#big data analytics and python in business
Explore tagged Tumblr posts
Text
In today’s digital age, data has become an invaluable asset for businesses across various industries.
0 notes
Text
Explore IGMPI’s Big Data Analytics program, designed for professionals seeking expertise in data-driven decision-making. Learn advanced analytics techniques, data mining, machine learning, and business intelligence tools to excel in the fast-evolving world of big data.
#Big Data Analytics#Data Science#Machine Learning#Predictive Analytics#Business Intelligence#Data Visualization#Data Mining#AI in Analytics#Big Data Tools#Data Engineering#IGMPI#Online Analytics Course#Data Management#Hadoop#Python for Data Science
0 notes
Text
Data Analytics Training In Marathahalli
Techyse Education in Marathahalli, Bangalore, offers specialized Data Analytics Training in Marathahalli for individuals looking to build expertise in Python, Power BI, and data analysis techniques. Their industry-aligned courses focus on practical learning through real-world projects, ensuring students gain hands-on experience in data manipulation, visualization, and dashboard creation. Whether you are a beginner or an experienced professional, Techyse’s programs are designed to enhance your skill set, making you job-ready for roles in data analytics.
Comprehensive Data Analytics Training in Marathahalli Techyse Education takes pride in delivering high-quality Data Analytics Training in Marathahalli, backed by experienced instructors with deep industry knowledge. The curriculum covers essential tools and techniques, from data wrangling with Python to creating interactive dashboards using Power BI, ensuring students are prepared to meet industry demands. With personalized mentorship, career support, and placement assistance, Techyse provides a well-rounded learning experience. Whether aiming for career growth or a fresh start in data analytics, Techyse Education equips learners with the skills to excel in a competitive job market.
Techyse Education | Data Analyst, Python, Power BI Training in Marathahalli, Bangalore
18, Krishna Summit, 307, 3rd Floor, Aswath Nagar, Next to Canara Bank, Marathahalli, Bangalore, Karnataka 560037
Phone: 098445 14333 Website : https://techyse.in/
Our Google Map Location is : https://maps.app.goo.gl/dLsBM669nKHTutxu9
Follow us: Facebook : https://www.facebook.com/techyse.education/ Twitter: https://x.com/techyse_edu/ Instagram : https://www.instagram.com/techyeseducation/ LinkedIn : https://www.linkedin.com/company/techyse-education/ Youtube: https://www.youtube.com/@TechyseEducation
#Data Analytics Marathahalli#Analytics Training#Data Science Marathahalli#Big Data Training#Data Analytics Course#Learn Data Analytics#Marathahalli Training#Data Science Bangalore#Analytics Experts#Data Analyst Training#Data Skills#Marathahalli Courses#Data Analytics India#Bangalore Training#Data Visualization#SQL Training#Python for Data Analytics#Machine Learning Training#Data Science Bootcamp#Business Intelligence#AI and Analytics#Learn Data Science#Career in Analytics#Analytics Certification#Marathahalli Academy#Data Driven#Advanced Analytics#Real World Analytics#Data Analytics Hub#Data Science Courses Bangalore
0 notes
Text
Big Data vs. Traditional Data: Understanding the Differences and When to Use Python

In the evolving landscape of data science, understanding the nuances between big data and traditional data is crucial. Both play pivotal roles in analytics, but their characteristics, processing methods, and use cases differ significantly. Python, a powerful and versatile programming language, has become an indispensable tool for handling both types of data. This blog will explore the differences between big data and traditional data and explain when to use Python, emphasizing the importance of enrolling in a data science training program to master these skills.
What is Traditional Data?
Traditional data refers to structured data typically stored in relational databases and managed using SQL (Structured Query Language). This data is often transactional and includes records such as sales transactions, customer information, and inventory levels.
Characteristics of Traditional Data:
Structured Format: Traditional data is organized in a structured format, usually in rows and columns within relational databases.
Manageable Volume: The volume of traditional data is relatively small and manageable, often ranging from gigabytes to terabytes.
Fixed Schema: The schema, or structure, of traditional data is predefined and consistent, making it easy to query and analyze.
Use Cases of Traditional Data:
Transaction Processing: Traditional data is used for transaction processing in industries like finance and retail, where accurate and reliable records are essential.
Customer Relationship Management (CRM): Businesses use traditional data to manage customer relationships, track interactions, and analyze customer behavior.
Inventory Management: Traditional data is used to monitor and manage inventory levels, ensuring optimal stock levels and efficient supply chain operations.
What is Big Data?
Big data refers to extremely large and complex datasets that cannot be managed and processed using traditional database systems. It encompasses structured, unstructured, and semi-structured data from various sources, including social media, sensors, and log files.
Characteristics of Big Data:
Volume: Big data involves vast amounts of data, often measured in petabytes or exabytes.
Velocity: Big data is generated at high speed, requiring real-time or near-real-time processing.
Variety: Big data comes in diverse formats, including text, images, videos, and sensor data.
Veracity: Big data can be noisy and uncertain, requiring advanced techniques to ensure data quality and accuracy.
Use Cases of Big Data:
Predictive Analytics: Big data is used for predictive analytics in fields like healthcare, finance, and marketing, where it helps forecast trends and behaviors.
IoT (Internet of Things): Big data from IoT devices is used to monitor and analyze physical systems, such as smart cities, industrial machines, and connected vehicles.
Social Media Analysis: Big data from social media platforms is analyzed to understand user sentiments, trends, and behavior patterns.
Python: The Versatile Tool for Data Science
Python has emerged as the go-to programming language for data science due to its simplicity, versatility, and robust ecosystem of libraries and frameworks. Whether dealing with traditional data or big data, Python provides powerful tools and techniques to analyze and visualize data effectively.
Python for Traditional Data:
Pandas: The Pandas library in Python is ideal for handling traditional data. It offers data structures like DataFrames that facilitate easy manipulation, analysis, and visualization of structured data.
SQLAlchemy: Python's SQLAlchemy library provides a powerful toolkit for working with relational databases, allowing seamless integration with SQL databases for querying and data manipulation.
Python for Big Data:
PySpark: PySpark, the Python API for Apache Spark, is designed for big data processing. It enables distributed computing and parallel processing, making it suitable for handling large-scale datasets.
Dask: Dask is a flexible parallel computing library in Python that scales from single machines to large clusters, making it an excellent choice for big data analytics.
When to Use Python for Data Science
Understanding when to use Python for different types of data is crucial for effective data analysis and decision-making.
Traditional Data:
Business Analytics: Use Python for traditional data analytics in business scenarios, such as sales forecasting, customer segmentation, and financial analysis. Python's libraries, like Pandas and Matplotlib, offer comprehensive tools for these tasks.
Data Cleaning and Transformation: Python is highly effective for data cleaning and transformation, ensuring that traditional data is accurate, consistent, and ready for analysis.
Big Data:
Real-Time Analytics: When dealing with real-time data streams from IoT devices or social media platforms, Python's integration with big data frameworks like Apache Spark enables efficient processing and analysis.
Large-Scale Machine Learning: For large-scale machine learning projects, Python's compatibility with libraries like TensorFlow and PyTorch, combined with big data processing tools, makes it an ideal choice.
The Importance of Data Science Training Programs
To effectively navigate the complexities of both traditional data and big data, it is essential to acquire the right skills and knowledge. Data science training programs provide comprehensive education and hands-on experience in data science tools and techniques.
Comprehensive Curriculum: Data science training programs cover a wide range of topics, including data analysis, machine learning, big data processing, and data visualization, ensuring a well-rounded education.
Practical Experience: These programs emphasize practical learning through projects and case studies, allowing students to apply theoretical knowledge to real-world scenarios.
Expert Guidance: Experienced instructors and industry mentors offer valuable insights and support, helping students master the complexities of data science.
Career Opportunities: Graduates of data science training programs are in high demand across various industries, with opportunities to work on innovative projects and drive data-driven decision-making.
Conclusion
Understanding the differences between big data and traditional data is fundamental for any aspiring data scientist. While traditional data is structured, manageable, and used for transaction processing, big data is vast, varied, and requires advanced tools for real-time processing and analysis. Python, with its robust ecosystem of libraries and frameworks, is an indispensable tool for handling both types of data effectively.
Enrolling in a data science training program equips you with the skills and knowledge needed to navigate the complexities of data science. Whether you're working with traditional data or big data, mastering Python and other data science tools will enable you to extract valuable insights and drive innovation in your field. Start your journey today and unlock the potential of data science with a comprehensive training program.
#Big Data#Traditional Data#Data Science#Python Programming#Data Analysis#Machine Learning#Predictive Analytics#Data Science Training Program#SQL#Data Visualization#Business Analytics#Real-Time Analytics#IoT Data#Data Transformation
0 notes
Text
What are the best data analytics courses?
In today's data- driven folks, the capability to dissect and decide perceptivity from vast quantities of information is a largely sought- after skill. Whether you are a freshman looking to dive into the instigative field of data analytics or an educated professional aiming to upskill, chancing the stylish data analytics courses is essential to boost your career.
Introductions that have been involved in the data analysis process include data mining, operation, statistics, and donation of data. The very first step is extracting the data from various sources like huge databases, textbooks, detector data, etc. Using the concept of ETL (extract, transform, load), raw data is converted into a manageable format. This process is called data mining and is the most crucial step in the channel. Data warehousing or operation is the next step in the analysis that implements the databases by deriving.
To embark on this informational trip, I have curated a collection of top- notch data analytics courses that offer unique perspectives, interactive literacy gests, and comprehensive knowledge.
Data analytics:
Data analytics can help small businesses in several ways. By understanding data analytics, businesses can make better opinions about where to allocate their coffers and how to price their products or services. also, data analytics can help businesses identify trends and understand their client base. Data analytics software can track and dissect data, allowing you to produce practicable reports and dashboards. However, read our companion to the stylish data analytics tools and software available moment if you ’re looking for a dependable result.
Let's explore these courses together and discover which bones reverberate with your literacy style and pretensions. Data analytics is the part of a data critic to find patterns in the information present to Ameliorate performance and effectiveness. They also need to work through a complete analysis of the channel of data. Which means there can be multitudinous ways to dissect data. These are the top online courses for data analysis. 1. Data Analytics with R and Excel
As a data analyst, Excel is a powerful tool that can help you organize, analyze, and visualize data. Here are some key skills and techniques you can use in Excel for data analysis: Excel provides various functions and tools to clean and transform your data. You can use functions like TRIM, CLEAN, and SUBSTITUTE to remove extra spaces, and special characters, or replace text. Tools like data filters and conditional formatting can help you identify and handle missing or inconsistent data.
2. Big Data Analysis with Python
Community Support Python has a large and active community that supports and contributes to the development of colourful data wisdom libraries and tools. This community has produced numerous useful libraries, including Pandas, NumPy, matplotlib, and SciPy, which are extensively used in data science.
3. Python for data analytics
Thanks to Python's focus on simplicity and readability, it boasts a gradational and low literacy wind. This ease of literacy makes Python an ideal tool for neophyte programmers. Python offers programmers the advantage of using smaller lines of law to perform tasks than is demanded when using aged languages. In other words, you spend further time playing and lower time working law.
4. Business Analytics specialization with Tableau
A powerful BI tool, Tableau is the second most popular one from our survey. It helps extract and gather data stored in multiple locations and comes with an intuitive drag-and-drop interface. Tableau makes data across departments readily available for data engineers and managers to create useful dashboards.
Conclusion:
Data analyst boot camps are suitable for individuals who want to kick-start their careers in data analysis or professionals seeking to upskill and transition into a data-related role. By providing a condensed and focused learning experience, boot camps aim to prepare participants for entry-level data analyst positions or provide them with a strong foundation to pursue further studies or certifications in the field of data analytics. Excel provides built-in data analysis tools, such as regression analysis, descriptive statistics, and goal-seeking. These tools can help you perform advanced analysis, such as identifying trends, finding correlations, forecasting, and performing what-if scenarios.
Here's how you can get started!
1 note
·
View note
Text
Why Python Will Thrive: Future Trends and Applications
Python has already made a significant impact in the tech world, and its trajectory for the future is even more promising. From its simplicity and versatility to its widespread use in cutting-edge technologies, Python is expected to continue thriving in the coming years. Considering the kind support of Python Course in Chennai Whatever your level of experience or reason for switching from another programming language, learning Python gets much more fun.
Let's explore why Python will remain at the forefront of software development and what trends and applications will contribute to its ongoing dominance.
1. Artificial Intelligence and Machine Learning
Python is already the go-to language for AI and machine learning, and its role in these fields is set to expand further. With powerful libraries such as TensorFlow, PyTorch, and Scikit-learn, Python simplifies the development of machine learning models and artificial intelligence applications. As more industries integrate AI for automation, personalization, and predictive analytics, Python will remain a core language for developing intelligent systems.
2. Data Science and Big Data
Data science is one of the most significant areas where Python has excelled. Libraries like Pandas, NumPy, and Matplotlib make data manipulation and visualization simple and efficient. As companies and organizations continue to generate and analyze vast amounts of data, Python’s ability to process, clean, and visualize big data will only become more critical. Additionally, Python’s compatibility with big data platforms like Hadoop and Apache Spark ensures that it will remain a major player in data-driven decision-making.
3. Web Development
Python’s role in web development is growing thanks to frameworks like Django and Flask, which provide robust, scalable, and secure solutions for building web applications. With the increasing demand for interactive websites and APIs, Python is well-positioned to continue serving as a top language for backend development. Its integration with cloud computing platforms will also fuel its growth in building modern web applications that scale efficiently.
4. Automation and Scripting
Automation is another area where Python excels. Developers use Python to automate tasks ranging from system administration to testing and deployment. With the rise of DevOps practices and the growing demand for workflow automation, Python’s role in streamlining repetitive processes will continue to grow. Businesses across industries will rely on Python to boost productivity, reduce errors, and optimize performance. With the aid of Best Online Training & Placement Programs, which offer comprehensive training and job placement support to anyone looking to develop their talents, it’s easier to learn this tool and advance your career.
5. Cybersecurity and Ethical Hacking
With cyber threats becoming increasingly sophisticated, cybersecurity is a critical concern for businesses worldwide. Python is widely used for penetration testing, vulnerability scanning, and threat detection due to its simplicity and effectiveness. Libraries like Scapy and PyCrypto make Python an excellent choice for ethical hacking and security professionals. As the need for robust cybersecurity measures increases, Python’s role in safeguarding digital assets will continue to thrive.
6. Internet of Things (IoT)
Python’s compatibility with microcontrollers and embedded systems makes it a strong contender in the growing field of IoT. Frameworks like MicroPython and CircuitPython enable developers to build IoT applications efficiently, whether for home automation, smart cities, or industrial systems. As the number of connected devices continues to rise, Python will remain a dominant language for creating scalable and reliable IoT solutions.
7. Cloud Computing and Serverless Architectures
The rise of cloud computing and serverless architectures has created new opportunities for Python. Cloud platforms like AWS, Google Cloud, and Microsoft Azure all support Python, allowing developers to build scalable and cost-efficient applications. With its flexibility and integration capabilities, Python is perfectly suited for developing cloud-based applications, serverless functions, and microservices.
8. Gaming and Virtual Reality
Python has long been used in game development, with libraries such as Pygame offering simple tools to create 2D games. However, as gaming and virtual reality (VR) technologies evolve, Python’s role in developing immersive experiences will grow. The language’s ease of use and integration with game engines will make it a popular choice for building gaming platforms, VR applications, and simulations.
9. Expanding Job Market
As Python’s applications continue to grow, so does the demand for Python developers. From startups to tech giants like Google, Facebook, and Amazon, companies across industries are seeking professionals who are proficient in Python. The increasing adoption of Python in various fields, including data science, AI, cybersecurity, and cloud computing, ensures a thriving job market for Python developers in the future.
10. Constant Evolution and Community Support
Python’s open-source nature means that it’s constantly evolving with new libraries, frameworks, and features. Its vibrant community of developers contributes to its growth and ensures that Python stays relevant to emerging trends and technologies. Whether it’s a new tool for AI or a breakthrough in web development, Python’s community is always working to improve the language and make it more efficient for developers.
Conclusion
Python’s future is bright, with its presence continuing to grow in AI, data science, automation, web development, and beyond. As industries become increasingly data-driven, automated, and connected, Python’s simplicity, versatility, and strong community support make it an ideal choice for developers. Whether you are a beginner looking to start your coding journey or a seasoned professional exploring new career opportunities, learning Python offers long-term benefits in a rapidly evolving tech landscape.
#python course#python training#python#technology#tech#python programming#python online training#python online course#python online classes#python certification
2 notes
·
View notes
Text
Short-Term vs. Long-Term Data Analytics Course in Delhi: Which One to Choose?
In today’s digital world, data is everywhere. From small businesses to large organizations, everyone uses data to make better decisions. Data analytics helps in understanding and using this data effectively. If you are interested in learning data analytics, you might wonder whether to choose a short-term or a long-term course. Both options have their benefits, and your choice depends on your goals, time, and career plans.
At Uncodemy, we offer both short-term and long-term data analytics courses in Delhi. This article will help you understand the key differences between these courses and guide you to make the right choice.
What is Data Analytics?
Data analytics is the process of examining large sets of data to find patterns, insights, and trends. It involves collecting, cleaning, analyzing, and interpreting data. Companies use data analytics to improve their services, understand customer behavior, and increase efficiency.
There are four main types of data analytics:
Descriptive Analytics: Understanding what has happened in the past.
Diagnostic Analytics: Identifying why something happened.
Predictive Analytics: Forecasting future outcomes.
Prescriptive Analytics: Suggesting actions to achieve desired outcomes.
Short-Term Data Analytics Course
A short-term data analytics course is a fast-paced program designed to teach you essential skills quickly. These courses usually last from a few weeks to a few months.
Benefits of a Short-Term Data Analytics Course
Quick Learning: You can learn the basics of data analytics in a short time.
Cost-Effective: Short-term courses are usually more affordable.
Skill Upgrade: Ideal for professionals looking to add new skills without a long commitment.
Job-Ready: Get practical knowledge and start working in less time.
Who Should Choose a Short-Term Course?
Working Professionals: If you want to upskill without leaving your job.
Students: If you want to add data analytics to your resume quickly.
Career Switchers: If you want to explore data analytics before committing to a long-term course.
What You Will Learn in a Short-Term Course
Introduction to Data Analytics
Basic Tools (Excel, SQL, Python)
Data Visualization (Tableau, Power BI)
Basic Statistics and Data Interpretation
Hands-on Projects
Long-Term Data Analytics Course
A long-term data analytics course is a comprehensive program that provides in-depth knowledge. These courses usually last from six months to two years.
Benefits of a Long-Term Data Analytics Course
Deep Knowledge: Covers advanced topics and techniques in detail.
Better Job Opportunities: Preferred by employers for specialized roles.
Practical Experience: Includes internships and real-world projects.
Certifications: You may earn industry-recognized certifications.
Who Should Choose a Long-Term Course?
Beginners: If you want to start a career in data analytics from scratch.
Career Changers: If you want to switch to a data analytics career.
Serious Learners: If you want advanced knowledge and long-term career growth.
What You Will Learn in a Long-Term Course
Advanced Data Analytics Techniques
Machine Learning and AI
Big Data Tools (Hadoop, Spark)
Data Ethics and Governance
Capstone Projects and Internships
Key Differences Between Short-Term and Long-Term Courses
FeatureShort-Term CourseLong-Term CourseDurationWeeks to a few monthsSix months to two yearsDepth of KnowledgeBasic and Intermediate ConceptsAdvanced and Specialized ConceptsCostMore AffordableHigher InvestmentLearning StyleFast-PacedDetailed and ComprehensiveCareer ImpactQuick Entry-Level JobsBetter Career Growth and High-Level JobsCertificationBasic CertificateIndustry-Recognized CertificationsPractical ProjectsLimitedExtensive and Real-World Projects
How to Choose the Right Course for You
When deciding between a short-term and long-term data analytics course at Uncodemy, consider these factors:
Your Career Goals
If you want a quick job or basic knowledge, choose a short-term course.
If you want a long-term career in data analytics, choose a long-term course.
Time Commitment
Choose a short-term course if you have limited time.
Choose a long-term course if you can dedicate several months to learning.
Budget
Short-term courses are usually more affordable.
Long-term courses require a bigger investment but offer better returns.
Current Knowledge
If you already know some basics, a short-term course will enhance your skills.
If you are a beginner, a long-term course will provide a solid foundation.
Job Market
Short-term courses can help you get entry-level jobs quickly.
Long-term courses open doors to advanced and specialized roles.
Why Choose Uncodemy for Data Analytics Courses in Delhi?
At Uncodemy, we provide top-quality training in data analytics. Our courses are designed by industry experts to meet the latest market demands. Here’s why you should choose us:
Experienced Trainers: Learn from professionals with real-world experience.
Practical Learning: Hands-on projects and case studies.
Flexible Schedule: Choose classes that fit your timing.
Placement Assistance: We help you find the right job after course completion.
Certification: Receive a recognized certificate to boost your career.
Final Thoughts
Choosing between a short-term and long-term data analytics course depends on your goals, time, and budget. If you want quick skills and job readiness, a short-term course is ideal. If you seek in-depth knowledge and long-term career growth, a long-term course is the better choice.
At Uncodemy, we offer both options to meet your needs. Start your journey in data analytics today and open the door to exciting career opportunities. Visit our website or contact us to learn more about our Data Analytics course in delhi.
Your future in data analytics starts here with Uncodemy!
2 notes
·
View notes
Text
Business Analytics vs. Data Science: Understanding the Key Differences
In today's data-driven world, terms like "business analytics" and "data science" are often used interchangeably. However, while they share a common goal of extracting insights from data, they are distinct fields with different focuses and methodologies. Let's break down the key differences to help you understand which path might be right for you.
Business Analytics: Focusing on the Present and Past
Business analytics primarily focuses on analyzing historical data to understand past performance and inform current business decisions. It aims to answer questions like:
What happened?
Why did it happen?
What is happening now?
Key characteristics of business analytics:
Descriptive and Diagnostic: It uses techniques like reporting, dashboards, and data visualization to summarize and explain past trends.
Structured Data: It often works with structured data from databases and spreadsheets.
Business Domain Expertise: A strong understanding of the specific business domain is crucial.
Tools: Business analysts typically use tools like Excel, SQL, Tableau, and Power BI.
Focus: Optimizing current business operations and improving efficiency.
Data Science: Predicting the Future and Building Models
Data science, on the other hand, focuses on building predictive models and developing algorithms to forecast future outcomes. It aims to answer questions like:
What will happen?
How can we make it happen?
Key characteristics of data science:
Predictive and Prescriptive: It uses machine learning, statistical modeling, and AI to predict future trends and prescribe optimal actions.
Unstructured and Structured Data: It can handle both structured and unstructured data from various sources.
Technical Proficiency: Strong programming skills (Python, R) and a deep understanding of machine learning algorithms are essential.
Tools: Data scientists use programming languages, machine learning libraries, and big data technologies.
Focus: Developing innovative solutions, building AI-powered products, and driving long-term strategic initiatives.
Key Differences Summarized:

Which Path is Right for You?
Choose Business Analytics if:
You are interested in analyzing past data to improve current business operations.
You have a strong understanding of a specific business domain.
You prefer working with structured data and using visualization tools.
Choose Data Science if:
You are passionate about building predictive models and developing AI-powered solutions.
You have a strong interest in programming and machine learning.
You enjoy working with both structured and unstructured data.
Xaltius Academy's Data Science & AI Course:
If you're leaning towards data science and want to delve into machine learning and AI, Xaltius Academy's Data Science & AI course is an excellent choice. This program equips you with the necessary skills and knowledge to become a proficient data scientist, covering essential topics like:
Python programming
Machine learning algorithms
Data visualization
And much more!
By understanding the distinct roles of business analytics and data science, you can make an informed decision about your career path and leverage the power of data to drive success.
2 notes
·
View notes
Text
Decoding Data Roles: A Comprehensive Guide to Data Analysts and Data Scientists
In today's data-driven landscape, the roles of data analysts and data scientists share some similarities but differ significantly in terms of their focus, skill sets, and the scope of their work. As organizations increasingly recognize the importance of these roles, the demand for skilled professionals has led to the emergence of various data science institutes. Let's explore the nuances that differentiate a data analyst from a data scientist, while also considering the importance of choosing the best Data Science institute for a comprehensive education in this field.
Let's delve into the nuances that differentiate a data analyst from a data scientist.
1. The Scope of Work:
Data analysts and data scientists play distinct roles when it comes to the scope of their work.
Data Analyst:
Data analysts are the interpreters of historical data. Their primary focus lies in uncovering trends, generating reports, and providing insights that aid day-to-day operations within an organization. They work with structured data, employing tools such as Excel, SQL, and visualization tools like Tableau or Power BI. The problems they address are typically well-defined and pertain to specific queries.
Data Scientist:
Data scientists, on the other hand, have a broader scope. While data analysis is a part of their work, they are also deeply involved in more complex tasks. This includes developing machine learning models, engaging in predictive modeling, and conducting advanced analytics. Data scientists deal with unstructured or semi-structured data, addressing more intricate and less defined problems. Their role extends beyond routine data interpretation to include exploratory data analysis, hypothesis testing, and the development of algorithms.
2. Skill Sets:
The skill sets required for data analysts and data scientists highlight the differences in their roles.
Data Analyst:
Data analysts need a strong foundation in statistical analysis, data cleaning, and proficiency in tools like Excel and SQL. While they may have some programming skills, they typically do not require the same level of expertise in machine learning. Visualization tools are a key part of their toolkit, enabling them to communicate insights effectively.
Data Scientist:
Data scientists require a more comprehensive skill set. They need a deep understanding of statistics, machine learning, and programming languages such as Python or R. Proficiency in data preprocessing, feature engineering, model selection, and evaluation is essential. Data scientists often work with big data technologies and possess advanced knowledge of analytical techniques, enabling them to create and implement complex algorithms.
3. Problem Complexity:
The complexity of problems tackled by data analysts and data scientists varies significantly.
Data Analyst:
Data analysts generally deal with well-defined problems and questions. Their focus is on providing answers to specific queries based on structured data. The insights they provide contribute to the day-to-day decision-making processes within an organization.
Data Scientist:
Data scientists thrive on addressing more complex and less structured problems. They engage in exploratory data analysis, hypothesis testing, and the creation of models capable of handling unstructured or semi-structured data. The solutions they develop often contribute to strategic decision-making, driving innovation, process optimization, and the creation of new products or services.
4. Business Impact:
The impact of data analysts and data scientists on an organization's decision-making processes is another area of distinction.
Data Analyst:
The insights provided by data analysts are integral to operational improvements and day-to-day decision-making. Their work contributes to the efficiency and effectiveness of ongoing processes within the organization.
Data Scientist:
Data scientists play a more strategic role in decision-making. Their insights have a broader impact on the organization, driving innovation, shaping long-term strategies, and contributing to the development of new products or services. The impact of a data scientist's work extends beyond routine operations, influencing the overall direction of the organization.
5. Educational Background:
The educational backgrounds of data analysts and data scientists reflect the differences in the complexity of their roles.
Data Analyst:
Data analysts may have a background in fields such as statistics, mathematics, economics, or business. While a bachelor's degree is often sufficient, some roles may require a master's degree.
Data Scientist:
Data scientists typically hold more advanced degrees, such as a master's or Ph.D., in fields like computer science, statistics, or data science. The nature of their work demands a deeper understanding of advanced mathematical and statistical concepts, as well as expertise in machine learning.
In conclusion, the divergence between data analysts and data scientists is profound, marked by distinctions in complexity, skill prerequisites, and organizational impact. Data analysts concentrate on offering actionable insights from existing data, enhancing day-to-day decision-making processes. In contrast, data scientists embark on tackling intricate issues, employing advanced analytics and machine learning to derive predictive and prescriptive insights that play a pivotal role in shaping an organization's strategic direction.As the demand for skilled professionals in these domains grows, the relevance of quality education becomes paramount. Choosing the best Data Science courses in Chennai is a crucial step in acquiring the necessary expertise for a successful career in the evolving landscape of data science.
3 notes
·
View notes
Text
Cracking the Code: Explore the World of Big Data Analytics
Welcome to the amazing world of Big Data Analytics! In this comprehensive course, we will delve into the key components and complexities of this rapidly growing field. So, strap in and get ready to embark on a journey that will equip you with the essential knowledge and skills to excel in the realm of Big Data Analytics.
Key Components
Understanding Big Data
What is big data and why is it so significant in today's digital landscape?
Exploring the three dimensions of big data: volume, velocity, and variety.
Overview of the challenges and opportunities associated with managing and analyzing massive datasets.
Data Analytics Techniques
Introduction to various data analytics techniques, such as descriptive, predictive, and prescriptive analytics.
Unraveling the mysteries behind statistical analysis, data visualization, and pattern recognition.
Hands-on experience with popular analytics tools like Python, R, and SQL.
Machine Learning and Artificial Intelligence
Unleashing the potential of machine learning algorithms in extracting insights and making predictions from data.
Understanding the fundamentals of artificial intelligence and its role in automating data analytics processes.
Applications of machine learning and AI in real-world scenarios across various industries.
Reasons to Choose the Course
Comprehensive Curriculum
An in-depth curriculum designed to cover all facets of Big Data Analytics.
From the basics to advanced topics, we leave no stone unturned in building your expertise.
Practical exercises and real-world case studies to reinforce your learning experience.
Expert Instructors
Learn from industry experts who possess a wealth of experience in big data analytics.
Gain insights from their practical knowledge and benefit from their guidance and mentorship.
Industry-relevant examples and scenarios shared by the instructors to enhance your understanding.
Hands-on Approach
Dive into the world of big data analytics through hands-on exercises and projects.
Apply the concepts you learn to solve real-world data problems and gain invaluable practical skills.
Work with real datasets to get a taste of what it's like to be a professional in the field.
Placement Opportunities
Industry Demands and Prospects
Discover the ever-increasing demand for skilled big data professionals across industries.
Explore the vast range of career opportunities in data analytics, including data scientist, data engineer, and business intelligence analyst.
Understand how our comprehensive course can enhance your prospects of securing a job in this booming field.
Internship and Job Placement Assistance
By enrolling in our course, you gain access to internship and job placement assistance.
Benefit from our extensive network of industry connections to get your foot in the door.
Leverage our guidance and support in crafting a compelling resume and preparing for interviews.
Education and Duration
Mode of Learning
Choose between online, offline, or blended learning options to cater to your preferences and schedule.
Seamlessly access learning materials, lectures, and assignments through our user-friendly online platform.
Engage in interactive discussions and collaborations with instructors and fellow students.
Duration and Flexibility
Our course is designed to be flexible, allowing you to learn at your own pace.
Depending on your dedication and time commitment, you can complete the course in as little as six months.
Benefit from lifetime access to course materials and updates, ensuring your skills stay up-to-date.
By embarking on this comprehensive course at ACTE institute, you will unlock the door to the captivating world of Big Data Analytics. With a solid foundation in the key components, hands-on experience, and placement opportunities, you will be equipped to seize the vast career prospects that await you. So, take the leap and join us on this exciting journey as we unravel the mysteries and complexities of Big Data Analytics.
5 notes
·
View notes
Text
Data Engineering Concepts, Tools, and Projects
All the associations in the world have large amounts of data. If not worked upon and anatomized, this data does not amount to anything. Data masterminds are the ones. who make this data pure for consideration. Data Engineering can nominate the process of developing, operating, and maintaining software systems that collect, dissect, and store the association’s data. In modern data analytics, data masterminds produce data channels, which are the structure armature.
How to become a data engineer:
While there is no specific degree requirement for data engineering, a bachelor's or master's degree in computer science, software engineering, information systems, or a related field can provide a solid foundation. Courses in databases, programming, data structures, algorithms, and statistics are particularly beneficial. Data engineers should have strong programming skills. Focus on languages commonly used in data engineering, such as Python, SQL, and Scala. Learn the basics of data manipulation, scripting, and querying databases.
Familiarize yourself with various database systems like MySQL, PostgreSQL, and NoSQL databases such as MongoDB or Apache Cassandra.Knowledge of data warehousing concepts, including schema design, indexing, and optimization techniques.
Data engineering tools recommendations:
Data Engineering makes sure to use a variety of languages and tools to negotiate its objects. These tools allow data masterminds to apply tasks like creating channels and algorithms in a much easier as well as effective manner.
1. Amazon Redshift: A widely used cloud data warehouse built by Amazon, Redshift is the go-to choice for many teams and businesses. It is a comprehensive tool that enables the setup and scaling of data warehouses, making it incredibly easy to use.
One of the most popular tools used for businesses purpose is Amazon Redshift, which provides a powerful platform for managing large amounts of data. It allows users to quickly analyze complex datasets, build models that can be used for predictive analytics, and create visualizations that make it easier to interpret results. With its scalability and flexibility, Amazon Redshift has become one of the go-to solutions when it comes to data engineering tasks.
2. Big Query: Just like Redshift, Big Query is a cloud data warehouse fully managed by Google. It's especially favored by companies that have experience with the Google Cloud Platform. BigQuery not only can scale but also has robust machine learning features that make data analysis much easier. 3. Tableau: A powerful BI tool, Tableau is the second most popular one from our survey. It helps extract and gather data stored in multiple locations and comes with an intuitive drag-and-drop interface. Tableau makes data across departments readily available for data engineers and managers to create useful dashboards. 4. Looker: An essential BI software, Looker helps visualize data more effectively. Unlike traditional BI tools, Looker has developed a LookML layer, which is a language for explaining data, aggregates, calculations, and relationships in a SQL database. A spectacle is a newly-released tool that assists in deploying the LookML layer, ensuring non-technical personnel have a much simpler time when utilizing company data.
5. Apache Spark: An open-source unified analytics engine, Apache Spark is excellent for processing large data sets. It also offers great distribution and runs easily alongside other distributed computing programs, making it essential for data mining and machine learning. 6. Airflow: With Airflow, programming, and scheduling can be done quickly and accurately, and users can keep an eye on it through the built-in UI. It is the most used workflow solution, as 25% of data teams reported using it. 7. Apache Hive: Another data warehouse project on Apache Hadoop, Hive simplifies data queries and analysis with its SQL-like interface. This language enables MapReduce tasks to be executed on Hadoop and is mainly used for data summarization, analysis, and query. 8. Segment: An efficient and comprehensive tool, Segment assists in collecting and using data from digital properties. It transforms, sends, and archives customer data, and also makes the entire process much more manageable. 9. Snowflake: This cloud data warehouse has become very popular lately due to its capabilities in storing and computing data. Snowflake’s unique shared data architecture allows for a wide range of applications, making it an ideal choice for large-scale data storage, data engineering, and data science. 10. DBT: A command-line tool that uses SQL to transform data, DBT is the perfect choice for data engineers and analysts. DBT streamlines the entire transformation process and is highly praised by many data engineers.
Data Engineering Projects:
Data engineering is an important process for businesses to understand and utilize to gain insights from their data. It involves designing, constructing, maintaining, and troubleshooting databases to ensure they are running optimally. There are many tools available for data engineers to use in their work such as My SQL, SQL server, oracle RDBMS, Open Refine, TRIFACTA, Data Ladder, Keras, Watson, TensorFlow, etc. Each tool has its strengths and weaknesses so it’s important to research each one thoroughly before making recommendations about which ones should be used for specific tasks or projects.
Smart IoT Infrastructure:
As the IoT continues to develop, the measure of data consumed with high haste is growing at an intimidating rate. It creates challenges for companies regarding storehouses, analysis, and visualization.
Data Ingestion:
Data ingestion is moving data from one or further sources to a target point for further preparation and analysis. This target point is generally a data storehouse, a unique database designed for effective reporting.
Data Quality and Testing:
Understand the importance of data quality and testing in data engineering projects. Learn about techniques and tools to ensure data accuracy and consistency.
Streaming Data:
Familiarize yourself with real-time data processing and streaming frameworks like Apache Kafka and Apache Flink. Develop your problem-solving skills through practical exercises and challenges.
Conclusion:
Data engineers are using these tools for building data systems. My SQL, SQL server and Oracle RDBMS involve collecting, storing, managing, transforming, and analyzing large amounts of data to gain insights. Data engineers are responsible for designing efficient solutions that can handle high volumes of data while ensuring accuracy and reliability. They use a variety of technologies including databases, programming languages, machine learning algorithms, and more to create powerful applications that help businesses make better decisions based on their collected data.
2 notes
·
View notes
Text
Best colleges for BCA in Artificial Intelligence & Machine Learning
BCA in Artificial Intelligence & Machine Learning: Starting the Journey into AI & ML
Artificial Intelligence (AI) and Machine Learning (ML) have become crucial technologies across various industries. They have changed the way we work, and interact with technology. Pursuing a Bachelor of Computer Applications (BCA) in Artificial Intelligence and Machine Learning meets the growing demand for professionals who possess a strong foundation in both AI and ML.
In this article, we will explore the significance of BCA in Artificial Intelligence and Machine Learning and how it can shape your career.
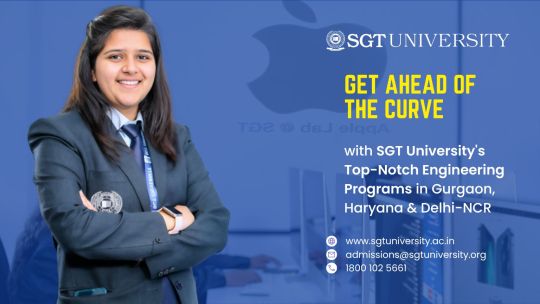
Introduction to BCA in Artificial Intelligence & Machine Learning
BCA in Artificial Intelligence and Machine Learning is a 3 year UG course that combines computer science with AI and ML concepts. It is designed to provide students with a comprehensive understanding of the theoretical foundations and practical applications of AI and ML technologies.
This program equips students with the skills required to develop intelligent systems, analyze complex data sets, and build predictive models using ML algorithms.
BCA in AI & ML Syllabus
The curriculum of BCA in Artificial Intelligence and Machine Learning is carefully crafted to provide students with a strong foundation in computer science, programming, mathematics, and statistics. Additionally, it includes specialized courses in AI and ML, covering topics such as:
Data Structures and Algorithms
Probability and Statistics
Data Mining and Data Warehousing
Deep Learning
Natural Language Processing
Computer Vision
Reinforcement Learning
Big Data Analytics
Cloud Computing
Learn more about the complete BCA in AI and ML syllabus at SGT University.
Job Opportunities for BCA in AI & ML Graduates
Upon completing BCA in Artificial Intelligence and Machine Learning, graduates can explore various career opportunities in both established companies and startups. Some of the common jobs in this field include:
AI Engineer
Machine Learning Engineer
Data Scientist
Business Intelligence Analyst
AI Researcher
Robotics Engineer
Data Analyst
Software Developer
Data Engineer
Salary Potential
BCA graduates in Artificial Intelligence and Machine Learning can expect competitive salaries due to the high demand for AI and ML professionals. Entry-level positions typically offer salaries ranging from 6 to 8 LPA according to Upgrad.
Future Scope of BCA in Artificial Intelligence & Machine Learning
The future scope of BCA in Artificial Intelligence and Machine Learning is promising.
As AI and ML continue to advance and permeate various sectors, the demand for skilled professionals in this field will only increase.
Industries such as healthcare, finance, retail, manufacturing, and transportation are actively adopting AI and ML technologies, creating a wealth of opportunities for BCA graduates.
How to Excel in Artificial Intelligence and Machine Learning Studies
To excel in BCA studies, follow these tips:
Stay Updated: Keep up with the latest advancements in AI and ML through academic journals, conferences, and online resources.
Practice Coding: Develop proficiency in programming languages commonly used in AI and ML, such as Python and R.
Hands-on Projects: Engage in practical projects to apply theoretical knowledge and build a strong portfolio.
Collaborate and Network: Join AI and ML communities, attend meetups, and participate in hackathons to collaborate with peers and learn from experts.
Continuous Learning: Embrace continuous learning to stay relevant in the rapidly evolving field of AI and ML.
Why Study BCA in Artificial Intelligence and Machine Learning from SGT University?
The following reasons make SGT University the best colleges for BCA in Artificial Intelligence & Machine Learning:
A future-proof career in technology.
Specialization in AI and ML.
Expertise in cutting-edge technologies.
Strong industry demand for graduates.
Access to renowned faculty and resources.
Networking with industry professionals.
Gateway to innovation and research.
Conclusion
BCA in Artificial Intelligence and Machine Learning offers a comprehensive education that combines computer science with AI and ML concepts.
With the increasing demand for AI and ML professionals, pursuing BCA in this domain can open up exciting career opportunities and provide a strong foundation for future growth.
Enroll now at SGT University to learn this course.
#Artificial Intelligence#Machine Learning#Ai#Bachelor of Computer Applications#BCA in Artificial Intelligence and Machine Learning#best colleges for BCA in Artificial Intelligence & Machine Learning
2 notes
·
View notes
Text
Techyse Education in Marathahalli, Bangalore, offers specialized Data Analytics Training in Marathahalli for individuals looking to build expertise in Python, Power BI, and data analysis techniques. Their industry-aligned courses focus on practical learning through real-world projects, ensuring students gain hands-on experience in data manipulation, visualization, and dashboard creation. Whether you are a beginner or an experienced professional, Techyse’s programs are designed to enhance your skill set, making you job-ready for roles in data analytics.
Comprehensive Data Analytics Training in Marathahalli Techyse Education takes pride in delivering high-quality Data Analytics Training in Marathahalli, backed by experienced instructors with deep industry knowledge. The curriculum covers essential tools and techniques, from data wrangling with Python to creating interactive dashboards using Power BI, ensuring students are prepared to meet industry demands. With personalized mentorship, career support, and placement assistance, Techyse provides a well-rounded learning experience. Whether aiming for career growth or a fresh start in data analytics, Techyse Education equips learners with the skills to excel in a competitive job market.
Techyse Education | Data Analyst, Python, Power BI Training in Marathahalli, Bangalore
18, Krishna Summit, 307, 3rd Floor, Aswath Nagar, Next to Canara Bank, Marathahalli, Bangalore, Karnataka 560037
Phone: 098445 14333 Website : https://techyse.in/
Our Google Map Location is : https://maps.app.goo.gl/dLsBM669nKHTutxu9
Follow us: Facebook : https://www.facebook.com/techyse.education/ Twitter: https://x.com/techyse_edu/ Instagram : https://www.instagram.com/techyeseducation/ LinkedIn : https://www.linkedin.com/company/techyse-education/ Youtube: https://www.youtube.com/@TechyseEducation
#Data Analytics Marathahalli#Analytics Training#Data Science Marathahalli#Big Data Training#Data Analytics Course#Learn Data Analytics#Marathahalli Training#Data Science Bangalore#Analytics Experts#Data Analyst Training#Data Skills#Marathahalli Courses#Data Analytics India#Bangalore Training#Data Visualization#SQL Training#Python for Data Analytics#Machine Learning Training#Data Science Bootcamp#Business Intelligence#AI and Analytics#Learn Data Science#Career in Analytics#Analytics Certification#Marathahalli Academy#Data Driven#Advanced Analytics#Real World Analytics#Data Analytics Hub#Data Science Courses Bangalore
0 notes
Text
Top Australian Universities for Cloud Computing and Big Data
In the age of digital transformation, data is the new oil; cloud computing is the infrastructure fuelling its refining. Big data and cloud computing together have created a dynamic ecosystem for digital services, business intelligence, and innovation generation. As industries shift towards cloud-first policies and data-driven decision-making, the demand for qualified individuals in these areas has increased. Known for its strong academic system and industry-aligned education, Australia offers excellent opportunities for foreign students to concentrate in Cloud Computing and Big Data. This post will examine the top Australian universities leading the way in these technical domains.
Why Study Cloud Computing and Big Data in Australia?
Ranked globally, Australia's universities are well-equipped with modern research tools, industry ties, and hands-on learning environments. Here are some fascinating reasons for learning Cloud Computing and Big Data in Australia:
Global Recognition:Ranked among the top 100 globally, Australian universities offer degrees recognised all around.
Industry Integration: Courses typically include capstone projects and internships as well as research collaborations with tech behemoths such as Amazon Web Services (AWS), Google Cloud, Microsoft Azure, and IBM.
High Employability: Graduates find decent employment in sectors including government, telecommunications, healthcare, and finance.
Post-Study Work Opportunities:Australia offers post-study work visas allowing foreign graduates to gain practical experience in the country.
Core Topics Covered in Cloud Computing and Big Data Courses
Courses in these fields typically cover:
Cloud Architecture & Security
Distributed Systems & Virtualization
Big Data Analytics
Machine Learning
Data Warehousing
Cloud Services (AWS, Google Cloud, Azure)
DevOps & Infrastructure Automation
Real-Time Data Processing (Apache Spark, Kafka)
Python, R, SQL, and NoSQL Databases
Top Australian Universities for Cloud Computing and Big Data
1. University of Melbourne
The University of Melbourne offers courses such the Master of Data Science and Master of Information Technology with a Cloud Computing emphasis. Renowned for its research excellence and global standing, the university emphasises a balance between fundamental knowledge and pragmatic cloud infrastructure training. Students benefit from close relationships with industry, including projects with AWS and Google Cloud, all run from its Parkville campus in Melbourne
2. University of Sydney
Emphasising Cloud Computing and Data Management, the University of Sydney provides the Master of Data Science and the Master of Information Technology. Its comprehensive course provides students information in data mining, architecture, and analytics. Internships and cooperative research in the heart of Sydney's Camperdown campus supported by the Sydney Informatics Hub allow students to engage with industry.
3. Monash University
Monash University offers a Master of Data Science as well as a Master of Information Technology concentrating in Enterprise Systems and Cloud Computing. Known for its multidisciplinary and practical approach, Monash mixes cloud concepts with artificial intelligence, cybersecurity, and IoT. Students located at the Melbourne Clayton campus have access to modern laboratories and industry-aligned projects.
4. University of New South Wales (UNSW Sydney)
University of New South Wales (UNSW Sydney) students can choose either the Master of Data Science and Decisions or the Master of IT. Under a curriculum covering distributed systems, networking, and scalable data services, UNSW provides practical training and close ties with Microsoft, Oracle, and other world players. The Kensington campus keeps a vibrant tech learning environment.
5. Australian National University (ANU)
The Australian National University (ANU), based in Canberra, offers the Master of Computing and the Master of Machine Learning and Computer Vision, both addressing Big Data and cloud tech. ANU's strength lies in its research-driven approach and integration of data analysis into scientific and governmental applications. Its Acton campus promotes high-level research with a global vi
6. University of Queensland (UQ)
The University of Queensland (UQ) offers the Master of Data Science as well as the Master of Computer Science with a concentration in Cloud and Systems Programming. UQ's courses are meant to include large-scale data processing, cloud services, and analytics. The St. Lucia campus in Brisbane also features innovation centres and startup incubators to enable students develop useful ideas.
7. RMIT University
RMIT University provides the Master of Data Science and the Master of IT with Cloud and Mobile Computing as a specialisation. RMIT, an AWS Academy member, places great importance on applied learning and digital transformation and provides cloud certifications in its courses. Students learn in a business-like environment at the centrally located Melbourne City campus.
8. University of Technology Sydney (UTS)
University of Technology Sydney (UTS) sets itself apart with its Master of Data Science and Innovation and Master of IT with Cloud Computing specialisation. At UTS, design thinking and data visualisation receive significant attention. Located in Ultimo, Sydney, the university features a "Data Arena" allowing students to interact with big-scale data sets visually and intuitively.
9. Deakin University
Deakin University offers a Master of Data Science as well as a Master of Information Technology with Cloud and Mobile Computing. Deakin's courses are flexible, allowing on-campus or online study. Its Burwood campus in Melbourne promotes cloud-based certifications and wide use of technologies including Azure and Google Cloud in course delivery.
10. Macquarie University
Macquarie University provides the Master of Data Science and the Master of IT with a Cloud Computing and Networking track. Through strong integration of cloud environments and scalable systems, the Macquarie Data Science Centre helps to foster industry cooperation. The North Ryde campus is famous for its research partnerships in smart infrastructure and public data systems.
Job Roles and Career Opportunities
Graduates from these programs can explore a wide range of roles, including:
Cloud Solutions Architect
Data Scientist
Cloud DevOps Engineer
Big Data Analyst
Machine Learning Engineer
Cloud Security Consultant
Database Administrator (Cloud-based)
AI & Analytics Consultant
Top Recruiters in Australia:
Amazon Web Services (AWS)
Microsoft Azure
Google Cloud
IBM
Atlassian
Accenture
Commonwealth Bank of Australia
Deloitte and PwC
Entry Requirements and Application Process
While specifics vary by university, here are the general requirements:
Academic Qualification: Bachelor’s degree in IT, Computer Science, Engineering, or a related field.
English Proficiency: IELTS (6.5 or above), TOEFL, or PTE.
Prerequisites:Some courses might need knowledge of statistics or programming (Python, Java).
Documents NeededSOP, academic transcripts, CV, current passport, and letters of recommendation.
Intakes:
February and July are the most common intakes.
Final Thoughts
Given the growing global reliance on digital infrastructure and smart data, jobs in Cloud Computing and Big Data are not only in demand but also absolutely essential. Australian universities are driving this transformation by offering overseas students the chance to learn from the best, interact with real-world technologies, and boldly enter global tech roles. From immersive courses and knowledgeable professors to strong industry ties, Australia provides the ideal launchpad for future-ready tech professionals.
Clifton Study Abroad is an authority in helping students like you negotiate the challenging road of overseas education. Our experienced advisors are here to help you at every turn, from choosing the right university to application preparation to getting a student visa. Your future in technology starts here; let us help you in opening your perfect Cloud Computing and Big Data job.
Are you looking for the best study abroad consultants in Kochi
#study abroad#study in uk#study abroad consultants#study in australia#study in germany#study in ireland#study blog
0 notes
Text
How Data Science is Making Waves in Tech and Beyond

Introduction
In the digital age, data has emerged as the new oil, fueling innovations across industries. Data science, the interdisciplinary field that extracts meaningful insights from vast datasets, is at the forefront of this transformation. From enhancing business strategies to revolutionizing healthcare, data science is making significant strides in tech and beyond.
The Pervasive Impact of Data Science
Data science's influence spans various sectors:
Healthcare: Predictive analytics assist in early disease detection and personalized treatment plans.
Finance: Algorithmic trading and fraud detection systems rely heavily on data-driven models.
Retail: Customer behavior analysis informs inventory management and personalized marketing.
Manufacturing: Predictive maintenance reduces downtime and optimizes operations.
These applications underscore the versatility and necessity of data science in today's world.
Essential Data Science Tools
To harness the power of data, professionals utilize a suite of data science tools:
Programming Languages: Python and R are staples for data manipulation and statistical analysis.
Data Visualization: Tools like Tableau and Power BI transform complex data into intuitive visuals.
Machine Learning Frameworks: TensorFlow and scikit-learn enable the development of predictive models.
Big Data Technologies: Apache Spark and Hadoop facilitate the processing of massive datasets.
Proficiency in these tools is crucial for any aspiring data scientist.
The Importance of Data Science Training
Given the field's rapid evolution, continuous learning is imperative. Structured data science training programs offer:
Comprehensive Curriculum: Covering statistics, machine learning, and data engineering.
Hands-On Experience: Real-world projects that simulate industry challenges.
Expert Guidance: Mentorship from seasoned professionals.
Such training equips individuals with the skills needed to excel in data-driven roles.
PyNet Labs: Your Partner in Data Science Education
PyNet Labs, based in NCR, Delhi, India, specializes in IT and networking training. Recognizing the growing demand for data science expertise, PyNet Labs offers comprehensive data science training with 100% job placement designed to:
Build Foundational Knowledge: Covering essential concepts in statistics and programming.
Develop Practical Skills: Through hands-on projects and case studies.
Prepare for Industry Certifications: Aligning with global standards.
With a focus on quality education and practical experience, PyNet Labs empowers students to thrive in the data-driven landscape.
Future Trends in Data Science
As technology advances, data science continues to evolve:
Artificial Intelligence Integration: Enhancing predictive capabilities and automating complex tasks.
Edge Computing: Processing data closer to the source for real-time insights.
Ethical Data Practices: Emphasizing transparency and accountability in data usage.
Staying abreast of these trends is essential for professionals aiming to remain competitive.
FAQs
What industries benefit most from data science?
Data science enhances sectors like healthcare (early disease detection), finance (fraud detection), retail (customer behavior analysis), and manufacturing (predictive maintenance).
2. Which tools are essential for data science?
Key tools include:
Programming: Python, R
Visualization: Tableau, Power BI
Machine Learning: TensorFlow, scikit-learn
Big Data: Apache Spark, Hadoop
3. Why is continuous data science training important?
Ongoing training keeps professionals updated with evolving technologies, ensuring they remain competitive and proficient in the field.
4. How does PyNet Labs support data science learners?
PyNet Labs offers a comprehensive Data Science course covering Python, Machine Learning, Deep Learning, AI, and Data Analytics, ensuring mastery of in-demand skills with 100% job placement.
5. What are the future trends in data science?
Emerging trends include:
AI Integration: Enhancing predictive capabilities
Edge Computing: Processing data closer to the source
Ethical Data Practices: Ensuring transparency and accountability
Conclusion
Data science is undeniably reshaping industries and redefining the way we interpret information. With the right tools and training, individuals can unlock new opportunities and drive innovation. Institutions like PyNet Labs play a pivotal role in cultivating the next generation of data scientists, ensuring a future where data-driven decision-making is the norm.
1 note
·
View note
Text
Top IT skills in very high demand in 2025

The technology world changes at the drop of a hat, and keeping up with the times is important for a successful career in the IT industry. It is very important to consider what skills the market will be demanding in the year 2025. Knowledge of these in-demand skills can help you align your career in the right way and stay competitive. Among many other IT skills, here are the prominent skills that will be edging towards high demand in 2025.
1. Artificial Intelligence and Machine Learning: Becoming the Future of Intelligence
What was once a mere concept is now rooted in reality and impacting, with applications ranging from personalized user experience design to the next level of automation. Companies around the world are resorting to AI and ML on an increasing scale for driving innovation; enhancing efficiency; and analyzing data for useful insights.
Why are they so much in demand? AI and ML are automating processes across industries, providing an optimisation of business processes and assisting in the creation of newer products and services. There exists a huge demand for professionals who can design, develop and operate AI/ML systems.
Key skills to develop: The applications of machine learning algorithms, deep learning abilities, natural language processing (NLP), computer vision, data modelling on top of programming languages such as Python and R.
2. Data Science and Analytics: Extracting Value from Information
In this instruction era of Big Data, the ability to analyse interpretable information, forming a chain of actions and designing experimentations, is a vital skill. Data scientists and analysts are indispensable in situations where organisations need to make informed decisions, identify trends, and get a competitive edge.
Why does it see demand? Data volume generated is growing exponentially. Organisations require such specialists who could collect, clean, analyse, visualise, and present data.
Key skills to focus on: statistical analysis, data visualisation techniques, data mining, basic concepts of machine learning (this is very closely related to data science), and tools and programming languages such as Python, SQL, and various data visualisation libraries.
3. Cybersecurity: Protecting the digital ecosystem
The need for cybersecurity professionals has been growing with the increasing instances and sophistication of cyber threats. Cybersecurity professionals ensure the safeguarding of sensitive information, critical infrastructure, and the issuance of trust.
Reason for demand: As more people create a digital footprint, more vulnerabilities emerge across cyberspace. Defense costs are very high. Minimizing the risks then creates the need for specialists with the skills to intercept security incidents before they occur, identify incidents fast in time, and put forth professional responses to such incidents.
Skills to focus on: Network security, ethical hacking, penetration testing, risk assessment and management, cloud security, data privacy laws, security frameworks, and compliance standards.
4. Cloud Computing: The Legitimate Infrastructure of Modern IT
With the likes of AWS, Microsoft Azure, and GCP, cloud platforms had made being the modern IT infrastructure for majority of operations. Scaling, flexibility, and cost-effectiveness have ensured these cloud solutions get a yet greater adoption.
Reasons for such demand: More and more organizations now transfer their applications and data into the cloud, thus creating a huge demand for individuals who can architect, deploy, manage, and secure cloud-based environments.
Key skills to be aware of: Cloud architecture and design, cloud security best practices, DevOps, containerization technologies (Docker, Kubernetes), serverless computing, and specific knowledge of cloud platforms.
5. Software Development and Programming:
Software development and programming will always be the backbone of the IT industry. Skilled developers are in great demand as companies build their solutions, maintain these solutions, and innovate digital solutions.
The reason for such demand? Almost every sector requires custom software solutions in this technology-driven world. Service to create new solutions, maintain existing ones, and resolve technical challenges are okay for the skillful programmers.
Key skills to Concentrate on: Various programming languages (Python, Java, JavaScript, C++, C#), software development life cycle (SDLC), algorithms and data structures, software architecture, testing and debugging, and some domain or technology expertise (mobile development, web development).
Conclusion
These are some of the leading IT skills of highest demand in 2025. By investing in the development of the above-mentioned skills, individuals can significantly improve the job market potential and set themselves on the way toward being industry-ready in the face of changing scenarios in the IT world. Continuous learning and constant adaptation will become necessary in remaining relevant and in making full use of these demanded skill sets.
Contact us
Call now on +91 9825618292
Visit Our Website: http://tccicomputercoaching.com/
0 notes