#combinatorial testing
Explore tagged Tumblr posts
Note
hi! I love your blog!
Can you maybe write a Jameson hawthorne x reader fic based off of I can see you by Taylor swift? If not it’s totally fine
i can see you
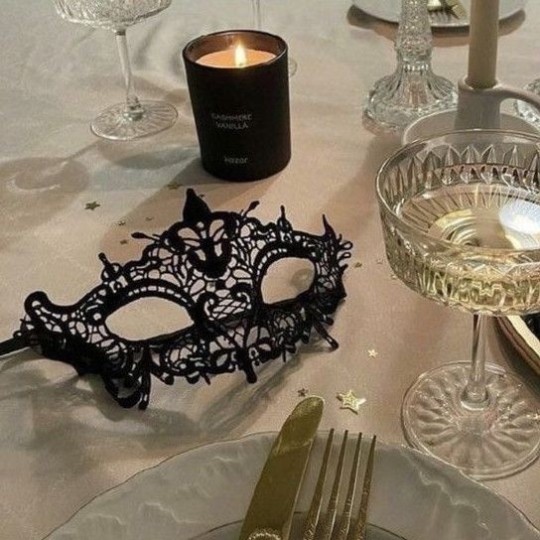
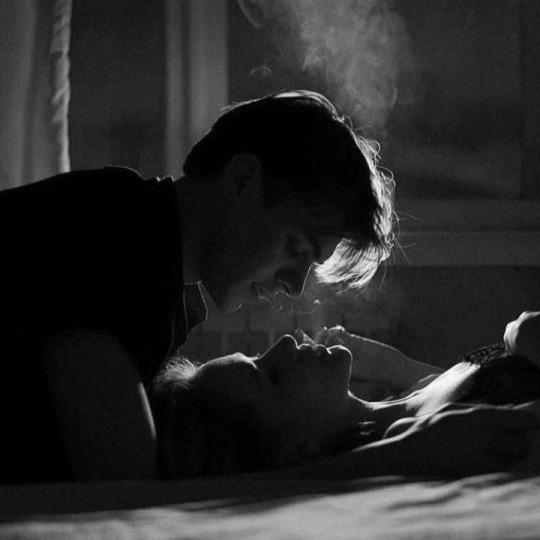

pairing - jameson hawthorne x fem!reader.
summary - a friendship becomes so much more, but it's not for other eyes to see.
warnings - none, just kissing and love.
navigation | masterlist | request | taglist
a/n: I AM SO SORRYYYYY, THIS TOOOK SOOOOO LOOONG TO WRITE. i just had no motivation or inspiration, but i will be doing other requests, too.
i've been watchin' you for ages
and i spend my time tryin' not to feel it
"you should just finally gather the courage and say it," y/n's classmate, lucy, spoke, beside her, "you're just torturing yourself."
the girl removed her gaze from him and looked at her, "absolutely not," she closed her locker, "and i'm getting over it, so just stop talking about him."
"then stop staring at him every time when he's in your view."
y/n rolled her eyes. but mostly because of how stupid her heart was.
she tried. she really did. to stop the warm feeling, ambraising her every time he was near. or to get rid of the goosebumps that appeared when his touch scarred her skin. or the desire to throw their friendship away just to feel his lips on hers.
it was like every time she saw him, she could imagine it. his arms around her. his lips on hers. their bodies pressed against each other up against the wall.
but it was all just a fantasy. a desire.
"let's go, or we will be late," she walked to class with lucy, not feeling the green eyes locked on her back. the same green eyes she fantasizes about all the time.
and we kept everything professional
but something's changed, it's somethin' i, i like
popular wasn't the word that you would describe jameson hawthorne as or the other hawthorne's. he was a dangerous threat. his mind a powerful weapon. jameson hawthorne was a boy with a different view to the world - it being a game. a riddle.
maybe that was the reason for people respecting him and his family. because of the wealth, reputation, and the name. or maybe it was because jameson hawthorne was a wanted treat, on whom all the eyes laid.
but like they didn't know a lot of things about the boy, they didn't also know one of the biggest secrets that hid behind his green eyes - that he was trying to move a piece on the board towards something frightening but exciting.
so, just like that, friendly conversations with flirty comments turned to secret touches and heated kisses.
it was important for jameson to keep the relationship as a secret. for a hawthorne grandson with a reputation so big it was a risk to show his private life to the public. the boy was scared that the talks and whispers would destroy something so magnificent and special. jameson hawthorne was scared that by making a mistake, she would get hurt, and he couldn't help.
'Cause I can see you waitin' down the hall from me
And I could see you up against the wall with me
his lips were on hers as soon as he pulled her into an empty classroom, his hands on her hips.
every time y/n was with jameson, she could feel her heart pound. the girl could feel the adrenaline rush through her body. with the hawthorne, she felt free. euphoric.
"i have maths in ten minutes," y/n murmured against his lips as they slowly backed away.
"mhm."
as soon as her thighs hit the desk, jameson lifted her up and stepped in between her legs. his lips still on hers.
her hand found its way to his hair, "we're revising for a test."
jameson started preparing light kisses along her jaw, while his thumb drew small circles on her thigh.
"it's very import-"
"i swear to god, if you don't shut up about studying for once, i'll drop you out from school," jameson finally spoke as he looked into her eyes.
the girl giggled.
"you'll do great, y/n/n, i know you will," he leaned in and kissed her neck, "and if you won't i'll help, but i think either way you'll need me, beacuse you're trash at combinatory."
the girl pushed him back with a shocked smile, "you just said that i'll do great!"
"didn't want to tell the hard truth," he smirked.
"jerk," y/n rolled her eyes while smiling.
"yeah, but you love me, and i love you, so you can't stay mad at me," he saw her grin and gave her a small kiss, "i love your smile."
y/n's stomach filled with butterflies, and she pulled jameson in by the neck as she connected their lips.
Oh, I see you, I see you, baby
I see you
"he's looking," lucy spoke.
y/n felt her cheeks heat up, but she continued doing her homework.
"what is he even doing in a library, doesn't he have important hawthorne stuff to do?"
"like what?"
"like getting the arrogant stick out of his ass?"
the girl snorted, which caused other people to look at her, "that's graysons job, not jamesons, " she whispered, ignoring the people.
before lucy could respond, y/n's phone vibrated. she checked and saw a message from a someone.
'i would love to hear that laugh again. meet me at midnight?''
'you actually are a shit'
'i love you too and i'll take that as a yes.'
y/n couldn't help the smile that appeared on her face. that boy was gonna be the death of her.
"it's him, isn't he?"
"what? no?" she put the phone down, and resumed to her work.
lucy chuckled, "gurl, you're a terrible liar."
y/n laughed and bumped the girl with her shoulder, "shut up."
taglist: @noaboacoa @k-pevensie28 @mochamvgz @formulalina15
#jameson hawthorne imagine#jameson winchester hawthorne#jameson hawthorne#jameson hawthorne x reader#jameson hawthorne x you#jameson hawthorne x y/n#the inheritance games#the hawthorne legacy#the final gambit#the hawthorne brothers#taylor swift#i can see you#jameson hawthorne fluff#booktok#grayson hawthorne#nash hawthorne#xander hawthorne#avery grambs
112 notes
·
View notes
Text
Undergrad research blast from the past. Here I am in 2020 assembling a micro fluidic flow cell with a gold electrode block. I think I took this video for myself so I knew what to clip to what. This was when I worked with electrochemical sensors, transducing signals via impedance spectroscopy.
A lot of electrochemical techniques rely on measuring voltages or currents, but in this lab we looked at impedance- which is a fancy combination of regular resistance (like the same one from ohms law) and the imaginary portion of the resistance that arises from the alternating current we supply.
I would functionalize different groups on the gold working electrode by exposing the surface to a solution of thiolated biomarker capture groups. Thiols love to form self-assembled mono layers over gold, so anything tagged with thiol ends up sticking. [Aside: Apparently after I left the group they moved away from gold thiol interactions because they weren't strong enough to modify the electrode surface in a stable and predictable way, especially if we were flowing the solution over the surface (which we wanted to do for various automation reasons)]. The capture groups we used were various modified cyclodextrins- little sugar cups with hydrophobic pockets inside and a hydrophilic exterior. Cyclodextrins are the basis of febreeze- a cyclodextrin spray that captures odor molecules in that hydrophobic pocket so they can't interact with receptors in your nose. We focused on capturing hydrophobic things in our little pocket because many different hydrophobic biomarkers are relevant to many different diseases, but a lot of sensors struggle to interact with them in the aqueous environment of bodily fluids.
My work was two fold:
1) setting up an automated system for greater reproducibility and less human labor. I had to figure out how to get my computer, the potentiostat (which controls the alternating current put in, and reads the working electrode response), the microfluidic pump, and the actuator that switched between samples to all talk to each other so I could set up my solutions, automatically flow the thiol solution for an appropriate time and flow rate to modify the surface, then automatically flow a bio fluid sample (or rather in the beginning, pure samples of specific isolated biomarkers, tho their tendency to aggregate in aqueous solution may have changed the way they would interact with the sensor from how they would in a native environment, stabilized in blood or urine) over the electrode and cue the potentiostat for multiple measurements, and then flow cleaning solutions to clean out the tubings and renew the electrode. This involved transistor level logic (pain) and working with the potentiostat company to interact with their proprietary software language (pain) and so much dicking around with the physical components.
2) coming up with new cyclodextrin variants to test, and optimizing the parameters for surface functionalization. What concentrations and times and flow rates to use? How do different groups around the edge of the cyclodextrin affect the ability to capture distinct classes of neurotransmitters? I wasn't working with specific sensors, I was trying to get cross reactivity for the purpose of constructing nonspecific sensor arrays (less akin to antibody/antigen binding of ELISAs and more like the nonspecific combinatorial assaying you do with receptors in your tongue or nose to identify "taste profiles" or "smell profiles"), so I wanted diverse responses to diverse assortments of molecules.
Idk where I'm going with this. Mostly reminiscing. I don't miss the math or programming or the physical experience of being at the bench (I find chemistry more "fun") but I liked the ultimate goal more. I think cross reactive sensor arrays and principle component analysis could really change how we do biosample testing, and could potentially be useful for defining biochemical subtypes of subjectively defined mental illnesses.... I think that could (maybe, possibly, if things all work and are sufficiently capturing relevant variance in biochemistry from blood or piss or sweat or what have you) be a more useful way to diagnose mental illness and correlate to possible responses to medications than phenotypic analysis/interviews/questionnaires/trial and error pill prescribing.
4 notes
·
View notes
Text
Qoro Quantum And CESGA For Distributed Quantum Simulation

Qoro Quantum
Qoro Quantum and CESGA represent distributed quantum circuits with high-performance computing. Using Qoro Quantum's orchestration software and CESGA's CUNQA emulator, a test study showed scalable, distributed quantum circuit simulations over 10 HPC nodes. To assess distributed VQE and QAOA implementations, Qoro's Divi software built and scheduled thousands of quantum circuits for simulation on CESGA's infrastructure.
VQE and QAOA workloads finished in less than a second, demonstrating that high-throughput quantum algorithm simulations may be done with little code and efficient resources.
The pilot proved that distributed emulators like CUNQA can prepare HPC systems for large-scale quantum computing deployments by validating hybrid quantum-classical operations.
A pilot research from the Galician Supercomputing Centre (CESGA) and Qoro Quantum reveals how high-performance computing platforms may facilitate scalable, distributed quantum circuit simulations. A Qoro Quantum release said the two-week collaboration involved implementing Qoro's middleware orchestration platform to execute distributed versions of the variational quantum eigensolver and quantum approximate optimisation algorithm across CESGA's QMIO infrastructure.
Quantum Workload Integration and HPC Systems
Qoro's Divi quantum application layer automates hybrid quantum-classical algorithm orchestration and parallelisation. Divi created and ran quantum workloads on 10 HPC nodes using CESGA's CUNQA distributed QPU simulation framework for the pilot.
The announcement states that CESGA's modular testbed CUNQA mimics distributed QPU settings with customisable topologies and noise models. Qoro's technology might simulate quantum workloads in a multi-node setup to meet the demands of emerging hybrid quantum-HPC systems.
Everything worked perfectly, communication went well, and end-to-end functionality worked as intended.
Comparing QAOA and VQE in Distributed HPC
The variational hybrid approach VQE is used to estimate the ground-state energy of quantum systems, a major problem in quantum chemistry. Qoro and CESGA modelled a hydrogen molecule using two ansätze Hartree-Fock and Unitary Coupled Cluster Singles and Doubles in this pilot. Divi made 6,000 VQE circuits based on 20 bond length values.
With 10 computational nodes, the CUNQA emulator investigated the ansatz parameter space via Monte Carlo optimisation. Qoro says it replicated full demand in 0.51 seconds. Data collected automatically and returned for analysis show that the platform can enable high-throughput testing with only 15 lines of Divi code.
The researchers also evaluated QAOA, a quantum-classical technique for Max-Cut and combinatorial optimisation. This data clustering, circuit design, and logistics challenge involves partitioning a graph to maximise edges between two subgroups.
A 150-node network was partitioned into 15 clusters for simulation, and Qoro's Divi software built Monte Carlo parameterised circuits.Tests included 21,375 circuits in 15.44 seconds and 2,850 circuits in 2.13 seconds. The quantum-classical cut size ratio grew from 0.51 to 0.65 with sample size. The CUNQA emulator ran all circuits in parallel again utilising CESGA's architecture.
Performance, Infrastructure, and Prospects
Several pilot research results demonstrate scalable hybrid quantum computing advances. According to the Qoro Quantum release, Qoro's orchestration platform and CESGA's distributed quantum emulator provided faultless communication between the simulated QPU infrastructure and application layer. The cooperation also demonstrated how Qoro's Divi software could automatically generate and plan enormous quantum workloads, simplifying complex quantum applications.
The experiment also shown that distributed execution of hybrid quantum-classical algorithms over several HPC nodes may enhance performance without much human setup. Finally, the pilot showed key technological elements for scaling quantum workloads in high-performance computing. These insights will inform future distributed quantum system design.
Simulating distributed quantum architectures shows how HPC infrastructure might manage future quantum workloads. Qoro Quantum and CESGA plan to improve this method to enable quantum computing in large classical contexts.
CUNQA is being established as part of Quantum Spain with EU and Spanish Ministry for Digital Transformation support. ERDF_REACT EU funded this project's QMIO infrastructure for COVID-19 response.
#QoroQuantum#QuantumQoro#QAOA#CESGA#quantumcircuit#CUNQA#technology#TechNews#technologynews#news#govindhtech
0 notes
Text
Researchers teach LLMs to solve complex planning challenges
New Post has been published on https://sunalei.org/news/researchers-teach-llms-to-solve-complex-planning-challenges/
Researchers teach LLMs to solve complex planning challenges

Imagine a coffee company trying to optimize its supply chain. The company sources beans from three suppliers, roasts them at two facilities into either dark or light coffee, and then ships the roasted coffee to three retail locations. The suppliers have different fixed capacity, and roasting costs and shipping costs vary from place to place.
The company seeks to minimize costs while meeting a 23 percent increase in demand.
Wouldn’t it be easier for the company to just ask ChatGPT to come up with an optimal plan? In fact, for all their incredible capabilities, large language models (LLMs) often perform poorly when tasked with directly solving such complicated planning problems on their own.
Rather than trying to change the model to make an LLM a better planner, MIT researchers took a different approach. They introduced a framework that guides an LLM to break down the problem like a human would, and then automatically solve it using a powerful software tool.
A user only needs to describe the problem in natural language — no task-specific examples are needed to train or prompt the LLM. The model encodes a user’s text prompt into a format that can be unraveled by an optimization solver designed to efficiently crack extremely tough planning challenges.
During the formulation process, the LLM checks its work at multiple intermediate steps to make sure the plan is described correctly to the solver. If it spots an error, rather than giving up, the LLM tries to fix the broken part of the formulation.
When the researchers tested their framework on nine complex challenges, such as minimizing the distance warehouse robots must travel to complete tasks, it achieved an 85 percent success rate, whereas the best baseline only achieved a 39 percent success rate.
The versatile framework could be applied to a range of multistep planning tasks, such as scheduling airline crews or managing machine time in a factory.
“Our research introduces a framework that essentially acts as a smart assistant for planning problems. It can figure out the best plan that meets all the needs you have, even if the rules are complicated or unusual,” says Yilun Hao, a graduate student in the MIT Laboratory for Information and Decision Systems (LIDS) and lead author of a paper on this research.
She is joined on the paper by Yang Zhang, a research scientist at the MIT-IBM Watson AI Lab; and senior author Chuchu Fan, an associate professor of aeronautics and astronautics and LIDS principal investigator. The research will be presented at the International Conference on Learning Representations.
Optimization 101
The Fan group develops algorithms that automatically solve what are known as combinatorial optimization problems. These vast problems have many interrelated decision variables, each with multiple options that rapidly add up to billions of potential choices.
Humans solve such problems by narrowing them down to a few options and then determining which one leads to the best overall plan. The researchers’ algorithmic solvers apply the same principles to optimization problems that are far too complex for a human to crack.
But the solvers they develop tend to have steep learning curves and are typically only used by experts.
“We thought that LLMs could allow nonexperts to use these solving algorithms. In our lab, we take a domain expert’s problem and formalize it into a problem our solver can solve. Could we teach an LLM to do the same thing?” Fan says.
Using the framework the researchers developed, called LLM-Based Formalized Programming (LLMFP), a person provides a natural language description of the problem, background information on the task, and a query that describes their goal.
Then LLMFP prompts an LLM to reason about the problem and determine the decision variables and key constraints that will shape the optimal solution.
LLMFP asks the LLM to detail the requirements of each variable before encoding the information into a mathematical formulation of an optimization problem. It writes code that encodes the problem and calls the attached optimization solver, which arrives at an ideal solution.
“It is similar to how we teach undergrads about optimization problems at MIT. We don’t teach them just one domain. We teach them the methodology,” Fan adds.
As long as the inputs to the solver are correct, it will give the right answer. Any mistakes in the solution come from errors in the formulation process.
To ensure it has found a working plan, LLMFP analyzes the solution and modifies any incorrect steps in the problem formulation. Once the plan passes this self-assessment, the solution is described to the user in natural language.
Perfecting the plan
This self-assessment module also allows the LLM to add any implicit constraints it missed the first time around, Hao says.
For instance, if the framework is optimizing a supply chain to minimize costs for a coffeeshop, a human knows the coffeeshop can’t ship a negative amount of roasted beans, but an LLM might not realize that.
The self-assessment step would flag that error and prompt the model to fix it.
“Plus, an LLM can adapt to the preferences of the user. If the model realizes a particular user does not like to change the time or budget of their travel plans, it can suggest changing things that fit the user’s needs,” Fan says.
In a series of tests, their framework achieved an average success rate between 83 and 87 percent across nine diverse planning problems using several LLMs. While some baseline models were better at certain problems, LLMFP achieved an overall success rate about twice as high as the baseline techniques.
Unlike these other approaches, LLMFP does not require domain-specific examples for training. It can find the optimal solution to a planning problem right out of the box.
In addition, the user can adapt LLMFP for different optimization solvers by adjusting the prompts fed to the LLM.
“With LLMs, we have an opportunity to create an interface that allows people to use tools from other domains to solve problems in ways they might not have been thinking about before,” Fan says.
In the future, the researchers want to enable LLMFP to take images as input to supplement the descriptions of a planning problem. This would help the framework solve tasks that are particularly hard to fully describe with natural language.
This work was funded, in part, by the Office of Naval Research and the MIT-IBM Watson AI Lab.
0 notes
Video
youtube
LEETCODE 17 : PHONE NUMBER LETTER COMBINATIONS
LeetCode Problem 17, titled "Letter Combinations of a Phone Number," asks you to generate all possible letter combinations that a given phone number could represent, similar to the old mobile phone keypads. Each digit from 2 to 9 maps to a set of letters, and the challenge is to produce all combinations that the input digits could generate. This problem tests your ability to use recursive backtracking to explore all potential combinations efficiently. It’s an excellent exercise for understanding how to implement recursive solutions for combinatorial problems and is crucial for developing skills in handling permutations and combinations in programming.
0 notes
Text
Mobile Game Testing: A Complete Guide
Mobile gaming is one of the fastest-growing segments in the entertainment industry, with millions of users worldwide. However, delivering a seamless and engaging gaming experience requires rigorous testing to ensure the game is functional, visually appealing, and performs well across a wide range of devices. In this guide, we’ll explore what mobile game testing is, why it’s important, the key testing techniques, and how tools like Genqe.ai can enhance your testing efforts.
What Is Mobile Game Testing?
Mobile game testing is the process of evaluating a mobile game to ensure it meets quality standards, functions as intended, and provides an enjoyable user experience. It involves testing various aspects of the game, including functionality, performance, compatibility, and security, across different devices, operating systems, and network conditions.
Why Is Mobile Game Testing Important?
Mobile game testing is critical for several reasons:
Ensures Functionality: Verifies that all game features work as intended.
Enhances User Experience: Identifies and fixes issues that could frustrate players.
Improves Performance: Ensures the game runs smoothly on different devices and under various conditions.
Boosts Retention: A well-tested game is more likely to retain players and generate positive reviews.
Reduces Costs: Early detection of bugs minimizes the cost of fixing them post-release.
Mobile Game Testing Techniques
To ensure comprehensive testing, mobile game testing involves a variety of techniques:
1. Functional Testing
Purpose: Verifies that all game features and mechanics work correctly.
Example: Testing character movements, game levels, and in-app purchases.
2. Compatibility Testing
Purpose: Ensures the game works across different devices, operating systems, and screen sizes.
Example: Testing the game on iOS and Android devices with varying resolutions.
3. Usability Testing
Purpose: Evaluates the game’s user interface (UI) and overall user experience (UX).
Example: Assessing menu navigation, button placement, and tutorial clarity.
4. Visual Testing
Purpose: Ensures the game’s graphics, animations, and visual elements are consistent and appealing.
Example: Checking for pixelation, alignment issues, or incorrect color schemes.
5. Localization Testing
Purpose: Verifies that the game is adapted for different languages, regions, and cultures.
Example: Testing translated text, date formats, and region-specific content.
6. Performance Testing
Purpose: Evaluates the game’s responsiveness, speed, and resource usage.
Example: Testing frame rates, load times, and battery consumption.
7. Recovery Testing
Purpose: Ensures the game can recover from crashes or interruptions.
Example: Testing how the game handles sudden app closures or network disconnections.
8. Soak Testing
Purpose: Checks the game’s stability over extended periods of play.
Example: Running the game continuously for several hours to identify memory leaks or crashes.
9. Combinatorial Testing
Purpose: Tests different combinations of inputs and scenarios to uncover edge cases.
Example: Testing various character abilities and environmental interactions.
10. Compliance Testing
Purpose: Ensures the game adheres to platform-specific guidelines (e.g., Apple App Store, Google Play).
Example: Verifying app size limits, age ratings, and in-app purchase policies.
11. Security Testing
Purpose: Identifies vulnerabilities that could compromise user data or game integrity.
Example: Testing for hacking, cheating, or unauthorized access.12. Beta Testing
Purpose: Gathers feedback from real users before the official release.
Example: Releasing a beta version to a select group of players to identify bugs and usability issues.
How to Perform Mobile Game Testing?
To perform effective mobile game testing, follow these steps:
Define Test Objectives: Identify the key areas to test, such as functionality, performance, and usability.
Create Test Cases: Develop detailed test cases covering all aspects of the game.
Select Testing Tools: Use tools like Genqe.ai to automate and streamline testing processes.
Execute Tests: Run tests on real devices, emulators, or cloud-based platforms.
Analyze Results: Review test results to identify and prioritize issues.
Report and Fix Bugs: Document bugs and collaborate with developers to resolve them.
Retest: Verify that fixes work as intended and do not introduce new issues.
Enhance Mobile Game Testing With Genqe.ai
Genqe.ai is a powerful AI-driven testing tool that can significantly enhance your mobile game testing efforts. Here’s how:
Automated Test Case Generation: Genqe.ai automatically generates test cases based on game requirements, saving time and effort.
Cross-Platform Testing: Test your game on multiple devices and operating systems simultaneously.
Performance Optimization: Identify and resolve performance bottlenecks with detailed analytics.
Visual Testing: Detect visual inconsistencies and ensure a polished user experience.
Localization Support: Test localized content for different languages and regions.
Security Testing: Identify vulnerabilities and ensure the game is secure from threats.
By leveraging Genqe.ai, you can streamline your testing processes, reduce manual effort, and deliver a high-quality gaming experience to your players.
Conclusion
Mobile game testing is a critical step in ensuring the success of your game. By employing a comprehensive testing strategy that includes functional, compatibility, usability, performance, and security testing, you can identify and resolve issues before they impact players. Tools like Genqe.ai further enhance your testing efforts by automating repetitive tasks, providing actionable insights, and enabling cross-platform testing.
As the mobile gaming industry continues to grow, investing in robust testing practices and advanced tools like Genqe.ai will help you stay competitive and deliver games that captivate and delight players. Start enhancing your mobile game testing today and take your gaming experience to the next level!
0 notes
Text
Complex and Intelligent Systems, Volume 11, Issue 2, February 2025
1) A low-carbon scheduling method based on improved ant colony algorithm for underground electric transportation vehicles
Author(s): Yizhe Zhang, Yinan Guo, Shirong Ge
2) A survey of security threats in federated learning
Author(s): Yunhao Feng, Yanming Guo, Gang Liu
3) Vehicle positioning systems in tunnel environments: a review
Author(s): Suying Jiang, Qiufeng Xu, Jiachun Li
4) Barriers and enhance strategies for green supply chain management using continuous linear diophantine neural networks
Author(s): Shougi S. Abosuliman, Saleem Abdullah, Nawab Ali
5) XTNSR: Xception-based transformer network for single image super resolution
Author(s): Jagrati Talreja, Supavadee Aramvith, Takao Onoye
6) Efficient guided inpainting of larger hole missing images based on hierarchical decoding network
Author(s): Xiucheng Dong, Yaling Ju, Jinqing He
7) A crossover operator for objective functions defined over graph neighborhoods with interdependent and related variables
Author(s): Jaume Jordan, Javier Palanca, Vicente Julian
8) A multitasking ant system for multi-depot pick-up and delivery location routing problem with time window
Author(s): Haoyuan Lv, Ruochen Liu, Jianxia Li
9) Short-term urban traffic forecasting in smart cities: a dynamic diffusion spatial-temporal graph convolutional network
Author(s): Xiang Yin, Junyang Yu, Xiaoli Liang
10) Vehicle-routing problem for low-carbon cold chain logistics based on the idea of cost–benefit
Author(s): Yan Liu, Fengming Tao, Rui Zhu
11) Enhancing navigation performance in unknown environments using spiking neural networks and reinforcement learning with asymptotic gradient method
Author(s): Xiaode Liu, Yufei Guo, Zhe Ma
12) Mape: defending against transferable adversarial attacks using multi-source adversarial perturbations elimination
Author(s): Xinlei Liu, Jichao Xie, Zhen Zhang
13) Robust underwater object tracking with image enhancement and two-step feature compression
Author(s): Jiaqing Li, Chaocan Xue, Bin Lin
14) DMR: disentangled and denoised learning for multi-behavior recommendation
Author(s): Yijia Zhang, Wanyu Chen, Feng Qi
15) A traffic prediction method for missing data scenarios: graph convolutional recurrent ordinary differential equation network
Author(s): Ming Jiang, Zhiwei Liu, Yan Xu
16) Enhancing zero-shot stance detection via multi-task fine-tuning with debate data and knowledge augmentation
Author(s): Qinlong Fan, Jicang Lu, Shouxin Shang
17) Adaptive temporal-difference learning via deep neural network function approximation: a non-asymptotic analysis
Author(s): Guoyong Wang, Tiange Fu, Mingchuan Zhang
18) A bi-subpopulation coevolutionary immune algorithm for multi-objective combinatorial optimization in multi-UAV task allocation
Author(s): Xi Chen, Yu Wan, Jun Tang
19) Protocol-based set-membership state estimation for linear repetitive processes with uniform quantization: a zonotope-based approach
Author(s): Minghao Gao, Pengfei Yang, Qi Li
20) Optimization of high-dimensional expensive multi-objective problems using multi-mode radial basis functions
Author(s): Jiangtao Shen, Xinjing Wang, Zhiwen Wen
21) Computationally expensive constrained problems via surrogate-assisted dynamic population evolutionary optimization
Author(s): Zan Yang, Chen Jiang, Jiansheng Liu
22) View adaptive multi-object tracking method based on depth relationship cues
Author(s): Haoran Sun, Yang Li, Kexin Luo
23) Preference learning based deep reinforcement learning for flexible job shop scheduling problem
Author(s): Xinning Liu, Li Han, Huadong Miao
24) Microscale search-based algorithm based on time-space transfer for automated test case generation
Author(s): Yinghan Hong, Fangqing Liu, Guizhen Mai
25) A hybrid Framework for plant leaf disease detection and classification using convolutional neural networks and vision transformer
Author(s): Sherihan Aboelenin, Foriaa Ahmed Elbasheer, Khalid M. Hosny
26) A novel group-based framework for nature-inspired optimization algorithms with adaptive movement behavior
Author(s): Adam Robson, Kamlesh Mistry, Wai-Lok Woo
27) A generalized diffusion model for remaining useful life prediction with uncertainty
Author(s): Bincheng Wen, Xin Zhao, Jianfeng Li
28) A joint learning method for low-light facial expression recognition
Author(s): Yuanlun Xie, Jie Ou, Wenhong Tian
29) MKER: multi-modal knowledge extraction and reasoning for future event prediction
Author(s): Chenghang Lai, Shoumeng Qiu
30) RL4CEP: reinforcement learning for updating CEP rules
Author(s): Afef Mdhaffar, Ghassen Baklouti, Bernd Freisleben
31) Practice of an improved many-objective route optimization algorithm in a multimodal transportation case under uncertain demand
Author(s): Tianxu Cui, Ying Shi, Kai Li
32) Sentimentally enhanced conversation recommender system
Author(s): Fengjin Liu, Qiong Cao, Huaiyu Liu
33) New Jensen–Shannon divergence measures for intuitionistic fuzzy sets with the construction of a parametric intuitionistic fuzzy TOPSIS
Author(s): Xinxing Wu, Qian Liu, Xu Zhang
34) TMFN: a text-based multimodal fusion network with multi-scale feature extraction and unsupervised contrastive learning for multimodal sentiment analysis
Author(s): Junsong Fu, Youjia Fu, Zihao Xu
35) Batch-in-Batch: a new adversarial training framework for initial perturbation and sample selection
Author(s): Yinting Wu, Pai Peng, Le Li
36) RenalSegNet: automated segmentation of renal tumor, veins, and arteries in contrast-enhanced CT scans
Author(s): Rashid Khan, Chao Chen, Bingding Huang
37) Light-YOLO: a lightweight detection algorithm based on multi-scale feature enhancement for infrared small ship target
Author(s): Ji Tang, Xiao-Min Hu, Wei-Neng Chen
38) CPP: a path planning method taking into account obstacle shadow hiding
Author(s): Ruixin Zhang, Qing Xu, Guo Zhang
39) A semi-supervised learning technique assisted multi-objective evolutionary algorithm for computationally expensive problems
Author(s): Zijian Jiang, Chaoli Sun, Sisi Wang
40) An adjoint feature-selection-based evolutionary algorithm for sparse large-scale multiobjective optimization
Author(s): Panpan Zhang, Hang Yin, Xingyi Zhang
41) Balanced coarse-to-fine federated learning for noisy heterogeneous clients
Author(s): Longfei Han, Ying Zhai, Xiankai Huang
0 notes
Text

Dr. Jane Cooke Wright (November 20, 1919 – February 19, 2013) was a pioneering cancer researcher and surgeon noted for her contributions to chemotherapy. In particular, she is credited with developing the technique of using human tissue culture rather than laboratory mice to test the effects of potential drugs on cancer cells. She pioneered the use of the drug methotrexate to treat breast cancer and skin cancer.
In becoming physicians, she and her sister Barbara Wright-Pierce both followed in their father’s and grandfather’s footsteps, overcoming both gender and racial bias to succeed in a largely white male profession.
She attended Smith College, majoring in pre-medical studies. After her studies at Smith College, she earned a full scholarship to study medicine at New York Medical College. She graduated as a part of an accelerated three-year program at the top of her class in 1945 with honors and earned an internship at Bellevue Hospital (1945-46). In 1947, she completed her surgical residency at Harlem Hospital 1948, where her father was.
Her research work involved studying the effects of various drugs on tumors, and she was the first to identify methotrexate, one of the foundational chemotherapy drugs, as an effective tool against cancerous tumors. Her early work brought chemotherapy out of the realm of an untested, experimental hypothetical treatment, into the realm of tested, proven effective cancer therapeutic, literally saving millions of lives. She and her father introduced nitrogen mustard agents, similar to the mustard gas compounds used in WWI, that were successful in treating the cancerous cells of leukemia patients. She pioneered combinatorial work in chemotherapeutics, focusing not simply on administering multiple drugs, but on sequential and dosage variations to increase the effectiveness of chemotherapy and minimize side effects. She was successful in identifying treatments for both breast and skin cancer, developing a chemotherapy protocol that increased skin cancer patient lifespans by up to ten years. #africanhistory365 #africanexcellence #alphakappaalpha
0 notes
Text
From Lab-on-a-Chip to Industrial Innovation: Milestones in Microfluidic Technology
The global market for microfluidic products surged to $9.98 billion in 2019, with microfluidic devices accounting for $3.48 billion of this figure. A notable trend in the industry is the ongoing acquisition of microfluidic companies by larger enterprises, signaling a trajectory of accelerated growth through capital infusion.
In the industrial landscape, in vitro diagnostics (IVD) stands out as the primary sector for microfluidic applications, driven by its lucrative returns. Demographic shifts, particularly aging populations, contribute to an escalating demand for microfluidic chips. Moreover, governmental policies prioritize the advancement of the microfluidics industry, a focus that has intensified amidst the backdrop of the pandemic. Moving forward, the critical hurdles facing microfluidic chip technology revolve around manufacturing costs and scalability. Achieving scalable production processes and cost reduction measures while maintaining product standardization and minimizing variations are imperative objectives.
The evolution of modern technology emphasizes miniaturization, integration, and intelligence. Microelectromechanical systems (MEMS) have played a pivotal role in this evolution, enabling the transition from bulky electronic systems to compact integrated circuit chips and handheld devices like smartphones. Similarly, microfluidic chips, often referred to as Lab-on-a-Chip technology, epitomize the manipulation of fluids at micro- and nanoscales. These chips condense essential laboratory functionalities, such as sample preparation, reaction, separation, and detection, onto a compact chip, typically a few square centimeters in size. The hallmark of microfluidic chips lies in their capacity for flexible integration and scaling of diverse unit technologies within a controllable microplatform.
Originating from MEMS technology, early microfluidic chips underwent fabrication processes on substrates like silicon, metals, polymers, glass, and quartz. These processes yielded microstructure units such as fluid channels, reaction chambers, filters, and sensors, with dimensions ranging from micrometers to sub-millimeters. Subsequent fluid manipulation within these microstructures enabled automated execution of biological laboratory procedures, including extraction, amplification, labeling, separation, and analysis, or cell manipulation and analysis.
In the early 1990s, A. Manz et al. demonstrated the potential of microfluidic chips as analytical chemistry tools by achieving electrophoretic separation—a technique previously confined to capillaries—on chips. Subsequently, spurred by the U.S. Department of Defense's requisition for portable biochemical self-test equipment, research in microfluidic chips burgeoned globally. Throughout the 1990s, microfluidic chips primarily served as platforms for analytical chemistry, often interchangeably referred to as "Micro Total Analysis Systems" (u-TAS). Consequently, these chips found applications across diverse fields, including biomedical diagnostics, food safety, environmental monitoring, forensics, military, and aerospace sciences.
Key milestones in the advancement of microfluidic chips include G. Whitesides et al.'s 2000 publication on PDMS soft lithography and S. Quake et al.'s 2002 article on "large-scale integration of microfluidic chips" featuring microvalve and micropump controls. These seminal works propelled microfluidic chips beyond the confines of traditional analytical systems, unlocking their potential for significant scientific and industrial applications. For instance, microfluidic chips enable the execution of combinatorial chemical reactions or droplet techniques, facilitating drug synthesis, high-throughput screening, and large-scale nanoparticle or microsphere production. In essence, microfluidic chips pave the way for the realization of a "chemical plant or pharmaceutical lab on a chip."
0 notes
Text
Immediate Matrix: Your Guide to Smart Trading Decisions

Trading, whether it's stocks or cryptocurrencies, has become a popular way for many people to make money. A new platform called Immediate Matrix says that its many features make it one of the best choices for traders who want to possibly make a lot of money. Some people who have only recently heard of the app may not know if it is real, though. To help you with this, we will carefully look over Immediate Matrix and rate every part of the platform to get a sense of how real it is as a trade platform.
✅ Trading App Name ╰┈➤ Immediate Matrix ⭐
✅ Offer Type ╰┈➤ Crypto ₿
✅ Traffic Cap ╰┈➤ N/A ❌
✅ Target Market ╰┈➤ Male and Female- 18-60+ years 👨🏼🤝👨🏻
✅ Investment ╰┈➤ $250 First Deposit 💰
✅ Fee ╰┈➤ No 🙅♂️
꧁༺✨❗Buy Now ❗✨༻꧂
https://www.offerplox.com/get-Immediate-Matrix
꧁༺✨❗ Official Facebook ❗✨༻꧂
https://www.facebook.com/groups/theimmediatematrix
꧁༺✨❗Shop Now ❗✨༻꧂
https://immediatematrix.company.site/
What Is Immediate Matrix?
Arrays, datatypes, and stored procedure snowflakes are used by this program to make trade execution more efficient. Price gaps or margins can be used to get or suggest order types with little risk. This is the idea behind the technology. What does it all mean? In simple words, it means that the technology that runs Immediate Matrix makes the things that happen behind the scenes faster and better.
💰 Click Here To Trade With Immediate Matrix For Free 🤑
All of this is done to raise the success rate so that members can get more benefits. Also, it's important to note that Immediate Matrix uses Python, Lisp, and Julia code. That being said, these programming languages, along with JavaScript and C++, are the most widely linked to AI development. Furthermore, AI sequence methods are used in real time for predictive analysis, along with formulas from combinatorial mathematics.
How Does Immediate Matrix Work?
Many people are interested in the Immediate Matrix Reviews because it has many analytical tools that can help users make important trading decisions that could lead to lucrative chances. There isn't much to talk about when it comes to how the platform works since the project doesn't include a trading robot or other features that make dealing easier for users.
We also couldn't find any details about the software and algorithms that were used to make the trading platform. In fact, buyers who sign up as users are the only ones who can even get to the dashboard that the website talks about. The platform's signup process seems to be as easy as it gets, since there is no Know Your Customer (KYC) step.
The trading charts, indicators, and other similar tools, on the other hand, look like they were made by the people who made the program and not by someone else. This means that users will be able to reach a platform that is better tailored to their needs.
Who Created Immediate Matrix?
As we looked into the platform, we found on a number of outside websites that the Immediate Matrix platform was created by a group of well-known and powerful people in the banking and blockchain industries. We did not find any proof, though, that such a group was behind the project. It looks like the developers decided to stay anonymous, which is common in the cryptocurrency world these days.
Immediate Matrix Features
Here are some of the most important parts of the Immediate Matrix program. The app is very far ahead of its time in the crypto space thanks to these abilities.
💰 Click Here To Trade With Immediate Matrix For Free 🤑
Strategy Tester
The Immediate Matrix Platform has a built-in strategy tester that lets traders test their favorite trading methods both in the past and in the future. Crypto traders can use the strategy tester tool to make their trading strategies better after setting their own preferences.
Demo Trading
Traders can use the demo account that comes with this advanced trading tool to try and improve their trading strategies before using it to trade with real money. People who trade on demo accounts can also learn how Immediate Matrix works.
High Customization
There are a lot of ways to change the Immediate Matrix program so that traders can have full control over their trading. Traders and buyers can change things about trading, such as the coins that can be traded, the amount staked, the trading times, the stop loss and take profit levels, and more. There is also room to switch between automated and manual trade modes in Immediate Matrix.
Demo Account
All Immediate Matrix partner brokers give all traders and buyers a free demo account that they can use as much as they want. This means that traders can test how well the software works and improve their trading skills before putting money into it for good.
Advanced Strategy
The Immediate Matrix trading software uses both advanced technical and fundamental methods to make sure that trades in the cryptocurrency markets are always correct. The app uses built-in artificial intelligence (AI) to figure out how people feel about the crypto market as a whole so that traders can make money in both trending and non-trending situations.
Automated Software
Based on coded algorithms, this cutting-edge training software helps people deal on the cryptocurrency market. Because it is automatic, investors don't need to do anything. The software does have a manual trading mode, though, which lets traders handle their accounts and take charge of their trading.
Safety
Immediate Matrix Software makes sure that its traders are safe by using the best security standards. People who trade in Bitcoin and its partner brokers help keep their money and personal information safe at all times. Immediate Matrix cares very much about the safety and security of its buyers.
How To Use Immediate Matrix?
If you're still interested in the site after reading the review, here's how to start trading on Immediate Matrix:
💰 Click Here To Trade With Immediate Matrix For Free 🤑
Step 1 – Visit the Immediate Matrix Website
Go to the official Immediate Matrix website and start the signup process to get things going. Giving simple information like your name, email address, and phone number is usually what this means.
Step 2 – Make the Minimum Deposit
After setting up and verifying your account, it's time to make your first payment. Before you can get to the screen, you have to do this. Several payment methods, such as credit cards and e-wallets that are accepted, can be used to add money to your account.
Step 3 – Start Trading with Immediate Matrix
Now that the deposit is complete, you can look at the different cryptocurrencies, buy in one, and start trading on the platform.
How Is Immediate Matrix Different Than Other Trading Apps?
Immediate Matrix is a platform for trading and getting tips about cryptocurrencies. It lets users customize alerts and signals for different cryptocurrencies. Here are some things that might make the Immediate Matrix trade app stand out or be important features:
Customizable Alerts: Stay up to date on important changes in the bitcoin market.
User-Friendly Interface: means a system that is easy for new or inexperienced users to use.
Market Data Aggregation: Immediate Matrix collects market data from different coin exchanges and makes it available.
Portfolio Tracking: The app has tools for portfolio tracking that let users see how their trades are doing.
Real-Time Notifications: Immediate Matrix wants to send real-time alerts when certain conditions are met.
Is Immediate Matrix a Scam?
It's not easy to say for sure whether Immediate Matrix Registration is a scam or a real cryptocurrency trading site. Many people are worried about the platform because they can't find much information about it before they put money, which is not ideal.
It also doesn't look like there are any clear social media names or other ways to get in touch with the platform before you make the minimum deposit and sign up. Even though Immediate Matrix makes bold claims about what it can do, it doesn't quite back them up with solid proof.
💰 Click Here To Trade With Immediate Matrix For Free 🤑
It is hard to make a firm decision about the platform because there isn't enough solid information to compare it to. Taking all of this into account, people who want to use the Immediate Matrix platform should be very careful and carefully consider their choices before they start trading.
Immediate Matrix Cost, Investment & Estimated Profit
You can use the Immediate Matrix Trading App for free. Traders only need to sign up and wait for approval from the team before they can use their account. You need to put at least $250 into your account before you can start betting. There are no fees to transfer money, withdraw money, or use Immediate Matrix's broking services. So, the only cost you have to pay is the money you put in to start trading. If you trade wisely, you can later make huge gains.
Conclusion
We did everything we could to find and analyze all the information we could find about Immediate Matrix. Although it advertises itself as a promising trading tool, there isn't much proof to back up its claims. It is very hard to tell if Immediate Matrix Official Website is real because there isn't enough solid information and data available.
We also saw that the site didn't have many reviews or comments from trustworthy sources. Because of these things, we highly advise that all users be very careful and do a lot of research before they open an Immediate Matrix account to trade.
ImmediateMatrix
ImmediateMatrixReviews
ImmediateMatrixPlatform
ImmediateMatrixSoftware
ImmediateMatrixLogin
ImmediateMatrixOfficialWebsite
ImmediateMatrixRegistration
ImmediateMatrixTrading
ImmediateMatrixApp
ImmediateMatrix2024
ImmediateMatrixLegit
ImmediateMatrixScam
ImmediateMatrixInvestment
ImmediateMatrixReal
ImmediateMatrixWealth
ImmediateMatrixDetails
ImmediateMatrixPlatformReviews
1 note
·
View note
Text
Price: [price_with_discount] (as of [price_update_date] - Details) [ad_1] Verilog Digital System Design, 2/e, shows electronics designers and students how to apply verilog in sophisticated digital system design. Using over a hundred skill-building, fully worked-out, and simulated examples, this completely updated edition covers Verilog 2001, new synthesis standards, testing and testbench development, and the new OVL verification library. Moving from simple concepts to the more complex, Navabi interprets verilog constructs related to design stages and design abstractions, including behavioral, dataflow, and structure description. With emphasis on the concepts of HDLs. Clear specification and learning objectives at the beginning of each chapter and end-of-chapter problems focus attention on key points. Written by a HDL expert, the book provides: * Design automation with Verilog * Design with Verilog * Combinatorial circuits in Verilog * Sequential circuits in Verilog * Language utilities * Test methodologies * Verification * CPU design and verification MUST-HAVE CD INCLUDED * Verilog and VHDL simulators * Synthesis tools * Mixed-level logic and Verilog design environment * FPGA design tools and environments from Altera * Related tutorials and standards * All worked examples from the book, including testbench and simulationrun reports for every example * Complete CPU examples with Verilog code and software tools * OVL verification libraries and tutorials [ad_2]
0 notes
Text
Automated Functional Testing Tools – A Complete Guide

Automated functional testing has become vital in software development to ensure applications meet high-quality standards. This blog explains the importance of using automated tools to verify the functionality of software. Automated testing improves efficiency, consistency, coverage, and cost-effectiveness by executing tests quickly, minimizing errors, covering various scenarios, and reducing manual intervention. Key tools like Selenium, QTP/UFT, TestComplete, and Ranorex are discussed for their capabilities in web, desktop, and mobile testing. Additionally, GenQE, with AI and ML integration, enhances automated test case generation, utilizing combinatorial design techniques, Jira stories, and requirement documents for comprehensive testing. These advancements ensure thorough coverage of all possible inputs, identifying potential defects and improving software quality. Automated functional testing, combined with GenQE’s cutting-edge features, enables developers to deliver robust software efficiently.
If you want to get complete information related to this topic click HERE.
#automatedtesting#functional testing#software testing#test automation#testingtools#genqe#aiintesting#qualityassurance#softwarequality#software development
0 notes
Text
How Quantum Algorithms Revolutionize Financial Portfolio Optimization for Risk Management
In the fast-paced world of finance, portfolio optimization is a critical component of risk management. Traditional methods often struggle to handle the complexities and vast datasets involved, leading to suboptimal decision-making. However, quantum algorithms are emerging as a powerful alternative, offering new avenues for enhancing financial portfolio optimization. Let’s explore how these algorithms revolutionize risk management in finance.
Understanding Portfolio Optimization
Portfolio optimization involves selecting the best mix of assets to maximize returns while minimizing risk. Key challenges include:
Complex Calculations: Evaluating potential asset combinations requires significant computational resources, especially as the number of assets increases.
Dynamic Market Conditions: Financial markets are volatile and influenced by numerous unpredictable factors, making it essential to adapt quickly.
Nonlinear Relationships: Asset correlations can be complex and nonlinear, complicating risk assessment.
Advantages of Quantum Algorithms
Increased Computational Power:
Exponential Speedup: Quantum algorithms can perform complex calculations much faster than classical computers. For example, using Grover’s algorithm, the search for optimal asset combinations can be accelerated, enabling quicker decision-making.
Simultaneous Processing: Quantum systems can analyze multiple potential portfolios at once, significantly reducing the time required for evaluations.
2. Improved Risk Assessment:
Advanced Models: Quantum algorithms can incorporate advanced mathematical models that better capture the nuances of financial data, leading to more accurate risk assessments.
Enhanced Simulations: Quantum Monte Carlo methods can simulate market scenarios with greater efficiency, allowing for more thorough stress testing and scenario analysis.
3. Complex Optimization Techniques:
Quantum Approximate Optimization Algorithm (QAOA): This algorithm is specifically designed for combinatorial optimization problems, such as selecting the best portfolio. It helps find optimal asset allocations by exploring various combinations more efficiently.
Quantum Machine Learning: Integrating quantum machine learning techniques can improve predictive analytics, identifying trends and patterns that inform better investment strategies.
Practical Applications in Finance
Dynamic Asset Allocation: Quantum algorithms enable real-time adjustments to portfolio allocations based on market changes, enhancing responsiveness to volatility and risk.
Risk Diversification: By analyzing complex relationships between assets, quantum algorithms can optimize diversification strategies, reducing overall portfolio risk.
Algorithmic Trading: Quantum-enhanced trading algorithms can quickly identify and exploit arbitrage opportunities, optimizing execution strategies while managing risk.
Case Studies and Real-World Implementations
Hedge Funds and Asset Managers: Several firms are exploring quantum computing for portfolio optimization, utilizing quantum algorithms to enhance their risk management strategies and gain competitive advantages.
Financial Institutions: Major banks are investigating the integration of quantum computing to improve their risk assessment models and portfolio optimization processes.
Challenges and Considerations
While the potential of quantum algorithms in portfolio optimization is significant, several challenges remain:
Technology Readiness: Quantum computing is still developing, and many financial institutions may not have access to the necessary hardware or expertise.
Data Security: As quantum computing advances, concerns about data security and privacy also rise, necessitating robust protective measures.
Integration with Existing Systems: Implementing quantum algorithms within traditional financial systems requires careful planning and technical knowledge.
Conclusion
Quantum algorithms are set to revolutionize financial portfolio optimization and risk management. By harnessing the power of quantum computing, financial professionals can enhance their ability to assess risk, optimize asset allocations, and respond rapidly to market changes. As the technology matures, those who embrace quantum innovations will likely gain a competitive edge in the ever-evolving financial landscape, paving the way for more informed, effective investment strategies.
1 note
·
View note
Text
Generative Quantum Eigensolver (GQE): A Quantum Advantage

The promise of quantum computing is its ability to address problems beyond the reach of ordinary computers. The novel approach Generative Quantum AI (GenQAI) is one of the best ways to fulfil that promise. This technique extensively on Generative Quantum Eigensolver.
GenQAI's simple yet successful concept combines AI's flexibility and intelligence with quantum technology's strengths. Using quantum devices to produce data and artificial intelligence (AI) to learn from and control data generation may create a powerful feedback loop that accelerates breakthroughs in many fields.
The quantum processing unit (QPU) generates data that classical systems cannot. Our advantage is that it delivers an AI new, meaningful knowledge that isn't available anyplace else, not just internet text.
GQE Meaning
Based on a classical generative model of quantum circuits, the Generative Quantum Eigensolver (GQE) estimates the ground-state energy of any molecular Hamiltonian 1.
Ground State Energy Search
One of the most intriguing quantum chemistry and materials science topics is calculating a molecule's ground state characteristics. Ground states are molecules' or materials' lowest energy states. Understand this condition to design novel drugs or materials and understand molecular behaviour.
It is difficult to calculate this state properly for systems other than the simplest. Since the number of quantum states doubles rapidly, measuring their energies and testing them brute force is not feasible. This shows the need for a sophisticated ground state energy and chemical characteristic location approach.
This case benefits from GQE. GQE trains a transformer using quantum computer data. The transformer proposes intriguing experimental quantum circuits that may prepare low-energy states. Similar to an AI-powered ground state search engine. The transformer is taught from scratch using component data, making it unique.
It works like this:
Start by running experimental quantum circuits on the QPU.
It measures the energetic quantum states created by each circuit in respect to its Hamiltonian.
A transformer model with the same design as GPT-2 uses such metrics to improve its outcomes.
Transformers create a circuit distribution that favours lower-energy state circuits.
Restart the procedure after running new QPU distribution samples.
Over time, the system learns and approaches the ground state.
This benchmark task involved finding the hydrogen molecule's (H₂) ground state energy to assess the program. It can validate the setup works because this issue has a recognised remedy. Thus, its GQE system located the ground state chemically.
The team was the first to tackle this problem with a QPU and transformer, ushering in a new era in computational chemistry.
Future of Quantum Chemistry
A generative model based on quantum measurements can be utilised for materials discovery, combinatorial optimisation, and even drug synthesis.
Combining AI with quantum computing skills unlocks their potential. This quantum processor can provide previously unreachable rich data. AIs can learn from the data. They can solve problems neither could alone when they work together.
This is only the start. In addition to exploring how this approach may be used to real-world use cases, GQE is being applied to more complex molecules that existing methods cannot solve. This creates many new chemical possibilities, and everyone is excited to see what occurs.
#technology#technews#govindhtech#news#technologynews#Generative Quantum Eigensolver#GQE#Generative Quantum AI#Quantum AI#quantum processing unit#QPU
0 notes
Text
From breakthrough to blockbuster, the business of biotechnology Donald Drakeman
============================

Recombinant DNA, monoclonal antibody twin foundations of modern biotechnology
Antisense technology, gene therapy, tumour vaccines, stem cell therapy, combinatorial chemistry, high throughput screening, gene chips, tissue engineering, bioinformatics,proteomics, rational drug design, novel delivery technologies
Tufts study
Estimated cost of drug development at $2.56 billion
After all these opportunity costs are factored in the actual money spent in the drug development process is estimated at $1.4 billion
Only a tiny number of the largest biotech companies have integrated R&D organizations capable of discovering new product candidates and then developing them all the way to the commercial market.
Contract research organizations enabled "virtual" biotech companies. The expertise of CROs spans the entire spectrum of the drug development process, from creating initial compounds to performing in vitro, in vivo and human clinical testing to manufacturing the necessary quantities of the product to applying for FDA approval and even providing contracted sales and marketing services.
The drug development process
Academic research - many thousands of ideas
Early research and preclinical - invitro invivo testing
IND submission (Investigative new drug)
Clinical trials - phase I safety, phase 2 safety and efficacy, phase 3 safety and efficacy at large scale
NDA/ BLA submission
FDA approval
In looking at drugs entering clinical development 11.83% of the product candidates had reached FDA approval
Bayh-Dole Act university patent technology licensing bolstered economy by$1.3 trillion. Life sciences accounted for 70% of licenses and 93% of gross technology transfer revenues.
Few cases where venture capitalists wait to successfully develop a new drug. They are focused on having the biotech company
achieve whatever technical and corporate milestones will create opportunities for a successful exit
Since there are dramatic ebbs and flows in the overall availability of investment capital for biotech companies, there can be a boom-or-burst feeling in the early stage biotech arena, irrespective of the rate at which exciting new technologies and products emerge from research universities and other medical centers.
Qualities for biotech entrepreneurship.
Do you always think there is a better way to do things?
Are you willing to take on just about anything, even if you don't know much about it?
Are you comfortable taking risks?
Do you like to do new things, or do you prefer routine?
Can you accept rejection and failure?
Why Biotech companies are more innovative than pharmaceutical companies
In contrast to a large centralized environment that can be prone to limiting the overall number of projects and then be slow to stop the unsuccessful one,a decentralized environment of multiple external investors maximizes the potential for following the two critical principles of (1) initiating many diverse projects and (2) stopping the ones that are not working out. Having many different decision makers who are responsible for allocating funding creates a favorable environment for trying many different things. It also minimizes the effects of the sunk cost fallacy and the intra-organizational perspectives that make it difficult for large, cemtralized structures to make responsive termination decisions.
In fields outside the life sciences, technological advances often lead of ways to do things faster, better amd cheaper.
The crucial financial point is that biotech's breakthroughs may be lifesaving but rarely been cost saving
Scientists and physicians can figure out if a new drug actually extends lives, and mathematicians can calculate the costs; but none of those analyses lead directly to a considered judgment about who should have those benefits and at what price.
National Institute of health social value judgments 4 principles
respect for autonomy
non-maleficence
beneficence
distributive justice
Eg monoclonal antibody technology was discoved in England but is so expensive that the NHS refused to pay for them. It will be cold comfort to know that the UK economy was stimulated by research funding that contributed to the development of a new drug if that stimulus was not financially potent enough to allow the nation to be able to afford to pay for the drug itself
1 note
·
View note
Text
Multi-omics approaches define novel aphid effector candidates associated with virulence and avirulence phenotypes
Background: Compatibility between plant parasites and their hosts is genetically determined by both interacting organisms. For example, plants may carry resistance (R) genes or deploy chemical defences. Aphid saliva contains many proteins that are secreted into host tissues. Subsets of these proteins are predicted to act as effectors, either subverting or triggering host immunity. However, associating particular effectors with virulence or avirulence outcomes presents challenges due to the combinatorial complexity. Here we use defined aphid and host genetics to test for co-segregation of expressed aphid transcripts and proteins with virulent or avirulent phenotypes. Results: We compared virulent and avirulent pea aphid parental genotypes, and their bulk segregant F1 progeny on Medicago truncatula genotypes carrying or lacking the RAP1 resistance quantitative trait locus. Differential gene expression analysis of whole body and head samples, in combination with proteomics of saliva and salivary glands, enabled us to pinpoint proteins associated with virulence/avirulence phenotypes. There was relatively little impact of host genotype, whereas large numbers of transcripts and proteins were differentially expressed between parental aphids, likely a reflection of their classification as divergent biotypes within the pea aphid species complex. Many fewer transcripts intersected with the equivalent differential expression patterns in the bulked F1 progeny, providing an effective filter for removing genomic background effects. Overall, there were more upregulated genes detected in the F1 avirulent dataset compared with the virulent one. Some genes were differentially expressed both in the transcriptome and in the proteome datasets, with aminopeptidase N proteins being the most frequent differentially expressed family. In addition, a substantial proportion (27%) of salivary proteins lack annotations, suggesting that many novel functions remain to be discovered. Conclusions: Especially when combined with tightly controlled genetics of both insect and host, multi-omics approaches are powerful tools for revealing and filtering candidate lists down to plausible genes for further functional analysis as putative aphid effectors. http://dlvr.it/TBJcD2
0 notes