance and hands-on training, SSSIT Computer Education in Hyderabad offers the right platform to build your skills and achieve your career goals.
0 notes
Text
How to Become a Software Engineer: A Full Guide
Software engineering is a rewarding and in-demand career that involves designing, developing, testing, and maintaining software systems. Whether you're a fresh graduate or looking for a career change, this guide will provide you with a roadmap to becoming a software engineer.
1. Foundational Knowledge
A strong foundation is crucial for any aspiring software engineer. Here's what you need to focus on:
Programming Fundamentals: Start with a beginner-friendly language like Python, JavaScript, or Java. Understand the basic concepts such as variables, data types, control structures, and object-oriented programming (OOP).
Data Structures and Algorithms: Learn how data is organized and manipulated. This includes arrays, linked lists, trees, graphs, and common algorithms like sorting and searching.
Operating Systems: Gain a basic understanding of how operating systems work, including memory management, processes, and file systems.
Databases: Learn how to design and manage databases using SQL or NoSQL.
Version Control: Familiarize yourself with Git for tracking changes in your code and collaborating with others.
2. Choose Your Path
Software engineering offers various specializations. Here are a few popular ones:
Web Development:
Frontend: Focuses on the user interface and user experience using HTML, CSS, and JavaScript frameworks like React or Angular.
Backend: Focuses on server-side logic, databases, and APIs using languages like Python, Java, or Node.js.
Full-Stack: Works on both frontend and backend development.
Mobile App Development: Develop applications for mobile devices using languages like Swift (iOS) or Java/Kotlin (Android).
Data Science: Focuses on extracting insights from data using statistical analysis, machine learning, and programming languages like Python or R.
Machine Learning: Develop algorithms and models that enable computers to learn from data.
3. Education and Learning Resources
Formal Education: A bachelor's degree in computer science or software engineering provides a comprehensive foundation.
Online Courses and Bootcamps: Platforms like Coursera, Udacity, edX, and freeCodeCamp offer structured learning paths and certifications.
Self-Learning: Utilize books, tutorials, and documentation to learn at your own pace.
4. Build Projects
Practical experience is essential for becoming a software engineer.
Personal Projects: Create your own applications or websites to showcase your skills.
Open Source Contributions: Contribute to existing projects on platforms like GitHub to collaborate with other developers and gain real-world experience.
Internships: Seek internships to gain professional experience and learn from industry experts.
5. Build a Portfolio
A portfolio is a collection of your projects and accomplishments that demonstrates your skills to potential employers.
Showcase Your Best Work: Include a variety of projects that highlight your technical abilities and problem-solving skills.
Provide Context: For each project, explain the problem you solved, the technologies you used, and the outcome.
Use a Professional Platform: Create a website or use platforms like GitHub Pages to host your portfolio.
6. Networking and Job Search
Networking: Attend industry events, join online communities, and connect with other software engineers on LinkedIn.
Job Search: Utilize online job boards, company websites, and networking to find job opportunities.
Interview Preparation: Practice coding challenges, review data structures and algorithms, and prepare for behavioral questions.
7. Continuous Learning
The field of software engineering is constantly evolving, so continuous learning is crucial.
Stay Updated: Follow industry blogs, attend conferences, and learn new technologies.
Explore New Areas: Be open to learning new programming languages, frameworks, and tools.
Seek Mentorship: Find a mentor who can provide guidance and support throughout your career.
Becoming a software engineer requires dedication, perseverance, and a passion for learning. By following this guide and continuously building your skills and knowledge, you can embark on a successful career in this dynamic and rewarding field.
0 notes
Text
Hands-On Data Science: Practical Steps for Aspiring Data Scientists
Embarking on the journey to study data science may initially seem like a complex and challenging task, but with a strategic approach, it can become a rewarding and accessible endeavor. Choosing the Best Data Science Institute can further accelerate your journey into this thriving industry. Let's explore a roadmap that can make your data science learning experience smoother and more manageable, breaking down the process into actionable steps.
1. Start with the Basics: Lay a Solid Foundation in Mathematics and Statistics
Commence your data science journey by establishing a robust foundation in the essentials of mathematics and statistics. Grasp fundamental concepts such as linear algebra and probability, which serve as the bedrock for advanced data science algorithms.
2. Learn a Programming Language: Begin Your Coding Journey with Python or R
Acquire proficiency in a programming language widely used in data science, such as Python or R. These languages are renowned for their user-friendliness and come equipped with extensive libraries and resources tailored for data science enthusiasts.
3. Explore Online Learning Platforms: Enroll in Accessible and Structured Courses
Embark on your learning adventure by enrolling in online courses specifically designed for beginners. Platforms like Coursera, edX, and ACTE Technologies offer a plethora of courses crafted by top universities and industry experts, covering fundamental topics like "Introduction to Data Science."
4. Hands-On Projects: Apply Theoretical Knowledge Through Real-world Applications
Translate theoretical knowledge into practical skills through hands-on projects. Platforms like Kaggle provide datasets and challenges that allow you to apply and implement what you've learned, solidifying your understanding through real-world applications.
5. Utilize Data Science Libraries: Master Essential Tools
Familiarize yourself with popular data science libraries in Python, such as Pandas, NumPy, and Scikit-Learn. These libraries simplify complex tasks and are widely adopted in the industry, making them indispensable tools in your data science toolkit.
6. Read Widely: Supplement Learning with In-Depth Resources
Enhance your online learning by delving into books on data science. Resources like "The Data Science Handbook" and "Python for Data Analysis" offer valuable insights into best practices and real-world applications. Follow reputable data science blogs to stay informed on emerging industry trends.
7. Engage with the Community: Join Forums and Discussions to Foster Connections
Immerse yourself in the vibrant data science community through platforms like Stack Overflow and Reddit. Actively participate in discussions, pose questions, and learn from the experiences of fellow enthusiasts. Networking is a valuable component of the learning process, offering diverse perspectives and insights.
8. Specialize Based on Interest: Explore and Deepen Your Understanding
As you advance in your studies, explore different areas within data science based on your interests. Whether it's machine learning, data engineering, or natural language processing, find a niche that resonates with your passion and curiosity.
9. Continuous Learning: Cultivate a Lifelong Learning Mindset
Recognize that data science is an ever-evolving field. Cultivate a mindset of continuous learning. Stay curious, explore advanced topics, and keep yourself updated on the latest industry developments to remain at the forefront of the field.
10. Practice Regularly: Consistency is Key to Mastery
Consistency is paramount in mastering data science. Dedicate regular time to your studies, practice coding, and engage in projects consistently. Building a habit ensures steady progress and reinforces your skills over time, enabling you to tackle increasingly complex challenges.
In conclusion, mastering data science is a journey that involves a combination of theoretical understanding, practical application, and a commitment to continuous learning. By following this roadmap and breaking down the learning process into manageable steps, you can navigate the world of data science with confidence and ease. Remember that the key to success lies not only in the destination but in the learning and growth that happens along the way. Choosing the best Data Science Courses in Chennai is a crucial step in acquiring the necessary expertise for a successful career in the evolving landscape of data science.
2 notes
·
View notes
Text
Back-End Development: A Complete Guide for Beginners in 2025
When you visit a website, everything you see—the layout, colors, text, and buttons—is the front end. But what happens when you log in, fill out a form, or make a payment? That’s where the back-end development magic begins.
In this complete guide, we’ll explore what back-end development is, why it’s crucial for the web, what technologies and skills you need, and how you can build a thriving career in this dynamic field. Whether you're a curious beginner or someone switching careers, this article has everything you need to know.
🚀 What is Back-End Development?
Back-end development refers to the server-side part of web development. It's everything that happens behind the scenes to make a website or app function properly—like servers, databases, application logic, and APIs.
Back-end development is all about how a website works rather than how it looks.
For example:
When you submit a login form, the back end checks your credentials in the database.
When you place an order online, the back end processes the order and stores the transaction.
⚙️ How Does Back-End Development Work?
The back end interacts with three key components:
Server – The machine that handles requests.
Database – Where data like user info and product listings are stored.
Application – The logic that ties it all together.
Here’s a simplified flow:
User clicks a button (front-end)
Front-end sends a request to the server
Back-end processes the request
Data is fetched from or saved to the database
Server sends a response back to the front-end
🧰 Core Technologies in Back-End Development
To become a back-end developer, you’ll need to learn these foundational tools and languages:
1. Programming Languages
LanguageUse CaseJavaScript (Node.js)Scalable server-side appsPythonFast prototyping, AI, APIsPHPWordPress and server scriptingRubyElegant, readable server-side codeJavaEnterprise-grade backend systemsC# (.NET)Enterprise, Windows-based applications
2. Databases
TypeExamplesRelationalMySQL, PostgreSQL, MS SQL ServerNoSQLMongoDB, CouchDB, Firebase
3. Frameworks
LanguageFrameworksJavaScriptExpress.js, Nest.jsPythonDjango, FlaskPHPLaravelRubyRuby on Rails
🌐 Back-End vs Front-End Development
FeatureFront-EndBack-EndFocusUser interface (UI/UX)Server logic and databaseLanguagesHTML, CSS, JSJS (Node), Python, PHP, JavaRuns OnBrowserServerPrimary ConcernDesign, interactivityLogic, data management, securityPopular ToolsReact, Vue, BootstrapDjango, Express.js, PostgreSQL
🧑💻 Roles & Responsibilities of a Back-End Developer
What does a back-end developer do?
Build APIs and server-side logic
Design and maintain databases
Secure user data and handle authentication
Ensure scalability and performance
Collaborate with front-end developers and DevOps teams
🛡️ Back-End and Security
Security is a core responsibility in back-end development.
Key areas include:
Data encryption
Secure APIs
Password hashing (bcrypt, Argon2)
Input validation
Authorization & Authentication (OAuth, JWT, etc.)
🧱 APIs and RESTful Architecture
APIs (Application Programming Interfaces) are bridges between the front end and back end.
Back-end developers often design:
REST APIs using HTTP methods (GET, POST, PUT, DELETE)
GraphQL APIs for flexible data querying
WebSockets for real-time communication
🔗 Database Management and ORM
Databases are the heart of any application.
Back-end developers use SQL for structured queries and ORMs (Object Relational Mappers) like:
Sequelize (Node.js)
Prisma
SQLAlchemy (Python)
Eloquent (Laravel)
📦 Hosting and Deployment
Once the server code is ready, it needs to be hosted.
Popular options:
Cloud: AWS, Google Cloud, Azure
Containers: Docker, Kubernetes
Serverless: Vercel, Netlify, AWS Lambda
CI/CD pipelines like GitHub Actions, Jenkins, and GitLab CI automate deployments.
🧠 Learning Path: How to Become a Back-End Developer
Here’s a structured roadmap:
Master a Programming Language – Start with Python or JavaScript (Node.js)
Understand the Internet and HTTP
Learn Databases – Start with MySQL or MongoDB
Build REST APIs
Practice Authentication & Security
Work with Real Projects
Use Git and GitHub
Explore DevOps Basics
Build a Portfolio with back-end apps
Contribute to Open Source
📊 Salary Insights and Job Opportunities (2025)
Back-end development is one of the most in-demand tech skills in 2025.CountryEntry-LevelMid-LevelSeniorIndia₹5–8 LPA₹10–20 LPA₹25+ LPAUSA$65K–$85K$90K–$120K$130K+UK£30K–£50K£55K–£75K£80K+
Common Job Titles:
Back-End Developer
Full-Stack Developer
API Engineer
Server-Side Developer
Cloud Functions Developer
💬 Real Developer Reviews
⭐⭐⭐⭐⭐ “As a back-end developer, I love building things people don’t even realize they’re using. It’s like being a wizard behind the curtain.” — Neha R., Software Engineer
⭐⭐⭐⭐☆ “Python and Django made it easy to get started. The logic is so clean and powerful.” — Mike T., Backend Developer
⭐⭐⭐⭐⭐ “Every startup needs someone who can build scalable back ends. It’s a career with massive growth potential.” — Ritika D., API Architect
🧠 Best Learning Resources (Free & Paid)
Free Platforms:
freeCodeCamp
MDN Web Docs
The Odin Project
Paid Options:
Udemy
"Node.js: The Complete Guide"
"Python & Django Bootcamp"
Coursera
"Back-End Development by Meta"
edX
Scrimba
📌 FAQs About Back-End Development
Q1. Do I need a degree to become a back-end developer?
A: No. Many successful developers are self-taught. Bootcamps and real-world projects matter more than degrees.
Q2. Which is better: back-end or front-end?
A: It depends on your interests. If you enjoy logic, data, and server operations—back-end is for you.
Q3. Is Node.js good for back-end?
A: Yes. Node.js is fast, efficient, and widely used for scalable server-side applications.
Q4. How long does it take to become job-ready?
A: With consistent learning, you can become a back-end developer in 6–12 months.
Q5. What is full-stack development?
A: Full-stack developers handle both front-end and back-end tasks. They’re skilled in end-to-end development.
Q6. What are the best languages for back-end development?
A: Python, JavaScript (Node.js), PHP, Java, and C# are top choices in 2025.
✨ Final Thoughts: Is Back-End Development Right for You?
If you love building logic, handling real-world data, working with APIs, and ensuring applications run smoothly—back-end development might be your ideal career path.
It’s a high-demand, well-paying, and technically rewarding field with endless opportunities for growth, especially with cloud computing, AI, and big data booming.
Whether you dream of joining a tech giant or launching your own SaaS app, mastering back-end development opens the door to some of the most impactful tech roles of the future.
0 notes