#Regression model
Explore tagged Tumblr posts
Text
The Dangers of Linear Correlation
The dangers of linear correlation arise when oversimplifying relationships between variables, leading to flawed conclusions, misguided decisions, or hidden risks, see recent blog. Here’s a breakdown of key pitfalls: 1. Assuming Linearity in Nonlinear Relationships Risk: Linear correlation (e.g., Pearson’s r) quantifies only straight-line relationships. Many real-world relationships are…
#advanced analytics#assumption of continuity#causality#generalized correlation#linear correlation#regression model
0 notes
Text
Artificial intelligence based model for Automatic Real-Time and Non-Invasive Estimation of blood potassium level in pediatric patients by Hamid Mokhtari Torshizi1, Negar Omidi2 in Journal of Clinical Case Reports Medical Images and Health Sciences
1PhD student of Biomedical Engineering and Physics Department, School of Medicine, Shahid Beheshti University of Medical Sciences, Tehran, Iran 2Associate Professor of Cardiology, Department of Cardiology, School of Medicine, Tehran Heart Center, Tehran University of Medical Sciences, Tehran, Iran 3Rajaie Heart Center and Department of Pediatric Cardiology, School of Medicine, Iran University of Medical Sciences, Tehran, Iran 4Digestive Disease Research Center, Digestive Disease Research Institute, Tehran University of Medical Sciences, Tehran, Iran 5Associate professor, Department of Biomedical Engineering, School of Medicine, Shahid Beheshti University of Medical Sciences, Tehran, Iran.
*Corresponding author
Mohsen Ahmadi, MD , Associate professor, Department of Biomedical Engineering, School of Medicine, Shahid Beheshti University of Medical Sciences, Tehran, Iran.
DOI: 10.55920/JCRMHS.2023.05.001213
Abstract
Objective: During the last decade, heart disease has become the main cause of death worldwide. One of the main causes of mortality in pediatric patients, especially in the intensive care unit, is related to cardiac arrhythmia. An abnormal variation in blood electrolytes, such as potassium, contributes to mortality in pediatric patients. Continuous and real-time monitoring of potassium serum levels can prevent fatal arrhythmias but this is not currently practical. Our Real-Time and Non-Invasive ECG-related technique uses machine learning to estimate blood potassium with good accuracy.
Patients and Methods: Hospitalized patients in emergency department of the Rajai Cardiology and Medical Research Center and Tehran Heart Center were recruited from December 2021 to June 2022.The electrocardiographic(ECG) features of patients were evaluated. We defined 16 features for each signal and extracted them automatically. With the assistance of the correlation matrix, the dimension reduction operation was performed. Linear regression, polynomials, decision trees, random forests, and support vector machine(SVM) algorithms have been used to find the relationship between characteristics and serum potassium levels. Finally, we used a scatter plot and mean square error (MSE) to display the results.
Results: ECG of 428 patients was analyzed. Random Forest Regression algorithm has the best estimate with an MSE of 0.3.
Conclusion: Accurate estimation of serum potassium level based on ECG signals is possible. This can potentially be a useful tool in predicting serum potassium level in specific patients.
Keywords: Serum potassium level, Machine learning, Regression model, Non-Invasive Estimation
Introduction
As one of the main electrolytes, potassium plays an important role in cellular membrane potential variations, especially in the heart (1). Normal cardiac function depends on regular sequential cardiac myocyte depolarization and repolarization. Any disruption in this circle may lead to cardiac conduction disorders and severe arrhythmia. The manifestation of these changes in the ECG signal is usually related to potassium concentration measured as potassium blood level (2). Children with cardiac and kidney diseases are more susceptible to the effects of potassium changes (3). Factors such as acute systemic illness, injection of potassium for electrolyte balance, and drugs and medicine intake can cause acute changes in potassium blood levels.
It should be noted that 98% of K+ is intracellular (140 mEq/l), and 2% is extracellular (3.8 to 5.0 mEq/l) (4). Hypokalemia is the most common electrolyte imbalance in cardiac patients, delaying ventricular repolarization and downgrading conduction velocity, especially at the atrioventricular node. This can lead to various arrhythmias, such as sinus bradycardia and atrioventricular block (5, 6). Also, hypokalemia can increase atrial and ventricular ectopic pulses and enhance digoxin’s toxic effect (7).
Until today, K+ levels have been measured in blood serum or plasma (for example: (8-12)). Blood sampling in children is a harmful process especially when frequent sampling is required. In some ICU conditions this much blood sampling results in anemia or unwanted cyanosis or apnea in children with congenital heart diseases. Furthermore, this method is invasive, expensive, and requires blood samples and some time to get the test results. On the other hand, Serum K+ measurements require clotting before analysis. Therefore, an abnormal potassium level may be reported due to hemolysis which occurs if long waiting periods before the analysis takes place. This can yield miss corrected blood samples (13-15).
In the face of these challenges, we recently designed a method for serum potassium concentration quantification ([K+]) from ECG analysis. In the past, many studies have been done on the heart signal with the help of machine learning algorithms (for example: (16-21)). In this study, our [K+] estimator was validated and tested on a large group of patients. Potassium value extraction using a single lead would permit its use in wearable, wireless ECG patches and possibly in implantable loop recorders and cardiac implantable electronic devices (pacemakers and defibrillators)
Methods
The studied community
In Total 428 hospitalized patients in the Rajai Cardiology and Medical Research Center and Tehran Heart Center emergency department were recruited from December 2021 to June 2022. The electrocardiographic (ECG) features of patients were evaluated. The patient’s serum K level, ECG, and serum sample were taken within 2 hours of admission. Patients having a history of heart failure, end stage renal disease, bundle branch block, strain pattern in ECG, premature ventricular contraction, and digoxin use were excluded from the study. A specialist nurse took ECGs which were stored in files based on age and gender. Patients whose ECGs had noise or artifacts for any reason were excluded from the study too. Information about the eligible patients, including their basic demographic information, has been collected through interviews and questionnaires.
Feature Extraction
We recorded each patient’s heart signal over 2.8 seconds using a Saadat ECG machine (12 channels). To analyze the heart signals, the data was transferred from the device’s memory to an external memory. We then developed a program in Python language on the Windows platform that can extract the amplitude and time values of P, Q, R, S, and T (the sequence of steps is shown in Figure 1). In total, 16 features were calculated using these points.
RtoT variable was defined as: The difference between the amplitude of the T wave and R wave
Dimensional reduction
The cross-correlation matrix was used for dimensionality reduction. For every two signal features with a correlation greater than 0.7, one was removed .
Preprocessing
We used z-score standardization according to the following formula:
is a data point (x1, x2 … xn)
is the sample mean
S is the sample standard deviation
2-5 Regression
A Regression model is one of the most common types of supervised learning in Machine Learning. When evaluating the linear relationship between the ECG characteristics and potassium serum level, we used the Pearson Correlation Coefficient and evaluated the non-linear relationship using Decision Trees and Random Forest algorithms. Python version 3 was used for cardiac signal processing.
SVR, Decision Tree, Random Forest, Linear Regression, and Polynomial Regression algorithms were used to test the linear and non-linear relationship between characteristics and potassium serum level. Based on the results, the Random Forest algorithm has the best performance in this research.
Random Forest:
It is a group learning method that involves combining several trees. Each tree is trained, and several random features are sampled at each tree node. The average of the regression results from all the decision trees is assigned to the final decision.
Model Validation:
In this study, we used 20% of the population as a test sample. The estimated potassium level was calculated from the obtained ECG data using the corresponding patient-specific potassium prediction model developed during the training phase. To assess the accuracy, we calculated the mean absolute error, which is the mean absolute value of the difference between the estimated and measured potassium for each patient.
Results
Among the 463 patients who were admitted to the hospitals in 1 years, 35 patients were excluded from the study due to high noise and distortion of the heart signal. In our community, 56% were boys and 44% were girls. The potassium chart of these patients is shown in Figure 2. The results of the cross-correlation matrix of data .
Based on the information in Figure 3, we remove one of the two parameters that correlate more than 0.7. PR, Ps, PT, Twidth, QS, QR, QT, RS, RT, ST, and RtoT are variables that we use to teach regression methods. Table 1 shows the efficiency of each regression algorithm based on the MSE. As indicated in the table, the polynomial method has the lowest accuracy, and the random forest method has the highest measurement accuracy.
Considering that most of our studied patients have a potassium level between 4 and 4.5, we use the scatter diagram to get better feedback than regression methods. Figure 4, shows the scatter diagram for different approaches.
Another important goal of this study is to calculate the importance of each feature in determining the level of potassium. In Figure 5, the importance of each feature by the random forest algorithm is shown in percentage terms. Figure 6 shows how the decision tree algorithm yields decisions.
How to allocate blood serum potassium level based on the input characteristics in the decision tree algorithm
Discussion
Acute electrolyte disturbance especially hyperkalemia in pediatrics is life-threatening and requires prompt attention. As well as the correct determination of K level, the on-time result is also critical because many children with acute ill conditions need immediate medical attention. Blood sampling is a well-known reliable method for the evaluation of this electrolyte. Yet, evaluation of K level has always been desirable and attempts have been made for K level determination with high accuracy and sensitivity.
The effect of potassium on the ECG cardiac signal has been known for many years (22-24). So far, several studies, generally based on T-wave morphology, have been conducted to determine serum potassium levels (25-29). ECG markers, which are defined as a specific time interval or range of neural signals, are prone to noise (30). To solve this problem, many studies have investigated T wave morphology over a long period of time (31-33). Major challenges with these studies have been the definition of morphologies based on complex mathematical rules, the long time to calculate optimal parameters (several hours), and the low statistical population.
Another group of studies has investigated blood potassium based on ECG and using deep learning algorithms. In this class of studies, only hyperkalemia is detected at high potassium levels.
The purpose of this study is to design and validate an online and non-invasive potassium level extraction technique based on machine learning algorithm. We allocated 2.8 seconds to determine the serum level of potassium. This time interval ensures that several cardiac cycles are considered. Another strength of this study is the application of two stages of filtering to remove the effect of unwanted distortion on heart signal parameters. Therefore, if a part of the signal is distorted, the result of evaluating the potassium level is still reliable. Another advantage of this method is that only one lead is considered. This helps to commercialize the mentioned method and assess the potassium level remotely. Considering the high number of subjects in the studied population, it can be assumed that all T-wave morphologies were covered. We are developing our algorithm in such a way that we can analyze different types of ECG signals.
So far, attempts have been made to measure blood potassium levels using cardiac signals. Shakil Aslam and her colleagues conducted a study on 74 end-stage renal disease (ESRD) patients in 2002 (22). In their study, they tried to provide a linear relationship between T-wave amplitude or T-wave to R-wave ratio and the serum level of potassium in a patient's blood. In the same way that Szerlip et al. (34), could not provide a relationship between the T wave to R wave ratio and the level of serum potassium in the blood of individuals.
In 2018, with the introduction of two parameters, T-right slope, and T-amp, a group of researchers tried to discover the potassium serum level with ECG (35). By examining two parameters (T-amp and T-right slope) on five lead waveforms (V3, V4, V5, V6, and II), they found that T-wave-based features were not correlated with serum potassium level.
Omar Z. Yasin, MD, and her colleagues investigated the potassium serum level and heart signal of 21 dialysis patients (36). The average absolute error between estimated and blood potassium was 0.38- 0.32 mEq/L. The sample size of our study and the wide range of serum potassium levels are the strengths of our work compared to theirs.
John J. Dillon and his colleagues tried to quantify the amount of potassium using the cardiac signal and the serum level of potassium in the blood of 12 patients (29). They used the following variables for the regression operator in their work: The slope of the T wave down stroke (T right slope), the amplitude of the T wave (T amplitude), the center of gravity (COG) of the T wave (T COG), the ratio of the amplitude of the T wave to the amplitude of the R wave (T/R amplitude), and the center of gravity of the last 25% of the area under the T wave curve (T4 COG). However, they have recommended the use of the cardiac signal as a non-invasive method. Yet, due to the small sample size of their study, more research is required. In our study, we tried to overcome the weaknesses of previous studies by considering a large statistical population with a wide range of serum potassium levels, more features of the cardiac signal, and applying machine learning methods.
The high statistical community of this study has made it possible to examine all the clinical factors of the cardiac signal. Based on the results presented in Figures 5 and 6, the PT parameter is considered as the most important influencing factor in predicting the amount of potassium. This means that the combination of PQ, QRS, and ST intervals is influential. However, the second most important parameter in determining serum potassium levels is the difference in R and T wave amplitudes. This finding is similar to studies that have considered the slope of the T wave as an important parameter in the determination of serum potassium.
As shown in Figure 4, the linear and polynomial methods that were used in most studies do not have adequate predictive power. Although the Polynomial method has high accuracy in the training phase, it has the lowest accuracy in the test phase (Table 1). This is caused by the phenomenon of overfitting. The linear method also predicts all values in the range of 4 to 4.5 mmol. Although the decision tree method works well for wide potassium levels, it is not suitable for low potassium (under 4 mmol). Prediction of potassium level through the SVR method only works somewhat well in medium values. But the random forest method has solved the problem of the decision tree algorithm to a great extent, and has improved the detection of potassium in low amounts. Finally, it can be said that in this study, the random forest algorithm is more efficient than other algorithms.
Besides to potassium, other factors such as other electrolytes and the location of the leads affect the cardiac signal. Despite the presence of all the sources influencing the cardiac signal, we managed to calculate the serum potassium level with an average error of 0.3 in this study. Perhaps, in future studies, the effect of other electrolytes on ECG can be processed and by considering their effects on the cardiac signal, an increase in the accuracy of predicting blood potassium can be attained.
Conclusions
In conclusion, we defined a comprehensive noninvasive method for evaluating of k level in pediatrics based on ECG signal. This study may start a noninvasive portable method for the determination of K level by monitoring ECG signal results and fast measurement of k level changes in response to systemic conditions in acute ill adult and child groups.
#Serum potassium level#Machine learning#Regression model#Non-Invasive Estimation#Journal of Clinical Case Reports Medical Images and Health Sciences#jcrmhs
0 notes
Text

workin on a baby earth model for vr :3 !! not sure if she'll be public yet since its mostly for self comfort , agedre , and other reasons , but if i do decide to make her public once im done she'll be in my avi world :3
#vrc model#vrc avatar#vrchat#laes#laes earth#earth sams#sams earth#tsams#tsams earth#age regression#sfw agere#age dreaming#sfw agedre#idiot artz
237 notes
·
View notes
Text









---
Model Train Agere and Blue Stimboard
PT: Model Train Agere and Blue Stimboard
Made for: @cottondaycarestims (Ches)
For a wonderful mutual, whose luck I hope improves soon. I hope this helped you smile today. Take care of yourself. This stimboard brought back memories of me and my dad's model trains, so thank you for that.
Credits: x x x x x x x x x
#gilear's creations#stimboard#stimblr#visual stim#sfw age regression#stimmy#sfw agere#agere stimboard#model trains#model train stim#model train stimboard#blue stim#blue stimboard#blue#paci stim#agere stim#age regression#train stim#train stimboard#plush stim#plush stimboard#sfw interaction only#blue agere stimboard#blue agere stim
22 notes
·
View notes
Text
Playing with a blue matchbox truck
#text#autismposting#stim#my gifs#agere#agere stim#boyre stim#boyre#matchbox cars#toy stim#toy truck stim#blue stim#medium blue stim#truck stim#toycore stim#kidre stim#up close#irl hands tw#boy regression#boy regressor#agere community#sfw sgere#toys#toycore#toy truck#metal stim#model cars stim#pretend play stim#pretend play#clusters tw
22 notes
·
View notes
Note
I didnt mean my Denji and Adrien comparisons in a negative way btw . Mari could kill 10 people in the show and I would forgive her
GIVE ME A SECOND GIRLYPOP I HAVENT ANSWERED IT BECAUSE I HAVENT REDRAWN HIM OVER THE I WANNA TOUCH SOME BOOBS PANEL
#you're so right thoughhhhhhh. forever ago I drew the two of them together actually so maybe instead I can just redraw that#it's a genre of headcanons that i completely understand people for not touching whatsoever but i think the idea of adrien#being hypersexual in his later teens/early 20s is not super out of the blue given the specific kind of childhood abuse he both canonically#goes through and the abuse he could've reasonably gone through when extrapolating stuff from his environment + his modeling career#like there's no question that his views on love and affection are incredibly disturbingly warped and after years of repression it would onl#be reasonable that he explodes like a power keg and seemingly “regresses” into having really shallow libertine desires etc etc#hence why in environments where he isn't being actively repressed i tend to write him as a bit of a hedonist anyways. blueblood is an#example of this#wissym answers
14 notes
·
View notes
Text


je silly is up !!! is in my avi world for all platforms :33 have fun wit da baby !!!! (still a wip , more things will be added later like an arm/sleeve toggle :3)
if i get more time later on then i may try sun/moon/etc vers , buuut no promises :P
link for the avi !!
#vrc model#vrc avatar#vrchat#laes#laes earth#tsams earth#sams earth#earth sams#tsams#age dreaming#sfw agere#sfw agedre#age regression#idiot artz
24 notes
·
View notes
Text
🪽´ˎ˗ Yuffie Kisaragi and Sephiroth (Final Fantasy) agere icons!!
╰➝ Credits... trans regressor flag
╰➝ Requested by... @soopiroth
#im not very familiar with ff so im not sure if i chose the best pictures?#i noticed you seem to prefer the 2d anime style so i went with that (also i think it looks way better than the 3d models)#sfw agere#agere sfw#agere blog#sfw agere blog#agere#age regression#age regressor#sfw regression#sfw regressor#agere icons#agere edit#agere pfp#trans agere#trans regressor#agere fandom#fandom agere#blankiefort ★´ˎ˗#kitty scribbles ★´ˎ˗
9 notes
·
View notes
Text
yes the rumours are true, i am 3 apples tall



#i'm dani pedrosa short :(#i love drawing myself#there aint no better model but ME#also i'm going through art regression but idc anymore#i should start posting on my insta art account again...i haven't put anything there since like september lol#edgar art
2 notes
·
View notes
Text
When you dont have the money for toys so you make your own /silly

Im modelling some car and truck toys that have connectable trailers and things! I have a 3d printer, so im using that to my advantage!
#age regression#agere#age regressor#agere blog#agedre#agere community#sfw littlespace#boyre#boy regressor#boy regression#car toys#kids toys#3d model
5 notes
·
View notes
Text
#this is like a regressive tax. but not applying to the existing account is sort of meaningless#this is getting closer to how I think the model should be#however I won’t talk about my model and give clues to Elon musk.#taiwantalk
2 notes
·
View notes
Text
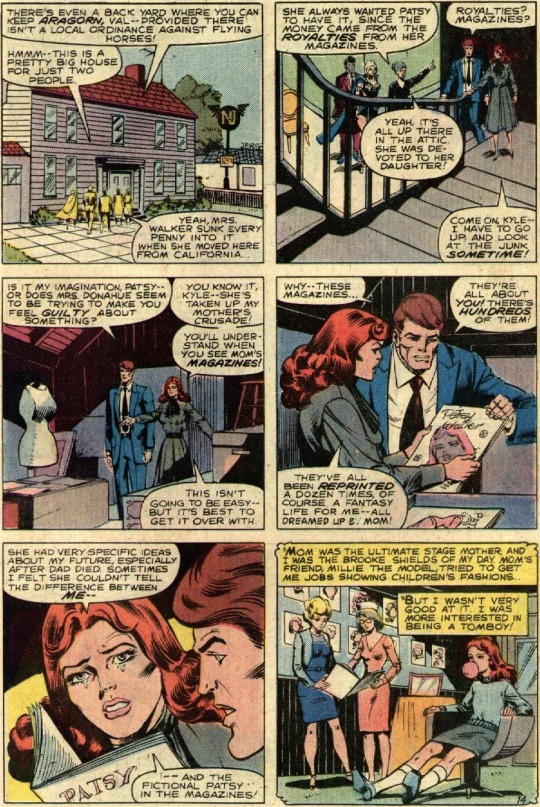
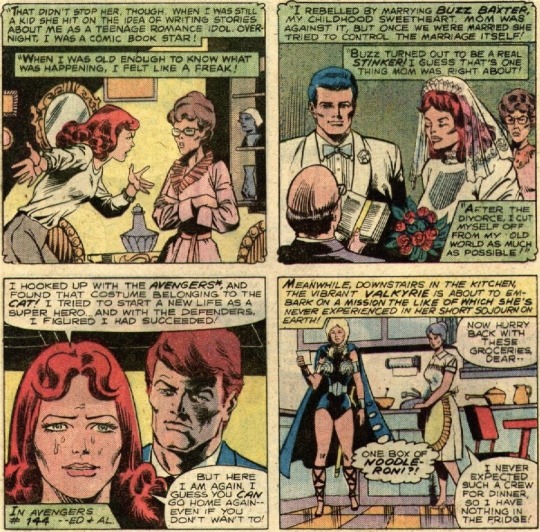
The Defenders (1972) #89
#I haven’t read any of the old Patsy teen girl comics#I didn’t think that there was going to be any relation between that Patsy and this Patsy#but I think that this is an interesting way to approach it#so that that image does exist in-universe for Patsy#and it’s a fictional concept that she’s been pressured into conforming to#I wonder how this would come across to Val#obviously her feminism isn’t very fleshed out#but I assume that those teen girl comics were pretty regressive/promoted something very specific#also I think the way that Patsy’s previous modeling career has been portrayed in this book as not that it was uncomfortable for her#because it was what her mom wanted or because she was a tomboy at heart#but because she wasn’t fulfilled by it#and then found that she really enjoyed being a superhero#she hasn’t been notably not feminine#she also hasn’t been uncomfortable with romance#in fact she’s been having some fun flirting#but I don’t think she’s interested in like having a traditional marriage at this point in her life#like her ex that she divorced seemed to have wanted from her#marvel#patsy walker#kyle richmond#my posts#comic panels
2 notes
·
View notes
Text
I love sketching people after a few years and suddenly I can do arms and hands
Like it's not perfect, but for some reason I can now understand "how" it connects? I don't remember doing much studying or practice 😅
Now I just need to get into the habit of drawing more, so I can do lore comics ✨️ And perhaps more Freddie Stray Gods Art (I miss her and the tags are mostly empty)
#maybe all the 3D modeling helped?#last time I drew it was Freddie from Stray Gods#everyone say thank you to Freddie#that was like several months ago?#I am drawing on my supernote#so maybe that's also a factor#it's weird regressing and progressing in drawing#happy I can sorta draw again though#post
0 notes
Text
Regression & Classification with MNIST
A Comprehensive Guide to Machine Learning: Regression and Classification with the MNIST Dataset A Comprehensive Guide to Machine Learning: Regression and Classification with the MNIST Dataset Introduction to Supervised Learning: Regression and Classification In the realm of machine learning, supervised learning involves training a model on a labeled dataset, which means the dataset includes both…
#artificial intelligence#classification#deep learning#evaluate model#machine learning#mnist#regression
1 note
·
View note
Text
Been feeling drained for the past week and been indulging in the idea of pet regression and kind of projecting the idea onto Zoan DF!Ray a bit so uhhhh Some headcanons for situations where Ray gets stuck/slips into beast mode after stressful situations :}
all the Strawhats have nicknames for puppy!Ray those ranging from your basic good pup, little pup, dewdrop, dumpling, little sun, and sunshine, but some of my personal favorites are Franky and Zoro’s just “dog” in the most affectionate way possible (think in the tone of ‘that’ll do pig that’ll do’)
It’s not unheard of after a particularly draining or stressful day Ray will almost Collapse into beast mode. When this happens the game plan is usually to coax them to go lay with Zoro or Sanji
When stuck in beast mode the crew tries to keep Ray entertained and comforted in their own ways. Sanji sneaks them scraps from cooking, Zoro takes extra steps to nap in areas Ray can find and join him, Luffy doesn’t mind the extra playmate, Robin always makes sure they’re taken care of and can properly decompress
Luffy, Zoro, and Nami have the most experience with puppy!Ray but the whole crew eventually is familiar with what happened and all are equipped to help and calm Ray.
Puppy!Ray’s prime napping/hang out spots they gravitate to are wherever Zoro is, by the door in the kitchen, in Franky’s workshop, and on the deck with Nami and Robin
Puppy!Ray isn't very energetic and tends to just want to relax and not do too much if possible but with people like Usopp, Luffy, and Chopper on the ship they're sure they keep fit and happy. Sometimes if they're docked on an island when they get suck in puppy mode it's not unheard of for the crew to take a few days to unwind. Sometimes they go on picnics or beach days and with Ray being in beast mode it definitely doesn't stop the navy from thinking the Strawhats just have two pets lol.
#ollie musings#identity#s/i stuff#🌸s.wildflower#🐕au.inu inu no mi: shepherd model#age regression#🏝️come aboard.fam
1 note
·
View note