#data Management
Explore tagged Tumblr posts
Note
Hi, very random question - would you have advice for naming and organizing files? I saw your reblog of how to turn off the Windows 11 internet search thing and had my eyes bug out at the amount of files you have. I struggle to keep things organized after like....twenty...
Sure thing! Before I got into 3D, I didn't pay much mind to my file names or where I saved things. After getting into 3D, where those things have an impact on your ability to work on your projects, I was forced to tighten up! 1. Folders are your friend. However you want to organize things is up to you; depending on what I'm working on, I group things by project or subject first. So, for example, on my computer I might have a folder titled "DND". Inside that folder, I have a sub-folder for each campaign, and inside that folder I have a sub-folder for things like maps & documents, and then another for character art with sub-folders divided by character. 2. Decide a naming convention for your stuff. This could be something like "projectShortName_pg#_MMDDYY", or "characterName_portrait_MMDDYY". Having an identifier that makes it clear it's different from other files with similar names is really helpful, and keeping it in the name itself (instead of relying on "last modified" can be a good move. 3. Keep it short, but keep it useful. This is something you might not want to implement-- I use it all the time because it's part of the 3D pipeline, but shortnames are big for knowing what files are "at a glance". Like instead of something like, "Legend of Zelda Link Fanart 112123", I'd go with something like "TLOZ_LFA_112123". This is most useful when the folder structures are in place; if you have a Legend_of_Zelda folder, TLOZ will likely click as "The Legend of Zelda". 4. Don't be afraid to clear it out. Every few months, I gather everything I'm finished with into a folder titled "DSKT_CLEAR_MMDDYY". All of my folders are moved into the core folder, that folder gets moved to my external(s), and I move on.
Doing this when you've never done it before is a hard habit to establish (again, I was only able to do it because it was required while I was in school and now that I'm teaching the same subject), and going back to organize old stuff can be really intimidating. For that reason, I'd suggest gathering everything you currently have, moving it into a "Folder_Holder" folder, and then trying to implement these tips in future file management.
Let me know if you have any questions!
161 notes
·
View notes
Text
Research Data Management. Or, How I made multiple backups and still almost lost my honours thesis.
This is a story I used to tell while teaching fieldworkers and other researchers about how to manage their data. It’s a moderately improbable story, but it happened to me and others have benefited from my misadventures. I haven't had reason to tell it much lately, and I thought it might be useful to put into writing. This is a story from before cloud storage was common - back when you could, and often would, run out of online email storage space. Content note: this story includes some unpleasant things that happened to me, including multiple stories of theft (cf. moderately improbable). Also, because it's stressful for most of the story, I want to reassure you that it does have a happy conclusion. It explains a lot of my enthusiasm for good research data management. In Australia, 'honours' is an optional fourth year for a three year degree. It's a chance to do some more advanced coursework and try your hand at research, with a small thesis project. Of course, it doesn't feel small when it's the first time you've done a project that takes a whole year and is five times bigger than anything you’ve ever written. I've written briefly about my honours story (here, and here in a longer post about my late honours supervisor Barb Kelly) . While I did finish my project, it all ended a bit weirdly when my supervisor Barb got ill and left during the analysis/writing crunch. The year after finishing honours I got an office job. I hoped to maybe do something more with my honours work, but I wasn't sure what, and figured I would wait until Barb was better. During that year, my sharehouse flat was broken into and the thief walked out with the laptop I'd used to do my honours project. The computer had all my university files on it, including my data and the Word version of my thesis. I lost interview video files, transcriptions, drafts, notes and everything except the PDF version I had uploaded to the University's online portal. Uploading was optional at the time, if I didn't do that I probably would have just been left with a single printed copy. I also lost all my jewellery and my brother’s base guitar, but I was most sad about the data (sorry bro). Thankfully, I made a backup of my data and files on a USB drive that I kept in my handbag. This was back when a 4GB thumb drive was an investment. That Friday, feeling sorry for myself after losing so many things I couldn't replace, I decided to go dancing to cheer myself up. While out with a group of friends, my bag was stolen. It was the first time I had a nice handbag, and I still miss it. Thankfully, I knew to make more than one back up. I had an older USB that I'd tucked down the back of the books on my shelf (a vintage 256MB drive my dad kindly got for me in undergrad after a very bad week when I lost an essay to a corrupted floppy disk). When I went to retrieve the files, the drive was (also) corrupted. This happens with hard drives sometimes. My three different copies in three different locations were now lost to me.
Thankfully, my computer had a CD/DVD burner. This was a very cool feature in the mid-tens, and I used to make a lot of mixed CDs for my friends. During my honours project I had burned backed up files on some discs and left them at my parents house. It was this third backup, kept off site, which became the only copy of my project. I very quickly made more copies. When Barb was back at work, and I rejoined her as a PhD student, it meant we could return to the data and all my notes. The thesis went through a complete rewrite and many years later was published as a journal article (Gawne & Kelly 2014). It would have probably never happened if I didn’t have those project files. I continued with the same cautious approach to my research data ever since, including sending home SD cards while on field trips, making use of online storage, and archiving data with institutional repositories while a project is ongoing.
I’m glad that I made enough copies that I learnt a good lesson from a terrible series of events. Hopefully this will prompt you, too, to think about how many copies you have, where they’re located, and what would happen if you lost access to your online storage.
72 notes
·
View notes
Text
instagram
Hey there! 🚀 Becoming a data analyst is an awesome journey! Here’s a roadmap for you:
1. Start with the Basics 📚:
- Dive into the basics of data analysis and statistics. 📊
- Platforms like Learnbay (Data Analytics Certification Program For Non-Tech Professionals), Edx, and Intellipaat offer fantastic courses. Check them out! 🎓
2. Master Excel 📈:
- Excel is your best friend! Learn to crunch numbers and create killer spreadsheets. 📊🔢
3. Get Hands-on with Tools 🛠️:
- Familiarize yourself with data analysis tools like SQL, Python, and R. Pluralsight has some great courses to level up your skills! 🐍📊
4. Data Visualization 📊:
- Learn to tell a story with your data. Tools like Tableau and Power BI can be game-changers! 📈📉
5. Build a Solid Foundation 🏗️:
- Understand databases, data cleaning, and data wrangling. It’s the backbone of effective analysis! 💪🔍
6. Machine Learning Basics 🤖:
- Get a taste of machine learning concepts. It’s not mandatory but can be a huge plus! 🤓🤖
7. Projects, Projects, Projects! 🚀:
- Apply your skills to real-world projects. It’s the best way to learn and showcase your abilities! 🌐💻
8. Networking is Key 👥:
- Connect with fellow data enthusiasts on LinkedIn, attend meetups, and join relevant communities. Networking opens doors! 🌐👋
9. Certifications 📜:
- Consider getting certified. It adds credibility to your profile. 🎓💼
10. Stay Updated 🔄:
- The data world evolves fast. Keep learning and stay up-to-date with the latest trends and technologies. 📆🚀
. . .
#programming#programmers#developers#mobiledeveloper#softwaredeveloper#devlife#coding.#setup#icelatte#iceamericano#data analyst road map#data scientist#data#big data#data engineer#data management#machinelearning#technology#data analytics#Instagram
8 notes
·
View notes
Text
Intelligent Data Management in Life Sciences: A Game Changer for the Pharmaceutical Industry
In the fast-paced world of life sciences and pharmaceuticals, data management is crucial for driving innovation, enhancing compliance, and ensuring patient safety. With an ever-growing volume of data being generated across clinical trials, drug development, and regulatory compliance, pharmaceutical companies face the challenge of managing and analyzing this vast amount of data efficiently. Intelligent data management offers a solution to these challenges, ensuring that organizations in the life sciences industry can harness the full potential of their data.
Mastech InfoTrellis is a leader in implementing AI-first data management solutions, enabling pharmaceutical companies to streamline their operations, improve decision-making, and accelerate their research and development efforts. This blog explores the critical role of intelligent data management in the pharmaceutical industry, focusing on how Mastech InfoTrellis helps companies navigate data complexity to enhance business outcomes.
What Is Intelligent Data Management in Life Sciences?
Intelligent data management refers to the use of advanced technologies, such as artificial intelligence (AI), machine learning (ML), and automation, to manage, analyze, and leverage data in a way that improves operational efficiency and decision-making. In the life sciences industry, data is generated from various sources, including clinical trials, electronic health records (EHR), genomic research, and regulatory filings. Intelligent data management solutions help pharmaceutical companies streamline the collection, organization, and analysis of this data, making it easier to extract actionable insights and comply with stringent regulatory requirements.
Mastech InfoTrellis applies cutting-edge data management solutions tailored to the pharmaceutical industry, focusing on improving data accessibility, enhancing data governance, and enabling real-time analytics for better decision-making.
Join - ReimAIgined Intelligence at Informatica World 2025
The Importance of Data Management in the Pharmaceutical Industry
Effective data management is the backbone of the pharmaceutical industry. With the increasing volume of data generated in drug discovery, clinical trials, and regulatory compliance, pharmaceutical companies need intelligent systems to handle this data efficiently. Poor data management can lead to significant challenges, such as:
Regulatory non-compliance: In the pharmaceutical industry, compliance with global regulations, including those from the FDA and EMA, is paramount. Mishandling data or failing to track changes in regulations can lead to severe penalties and delays in product approvals.
Data silos: In many organizations, data is stored in different departments or systems, making it difficult to access and analyze holistically. This leads to inefficiencies and delays in decision-making.
Inaccurate data insights: Inaccurate or incomplete data can hinder the development of new drugs or the identification of critical health trends, affecting the overall success of research and development projects.
Intelligent data management solutions, such as those offered by Mastech InfoTrellis, address these issues by ensuring that data is accurate, accessible, and actionable, helping pharmaceutical companies optimize their workflows and drive better business outcomes.
Key Benefits of Intelligent Data Management in Life Sciences
1. Improved Data Governance and Compliance
In the pharmaceutical industry, data governance is a critical function, particularly when it comes to regulatory compliance. Intelligent data management solutions automate the processes of data validation, audit trails, and reporting, ensuring that all data handling processes comply with industry regulations.
Mastech InfoTrellis provides Informatica CDGC (Cloud Data Governance and Compliance), which ensures that data management processes align with industry standards such as Good Clinical Practice (GCP), Good Manufacturing Practice (GMP), and 21 CFR Part 11. This integration enhances data traceability and ensures that pharmaceutical companies can provide accurate and timely reports to regulatory bodies.
2. Enhanced Data Access and Collaboration
In a complex, multi-departmental organization like a pharmaceutical company, it is essential to have data that is easily accessible to the right stakeholders at the right time. Intelligent data management systems ensure that data from clinical trials, research teams, and regulatory departments is integrated into a unified platform.
With Mastech InfoTrellis's AI-powered Reltio MDM (Master Data Management) solution, pharmaceutical companies can break down data silos and provide a 360-degree view of their operations. This enables seamless collaboration between teams and faster decision-making across departments.
3. Faster Drug Development and Innovation
Pharmaceutical companies must make data-driven decisions quickly to bring new drugs to market efficiently. Intelligent data management accelerates the process by enabling faster access to real-time data, reducing the time spent on data gathering and analysis.
By leveraging AI and machine learning algorithms, Mastech InfoTrellis can automate data analysis, providing real-time insights into clinical trial results and research data. This accelerates the identification of promising drug candidates and speeds up the development process.
4. Real-Time Analytics for Better Decision-Making
In life sciences, every minute counts, especially during clinical trials and regulatory submissions. Intelligent data management systems provide pharmaceutical companies with real-time analytics that can help them make informed decisions faster.
By applying AI-powered analytics, pharmaceutical companies can quickly identify trends, predict outcomes, and optimize clinical trial strategies. This allows them to make data-backed decisions that improve drug efficacy, reduce adverse reactions, and ensure patient safety.
Mastech InfoTrellis: Transforming Data Management in the Pharmaceutical Industry
Mastech InfoTrellis is at the forefront of intelligent data management in the life sciences sector. The company's AI-first approach combines the power of Reltio MDM, Informatica CDGC, and AI-driven analytics to help pharmaceutical companies streamline their data management processes, improve data quality, and accelerate decision-making.
By leveraging Master Data Management (MDM) and Cloud Data Governance solutions, Mastech InfoTrellis empowers pharmaceutical companies to:
Integrate data from multiple sources for a unified view
Enhance data accuracy and integrity for better decision-making
Ensure compliance with global regulatory standards
Optimize the drug development process and improve time-to-market
Real-World Use Case: Improving Clinical Trial Efficiency
One real-world example of how intelligent data management is revolutionizing the pharmaceutical industry is the use of Mastech InfoTrellis's Reltio MDM solution in clinical trials. By integrating data from multiple trial sites, research teams, and regulatory bodies, Mastech InfoTrellis helped a major pharmaceutical company reduce the time spent on data gathering and processing by over 30%, enabling them to focus on analyzing results and making quicker decisions. This improvement led to a faster drug approval process and better patient outcomes.
People Also Ask
How does data management benefit the pharmaceutical industry?
Data management in the pharmaceutical industry ensures that all data, from clinical trials to regulatory filings, is accurate, accessible, and compliant with industry regulations. It helps streamline operations, improve decision-making, and speed up drug development.
What is the role of AI in pharmaceutical data management?
AI enhances pharmaceutical data management by automating data analysis, improving data accuracy, and providing real-time insights. AI-driven analytics allow pharmaceutical companies to identify trends, predict outcomes, and optimize clinical trials.
What are the challenges of data management in the pharmaceutical industry?
The pharmaceutical industry faces challenges such as data silos, regulatory compliance, and the sheer volume of data generated. Intelligent data management solutions help address these challenges by integrating data, automating governance, and providing real-time analytics.
Conclusion: The Future of Data Management in Life Sciences
Intelligent data management is no longer just an option for pharmaceutical companies—it's a necessity. With the power of AI, machine learning, and advanced data integration tools, Mastech InfoTrellis is helping pharmaceutical companies improve efficiency, compliance, and decision-making. By adopting these solutions, life sciences organizations can not only enhance their current operations but also position themselves for future growth and innovation.
As the pharmaceutical industry continues to evolve, intelligent data management will play a critical role in transforming how companies develop and deliver life-changing therapies to the market.
2 notes
·
View notes
Text

Thursday, March 27, 2025
It was an exellent exalent exalint excellant excellent day cleaning up PastPerfect data.
3 notes
·
View notes
Text
In the subject of data analytics, this is the most important concept that everyone needs to understand. The capacity to draw insightful conclusions from data is a highly sought-after talent in today's data-driven environment. In this process, data analytics is essential because it gives businesses the competitive edge by enabling them to find hidden patterns, make informed decisions, and acquire insight. This thorough guide will take you step-by-step through the fundamentals of data analytics, whether you're a business professional trying to improve your decision-making or a data enthusiast eager to explore the world of analytics.
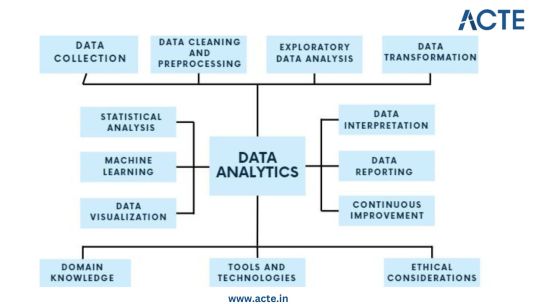
Step 1: Data Collection - Building the Foundation
Identify Data Sources: Begin by pinpointing the relevant sources of data, which could include databases, surveys, web scraping, or IoT devices, aligning them with your analysis objectives. Define Clear Objectives: Clearly articulate the goals and objectives of your analysis to ensure that the collected data serves a specific purpose. Include Structured and Unstructured Data: Collect both structured data, such as databases and spreadsheets, and unstructured data like text documents or images to gain a comprehensive view. Establish Data Collection Protocols: Develop protocols and procedures for data collection to maintain consistency and reliability. Ensure Data Quality and Integrity: Implement measures to ensure the quality and integrity of your data throughout the collection process.
Step 2: Data Cleaning and Preprocessing - Purifying the Raw Material
Handle Missing Values: Address missing data through techniques like imputation to ensure your dataset is complete. Remove Duplicates: Identify and eliminate duplicate entries to maintain data accuracy. Address Outliers: Detect and manage outliers using statistical methods to prevent them from skewing your analysis. Standardize and Normalize Data: Bring data to a common scale, making it easier to compare and analyze. Ensure Data Integrity: Ensure that data remains accurate and consistent during the cleaning and preprocessing phase.
Step 3: Exploratory Data Analysis (EDA) - Understanding the Data
Visualize Data with Histograms, Scatter Plots, etc.: Use visualization tools like histograms, scatter plots, and box plots to gain insights into data distributions and patterns. Calculate Summary Statistics: Compute summary statistics such as means, medians, and standard deviations to understand central tendencies. Identify Patterns and Trends: Uncover underlying patterns, trends, or anomalies that can inform subsequent analysis. Explore Relationships Between Variables: Investigate correlations and dependencies between variables to inform hypothesis testing. Guide Subsequent Analysis Steps: The insights gained from EDA serve as a foundation for guiding the remainder of your analytical journey.
Step 4: Data Transformation - Shaping the Data for Analysis
Aggregate Data (e.g., Averages, Sums): Aggregate data points to create higher-level summaries, such as calculating averages or sums. Create New Features: Generate new features or variables that provide additional context or insights. Encode Categorical Variables: Convert categorical variables into numerical representations to make them compatible with analytical techniques. Maintain Data Relevance: Ensure that data transformations align with your analysis objectives and domain knowledge.
Step 5: Statistical Analysis - Quantifying Relationships
Hypothesis Testing: Conduct hypothesis tests to determine the significance of relationships or differences within the data. Correlation Analysis: Measure correlations between variables to identify how they are related. Regression Analysis: Apply regression techniques to model and predict relationships between variables. Descriptive Statistics: Employ descriptive statistics to summarize data and provide context for your analysis. Inferential Statistics: Make inferences about populations based on sample data to draw meaningful conclusions.
Step 6: Machine Learning - Predictive Analytics
Algorithm Selection: Choose suitable machine learning algorithms based on your analysis goals and data characteristics. Model Training: Train machine learning models using historical data to learn patterns. Validation and Testing: Evaluate model performance using validation and testing datasets to ensure reliability. Prediction and Classification: Apply trained models to make predictions or classify new data. Model Interpretation: Understand and interpret machine learning model outputs to extract insights.
Step 7: Data Visualization - Communicating Insights
Chart and Graph Creation: Create various types of charts, graphs, and visualizations to represent data effectively. Dashboard Development: Build interactive dashboards to provide stakeholders with dynamic views of insights. Visual Storytelling: Use data visualization to tell a compelling and coherent story that communicates findings clearly. Audience Consideration: Tailor visualizations to suit the needs of both technical and non-technical stakeholders. Enhance Decision-Making: Visualization aids decision-makers in understanding complex data and making informed choices.
Step 8: Data Interpretation - Drawing Conclusions and Recommendations
Recommendations: Provide actionable recommendations based on your conclusions and their implications. Stakeholder Communication: Communicate analysis results effectively to decision-makers and stakeholders. Domain Expertise: Apply domain knowledge to ensure that conclusions align with the context of the problem.
Step 9: Continuous Improvement - The Iterative Process
Monitoring Outcomes: Continuously monitor the real-world outcomes of your decisions and predictions. Model Refinement: Adapt and refine models based on new data and changing circumstances. Iterative Analysis: Embrace an iterative approach to data analysis to maintain relevance and effectiveness. Feedback Loop: Incorporate feedback from stakeholders and users to improve analytical processes and models.
Step 10: Ethical Considerations - Data Integrity and Responsibility
Data Privacy: Ensure that data handling respects individuals' privacy rights and complies with data protection regulations. Bias Detection and Mitigation: Identify and mitigate bias in data and algorithms to ensure fairness. Fairness: Strive for fairness and equitable outcomes in decision-making processes influenced by data. Ethical Guidelines: Adhere to ethical and legal guidelines in all aspects of data analytics to maintain trust and credibility.
Data analytics is an exciting and profitable field that enables people and companies to use data to make wise decisions. You'll be prepared to start your data analytics journey by understanding the fundamentals described in this guide. To become a skilled data analyst, keep in mind that practice and ongoing learning are essential. If you need help implementing data analytics in your organization or if you want to learn more, you should consult professionals or sign up for specialized courses. The ACTE Institute offers comprehensive data analytics training courses that can provide you the knowledge and skills necessary to excel in this field, along with job placement and certification. So put on your work boots, investigate the resources, and begin transforming.
24 notes
·
View notes
Text
DNA technology now offers both data storage and computing functions for the first time
- By Nuadox Crew -
Researchers from North Carolina State University and Johns Hopkins University have developed a technology that uses DNA for both data storage and computing functions, enabling operations like storing, retrieving, erasing, and rewriting data, which was previously impossible with DNA-based systems.
Led by Albert Keung, the project aims to overcome challenges in integrating storage and processing, common in conventional computing, within a DNA framework.
The breakthrough relies on soft polymer structures called dendricolloids, which support high-density DNA storage. This structure allows for key functions like copying, erasing, and rewriting DNA data without damaging it. The team successfully solved basic computational problems with this technology, demonstrating its potential to store massive amounts of data securely for long periods.
The system, dubbed a “primordial DNA store and compute engine,” is a collaborative effort involving contributions from experts in microfluidics, sequencing, and data algorithms. Researchers hope this advancement will inspire future work in molecular computing, much like early computers sparked the digital age.
The research, published in Nature Nanotechnology, was funded by the National Science Foundation (NSF), with some researchers holding interests in commercializing DNA-based systems.
Read more at NC State University
Scientific paper: Lin, K.N., Volkel, K., Cao, C. et al. A primordial DNA store and compute engine. Nat. Nanotechnol. (2024). https://doi.org/10.1038/s41565-024-01771-6
--
Other recent news
Microscopy Breakthrough: Scientists at the Fritz Haber Institute of the Max Planck Society have developed a revolutionary microscopy technique that unveils hidden worlds. This could significantly advance our understanding of microscopic structures.
3 notes
·
View notes
Text
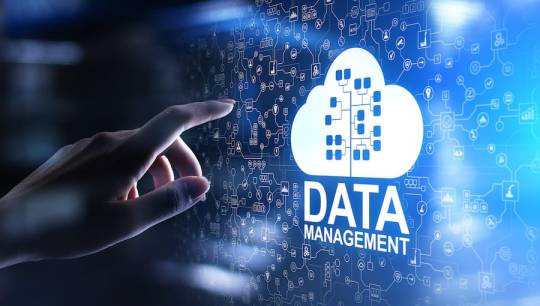
The Comprehensive Guide to Web Development, Data Management, and More
Introduction
Everything today is technology driven in this digital world. There's a lot happening behind the scenes when you use your favorite apps, go to websites, and do other things with all of those zeroes and ones — or binary data. In this blog, I will be explaining what all these terminologies really means and other basics of web development, data management etc. We will be discussing them in the simplest way so that this becomes easy to understand for beginners or people who are even remotely interested about technology. JOIN US
What is Web Development?
Web development refers to the work and process of developing a website or web application that can run in a web browser. From laying out individual web page designs before we ever start coding, to how the layout will be implemented through HTML/CSS. There are two major fields of web development — front-end and back-end.
Front-End Development
Front-end development, also known as client-side development, is the part of web development that deals with what users see and interact with on their screens. It involves using languages like HTML, CSS, and JavaScript to create the visual elements of a website, such as buttons, forms, and images. JOIN US
HTML (HyperText Markup Language):
HTML is the foundation of all website, it helps one to organize their content on web platform. It provides the default style to basic elements such as headings, paragraphs and links.
CSS (Cascading Style Sheets):
styles and formats HTML elements. It makes an attractive and user-friendly look of webpage as it controls the colors, fonts, layout.
JavaScript :
A language for adding interactivity to a website Users interact with items, like clicking a button to send in a form or viewing images within the slideshow. JOIN US
Back-End Development
The difference while front-end development is all about what the user sees, back end involves everything that happens behind. The back-end consists of a server, database and application logic that runs on the web.
Server:
A server is a computer that holds website files and provides them to the user browser when they request it. Server-Side: These are populated by back-end developers who build and maintain servers using languages like Python, PHP or Ruby.
Database:
The place where a website keeps its data, from user details to content and settings The database is maintained with services like MySQL, PostgreSQL, or MongoDB. JOIN US
Application Logic —
the code that links front-end and back-end It takes user input, gets data from the database and returns right informations to front-end area.
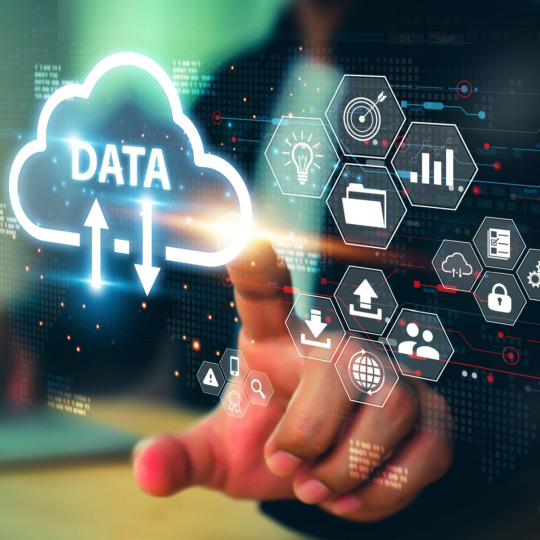
Why Proper Data Management is Absolutely Critical
Data management — Besides web development this is the most important a part of our Digital World. What Is Data Management? It includes practices, policies and procedures that are used to collect store secure data in controlled way.
Data Storage –
data after being collected needs to be stored securely such data can be stored in relational databases or cloud storage solutions. The most important aspect here is that the data should never be accessed by an unauthorized source or breached. JOIN US
Data processing:
Right from storing the data, with Big Data you further move on to process it in order to make sense out of hordes of raw information. This includes cleansing the data (removing errors or redundancies), finding patterns among it, and producing ideas that could be useful for decision-making.
Data Security:
Another important part of data management is the security of it. It refers to defending data against unauthorized access, breaches or other potential vulnerabilities. You can do this with some basic security methods, mostly encryption and access controls as well as regular auditing of your systems.
Other Critical Tech Landmarks
There are a lot of disciplines in the tech world that go beyond web development and data management. Here are a few of them:
Cloud Computing
Leading by example, AWS had established cloud computing as the on-demand delivery of IT resources and applications via web services/Internet over a decade considering all layers to make it easy from servers up to top most layer. This will enable organizations to consume technology resources in the form of pay-as-you-go model without having to purchase, own and feed that infrastructure. JOIN US
Cloud Computing Advantages:
Main advantages are cost savings, scalability, flexibility and disaster recovery. Resources can be scaled based on usage, which means companies only pay for what they are using and have the data backed up in case of an emergency.
Examples of Cloud Services:
Few popular cloud services are Amazon Web Services (AWS), Microsoft Azure, and Google Cloud. These provide a plethora of services that helps to Develop and Manage App, Store Data etc.
Cybersecurity
As the world continues to rely more heavily on digital technologies, cybersecurity has never been a bigger issue. Protecting computer systems, networks and data from cyber attacks is called Cyber security.
Phishing attacks, Malware, Ransomware and Data breaches:
This is common cybersecurity threats. These threats can bear substantial ramifications, from financial damages to reputation harm for any corporation.
Cybersecurity Best Practices:
In order to safeguard against cybersecurity threats, it is necessary to follow best-practices including using strong passwords and two-factor authorization, updating software as required, training employees on security risks.
Artificial Intelligence and Machine Learning
Artificial Intelligence (AI) and Machine Learning (ML) represent the fastest-growing fields of creating systems that learn from data, identifying patterns in them. These are applied to several use-cases like self driving cars, personalization in Netflix.
AI vs ML —
AI is the broader concept of machines being able to carry out tasks in a way we would consider “smart”. Machine learning is a type of Artificial Intelligence (AI) that provides computers with the ability to learn without being explicitly programmed. JOIN US
Applications of Artificial Intelligence and Machine Learning: some common applications include Image recognition, Speech to text, Natural language processing, Predictive analytics Robotics.
Web Development meets Data Management etc.
We need so many things like web development, data management and cloud computing plus cybersecurity etc.. but some of them are most important aspects i.e. AI/ML yet more fascinating is where these fields converge or play off each other.
Web Development and Data Management
Web Development and Data Management goes hand in hand. The large number of websites and web-based applications in the world generate enormous amounts of data — from user interactions, to transaction records. Being able to manage this data is key in providing a fantastic user experience and enabling you to make decisions based on the right kind of information.
E.g. E-commerce Website, products data need to be saved on server also customers data should save in a database loosely coupled with orders and payments. This data is necessary for customization of the shopping experience as well as inventory management and fraud prevention.
Cloud Computing and Web Development
The development of the web has been revolutionized by cloud computing which gives developers a way to allocate, deploy and scale applications more or less without service friction. Developers now can host applications and data in cloud services instead of investing for physical servers.
E.g. A start-up company can use cloud services to roll out the web application globally in order for all users worldwide could browse it without waiting due unavailability of geolocation prohibited access.
The Future of Cybersecurity and Data Management
Which makes Cybersecurity a very important part of the Data management. The more data collected and stored by an organization, the greater a target it becomes for cyber threats. It is important to secure this data using robust cybersecurity measures, so that sensitive information remains intact and customer trust does not weaken. JOIN US
Ex: A healthcare provider would have to protect patient data in order to be compliant with regulations such as HIPAA (Health Insurance Portability and Accountability Act) that is also responsible for ensuring a degree of confidentiality between a provider and their patients.
Conclusion
Well, in a nutshell web-developer or Data manager etc are some of the integral parts for digital world.
As a Business Owner, Tech Enthusiast or even if you are just planning to make your Career in tech — it is important that you understand these. With the progress of technology never slowing down, these intersections are perhaps only going to come together more strongly and develop into cornerstones that define how we live in a digital world tomorrow.
With the fundamental knowledge of web development, data management, automation and ML you will manage to catch up with digital movements. Whether you have a site to build, ideas data to manage or simply interested in what’s hot these days, skills and knowledge around the above will stand good for changing tech world. JOIN US
#Technology#Web Development#Front-End Development#Back-End Development#HTML#CSS#JavaScript#Data Management#Data Security#Cloud Computing#AWS (Amazon Web Services)#Cybersecurity#Artificial Intelligence (AI)#Machine Learning (ML)#Digital World#Tech Trends#IT Basics#Beginners Guide#Web Development Basics#Tech Enthusiast#Tech Career#america
4 notes
·
View notes
Text
#CRM benefits#customer relationship management#business success#increase sales#improve customer satisfaction#data management#sales automation#customer retention#CRM insights#streamline tasks#marketing automation
2 notes
·
View notes
Text
Elevate Your Data Strategy with Estelle Technologies
In today’s data-driven world, the need for efficient data management solutions is paramount. As businesses and organizations continue to generate vast amounts of data, the role of data management software companies becomes increasingly critical. These companies provide the tools and systems that allow businesses to organize, store, and analyze their data efficiently.
Estelle Technologies is at the forefront of this revolution, offering cutting-edge solutions that align with the best practices of leading data management software companies. With a focus on innovation, Estelle Technologies ensures that businesses can handle their data with precision, security, and ease.
Why is partnering with top data management software companies like Estelle Technologies essential for your business? Here’s what sets us apart:
Tailored Solutions: At Estelle Technologies, we understand that each business has unique needs. Our data management software is customizable, ensuring that your specific data requirements are met with the utmost efficiency.
Robust Security: In a world where data breaches are a significant concern, Estelle Technologies employs advanced security protocols to protect your valuable information. This is a hallmark of leading data management software companies.
Scalability: As your business grows, so does your data. Estelle Technologies’ solutions are scalable, ensuring that your data management processes can expand alongside your business.
User-Friendly Interface: We believe that managing your data shouldn’t be a complex task. Estelle Technologies designs intuitive interfaces that allow users of all skill levels to manage data seamlessly.
2 notes
·
View notes
Text
From Chaos to Clarity: The Importance of Streamlining Your Data Management Strategy
In the blog post "Streamlining Data Management Strategy," SG Analytics emphasizes the importance of a robust data management strategy in today's digital landscape. The article discusses the challenges posed by the explosion of data, such as data silos, security risks, and quality issues. The post provides a step-by-step guide to assess the current data landscape and implement a streamlined data management approach to harness the full potential of data assets. Read more : https://www.sganalytics.com/blog/streamlining-data-management-strategy/

3 notes
·
View notes
Text

Discovering the ideal Bachelor of Science in Data Management and Warehousing program is pivotal for aspiring data professionals. This guide delves into critical factors like program accreditation, curriculum depth, faculty expertise, and career prospects, aiding prospective students in selecting the best-fit data management degree. It highlights the significance of data warehousing, elucidates the nuances of data management, and underscores the diverse career opportunities awaiting graduates. Whether aspiring to study for a BSc in data management and warehousing or pursuing a data warehousing degree, this comprehensive overview equips individuals with essential insights for informed decision-making.
2 notes
·
View notes
Text
Activity 01
Following my review of the Gapminder study codebook, I have chosen to conduct an analysis of a global health concern: breast cancer. In conjunction with this primary focus, I will also explore the issue of suicide rates per hundred thousand individuals.
Question: Is breast cancer associated with suicide per 100th?
Variables: breastcancerper100th and suicideper100th
Hypothesis: Women diagnosed with breast cancer may be more likely to commit suicide compared to the general population.
Literature review:
Suicide After Breast Cancer: an International Population-Based Study of 723 810 Women
Catherine Schairer, Linda Morris Brown, Bingshu E. Chen, Regan Howard, Charles F. Lynch, Per Hall, Hans Storm, Eero Pukkala, Aage Anderson, Magnus Kaijser ... Show more
Summary: Few studies have examined long-term suicide risk among breast cancer survivors, and there are no data for women in the United States. We quantified suicide risk through 2002 among 723 810 1-year breast cancer survivors diagnosed between January 1, 1953, and December 31, 2001, and reported to 16 population-based cancer registries in the United States and Scandinavia. Among breast cancer survivors, we calculated standardized mortality ratios (SMRs) and excess absolute risks (EARs) compared with the general population, and the probability of suicide. We used Poisson regression likelihood ratio tests to assess heterogeneity in SMRs; all statistical tests were two-sided, with a .05 cutoff for statistical significance. In total 836 breast cancer patients committed suicide (SMR = 1.37, 95% confidence interval [CI] = 1.28 to 1.47; EAR = 4.1 per 100 000 person-years). Although SMRs ranged from 1.25 to 1.53 among registries, with 245 deaths among the sample of US women (SMR = 1.49, 95% CI = 1.32 to 1.70), differences among registries were not statistically significant ( P for heterogeneity = .19). Risk was elevated throughout follow-up, including for 25 or more years after diagnosis (SMR = 1.35, 95% CI = 0.82 to 2.12), and was highest among black women (SMR = 2.88, 95% CI = 1.44 to 5.17) ( P for heterogeneity = .06). Risk increased with increasing stage of breast cancer ( P for heterogeneity = .08) and remained elevated among women diagnosed between 1990 and 2001 (SMR = 1.36, 95% CI = 1.18 to 1.57). The cumulative probability of suicide was 0.20% 30 years after breast cancer diagnosis.
Topic: cancerheterogeneityearfollow-upscandinaviasurvivorsdiagnosissuicidebreast cancerlikelihood ratiosuicidal behaviortnm breast tumor stagingstandardized mortality ratio
Issue Section: Brief Communications
References:
(1) Rowland J, Mariotto A, Aziz N, Tesauro G, Feuer EJ, Blackman D, et al. Cancer survivorship — United States, 1971 – 2001. MMWR Morb Mortal Wkly Rep 2004 ; 53 : 526 – 9.
(2) Ries LAG, Eisner MP, Kosary CL, Hankey BF, Miller BA, Clegg L, et al., editors. SEER cancer statistics review, 1975 – 2002. Bethesda (MD): National Cancer Institute; 2004. Available at: http://seer.cancer.gov/csr/1975_2002 . [Last accessed: September 22, 2005.]
(3) Yousaf U, Christensen M-LM, Engholm G, Storm HH. Suicides among Danish cancer patients 1971 – 1999. Br J Cancer 2005 ; 92 : 995 – 1000.
2 notes
·
View notes
Text
THE IMPORTANCE OF DATA MANAGEMENT AND DATA SECURITY IN HEALTHCARE
Data is the lifeblood of the healthcare industry. It encompasses patient records, treatment plans, medical histories, and an array of vital information that healthcare providers rely on to deliver the best possible care. As the healthcare landscape becomes increasingly digital, the importance of data management and data security has never been more critical. In this Blog, we’ll delve into the significance of safeguarding patient data and how E_care, a leader in healthcare management software, prioritizes data security to protect sensitive patient information.
Preserving Patient Confidentiality:
Compliance with Data Protection Regulations:
The Importance of Data Management & Data Security in Healthcare
Data Encryption:
Access Control:
Data Backups and Recovery:
Security Audits and Monitoring:
Education and Training:
Data Security as a Competitive Advantage:
E Care Hospital Management Software
Our Social medias:
LinkedIn: E CARE - Hospital Management Software
Facebook: E-care
Instagram: e_care_hms
X: E-Care Hospital Management Software
#e care#hms#hospital management software#hospital management system#health#data management#data security
3 notes
·
View notes
Text

Wednesday, February 19, 2025
I spent the day cleaning up the People Biographies file in our PastPerfect database.
2 notes
·
View notes