#data analytics vs data science
Explore tagged Tumblr posts
Text
Best Big Data Hadoop Course: Unlocking the Power of Data Analytics
Unlock the power of data analytics with the best Big Data Hadoop course. Gain in-depth knowledge and practical skills to harness the potential of big data. Our comprehensive training program covers all aspects of Hadoop, including data storage, processing, and analysis. Learn from industry experts who have hands-on experience in handling large-scale data projects. Discover how to leverage Hadoop's distributed computing framework to extract valuable insights and make data-driven decisions. With our cutting-edge curriculum and hands-on exercises, you'll acquire the expertise to work with big data efficiently and effectively. Take the first step towards a successful career in data analytics. Enroll in the best Big Data Hadoop course today and unlock a world of opportunities.
#Power of Data Analytics#Power of data analytics examples#Power of data analytics in business#data analytics examples#importance of data analytics#data analytics vs data analysis#why data analytics is the future of everything#data analytics vs data science#future of data analytics
0 notes
Text
Data Science vs. Business Analytics
Business Analytics and Data Science are two prominent fields that revolve around data-driven decision-making, yet they serve distinct purposes. Business Analytics primarily focuses on analyzing historical and current business data to improve processes and guide corporate strategies. It employs statistical methods, visualization tools, and business modeling techniques to derive insights that help organizations optimize operations and achieve their goals. On the other hand, Data Science goes beyond business applications by working with both structured and unstructured data to develop predictive and prescriptive models. It leverages programming, machine learning, and artificial intelligence to extract deeper patterns and automate decision-making processes.
While Business Analytics requires fundamental coding skills and a strong grasp of statistical analysis, Data Science demands proficiency in programming languages like Python and R, as well as expertise in advanced algorithms. The career paths in these fields also differ significantly. Business Analysts, IT Analysts, and Market Analysts are common roles in the business analytics domain, whereas Data Scientists, Machine Learning Engineers, and Data Architects dominate the data science landscape. Both fields offer lucrative career opportunities, but their focus and methodologies distinguish them. Business Analytics is ideal for those looking to enhance corporate efficiency, while Data Science is suited for individuals interested in innovation and complex problem-solving.
Click here to learn more.
#DataScienceVsBusinessAnalytics#business analytics#datasciencecareers#AnalyticsVsDataScience#TechAndBusinessInsights#data science vs business analytics#difference between data science and business analytics
1 note
·
View note
Text
Data Scientist vs. Data Engineer: Key Differences & Career Insights

Data is everywhere in today's world. Whatever apps we use or whatever websites we visit, everything is based on data. But have you ever thought about who does all this magic behind data? There are two very important roles: Data Scientists and Data Engineers. Though they deal with data, their jobs are completely different.
So, let's break it down in basic terms and go through the main key differences between a Data scientist vs Data engineer to help you decide which path might be right for you.
Difference Between Data Engineer And Data Scientist
In Data engineer vs Data scientist think of a Data Engineer as the person who designs the foundation and building of structures to allow data to flow well. Their job involves making sure data gets collected stored, and kept ready to analyze. A Data Scientist, however, works like a detective to use data to uncover patterns, trends, and insights that help businesses make smart choices.
Educational Requirements
In India, skill sets are more crucial than degree requirements for becoming a successful Data Scientist vs Data Engineer. You may observe many successful Data Scientists and Data Engineers from various educational backgrounds who are excelling in this industry, but if becoming a data scientist or data engineer is your ultimate aim, then studying data analytics courses in college can undoubtedly help you become the greatest.
Educational qualification to become a Data Scientist.
1. You will need a bachelor's degree in Computer Science, Mathematics, Statistics, or Engineering to start a career in data science. To get better opportunities you can also go for a master's and PHD degree.
2. If you have already completed your degree in another stream and then chose data analysis as your career short-duration data analyst course can help you with that.
3. Familiarity with data analysis, SQL, Excel, and software such as Tableau or Power BI are also important to be a good data scientist.
4. A good understanding of statistics and probability is important for analyzing data correctly.
Educational qualification to become a Data Engineer.
1. A bachelor's degree in Computer Science, Information Technology, or Engineering.
2. Strong programming skills in languages like Python, Java, or Scala. You also need to know databases and data processing frameworks.
3. Tools: Experience with cloud platforms (AWS, Google Cloud) and managing data pipelines (such as Apache Kafka or Hadoop) is important for designing efficient data systems.
4. The ability to solve problems and design systems that store and process large amounts of data efficiently.
Career Tips: Getting a Job as a Data Science VS Data Engineering
Here are some tips that can help you get jobs in data science vs data engineering.
Learn the Basics
Start by learning the basics of programming, databases, and math. These are the foundation for both data engineer vs data scientist. Once you understand these, you'll be able to learn more advanced topics easily.
Online Courses
There are many free online courses that teach skills like Python, SQL, and machine learning. Take advantage of these courses to learn at your own pace and build your knowledge step by step.
Hands-On Practice
Theory is important, but nothing beats practical experience. Work on small projects, such as analyzing datasets or building basic machine learning models. Use platforms like Kaggle to practice with real-world datasets.
Internships
Look for internships or part-time jobs related to data. Even a small role can help you gain experience and make your resume stronger in the data engineer vs data scientist vs data analyst field. You’ll also learn a lot by working in a real-world environment.
Stay Updated
The tech world moves quickly, so it’s important to keep learning new tools and techniques related to data engineer vs data analyst. Stay up-to-date to stay competitive in your field.
Networking
Connect with others in the field. Join online communities, attend meetups, or follow experts on social media. Networking can help you learn from others and lead to job opportunities in the data engineer vs data scientist field.
How to Enhance Your Job Application
To enhance your job application in data science vs data engineering, follow these simple tips.
Tailor Your Resume: Customize your resume to match the job you're applying for. Highlight your skills and experience that are most relevant to the role.
Write a Strong Cover Letter: Write a short, personalized cover letter and mention why you want the job and why you’re a great fit.
Showcase Your Achievements: Focus on your accomplishments, not just your responsibilities. Use numbers to show your impact if possible.
Keep it short and simple: Avoid big words or complicated sentences. Make everything easy to read and straightforward.
Proofread: Always look for errors. You may look careless to interviewers if one simple mistake occurs.
Be honest: Never exaggerate and write what you can not explain. Employers value honesty most of all.
Comparison Of Salary: Data Engineer VS Data Scientist
Both Data Engineers and Data Scientists have high-paying jobs, but data scientist vs data engineer salaries can vary a bit. In India, the average data science engineer salary is between ₹9,00,000 and ₹22,00,000, depending on their skills like machine learning and analytics, which are in high demand. If you're a senior Data Scientist, you could earn ₹25,00,000 or even more. Additionally, if you're considering a career as a data analyst, the data analyst job salary typically ranges between ₹4,00,000 and ₹8,00,000, depending on experience and expertise.
On the other hand, a Data Engineer's salary is usually between ₹7,00,000 and ₹15,00,000, depending on the type of work and experience. If you have knowledge of cloud computing and big data tools, your salary can be higher. Also, these are the estimates, it also depends on the company you are working with and the knowledge you have gained.
Career Growth and Pathways
The career growth you can expect as a Data engineer vs Data analyst or scientist.
Career Pathway for Data Engineers.
Starting Out: You might begin as a junior data engineer or a data analyst. This helps you understand how to handle data and learn the tools.
Mid-Level: After gaining some experience, you can become a senior data engineer. At this stage, you’ll take on bigger projects and maybe even manage teams.
Top-Level: With more experience, you could become a lead data engineer or even a chief data officer. These roles involve more decision-making and overseeing larger data systems for the company.
Career Pathway for Data Scientists.
Starting Out: Most people start as junior data scientists or data analysts. This is where you learn how to work with data and do basic analysis.
Mid-Level: As you gain experience, you can become a senior data scientist. You’ll work on bigger problems, analyze more complex data, and might even guide junior team members.
Top-Level: At this level, you can become a lead data scientist or a machine learning engineer. These positions involve using advanced techniques to solve difficult problems and sometimes creating new tools for analysis.
Conclusion
If you're looking to master Python, Analytics Shiksha is the perfect choice. Their Super30 Analytics course, part of their data analytics courses online, offers comprehensive training in data analysis and job preparation. Don’t miss out—secure your spot today, as only 30 seats are available!
The opportunities in both fields are immense, and with the right skills, you can make your mark in the data world.
Be sure to enroll and reserve your spot in our Super30 data analytics course to advance your skills. This program is built around problem-solving techniques and allows you to work with real data.
Frequently Asked Questions: Data Engineer vs. Data Scientist
Can Data Scientists Transition with Data Analytics Courses to Become Data Engineers?
Yes, by increasing knowledge of coding, databases, and system design through data analytics courses, a data scientist can easily become a data engineer.
Which is Better: Data Scientist Data Engineer vs Data Scientist?
It depends on what you enjoy doing to solve problems and make predictions or to build the infrastructure and tools to store and process data. If you love analyzing data and creating models, a data scientist role might be better. If you prefer working on technology and systems, a data engineer job could be the right fit for you.
What is The Difference Between Data Engineer and Data Scientist
A data engineer works on systems that collect, store, and process data. They make sure the data is clean and ready for analysis. A data scientist analyzes the data, creates models, and discovers insights to help businesses solve problems. In a nutshell, engineers prepare the data, while scientists analyze it. To excel in either role, enrolling in data analytics courses can help you build the necessary skills and expertise.
#data analytics courses#data analytics courses in Delhi#data analytics courses online#data analyst job salary#data science vs data engineering
0 notes
Text
Data Science vs Data Analytics: A Complete Guide for Beginners
In today’s data-driven world, understanding the difference between Data Science vs Data Analytics is essential for anyone looking to pursue a career in these fields. Both disciplines revolve around data, yet they serve different purposes and require distinct skill sets. This guide will help beginners navigate the key differences and applications of Data Science vs Data Analytics to determine the best fit for their interests and goals.
What is Data Science?
Data Science is a multidisciplinary field that combines statistics, programming, and domain knowledge to extract insights from structured and unstructured data. It involves using advanced tools and techniques like machine learning, artificial intelligence (AI), and big data analytics to predict future trends, automate decision-making, and solve complex problems.

Data scientists work on diverse tasks, such as building predictive models, developing algorithms, and exploring large datasets to uncover hidden patterns. The field requires proficiency in programming languages like Python and R, as well as expertise in frameworks like TensorFlow and Apache Spark. Applications of Data Science span industries, including healthcare, finance, marketing, and e-commerce.
What is Data Analytics?
Data Analytics, on the other hand, focuses on analyzing historical data to provide actionable insights and answer specific business questions. It is a subset of Data Science but with a narrower scope. Data analysts use tools like Excel, Tableau, and SQL to interpret data, create reports, and make recommendations that drive business decisions.
Unlike Data Science, which is more exploratory and predictive, Data Analytics is descriptive and diagnostic. Analysts look at past performance to identify trends, patterns, and opportunities for improvement. Common applications include customer behavior analysis, sales forecasting, and operational optimization in industries like retail, logistics, and hospitality.
Key Differences Between Data Science and Data Analytics
Scope: Data Science is broad and future-oriented, while Data Analytics is focused on specific problems and past data.
Techniques: Data Science leverages advanced technologies like machine learning, while Data Analytics relies on simpler tools for data interpretation.
Outcome: Data Science aims to create predictive models, whereas Data Analytics delivers actionable insights.
Choosing the Right Path
Whether you choose Data Science vs Data Analytics depends on your interests and career aspirations. If you’re intrigued by programming, AI, and solving complex problems, Data Science is ideal. If you enjoy interpreting data to make strategic decisions, Data Analytics is the way to go.
By understanding the differences between Data Science vs Data Analytics, you can make an informed decision and embark on a rewarding career in the data domain.
0 notes
Text
Data Science and Engineering Driving industry Innovations
The integration of data science and engineering is revolutionizing industries, enabling smarter decision-making, process optimization, and predictive capabilities. At M.Kumaraswamy College of Engineering (MKCE), students are equipped to harness data science to solve complex challenges and drive innovation. By combining theoretical knowledge with practical applications, MKCE prepares students to optimize processes in manufacturing, healthcare, transportation, energy, and urban planning. The curriculum includes courses on machine learning, big data analytics, and programming, alongside hands-on projects and internships. MKCE’s focus on industry collaborations ensures students stay ahead of emerging trends like AI, IoT, and digital twins. This interdisciplinary approach empowers students to lead in data-driven industries and shape the future of engineering.
To Know More : https://mkce.ac.in/blog/data-science-and-engineering-driving-innovation-across-industries/
#best engineering college#mkce college#private college#engineering college#top 10 colleges in tn#libary#best engineering college in karur#engineering college in karur#mkce.ac.in#mkce#Data Science#Engineering Innovation#Predictive Maintenance#Process Optimization#Machine Learning#Big Data Analytics#Smart Manufacturing#Healthcare Engineering#Smart Cities#mkce placement#cse future#ece full form in engineering#ece job roles#mkce fees payment#cse vs mechanical engineering
0 notes
Text
Critical Differences: Between Database vs Data Warehouse
Summary: This blog explores the differences between databases and data warehouses, highlighting their unique features, uses, and benefits. By understanding these distinctions, you can select the optimal data management solution to support your organisation’s goals and leverage cloud-based options for enhanced scalability and efficiency.
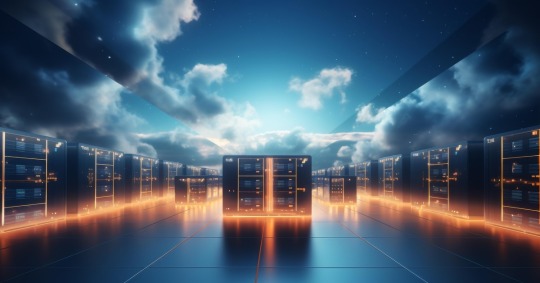
Introduction
Effective data management is crucial for organisational success in today's data-driven world. Understanding the concepts of databases and data warehouses is essential for optimising data use. Databases store and manage transactional data efficiently, while data warehouses aggregate and analyse large volumes of data for strategic insights.
This blog aims to clarify the critical differences between databases and data warehouses, helping you decide which solution best fits your needs. By exploring "database vs. data warehouse," you'll gain valuable insights into their distinct roles, ensuring your data infrastructure effectively supports your business objectives.
What is a Database?
A database is a structured collection of data that allows for efficient storage, retrieval, and management of information. It is designed to handle large volumes of data and support multiple users simultaneously.
Databases provide a systematic way to organise, manage, and retrieve data, ensuring consistency and accuracy. Their primary purpose is to store data that can be easily accessed, manipulated, and updated, making them a cornerstone of modern data management.
Common Uses and Applications
Databases are integral to various applications across different industries. Businesses use databases to manage customer information, track sales and inventory, and support transactional processes.
In the healthcare sector, databases store patient records, medical histories, and treatment plans. Educational institutions use databases to manage student information, course registrations, and academic records.
E-commerce platforms use databases to handle product catalogues, customer orders, and payment information. Databases also play a crucial role in financial services, telecommunications, and government operations, providing the backbone for data-driven decision-making and efficient operations.
Types of Databases
Knowing about different types of databases is crucial for making informed decisions in data management. Each type offers unique features for specific tasks. There are several types of databases, each designed to meet particular needs and requirements.
Relational Databases
Relational databases organise data into tables with rows and columns, using structured query language (SQL) for data manipulation. They are highly effective for handling structured data and maintaining relationships between different data entities. Examples include MySQL, PostgreSQL, and Oracle.
NoSQL Databases
NoSQL databases are designed to handle unstructured and semi-structured data, providing flexibility in data modelling. They are ideal for high scalability and performance applications like social media and big data. Types of NoSQL databases include:
Document databases (e.g., MongoDB).
Key-value stores (e.g., Redis).
Column-family stores (e.g., Cassandra).
Graph databases (e.g., Neo4j).
In-Memory Databases
In-memory databases store data in the main memory (RAM) rather than on disk, enabling high-speed data access and processing. They are suitable for real-time applications that require low-latency data retrieval, such as caching and real-time analytics. Examples include Redis and Memcached.
NewSQL Databases
NewSQL databases aim to provide the scalability of NoSQL databases while maintaining the ACID (Atomicity, Consistency, Isolation, Durability) properties of traditional relational databases. They are used in applications that require high transaction throughput and firm consistency. Examples include Google Spanner and CockroachDB.
Examples of Database Management Systems (DBMS)
Understanding examples of Database Management Systems (DBMS) is essential for selecting the right tool for your data needs. DBMS solutions offer varied features and capabilities, ensuring better performance, security, and integrity across diverse applications. Some common examples of Database Management Systems (DBMS) are:
MySQL
MySQL is an open-source relational database management system known for its reliability, performance, and ease of use. It is widely used in web applications, including popular platforms like WordPress and Joomla.
PostgreSQL
PostgreSQL is an advanced open-source relational database system that supports SQL and NoSQL data models. It is known for its robustness, extensibility, and standards compliance, making it suitable for complex applications.
MongoDB
MongoDB is a leading NoSQL database that stores data in flexible, JSON-like documents. It is designed for scalability and performance, making it a popular choice for modern applications that handle large volumes of unstructured data.
Databases form the foundation of data management in various domains, offering diverse solutions to meet specific data storage and retrieval needs. By understanding the different types of databases and their applications, organisations can choose the proper database technology to support their operations.
Read More: What are Attributes in DBMS and Its Types?
What is a Data Warehouse?
A data warehouse is a centralised repository designed to store, manage, and analyse large volumes of data. It consolidates data from various sources, enabling organisations to make informed decisions through comprehensive data analysis and reporting.
A data warehouse is a specialised system optimised for query and analysis rather than transaction processing. It is structured to enable efficient data retrieval and analysis, supporting business intelligence activities. The primary purpose of a data warehouse is to provide a unified, consistent data source for analytical reporting and decision-making.
Common Uses and Applications
Data warehouses are commonly used in various industries to enhance decision-making processes. Businesses use them to analyse historical data, generate reports, and identify trends and patterns. Applications include sales forecasting, financial analysis, customer behaviour, and performance tracking.
Organisations leverage data warehouses to gain insights into operations, streamline processes, and drive strategic initiatives. By integrating data from different departments, data warehouses enable a holistic view of business performance, supporting comprehensive analytics and business intelligence.
Key Features of Data Warehouses
Data warehouses offer several key features that distinguish them from traditional databases. These features make data warehouses ideal for supporting complex queries and large-scale data analysis, providing organisations with the tools for in-depth insights and informed decision-making. These features include:
Data Integration: Data warehouses consolidate data from multiple sources, ensuring consistency and accuracy.
Scalability: They are designed to handle large volumes of data and scale efficiently as data grows.
Data Transformation: ETL (Extract, Transform, Load) processes clean and organise data, preparing it for analysis.
Performance Optimisation: Data warehouses enhance query performance using indexing, partitioning, and parallel processing.
Historical Data Storage: They store historical data, enabling trend analysis and long-term reporting.
Read Blog: Top ETL Tools: Unveiling the Best Solutions for Data Integration.
Examples of Data Warehousing Solutions
Several data warehousing solutions stand out in the industry, offering unique capabilities and advantages. These solutions help organisations manage and analyse data more effectively, driving better business outcomes through robust analytics and reporting capabilities. Prominent examples include:
Amazon Redshift
Amazon Redshift is a fully managed, petabyte-scale data warehouse service in the cloud. It is designed to handle complex queries and large datasets, providing fast query performance and easy scalability.
Google BigQuery
Google BigQuery is a serverless, highly scalable, cost-effective multi-cloud data warehouse that enables super-fast SQL queries using the processing power of Google's infrastructure.
Snowflake
Snowflake is a cloud data platform that provides data warehousing, data lakes, and data sharing capabilities. It is known for its scalability, performance, and ability to handle diverse data workloads.
Key Differences Between Databases and Data Warehouses
Understanding the distinctions between databases and data warehouses is crucial for selecting the right data management solution. This comparison will help you grasp their unique features, use cases, and data-handling methods.
Databases and data warehouses serve distinct purposes in data management. While databases handle transactional data and support real-time operations, data warehouses are indispensable for advanced data analysis and business intelligence. Understanding these key differences will enable you to choose the right solution based on your specific data needs and goals.
Choosing Between a Database and a Data Warehouse
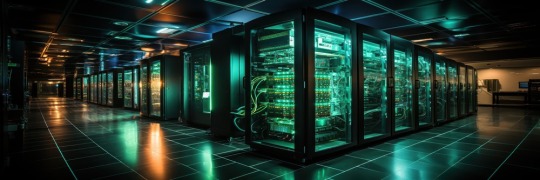
Several critical factors should guide your decision-making process when deciding between a database and a data warehouse. These factors revolve around the nature, intended use, volume, and complexity of data, as well as specific use case scenarios and cost implications.
Nature of the Data
First and foremost, consider the inherent nature of your data. Suppose you focus on managing transactional data with frequent updates and real-time access requirements. In that case, a traditional database excels in this operational environment.
On the other hand, a data warehouse is more suitable if your data consists of vast historical records and complex data models and is intended for analytical processing to derive insights.
Intended Use: Operational vs. Analytical
The intended use of the data plays a pivotal role in determining the appropriate solution. Operational databases are optimised for transactional processing, ensuring quick and efficient data manipulation and retrieval.
Conversely, data warehouses are designed for analytical purposes, facilitating complex queries and data aggregation across disparate sources for business intelligence and decision-making.
Volume and Complexity of Data
Consider the scale and intricacy of your data. Databases are adept at handling moderate to high volumes of structured data with straightforward relationships. In contrast, data warehouses excel in managing vast amounts of both structured and unstructured data, often denormalised for faster query performance and analysis.
Use Case Scenarios
Knowing when to employ each solution is crucial. Use a database when real-time data processing and transactional integrity are paramount, such as in e-commerce platforms or customer relationship management systems. Opt for a data warehouse when conducting historical trend analysis, business forecasting, or consolidating data from multiple sources for comprehensive reporting.
Cost Considerations
Finally, weigh the financial aspects of your decision. Databases typically involve lower initial setup costs and are easier to scale incrementally. In contrast, data warehouses may require more substantial upfront investments due to their complex infrastructure and storage requirements.
To accommodate your budgetary constraints, factor in long-term operational costs, including maintenance, storage, and data processing fees.
By carefully evaluating these factors, you can confidently select the database or data warehouse solution that best aligns with your organisation's specific needs and strategic objectives.
Cloud Databases and Data Warehouses
Cloud-based solutions have revolutionised data management by offering scalable, flexible, and cost-effective alternatives to traditional on-premises systems. Here's an overview of how cloud databases and data warehouses transform modern data architectures.
Overview of Cloud-Based Solutions
Cloud databases and data warehouses leverage the infrastructure and services provided by cloud providers like AWS, Google Cloud, and Microsoft Azure. They eliminate the need for physical hardware and offer pay-as-you-go pricing models, making them ideal for organisations seeking agility and scalability.
Advantages of Cloud Databases and Data Warehouses
The primary advantages include scalability to handle fluctuating workloads, reduced operational costs by outsourcing maintenance and updates to the cloud provider and enhanced accessibility for remote teams. Cloud solutions facilitate seamless integration with other cloud services and tools, promoting collaboration and innovation.
Popular Cloud Providers and Services
Leading providers such as AWS with Amazon RDS and Google Cloud's Cloud SQL offer managed database services supporting engines like MySQL, PostgreSQL, and SQL Server. For data warehouses, options like AWS Redshift, Google BigQuery, and Azure Synapse Analytics provide powerful analytical capabilities with elastic scaling and high performance.
Security and Compliance Considerations
Despite the advantages, security remains a critical consideration. Cloud providers implement robust security measures, including encryption, access controls, and compliance certifications (e.g., SOC 2, GDPR, HIPAA).
Organisations must assess data residency requirements and ensure adherence to industry-specific regulations when migrating sensitive data to the cloud.
By embracing cloud databases and data warehouses, organisations can optimise data management, drive innovation, and gain competitive advantages in today's data-driven landscape.
Frequently Asked Questions
What is the main difference between a database and a data warehouse?
A database manages transactional data for real-time operations, supporting sales and inventory management activities. In contrast, a data warehouse aggregates and analyses large volumes of historical data, enabling strategic insights, comprehensive reporting, and business intelligence activities critical for informed decision-making.
When should I use a data warehouse over a database?
Use a data warehouse when your primary goal is to conduct historical data analysis, generate complex queries, and create comprehensive reports. A data warehouse is ideal for business intelligence, trend analysis, and strategic planning, consolidating data from multiple sources for a unified, insightful view of your operations.
How do cloud databases and data warehouses benefit organisations?
Cloud databases and data warehouses provide significant advantages, including scalability to handle varying workloads, reduced operational costs due to outsourced maintenance, and enhanced accessibility for remote teams. They integrate seamlessly with other cloud services, promoting collaboration, innovation, and data management and analysis efficiency.
Conclusion
Understanding the critical differences between databases and data warehouses is essential for effective data management. Databases excel in handling transactional data, ensuring real-time updates and operational efficiency.
In contrast, data warehouses are designed for in-depth analysis, enabling strategic decision-making through comprehensive data aggregation. You can choose the solution that best aligns with your organisation's needs by carefully evaluating factors like data nature, intended use, volume, and cost.
Embracing cloud-based options further enhances scalability and flexibility, driving innovation and competitive advantage in today’s data-driven world. Choose wisely to optimise your data infrastructure and achieve your business objectives.
#Differences Between Database and Data Warehouse#Database vs Data Warehouse#Database#Data Warehouse#data management#data analytics#data storage#data science#pickl.ai#data analyst
0 notes
Text
#data science vs data analytics#data science#data analytics#comparison#quick insights#data science career
0 notes
Text
Unveiling the Distinctions Between Data Science and Data Analytics
Explore the intricate realms of data as we embark on a journey of discovery, "Unlocking the Key Differences Between Data Science and Data Analytics." Delve into the core of these dynamic fields, gaining insights into their distinct methodologies, tools, and applications. This enlightening exploration navigates through the complexities, unraveling the nuanced threads that set data science and data analytics apart. Whether you're a seasoned professional or a curious enthusiast, this comprehensive analysis unveils the essential distinctions, empowering you to make informed decisions in the data-driven landscape. Embrace the knowledge that transcends the surface, and embark on a deeper understanding of the pivotal disparities shaping the future of data science and analytics.
0 notes
Text
Python for Beginners: Launch Your Tech Career with Coding Skills
Are you ready to launch your tech career but don’t know where to start? Learning Python is one of the best ways to break into the world of technology—even if you have zero coding experience.
In this guide, we’ll explore how Python for beginners can be your gateway to a rewarding career in software development, data science, automation, and more.
Why Python Is the Perfect Language for Beginners
Python has become the go-to programming language for beginners and professionals alike—and for good reason:
Simple syntax: Python reads like plain English, making it easy to learn.
High demand: Industries spanning the spectrum are actively seeking Python developers to fuel their technological advancements.
Versatile applications: Python's versatility shines as it powers everything from crafting websites to driving artificial intelligence and dissecting data.
Whether you want to become a software developer, data analyst, or AI engineer, Python lays the foundation.
What Can You Do With Python?
Python is not just a beginner language—it’s a career-building tool. Here are just a few career paths where Python is essential:
Web Development: Frameworks like Django and Flask make it easy to build powerful web applications. You can even enroll in a Python Course in Kochi to gain hands-on experience with real-world web projects.
Data Science & Analytics: For professionals tackling data analysis and visualization, the Python ecosystem, featuring powerhouses like Pandas, NumPy, and Matplotlib, sets the benchmark.
Machine Learning & AI: Spearheading advancements in artificial intelligence development, Python boasts powerful tools such as TensorFlow and scikit-learn.
Automation & Scripting: Simple yet effective Python scripts offer a pathway to amplified efficiency by automating routine workflows.
Cybersecurity & Networking: The application of Python is expanding into crucial domains such as ethical hacking, penetration testing, and the automation of network processes.
How to Get Started with Python
Starting your Python journey doesn't require a computer science degree. Success hinges on a focused commitment combined with a thoughtfully structured educational approach.
Step 1: Install Python
Download and install Python from python.org. It's free and available for all platforms.
Step 2: Choose an IDE
Use beginner-friendly tools like Thonny, PyCharm, or VS Code to write your code.
Step 3: Learn the Basics
Focus on:
Variables and data types
Conditional statements
Loops
Functions
Lists and dictionaries
If you prefer guided learning, a reputable Python Institute in Kochi can offer structured programs and mentorship to help you grasp core concepts efficiently.
Step 4: Build Projects
Learning by doing is key. Start small:
Build a calculator
Automate file organization
Create a to-do list app
As your skills grow, you can tackle more complex projects like data dashboards or web apps.
How Python Skills Can Boost Your Career
Adding Python to your resume instantly opens up new opportunities. Here's how it helps:
Higher employability: Python is one of the top 3 most in-demand programming languages.
Better salaries: Python developers earn competitive salaries across the globe.
Remote job opportunities: Many Python-related jobs are available remotely, offering flexibility.
Even if you're not aiming to be a full-time developer, Python skills can enhance careers in marketing, finance, research, and product management.
If you're serious about starting a career in tech, learning Python is the smartest first step you can take. It’s beginner-friendly, powerful, and widely used across industries.
Whether you're a student, job switcher, or just curious about programming, Python for beginners can unlock countless career opportunities. Invest time in learning today—and start building the future you want in tech.
Globally recognized as a premier educational hub, DataMites Institute delivers in-depth training programs across the pivotal fields of data science, artificial intelligence, and machine learning. They provide expert-led courses designed for both beginners and professionals aiming to boost their careers.
Python Modules Explained - Different Types and Functions - Python Tutorial
youtube
#python course#python training#python#learnpython#pythoncourseinindia#pythoncourseinkochi#pythoninstitute#python for data science#Youtube
3 notes
·
View notes
Text
Business Analytics vs. Data Science: Understanding the Key Differences
In today's data-driven world, terms like "business analytics" and "data science" are often used interchangeably. However, while they share a common goal of extracting insights from data, they are distinct fields with different focuses and methodologies. Let's break down the key differences to help you understand which path might be right for you.
Business Analytics: Focusing on the Present and Past
Business analytics primarily focuses on analyzing historical data to understand past performance and inform current business decisions. It aims to answer questions like:
What happened?
Why did it happen?
What is happening now?
Key characteristics of business analytics:
Descriptive and Diagnostic: It uses techniques like reporting, dashboards, and data visualization to summarize and explain past trends.
Structured Data: It often works with structured data from databases and spreadsheets.
Business Domain Expertise: A strong understanding of the specific business domain is crucial.
Tools: Business analysts typically use tools like Excel, SQL, Tableau, and Power BI.
Focus: Optimizing current business operations and improving efficiency.
Data Science: Predicting the Future and Building Models
Data science, on the other hand, focuses on building predictive models and developing algorithms to forecast future outcomes. It aims to answer questions like:
What will happen?
How can we make it happen?
Key characteristics of data science:
Predictive and Prescriptive: It uses machine learning, statistical modeling, and AI to predict future trends and prescribe optimal actions.
Unstructured and Structured Data: It can handle both structured and unstructured data from various sources.
Technical Proficiency: Strong programming skills (Python, R) and a deep understanding of machine learning algorithms are essential.
Tools: Data scientists use programming languages, machine learning libraries, and big data technologies.
Focus: Developing innovative solutions, building AI-powered products, and driving long-term strategic initiatives.
Key Differences Summarized:

Which Path is Right for You?
Choose Business Analytics if:
You are interested in analyzing past data to improve current business operations.
You have a strong understanding of a specific business domain.
You prefer working with structured data and using visualization tools.
Choose Data Science if:
You are passionate about building predictive models and developing AI-powered solutions.
You have a strong interest in programming and machine learning.
You enjoy working with both structured and unstructured data.
Xaltius Academy's Data Science & AI Course:
If you're leaning towards data science and want to delve into machine learning and AI, Xaltius Academy's Data Science & AI course is an excellent choice. This program equips you with the necessary skills and knowledge to become a proficient data scientist, covering essential topics like:
Python programming
Machine learning algorithms
Data visualization
And much more!
By understanding the distinct roles of business analytics and data science, you can make an informed decision about your career path and leverage the power of data to drive success.
2 notes
·
View notes
Text
Business Analytics Vs. Data Science: Understanding the Key Differences
As data takes center stage, business analytics and data science are growing in popularity. Both these disciplines use data to obtain information and reach decisions. As a result, businesses are becoming more data-driven. However, there are several significant contrasts between business analytics and data science. Keeping this in mind, this blog has been dedicated to discussing business analytics…
#business analytics vs data science#data science#data science vs business analytics#difference between business analytics and data science#difference between data science and business analytics#education#pgdm in business analytics
0 notes
Text
Predictive vs Prescriptive vs Descriptive Analytics Explained
Business analytics leveraging data patterns for strategic moves comes in three key approaches – descriptive identifying ��what has occurred", predictive forecasting “what could occur” and prescriptive recommending “what should occur” to optimize decisions. We decode the science behind each for aspiring analytics professionals.
Descriptive analytics convert volumes of historical data into insightful summaries around metrics revealing business health, customer trends, operational efficiencies etc. using direct analysis, aggregation and mining techniques producing current reports.
Predictive analytics forecast unknown future probabilities applying statistical, econometric and machine learning models over existing data to minimize uncertainties and capture emerging behaviors early for mitigation actions. Risk models simulate scenarios balancing upside/downside tradeoffs.
Prescriptive analytics take guidance one step further by dynamically recommending best decision options factoring in key performance indicators for business objective improvements after predicting multiple futures using bell curve simulations. Optimization algorithms deliver preferred actions.
While foundational data comprehension and wrangling abilities fuel all models – pursuing analytics specializations focused on statistical, computational or operational excellence boosts career-readiness filling different priorities global employers seek!
Posted By:
Aditi Borade, 4th year Barch,
Ls Raheja School of architecture
Disclaimer: The perspectives shared in this blog are not intended to be prescriptive. They should act merely as viewpoints to aid overseas aspirants with helpful guidance. Readers are encouraged to conduct their own research before availing the services of a consultant.
#analytics#types#predictive#prescriptive#descriptive#PrescriptiveAnalytics#StrategicMoves#AnalyticsProfessionals#DataScience#HistoricalData#Metrics#BusinessHealth#CustomerTrends#OperationalEfficiencies#StatisticalModels#EconometricModels#MachineLearningModels#EnvoyOverseas#EthicalCounselling#EnvoyInternationalStudents#EnvoyCounselling
4 notes
·
View notes
Text
Data Cleaning in Data Science
Data cleaning is an integral part of data preprocessing viz., removing or correcting inaccurate information within a data set. This could mean missing data, spelling mistakes, and duplicates to name a few issues. Inaccurate information can lead to issues during analysis phase if not properly addressed at the earlier stages.
Data Cleaning vs Data Wrangling : Data cleaning focuses on fixing inaccuracies within your data set. Data wrangling, on the other hand, is concerned with converting the data’s format into one that can be accepted and processed by a machine learning model.
Data Cleaning steps to follow :
Remove irrelevant data
Resolve any duplicates issues
Correct structural errors if any
Deal with missing fields in the dataset
Zone in on any data outliers and remove them
Validate your data
At EduJournal, we understand the importance of gaining practical skills and industry-relevant knowledge to succeed in the field of data analytics / data science. Our certified program in data science and data analytics is designed to equip freshers / experienced with the necessary expertise and hands-on experience experience so they are well equiped for the job.
URL : http://www.edujournal.com
#data_science#training#upskilling#irrevelant_data#duplicate_issue#datasets#validation#outliers#data_cleaning#trends#insights#machine_learning
2 notes
·
View notes
Text
Business Intelligence vs Business Analytics
Summary: While both BI and BA leverage data, BI focuses on understanding current performance through reports and dashboards. BA dives deeper, using statistical models to predict future trends and optimize decision-making for growth.

Introduction
In today's data-driven world, businesses are constantly bombarded with information. But how do you turn this data into actionable insights that drive growth and success? This is where Business Intelligence (BI) and Business Analytics (BA) come in.
While these terms are often used interchangeably, there are key differences between them. Understanding these distinctions is crucial for businesses looking to leverage their data effectively.
Defining Business Intelligence (BI)
Business Intelligence (BI) can be thought of as the foundation for data-driven decision making. It's a set of methodologies, architectures, and tools that enable businesses to gather, store, access, and analyze data to gain a comprehensive understanding of their operations.
BI focuses on presenting historical and present data in an easily digestible format, allowing users to answer questions like:
What are our top-selling products?
What are our current sales trends?
How are our marketing campaigns performing?
Key Components of BI
Data Warehousing: A central repository that stores historical data from various sources within an organization.
Data Extraction, Transformation, and Loading (ETL): The process of extracting data from various sources, transforming it into a consistent format, and loading it into the data warehouse.
Data Analysis and Reporting Tools: Software applications that allow users to query the data,
create reports, and visualize trends.
Dashboards and Scorecards: Real-time or near-real-time visual representations of key metrics and performance indicators.
Primary Functions of BI
Data Visualization: BI tools translate complex data sets into easy-to-understand charts, graphs, and dashboards. This allows users to identify trends, patterns, and outliers quickly.
Reporting and Analysis: BI enables users to generate reports that answer specific business questions. These reports can be static or interactive, allowing for deeper analysis.
Performance Monitoring: BI helps businesses track key performance indicators (KPIs) to measure progress towards goals and identify areas for improvement.
Improved Decision Making: By providing clear and concise insights into business operations, BI empowers users to make informed decisions based on data, not gut feeling.
Defining Business Analytics (BA)
Business Analytics (BA) builds upon the foundation of BI. It's a more advanced practice that uses statistical models and techniques to uncover hidden patterns, trends, and relationships within data. BA goes beyond simply describing what's happening to answer the "why" and "what if" questions. It helps businesses:
Predict future outcomes
Identify potential risks and opportunities
Develop data-driven strategies for growth
Optimize processes and resource allocation
Key Components of BA
By combining the following key components, Business Analytics empowers you to go beyond simply understanding what's happening to uncover the "why" and "what if" questions hidden within your data. Here is the breakdown of the same:
Statistical Modeling
This component focuses on applying statistical techniques to data to identify patterns, relationships, and trends. Statistical models can be used for various purposes in BA, such as:
Regression Analysis: This technique helps understand the relationship between one dependent variable (what you're trying to predict) and one or more independent variables (factors that influence the dependent variable). It allows you to estimate how much a change in the independent variable will affect the dependent variable.
Time Series Analysis: This technique focuses on analyzing data collected over time to forecast future trends and seasonality. For example, a company might use time series analysis to predict future sales based on historical sales data.
Hypothesis Testing: This technique helps determine if a particular hypothesis about a population is likely to be true based on sample data. For instance, a marketing team might use hypothesis testing to determine if a new ad campaign has a statistically significant impact on sales.
Data Mining
Data mining involves extracting hidden patterns and knowledge from large datasets that might not be readily apparent through traditional analysis techniques. Here are some common data mining techniques used in BA:
Classification: This technique categorizes data points into predefined groups based on their characteristics. An e-commerce company might use classification to segment customers based on their purchase history.
Clustering: This technique groups data points together based on their similarities, helping uncover hidden patterns and relationships within the data. For example, a bank might use clustering to identify groups of customers with similar financial risk profiles.
Association Rule Learning: This technique discovers relationships between different variables within a dataset. A grocery store might use association rule learning to identify products that are frequently bought together, allowing them to optimize product placement.
Machine Learning (ML)
Machine Learning algorithms learn from data without being explicitly programmed. These algorithms can then be used to make predictions about future events or customer behaviour. Commonly used ML techniques in BA include:
Decision Trees: These algorithms create tree-like structures that classify data based on a series of sequential questions.
Support Vector Machines (SVMs): These algorithms create hyperplanes that separate data points into distinct categories.
Neural Networks: Inspired by the human brain, these complex algorithms learn from data and can identify complex patterns and relationships.
Data Visualization
While data visualization is a key component of BI as well, BA often utilizes more advanced visualization techniques to present complex data insights effectively. These techniques include:
Interactive Dashboards: These dynamic dashboards allow users to drill down into data and explore different dimensions, providing a deeper understanding of the information.
Predictive Modeling Visualization: Techniques like heatmaps and treemaps help visualize the relationships between variables and the impact of different factors on the predicted outcome.
Primary Functions of BA
By leveraging statistical modelling and machine learning techniques, BA can transform historical data into a crystal ball, providing businesses with invaluable insights into what lies ahead. Let's delve deeper into the world of predictive analytics and explore how it empowers businesses to make data-driven decisions for a more successful future.
Predictive Analytics
BA uses historical data to forecast future trends, allowing businesses to anticipate changes in the market or customer behavior. For instance, a retail company might use BA to predict demand for specific products during the holiday season.
Prescriptive Analytics
It goes beyond simple prediction to suggest optimal courses of action based on data analysis. Imagine a company analyzing customer churn data to identify factors that contribute to customer dissatisfaction. BA can then recommend strategies to address these factors and retain customers.
Risk Assessment
BA helps businesses identify and mitigate potential risks by analyzing historical data and industry trends. An insurance company might use BA to assess the risk of policyholders filing claims.
Customer Segmentation
Business Analytics helps businesses segment their customer base into distinct groups with similar characteristics and behaviors. This allows for targeted marketing campaigns and improved customer satisfaction. For example, a clothing retailer might segment its customers by age, gender, and purchase history to create targeted email campaigns.
Key Differences Between BI and BA
Choosing Between BI and BA
In many cases, BI and BA work best when used together. BI provides the foundation for understanding your current state, while BA helps you leverage that understanding to make better decisions about the future. The choice between BI and BA depends on your specific needs. Here's a breakdown to help you decide:
Need BI if:
You need a clear picture of your current performance.
You want to track key metrics and identify trends.
You want to empower business users with self-service analytics.
You have a large volume of data that needs to be organized and easily accessible.
Need BA if:
You want to predict future outcomes and make data-driven forecasts.
You want to identify root causes of problems and optimize processes.
You want to develop data-driven strategies for growth and innovation.
You have a team of data analysts or data scientists who can leverage advanced statistical techniques.
Here's an analogy: Imagine BI as the rearview mirror of your car, giving you a clear view of where you've been. BA is like the windshield, helping you see where you're going and navigate the road ahead.
Conclusion
In today's data-driven world, both BI and BA are essential tools for businesses of all sizes. By understanding the key differences between these two disciplines, you can make informed decisions about how to leverage your data to gain a competitive advantage.
BI empowers you to understand your current situation, while BA equips you to predict future trends and make data-driven decisions for growth and success. Remember, BI is the foundation, and BA is the strategy builder. Together, they form a powerful combination for unlocking the true potential of your data.
Frequently Asked Questions
What is the Difference Between BI and BA?
BI provides a rearview mirror view of your business, analyzing past and present data. BA acts like a windshield, using data to predict future trends and make data-driven decisions.
When Should I Use BI?
Use BI if you need clear insights into current performance, want to track key metrics, or empower business users to analyze data.
When Should I Use BA?
Use BA if you want to predict future trends, identify root causes of problems, or develop data-driven strategies for growth and innovation.
#business intelligence vs business analytics#business analytics#data analytics#business intelligence#business#power bi#bi#ba#bi vs ba#big data analytics#data science#pickl.ai#data analyst
1 note
·
View note
Text
Data Science vs Data Analytics: What’s the Difference and Which One is Right for You?
In today’s data-driven world, “Data Science” and “Data Analytics” are two buzzwords that often get thrown around — sometimes even interchangeably. But while they’re related, they’re definitely not the same thing. So, what sets them apart? And more importantly, which one should you pursue?
Let’s break it down.

What is Data Analytics?
Data Analytics is all about examining existing data to find actionable insights. Think of it as zooming in on the present and past to understand what’s happening or what has already happened.
🔍 Key Tasks:
Cleaning and organizing data
Identifying trends and patterns
Creating dashboards and reports
Supporting business decisions
🛠 Common Tools:
Excel
SQL
Power BI / Tableau
Python (Pandas) or R
Who hires data analysts?
Almost every industry — from healthcare and finance to marketing and sports — needs people who can interpret data and make it useful.
What is Data Science?
Data Science takes things a step further. It’s a broader, more advanced field that involves creating models, algorithms, and even AI systems to predict future outcomes and uncover deeper insights.
Key Tasks:
Data wrangling and analysis
Building predictive models
Machine learning & AI
Big data processing
Common Tools:
Python / R
SQL / NoSQL databases
Jupyter Notebook
TensorFlow / Scikit-learn / PyTorch
Hadoop / Spark
Who hires data scientists?
Tech companies, research labs, financial institutions, and even startups rely on data scientists to drive innovation and strategic decisions.
🆚 So, What’s the Difference?
FeatureData AnalyticsData ScienceFocusDescriptive & diagnostic insightsPredictive & prescriptive modelingGoalUnderstand what happenedPredict what will happen and whySkillsStatistics, Excel, SQL, VisualizationProgramming, Machine Learning, AIToolsExcel, Tableau, Power BIPython, R, TensorFlow, Spark, HadoopComplexityModerateHigh
Which One is Right for You?
It depends on your interests, goals, and background:
Choose Data Analytics if you enjoy finding patterns, love organizing data, and want a business-focused role with quicker entry points.
Choose Data Science if you're into programming, math, and building systems that can predict the future.
Both paths are exciting, high-paying, and in demand. If you're just getting started, data analytics can be a great way to enter the field and then grow into data science later.
Final Thoughts
There’s no right or wrong choice — only what’s right for you. Whether you become a data analyst who shapes daily decisions or a data scientist driving long-term strategies with cutting-edge tech, you’ll be a part of the most powerful force in modern business: data.
0 notes