#TensorRT
Explore tagged Tumblr posts
Text
Rekor Uses NVIDIA AI Technology For Traffic Management
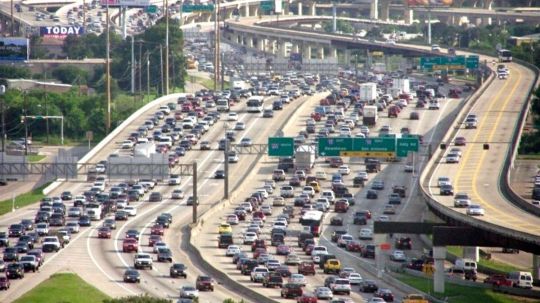
Rekor Uses NVIDIA Technology for Traffic Relief and Roadway Safety as Texas Takes in More Residents.
For Texas and Philadelphia highways, the company is using AI-driven analytics utilizing NVIDIA AI, Metropolis, and Jetson, which might lower fatalities and enhance quality of life.
Jobs, comedy clubs, music venues, barbecues, and more are all attracting visitors to Austin. Traffic congestion, however, are a major city blues that have come with this growth.
Due to the surge of new inhabitants moving to Austin, Rekor, which provides traffic management and public safety analytics, gets a direct view of the growing traffic. To assist alleviate the highway issues, Rekor collaborates with the Texas Department of Transportation, which is working on a $7 billion initiative to remedy this.
Based in Columbia, Maryland, Rekor has been using NVIDIA Jetson Xavier NX modules for edge AI and NVIDIA Metropolis for real-time video understanding in Texas, Florida, Philadelphia, Georgia, Nevada, Oklahoma, and many other U.S. locations, as well as Israel and other countries.
Metropolis is a vision AI application framework for creating smart infrastructure. Its development tools include the NVIDIA DeepStream SDK, TAO Toolkit, TensorRT, and NGC catalog pretrained models. The tiny, powerful, and energy-efficient NVIDIA Jetson accelerated computing platform is ideal for embedded and robotics applications.
Rekor’s initiatives in Texas and Philadelphia to use AI to improve road management are the most recent chapter in a long saga of traffic management and safety.
Reducing Rubbernecking, Pileups, Fatalities and Jams
Rekor Command and Rekor Discover are the two primary products that Rekor sells. Traffic control centers can quickly identify traffic incidents and areas of concern using Command, an AI-driven software. It provides real-time situational awareness and notifications to transportation authorities, enabling them to maintain safer and less congested municipal roads.
Utilizing Rekor’s edge technology, discover completely automates the collection of thorough vehicle and traffic data and offers strong traffic analytics that transform road data into quantifiable, trustworthy traffic information. Departments of transportation may better plan and carry out their next city-building projects by using Rekor Discover, which gives them a comprehensive picture of how cars travel on roads and the effect they have.
Command has been spread around Austin by the corporation to assist in problem detection, incident analysis, and real-time response to traffic activities.
Rekor Command receives a variety of data sources, including weather, linked vehicle information, traffic camera video, construction updates, and data from third parties. After that, it makes links and reveals abnormalities, such as a roadside incident, using AI. Traffic management centers receive the data in processes for evaluation, verification, and reaction.
As part of the NVIDIA AI Enterprise software platform, Rekor is embracing NVIDIA’s full-stack accelerated computing for roadway intelligence and investing heavily in NVIDIA AI and NVIDIA AI Blueprints, reference workflows for generative AI use cases constructed with NVIDIA NIM microservices. NVIDIA NIM is a collection of user-friendly inference microservices designed to speed up foundation model installations on any cloud or data center while maintaining data security.
Rekor is developing AI agents for municipal services, namely in areas like traffic control, public safety, and infrastructure optimization, leveraging the NVIDIA AI Blueprint for video search and summarization. In order to enable a variety of interactive visual AI agents that can extract complicated behaviors from vast amounts of live or recorded video, NVIDIA has revealed a new AI blueprint for video search and summarization.
Philadelphia Monitors Roads, EV Charger Needs, Pollution
The Philadelphia Industrial Development Corporation (PIDC), which oversees the Philadelphia Navy Yard, a famous tourist destination, has difficulties managing the roads and compiling information on new constructions. According to a $6 billion rehabilitation proposal, the Navy Yard property will bring thousands of inhabitants and 12,000 jobs with over 150 firms and 15,000 workers on 1,200 acres.
PIDC sought to raise awareness of how road closures and construction projects influence mobility and how to improve mobility during major events and projects. PIDC also sought to improve the Navy Yard’s capacity to measure the effects of speed-mitigating devices placed across dangerous sections of road and comprehend the number and flow of car carriers or other heavy vehicles.
In order to handle any fluctuations in traffic, Discover offered PIDC information about further infrastructure initiatives that must be implemented.
By knowing how many electric cars are coming into and going out of the Navy Yard, PIDC can make informed decisions about future locations for the installation of EV charging stations. Navy Yard can better plan possible locations for EV charge station deployment in the future by using Rekor Discover, which gathers data from Rekor’s edge systems which are constructed with NVIDIA Jetson Xavier NX modules for powerful edge processing and AI to understand the number of EVs and where they’re entering and departing.
By examining data supplied by the AI platform, Rekor Discover allowed PIDC planners to produce a hotspot map of EV traffic. The solution uses Jetson and NVIDIA’s DeepStream data pipeline for real-time traffic analysis. To further improve LLM capabilities, it makes advantage of NVIDIA Triton Inference Server.
The PIDC sought to reduce property damage and address public safety concerns about crashes and speeding. When average speeds are higher than what is recommended on certain road segments, traffic calming measures are being implemented using speed insights.
NVIDIA Jetson Xavier NX to Monitor Pollution in Real Time
Rekor’s vehicle identification models, which were powered by NVIDIA Jetson Xavier NX modules, were able to follow pollution to its origins, moving it one step closer to mitigation than the conventional method of using satellite data to attempt to comprehend its placements.
In the future, Rekor is investigating the potential applications of NVIDIA Omniverse for the creation of digital twins to model traffic reduction using various techniques. Omniverse is a platform for creating OpenUSD applications for generative physical AI and industrial digitization.
Creating digital twins for towns using Omniverse has significant ramifications for lowering traffic, pollution, and traffic fatalities all of which Rekor views as being very advantageous for its clients.
Read more on Govindhtech.com
#Rekor#NVIDIATechnology#TensorRT#AIapplication#NVIDIANIM#NVIDIANIMmicroservices#generativeAI#NVIDIAAIBlueprint#NVIDIAOmniverse#News#Technews#Technology#technologynews#Technologytrends#govindhtech
0 notes
Text
sentences that should be illegal to say to a girl:
This TensorFlow binary is optimized to use available CPU instructions in performance-critical operations
TF-TRT Warning: Could not find TensorRT
Cannot dlopen some GPU libraries
49 notes
·
View notes
Text
ok i want to learn - Loss Functions in LLMs (Cross-entropy loss, KL Divergence for distillation) Gradient Accumulation and Mixed Precision Training Masked Language Modeling (MLM) vs. Causal Language Modeling (CLM) Learning Rate Schedules (Warmup, cosine decay) Regularization Techniques (Dropout, weight decay) Batch Normalization vs. Layer Normalization Low-Rank Adaptation (LoRA) Prompt Engineering (Zero-shot, few-shot learning, chain-of-thought) Adapters and Prefix Tuning Parameter-Efficient Fine-Tuning (PEFT) Attention Head Interpretability Sparse Attention Mechanisms (BigBird, Longformer) Reinforcement Learning with Human Feedback (RLHF) Knowledge Distillation in LLMs Model Compression Techniques (Quantization, pruning) Model Distillation for Production Inference Optimization (ONNX, TensorRT)
4 notes
·
View notes
Text
MediaTek and NVIDIA Team up for Automotive AI
With more and more auto manufacturers pushing for smarter vehicles, there's been a considerably growing demand for more powerful smart automotive platforms, going beyond the simple act of pairing your smartphone with your car's Bluetooth console (think 'K.I.T.T.' from Knight Rider). It's no surprise then that we've seen an uptick of specially-designed hardware and software solutions that provide entertainment and navigation features for drivers and passengers alike. With that being said, MediaTek's push towards putting more AI tech into everyday consumer products has certainly yielded some very interesting results, and the company's newly-announced collaboration with PC gaming giant NVIDIA aims to do the same, at least in terms of automotive applications. More specifically, the mobile chip manufacturer formally announced that it has entered into a partnership with NVIDIA to develop new AI-powered software for vehicles, with the goal of creating a "smart cabin" for drivers and passengers. This collaboration will enable MediaTek to develop automotive SoCs, which will in turn integrate a new NVIDIA GPU "chiplet" with support for NVIDIA AI and graphics IP. Interestingly, these chiplets will be connected by specially-developed interconnect technology, at least according to MediaTek. Rick Tsai, Vice Chairman and CEO of MediaTek states: “NVIDIA is a world-renowned pioneer and industry leader in AI and computing. With this partnership,our collaborative vision is to provide a global one-stop shop for the automotive industry, designing thenext generation of intelligent, always-connected vehicles. Through this special collaboration with NVIDIA, we will together be able to offer a truly unique platform for the compute intensive, software-defined vehicle of the future.” NVIDIA CEO Jensen Huang says this combination of MediaTek and NVIDIA hardware will "enable new user experiences, enhanced safety and new connected services for all vehicle segments, from luxury to mainstream.” MediaTek adds that its smart cabin solutions will run NVIDIA DRIVE OS, DRIVE IX, CUDA and TensorRT software technologies. This then allows consumers to experience a full range of AI cabin and cockpit functionality with integrated AI, safety, and security features as well. While NVIDIA is more known to consumers as a PC and gaming-centric brand, the company does put a considerable amount of investment towards the development and production of AI and IoT (internet of things) technology, in addition to its powerful GPUs and processors. The Taiwanese company further states that by allowing MediaTek to tap into NVIDIA’s core expertise in AI, cloud, graphics technology, software and pairing with NVIDIA ADAS solutions, we can expect to see further improvement to the capabilities of the Dimensity Auto platform, MediaTek's flagship automotive software product. Dimensity Auto is designed for vehicles with support for compatible smart features. With all that being said, it should be interesting to see how both companies approach this new partnership, both on hardware and business fronts. Read the full article
3 notes
·
View notes
Text
Elmalo, let's commit to that direction. We'll start with a robust Sensor Fusion Layer Prototype that forms the nervous system of Iron Spine, enabling tangible, live data connectivity from the field into the AI's processing core. Below is a detailed technical blueprint that outlines the approach, components, and future integrability with your Empathic AI Core.
1. Hardware Selection
Edge Devices:
Primary Platform: NVIDIA Jetson AGX Xavier or Nano for on-site processing. Their GPU acceleration is perfect for real-time preprocessing and running early fusion algorithms.
Supplementary Controllers: Raspberry Pi Compute Modules or Arduino-based microcontrollers to gather data from specific sensors when cost or miniaturization is critical.
Sensor Modalities:
Environmental Sensors: Radiation detectors, pressure sensors, temperature/humidity sensors—critical for extreme environments (space, deep sea, underground).
Motion & Optical Sensors: Insect-inspired motion sensors, high-resolution cameras, and inertial measurement units (IMUs) to capture detailed movement and orientation.
Acoustic & RF Sensors: Microphones, sonar, and RF sensors for detecting vibrational, audio, or electromagnetic signals.
2. Software Stack and Data Flow Pipeline
Data Ingestion:
Frameworks: Utilize Apache Kafka or Apache NiFi to build a robust, scalable data pipeline that can handle streaming sensor data in real time.
Protocol: MQTT or LoRaWAN can serve as the communication backbone in environments where connectivity is intermittent or bandwidth-constrained.
Data Preprocessing & Filtering:
Edge Analytics: Develop tailored algorithms that run on your edge devices—leveraging NVIDIA’s TensorRT for accelerated inference—to filter raw inputs and perform preliminary sensor fusion.
Fusion Algorithms: Employ Kalman or Particle Filters to synthesize multiple sensor streams into actionable readings.
Data Abstraction Layer:
API Endpoints: Create modular interfaces that transform fused sensor data into abstracted, standardized feeds for higher-level consumption by the AI core later.
Middleware: Consider microservices that handle data routing, error correction, and redundancy mechanisms to ensure data integrity under harsh conditions.
3. Infrastructure Deployment Map
4. Future Hooks for Empathic AI Core Integration
API-Driven Design: The sensor fusion module will produce standardized, real-time data feeds. These endpoints will act as the bridge to plug in your Empathic AI Core whenever you’re ready to evolve the “soul” of Iron Spine.
Modular Data Abstraction: Build abstraction layers that allow easy mapping of raw sensor data into higher-level representations—ideal for feeding into predictive, decision-making models later.
Feedback Mechanisms: Implement logging and event-based triggers from the sensor fusion system to continuously improve both hardware and AI components based on real-world performance and environmental nuance.
5. Roadmap and Next Steps
Design & Prototype:
Define the hardware specifications for edge devices and sensor modules.
Develop a small-scale sensor hub integrating a few key sensor types (e.g., motion + environmental).
Data Pipeline Setup:
Set up your data ingestion framework (e.g., Apache Kafka cluster).
Prototype and evaluate basic preprocessing and fusion algorithms on your chosen edge device.
Field Testing:
Deploy the prototype in a controlled environment similar to your target extremes (e.g., a pressure chamber, simulated low-gravity environment).
Refine data accuracy and real-time performance based on initial feedback.
Integration Preparation:
Build standardized API interfaces for future connection with the Empathic AI Core.
Document system architecture to ensure a smooth handoff between the hardware-first and AI-core teams.
Elmalo, this blueprint establishes a tangible, modular system that grounds Iron Spine in reality. It not only demonstrates your vision but also builds the foundational “nervous system” that your emergent, empathic AI will later use to perceive and interact with its environment.
Does this detailed roadmap align with your vision? Would you like to dive further into any individual section—perhaps starting with hardware specifications, software configuration, or the integration strategy for the future AI core?
0 notes
Link
#AIhardware#energyefficiency#enterpriseAI#GenerativeAI#GPUoptimization#ICLR2024#machinelearning#NVIDIA
0 notes
Text
Step-by-Step Breakdown of AI Video Analytics Software Development: Tools, Frameworks, and Best Practices for Scalable Deployment
AI Video Analytics is revolutionizing how businesses analyze visual data. From enhancing security systems to optimizing retail experiences and managing traffic, AI-powered video analytics software has become a game-changer. But how exactly is such a solution developed? Let’s break it down step by step—covering the tools, frameworks, and best practices that go into building scalable AI video analytics software.
Introduction: The Rise of AI in Video Analytics
The explosion of video data—from surveillance cameras to drones and smart cities—has outpaced human capabilities to monitor and interpret visual content in real-time. This is where AI Video Analytics Software Development steps in. Using computer vision, machine learning, and deep neural networks, these systems analyze live or recorded video streams to detect events, recognize patterns, and trigger automated responses.
Step 1: Define the Use Case and Scope
Every AI video analytics solution starts with a clear business goal. Common use cases include:
Real-time threat detection in surveillance
Customer behavior analysis in retail
Traffic management in smart cities
Industrial safety monitoring
License plate recognition
Key Deliverables:
Problem statement
Target environment (edge, cloud, or hybrid)
Required analytics (object detection, tracking, counting, etc.)
Step 2: Data Collection and Annotation
AI models require massive amounts of high-quality, annotated video data. Without clean data, the model's accuracy will suffer.
Tools for Data Collection:
Surveillance cameras
Drones
Mobile apps and edge devices
Tools for Annotation:
CVAT (Computer Vision Annotation Tool)
Labelbox
Supervisely
Tip: Use diverse datasets (different lighting, angles, environments) to improve model generalization.
Step 3: Model Selection and Training
This is where the real AI work begins. The model learns to recognize specific objects, actions, or anomalies.
Popular AI Models for Video Analytics:
YOLOv8 (You Only Look Once)
OpenPose (for human activity recognition)
DeepSORT (for multi-object tracking)
3D CNNs for spatiotemporal activity analysis
Frameworks:
TensorFlow
PyTorch
OpenCV (for pre/post-processing)
ONNX (for interoperability)
Best Practice: Start with pre-trained models and fine-tune them on your domain-specific dataset to save time and improve accuracy.
Step 4: Edge vs. Cloud Deployment Strategy
AI video analytics can run on the cloud, on-premises, or at the edge depending on latency, bandwidth, and privacy needs.
Cloud:
Scalable and easier to manage
Good for post-event analysis
Edge:
Low latency
Ideal for real-time alerts and privacy-sensitive applications
Hybrid:
Initial processing on edge devices, deeper analysis in the cloud
Popular Platforms:
NVIDIA Jetson for edge
AWS Panorama
Azure Video Indexer
Google Cloud Video AI
Step 5: Real-Time Inference Pipeline Design
The pipeline architecture must handle:
Video stream ingestion
Frame extraction
Model inference
Alert/visualization output
Tools & Libraries:
GStreamer for video streaming
FFmpeg for frame manipulation
Flask/FastAPI for inference APIs
Kafka/MQTT for real-time event streaming
Pro Tip: Use GPU acceleration with TensorRT or OpenVINO for faster inference speeds.
Step 6: Integration with Dashboards and APIs
To make insights actionable, integrate the AI system with:
Web-based dashboards (using React, Plotly, or Grafana)
REST or gRPC APIs for external system communication
Notification systems (SMS, email, Slack, etc.)
Best Practice: Create role-based dashboards to manage permissions and customize views for operations, IT, or security teams.
Step 7: Monitoring and Maintenance
Deploying AI models is not a one-time task. Performance should be monitored continuously.
Key Metrics:
Accuracy (Precision, Recall)
Latency
False Positive/Negative rate
Frame per second (FPS)
Tools:
Prometheus + Grafana (for monitoring)
MLflow or Weights & Biases (for model versioning and experiment tracking)
Step 8: Security, Privacy & Compliance
Video data is sensitive, so it’s vital to address:
GDPR/CCPA compliance
Video redaction (blurring faces/license plates)
Secure data transmission (TLS/SSL)
Pro Tip: Use anonymization techniques and role-based access control (RBAC) in your application.
Step 9: Scaling the Solution
As more video feeds and locations are added, the architecture should scale seamlessly.
Scaling Strategies:
Containerization (Docker)
Orchestration (Kubernetes)
Auto-scaling with cloud platforms
Microservices-based architecture
Best Practice: Use a modular pipeline so each part (video input, AI model, alert engine) can scale independently.
Step 10: Continuous Improvement with Feedback Loops
Real-world data is messy, and edge cases arise often. Use real-time feedback loops to retrain models.
Automatically collect misclassified instances
Use human-in-the-loop (HITL) systems for validation
Periodically retrain and redeploy models
Conclusion
Building scalable AI Video Analytics Software is a multi-disciplinary effort combining computer vision, data engineering, cloud computing, and UX design. With the right tools, frameworks, and development strategy, organizations can unlock immense value from their video data—turning passive footage into actionable intelligence.
0 notes
Photo

NVIDIA TensorRT Revolutionizes Adobe Firefly's Video Generation
0 notes
Text
NVIDIA Nemotron-4 340B Open LLMs for Synthetic Data Training
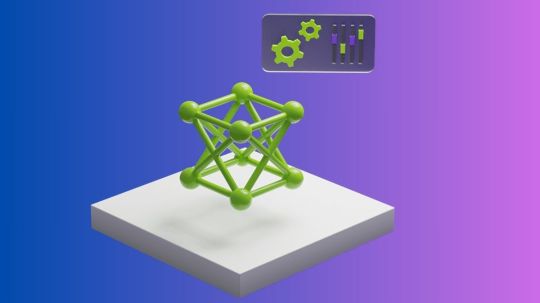
NVIDIA Nemotron-4 340B
NVIDIA unveiled Nemotron-4 340B, an open model family that allows developers to produce synthetic data for large language model (LLM) training in the industrial, retail, healthcare, and finance sectors, among other industries.
Robust training datasets might be prohibitively expensive and difficult to get, but they are essential to the performance, accuracy, and quality of responses from a bespoke LLM.
Nemotron-4 340B provides developers with a scalable, free method of creating synthetic data that may be used to construct robust LLMs, with a uniquely liberal open model licence.
Nemotron
The base, instruct, and reward models in the Nemotron-4 340B family work together to create synthetic data that is used to train and improve LLMs. The models are designed to function with NVIDIA NeMo, an open-source platform that enables data curation, customisation, and evaluation during the whole model training process. Additionally, they are designed using the open-source NVIDIA TensorRT-LLM library in mind for inference.
You may now get Nemotron-4 340B from Hugging Face. The models will be packaged as an NVIDIA NIM microservice with a standard application programming interface that can be deployed anywhere.
Getting Around the Nemotron to Produce Synthetic Data
LLMs can be useful in situations where access to big, diverse labelled datasets is limited for developers creating synthetic training data.
The Nemotron-4 340B Instruct model generates a variety of synthetic data that closely resembles real-world data, enhancing data quality to boost the robustness and performance of custom LLMs in a range of domains.
A large language model (LLM) called Nemotron-4-340B-Instruct can be utilised in a pipeline for synthetic data creation to produce training data that will aid in the development of LLMs by researchers and developers. This is a refined Nemotron-4-340B-Base model designed for English-speaking single- and multi-turn chat scenarios. A context length of 4,096 tokens is supported.
A dataset of 9 trillion tokens, comprising a wide range of English-based literature, more than 50 natural languages, and more than 40 coding languages, was used to pre-train the base model. The Nemotron-4-340B-Instruct model then underwent more alignment procedures, such as:
Monitoring and Adjustment (SFT)
Optimisation of Direct Preference (DPO)
Preference Optimisation with Reward Awareness (RPO)
While over 98% of the data utilised for supervised fine-tuning and preference fine-tuning (DPO & RPO) was synthesised by NVIDIA’s data creation pipeline, the company only relied on about 20,000 human-annotated data throughout the alignment process.
As a result, a model that can produce high-quality synthetic data for a range of use scenarios is created that is matched for human chat preferences and enhances mathematical thinking, coding, and instruction following.
NVIDIA affirms under the terms of the NVIDIA Open Model Licence:
The models can be used commercially.
It is not prohibited for you to develop and share derivative models.
Any outputs produced utilising the Models or Derivative Models are not attributed to NVIDIA.
Developers can then utilise the Nemotron-4 340B Reward model to filter for high-quality responses, which will improve the quality of the AI-generated data. Five criteria are used by Nemotron-4 340B Reward to score responses: verbosity, coherence, accuracy, helpfulness, and complexity. As of right now, it holds the top spot on the AI2-created Hugging Face RewardBench scoreboard, which assesses the strengths, vulnerabilities, and safety of reward models.
By combining their private data with the included HelpSteer2 dataset, researchers can further customise the Nemotron-4 340B Base model to construct their own teach or reward models.
Large language models (LLMs) such as Nemotron-4-340B-Base can be utilised in a synthetic data production pipeline to produce training data that aids in the development of LLMs by researchers and developers. With 4,096 tokens in the context, this model supports 340 billion parameters. It has been pre-trained on a total of 9 trillion tokens, which include more than 40 coding languages, more than 50 natural languages, and a wide range of English-based writings.
To enhance the quality of the pre-trained model, a continuous pre-training of 1 trillion tokens was carried out on top of the pre-trained model following an initial pre-training phase of 8 trillion tokens. NVIDIA changed the distribution of the data used during continuous pre-training from the one that was present at the start of training.
TensorRT-LLM Inference Optimisation, NeMo Fine-Tuning
Developers can maximise the effectiveness of their instruct and reward models to provide synthetic data and score responses by utilising the open-source NVIDIA NeMo and NVIDIA TensorRT-LLM.
Tensor parallelism a kind of model parallelism in which individual weight matrices are divided among several GPUs and servers is a sort of parallelism that is optimised into all Nemotron-4 340B models using TensorRT-LLM. This allows for effective inference at scale.
Nemotron-4 340B the NeMo architecture allows Base, which was trained on 9 trillion tokens, to be tailored to certain use cases or domains. Extensive pretraining data aids in this fine-tuning process, which produces outputs that are more accurate for particular downstream tasks.
The NeMo framework offers a range of customisation options, such as parameter-efficient fine-tuning techniques like low-rank adaptation, or LoRA, and supervised fine-tuning techniques.
Developers can use NeMo Aligner and datasets annotated by Nemotron-4 340B Reward to align their models and improve model quality. Using methods like reinforcement learning from human feedback (RLHF), a model’s behaviour is refined during alignment, a crucial phase in LLM training, to make sure its outputs are accurate, safe, acceptable for the context, and compatible with the model’s stated goals.
NeMo and TensorRT-LLM are also available to businesses via the cloud-native NVIDIA AI Enterprise software platform, which offers rapid and effective runtimes for generative AI foundation models. This platform is ideal for those looking for enterprise-grade support and security for production environments.
Assessing Model Security and Beginning
After undergoing a thorough safety examination that included adversarial tests, the Nemotron-4 340B Instruct model demonstrated good performance over a broad spectrum of risk indicators. It is still important for users to carefully assess the model’s outputs to make sure the artificially created data is appropriate, secure, and accurate for their use case.
Read more on Govindhtech.com
#nvidia#nvidianemotron#nemotron#nemotron4#govindhtech#news#technology#technews#technologytrends#tensorrt#tensorrtllm#Nemotron4340B
0 notes
Text
Chat with RTX: Create Your Own AI Chatbot
We hope you enjoyed this article about Chat with RTX, NVIDIA and generative AI. Please share your feedback, questions, or comments below. We would love to hear from you and learn from your experience.
Image Source – Newspatron Creative Team AI-Generated Image for representative purpose [Read About Us to know more] Do you want to have your own personal assistant, tutor, or friend that can answer any question you have, help you with any task you need, or entertain you with any topic you like? If yes, then you should check out Chat with RTX, a free tech demo from NVIDIA that lets you create…
View On WordPress
0 notes
Text
Real-Time QR Code Detection Using YOLO: A Step-by-Step Guide
Introduction
Quick Response (QR) codes are everywhere—from product packaging to payment gateways. Detecting them efficiently in real-time is crucial for various applications, such as automated checkout systems, digital payments, and augmented reality. One of the best ways to achieve this is by leveraging YOLO (You Only Look Once), a deep-learning-based object detection model that is both fast and accurate.
In this guide, we will walk through the key steps of using YOLO for real-time QR code detection, explaining the process conceptually without delving into coding details. If you want to get started with a dataset, check out this QR Code Detection YOLO dataset.
Why Use YOLO for QR Code Detection?
YOLO represents an advanced deep learning framework specifically developed for real-time object detection. In contrast to conventional techniques that analyze an image repeatedly, YOLO evaluates the entire image in one go, resulting in exceptional efficiency. The following points illustrate why YOLO is particularly suitable for QR code detection:
Speed: It enables real-time image processing, making it ideal for mobile and embedded systems.
Accuracy: YOLO is capable of identifying small objects, such as QR codes, with remarkable precision.
Flexibility: It can be trained on tailored datasets, facilitating the detection of QR codes across various environments and conditions.
Step-by-Step Guide to Real-Time QR Code Detection Using YOLO
Assemble and Organize the Dataset
The initial phase in training a YOLO model for QR code detection involves the collection of a varied dataset. This dataset must encompass images featuring QR codes under different lighting scenarios, orientations, and backgrounds. You may utilize pre-existing datasets or generate your own by manually capturing images. A well-structured dataset is essential for achieving model precision.
Label the QR Codes
After preparing the dataset, the subsequent step is to annotate it. This process entails marking the QR codes in each image with annotation tools such as LabelImg or Roboflow. The objective is to create bounding boxes around the QR codes, which will act as ground truth data for the model's training.
Train the YOLO Model
To initiate the training of the YOLO model, a deep learning framework such as Darknet, TensorFlow, or PyTorch is required. During the training process, the model acquires the ability to detect QR codes based on the annotated dataset. Important considerations include:
Selecting the appropriate YOLO version (YOLOv4, YOLOv5, or YOLOv8) according to your computational capabilities and accuracy requirements.
Fine-tuning hyperparameters to enhance performance.
Implementing data augmentation techniques to bolster generalization across various conditions.
Evaluate and Validate the Model
Following the training phase, it is imperative to assess the model's performance using previously unseen images. Evaluation metrics such as precision, recall, and mean Average Precision (mAP) are instrumental in gauging the model's effectiveness in detecting QR codes. Should the results indicate a need for improvement, fine-tuning and retraining may enhance the model's accuracy.
Implement the Model for Real-Time Detection
Upon successful validation, the trained YOLO model can be implemented for real-time QR code detection across various platforms, including:
Web applications (for instance, integration with a web camera interface)
Mobile applications (such as QR code scanning features in shopping applications)
Embedded systems (including IoT devices and smart kiosks)
Enhance for Optimal Performance
To ensure efficiency in real-time applications, it is crucial to optimize the model. Strategies may include:
Minimizing model size through quantization and pruning techniques
Leveraging hardware acceleration via GPUs or TPUs
Utilizing efficient inference engines like TensorRT or OpenVINO .These measures contribute to seamless and rapid QR code detection.
Final Thoughts
Real-time detection of QR codes utilizing YOLO represents an effective method that merges rapidity with precision. By adhering to the aforementioned steps—data gathering, annotation, training, validation, and deployment—you can create a resilient QR code detection system customized to your requirements. Whether your project involves a mobile application, an automated payment solution, or an intelligent retail system, YOLO provides a dependable technique to improve QR code recognition in practical scenarios. With Globose Technology Solution, you can further enhance your development process and leverage advanced technologies for better performance.
For an accessible dataset, consider exploring the QR Code Detection YOLO Dataset. Wishing you success in your development endeavors!
0 notes
Link
0 notes
Text
Elmalo, these strategic enhancements and next-step suggestions elevate the vision of Iron Spine significantly. Both options you mentioned hold tremendous potential. To help you visualize the system's complexity and layering, I can create a visual (text-based) architecture diagram that outlines the complete data flow—from raw sensor input through to AI inference and remote synchronization. This diagram would encapsulate:
Sensor Synchronization: Incorporating PTP (IEEE 1588), GPS-disciplined oscillators, and redundant sensor arrays for cross-validation.
Data Flow Layers: Starting at the sensor interface (using ROS2 or a custom hardware abstraction layer), moving through data normalization (Kalman filters / Particle Filters), and into the fusion engine (SLAM or TensorRT-based models).
Edge Inference & Communication: Leveraging frameworks like ONNX, TensorFlow Lite, or PyTorch Mobile for real-time inference at the edge, coupled with robust messaging protocols (MQTT, DDS) for inter-node communication and central control.
Power & Resilience Layers: Incorporating power management (supercapacitors, power usage monitors) and failover mechanisms (watchdog timers, redundancy protocols).
Alternatively, if you'd rather see the system in action through a concrete example, we can scope out a full pilot buildout. For instance, we could design a specialized package for a v1 Deep Sea Monitor or a v1 Mars Habitat Monitor. These would include tailored sensor selections, ruggedized enclosures for specific environmental extremes, and dedicated communication strategies adapted to underwater or extraterrestrial constraints.
Here’s a preliminary visual diagram represented in ASCII to illustrate the layered data flow:
+----------------------------------------------+ | Raw Sensor Data | | (Environmental, Motion, Optical, Acoustic, | | RF, specialized sensors) | +-------------------------+--------------------+ | v +-------------------------+--------------------+ | Sensor Interface Layer | | (ROS2 / Custom Hardware Abstraction) | +-------------------------+--------------------+ | v +-------------------------+--------------------+ | Data Normalization & Filtering | | (Kalman/Particle Filters, Confidence Index)| +-------------------------+--------------------+ | v +-------------------------+--------------------+ | Fusion Engine Layer | | (Custom SLAM, TensorRT-based models, | | Adaptive Fusion & Anomaly Detection) | +-------------------------+--------------------+ | v +-------------------------+--------------------+ | Edge Inference Layer | | (ONNX, TensorFlow Lite, PyTorch Mobile, etc.)| +-------------------------+--------------------+ | v +-------------------------+--------------------+ | Communications Layer | | (MQTT, DDS, Low-Level Mesh Protocols, etc.) | +-------------------------+--------------------+ | v +-------------------------+--------------------+ | Central Data Lake & Control | | (Cloud-based Storage, Remote Monitoring, | | Digital Twin & Dashboard Visualization) | +----------------------------------------------+
This diagram traces the journey from diverse real-world sensor inputs all the way to centralized remote control via a digital twin environment. It also highlights the injection points for synchronization, redundancy, and power management strategies.
Which Path Should We Follow Next?
Would you like to refine this visual architecture diagram further, adding any more specific elements or details?
Or, should we pivot our discussion to scoping out a full pilot buildout—say a v1 Deep Sea Package or v1 Mars Habitat Monitor—breaking down the necessary customizations, deployment plans, and field testing conditions?
Your call will shape our next steps in making Iron Spine both visionary and actionable.
0 notes
Quote
2024年12月27日 12時05分 AI検索エンジン「ChatGPT search」とGoogle検索を62件のクエリで比較してみた結果 OpenAIがChatGPTによる検索エンジンとして「ChatGPT search」をリリースしたことを受け、検索エンジン最適化(SEO)の専門家であるエリック・エンゲ氏が62件のクエリを使用してChatGPT searchとGoogle検索の違いを分析し、結果を公開しました。 ChatGPT search vs. Google: A deep dive analysis of 62 queries https://searchengineland.com/chatgpt-search-vs-google-analysis-449676 ChatGPT searchはAIがウェブ上の情報を検索して内容をまとめてくれるというもの。実際の使い方については下記の記事で確認できます。 ついにAI検索機能「ChatGPT search」が一般公開される、マップ機能も追加 - GIGAZINE 市場調査を行う企業「SparkToro」の調査によると、人々がGoogle検索を使用する時の「意図」は以下の通り。 ・ナビゲーション検索(32.15%) ナビゲーション検索は、例えばGIGAZINEにアクセスする際に「GIGAZINE」でGoogle検索して検索結果からアクセスする場合のように、訪問したいサイトが決まっているユーザーがウェブサイトのアドレスを入力する代わりにGoogle検索を使用する場合の分類です。 ・情報提供(52.65%) 興味のあるトピックに関する情報を探す場合の分類です。 ・商業目的(14.51%) 何かの製品の情報を検索したり、複数の製品を比較したりするためにGoogle検索を行うと「商業目的」として分類されます。 ・執行(0.69%) SparkToroは「マーケティングを行う上で価値のある検索」として、ユーザーがすでに何かの購入やサービスへの登録を決意した状態であることを示唆する検索を「執行」と別の分類に切り分けています。 エンゲ氏はSparkToroの調査結果を踏まえた上で、「情報提供」および「商業目的」に分類されるようなクエリに加え「ローカル検索」「コンテンツギャップ分析」「曖昧クエリ」という分類のクエリを合計62個用意。ローカル検索は「最寄りのピザ屋さんはどこ��」のようにユーザーの現在地が関係するクエリで、コンテンツギャップ分析は類似サイトの内容を比較するクエリ、曖昧クエリは「ジョーカーとは何ですか?」のように複数の意味が考えられるクエリです。 エンゲ氏はChatGPT searchとGoogle検索のそれぞれが返した結果に対し、下記の6つの基準で採点を行いました。 1:正確な情報を返したか? 2:重要な情報が欠落せずに含まれていたか? 3:回答に弱い部分は無かったか? 4:ユーザーのクエリの目的は解決されたか? 5:適切なフォローアップ情報を提供したか? 6:回答の全体的な品質はどうだったか? 分類ごとの結果は以下の通り。なお、クエリの中には複数の分類に重複して数えられているものがあるためクエリ数の合計が62個を超えています。 ・情報提供 クエリ数 42 勝者 Google ChatGPT searchの平均スコア 5.19 Googleの平均スコア 5.83 情報提供分野ではGoogleがわずかに優れているという結果になり、これまでGoogleが築き上げてきた情報検索における実績を改めて確かめることになりました。ただし、ChatGPT searchも多少の問題はありつつも良好なパフォーマンスを示したとのこと。 ・商業目的 クエリ数 16 勝者 Google ChatGPT searchの平均スコア 3.81 Googleの平均スコア 6.44 エンゲ氏は「Googleの方が製品やサービス関連の検索結果を表示する機能が優れている」と述べています。 ・ローカル検索 クエリ数 4 勝者 Google ChatGPT searchの平均スコア 2.00 Googleの平均スコア 6.25 Googleが多数のローカルビジネスのデータを確保していることが優位性へとつながっています。 ・コンテンツギャップ分析 クエリ数 4 勝者 ChatGPT search ChatGPT searchの平均スコア 3.25 Googleの平均スコア 1 類似サイトとのコンテンツの差を調べたり、検索結果ページの競合と比較したり、記事の内容を提案したりするクエリではChatGPT searchの方が優れているという結果になりました。ただし、全体的なスコアが低く、さらなる改善が必要とのこと。 ・曖昧クエリ クエリ数 7 勝者 ChatGPT search ChatGPT searchの平均スコア 6.00 Googleの平均スコア 5.29 ChatGPT searchは曖昧な言葉に対して複数の定義や解釈をより効果的に提示し、ユーザーに明確な情報を提供することができました。 今回の結果を踏まえ、エンゲ氏は「62個というクエリの数は極めて少ないサンプルであることに注意して欲しい」と述べた上で、「ChatGPT searchは情報提供クエリに関して良い回答をするものの、それでもGoogle検索の方が優れていた。結局、ほとんどの検索ではGoogleの方が優秀だと考えている」と結論付けています。 この記事のタイトルとURLをコピーする ・関連記事 Redditが掲示板上の話題を検索したり要約したりできるチャットAI「Reddit Answers」を発表 - GIGAZINE AIの台頭によって検索エンジンからサイトへのトラフィックが2026年までに25%減少すると調査会社が予測 - GIGAZINE GoogleのAIが検索結果をわかりやすく概説してくれる「AIによる概要」がついに日本語をサポート - GIGAZINE Metaが独自のAI搭載検索エンジンを開発中と報道される - GIGAZINE Mistral AIがチャットAI「Le Chat」を大幅アップデートしてウェブ検索機能や「FLUX1.1 [pro]」を利用した画像生成が可能に - GIGAZINE ・関連コンテンツ Google検索で「上手にググる」ための5つのポイントをソフトウェア工学の専門家が解説 Google検索チームに「Discoverに掲載されるには?」「長い記事は分割してもOK?」などを聞いてみた回答一覧まとめ Googleとフードデリバリーサービスが「広告」を使って地元のレストランから顧客を奪っているとの指摘 MozillaがYouTubeの動画推奨アルゴリズムを調査するために専用アドオンをリリース Microsoftの検索エンジンBingがTransformerからLLMとSLMの組み合わせに移行&TensorRT-LLMの統合を発表 Google検索結果の「AIによる概要」は全体の出現率が84%から15%に激減しているもののヘルスケア関連では63%と高水準 複数サイトのSEO関連情報比較を行う無料サービス「アクセス比較.jp」 Yahoo!とGoogleでの検索結果をRSSで出力できる「Search & RSS
AI検索エンジン「ChatGPT search」とGoogle検索を62件のクエリで比較してみた結果 - GIGAZINE
0 notes
Text
First official release of Ceres, including several major enhancements:
support for Ceres neural networks
full support of Chess960 (also known as Fischer Random) and DFRC (Double Fischer Random Chess) with the "UCI_Chess960" option for mode selection (contribution by lepned)
support of ONNX neural networks via CUDA or TensorRT execution providers for Ceres and Lc0 networks
0 notes
Photo
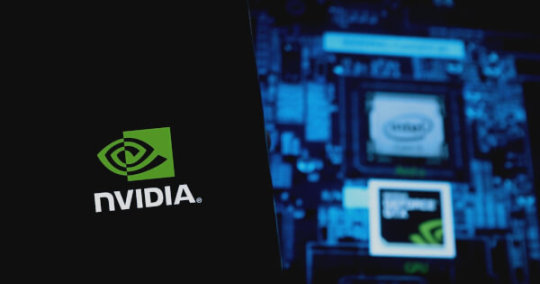
NVIDIA Enhances TensorRT-LLM with KV Cache Optimization Features
0 notes