#data engineering tool
Explore tagged Tumblr posts
Text
Unlocking the Future of Data Engineering with Ask On Data: An Open Source, GenAI-Powered NLP-Based Data Engineering Tool
In the rapidly evolving world of data engineering, businesses and organizations face increasing challenges in managing and integrating vast amounts of data from diverse sources. Traditional data engineering processes, including ETL (Extract, Transform, Load) and ELT (Extract, Load, Transform), often require specialized expertise, are time-consuming, and can be error-prone. To address these challenges, innovative technologies like Generative AI (GenAI), Large Language Models (LLM), and Natural Language Processing (NLP) are reshaping how data engineers and analysts work with data. One such breakthrough solution is Ask On Data, an NLP based data engineering tool that simplifies the complexities of data integration, transformation, and loading through conversational interfaces.
What is Ask On Data?
Ask On Data is an open-source NLP based ETL tool that leverages Generative AI and Large Language Models (LLMs) to enable users to interact with complex data systems in natural language. With Ask On Data, the traditionally technical processes of data extraction, data transformation, and data loading are streamlined and made accessible to a broader range of users, including those without a deep technical background in data engineering.
The tool bridges the gap between the technical demands of data engineering and the need for more intuitive, accessible interfaces. Through simple, conversational commands, users can query, transform, and integrate data, eliminating the need for writing complex code. This functionality significantly reduces the time and effort required for managing data pipelines, making it ideal for organizations looking to automate and optimize their data workflows.
How Ask On Data Works: NLP Meets Data Engineering
The core innovation of Ask On Data lies in its ability to understand and process natural language commands related to data integration, data transformation, and data loading. The tool uses Natural Language Processing (NLP) to parse and execute commands, enabling users to work with data systems without needing to master SQL or scripting languages.
Data Integration: Ask On Data connects seamlessly with various data sources, whether it’s a data lake, data warehouse, or cloud-based data storage system. Users can simply input commands like, “Integrate data from my sales database with customer feedback from the cloud,” and Ask On Data will manage the connection, data extraction, and integration processes.
Data Transformation: Data transformation is another area where Ask On Data excels. Users can request transformations in natural language, such as “Convert all dates in this dataset to ISO format” or “Aggregate sales data by region for the last quarter,” and the tool will apply the transformations without needing complex scripts.
Data Loading: Once data is transformed, Ask On Data can automatically load it into the target system, whether it’s a data warehouse for analytics or a data lake for storage. Users can issue simple commands to initiate loading, such as “Load transformed data into the reporting database.”
Benefits of Ask On Data in Data Engineering
Simplified Data Operations: By using natural language to manage ETL/ELT processes, Ask On Data lowers the barrier for non-technical users to access and manipulate data. This democratization of data engineering allows business analysts, data scientists, and even executives to interact with data more easily.
Increased Efficiency: Automation of routine data tasks, like data extraction, transformation, and loading, speeds up the process and reduces human errors. With GenAI at its core, Ask On Data can also generate code or queries based on user instructions, making it a powerful assistant for data engineers.
Open-Source Flexibility: Ask On Data is an open-source tool, meaning it is freely available and highly customizable. Organizations can adapt the tool to fit their specific needs, from integrating it into custom workflows to extending its capabilities through plugins or custom scripts.
Improved Collaboration: With its intuitive, chat-based interface, Ask On Data fosters better collaboration across teams. Data engineers can focus on more complex tasks while empowering other stakeholders to interact with data without fear of making mistakes or having to understand complex technologies.
The Future of Data Engineering with NLP and GenAI
The integration of Generative AI and LLMs into data engineering tools like Ask On Data represents a paradigm shift in how data operations are managed. By combining the power of AI with the simplicity of NLP, Ask On Data enables organizations to make smarter, faster decisions and streamline their data workflows.
As businesses continue to generate more data and migrate towards cloud-based solutions, tools like Ask On Data will become increasingly important in helping them integrate, transform, and analyze data efficiently. By making ETL and ELT processes more accessible and intuitive, Ask On Data is laying the groundwork for the future of data transformation, data loading, and data integration.
Conclusion,
Ask On Data is an innovative NLP-based data engineering tool that empowers users to manage complex data workflows with ease. Whether you're working with a data lake, a data warehouse, or cloud-based platforms, Ask On Data’s conversational interface simplifies the tasks of data transformation, data integration, and data loading, making it a must-have tool for modern data engineering teams.
0 notes
Text
As someone with a passing knowledge of how AI/computer image generation works, I really wouldn't have minded my stuff being plugged into an engine before a handful of months ago.
That isn't to say that artists shouldn't have control over whether their content is used as training data (the general lack of discretion in the data sets is a whole issue of its own), but up until recently these programs were a lot more of a novelty, and also commonly acknowledged to be very bad at what they were trying to do. While stable diffusion has made the outputs scan better, they're clearly still lacking a lot. Of course, I've never been personally at risk, because I don't have a singular distinctive visual art style - I have a good handful which I apply depending on my mood or what the project demands. My writing, also, is geared toward clear and concise communication, and that simply can't be imitated if the engine doesn't understand the concept behind what it's trying to communicate.
But moreso, it seemed absurd to me that image generation in its current state could compete with actual artists, and I still don't really see that as being as much of a concern as some people think. The people who understand the value of human-made art will still want it regardless of replicability. Anyone willing to substitute it for something that gives them much less flexibility with the final output probably either wasn't compensating artists fairly before, or wasn't buying art at all. I'm not really convinced this hypothetical guy exists who previously would have paid full price for a work by a popular digital artist, but won't anymore because they can generate a facsimile of the artist's style. I think the problem is way more with the general devaluation of art and design work.
There certainly may be less commercial work, but the survival of artists shouldn't depend on their commercial viability. In our capitalist hellscape those corners are liable to be cut when any substitute is available. That could be computer image generation, or it could be a guy who can whip out a barely-passable Photoshop job in 20 minutes where an artist (or a designer who cared and was paid enough to make it more than barely-passable) would spend hours.
Anyone who's argued for modern art before - or on the other end of the spectrum, for photorealism - knows that art shouldn't be valued only by how easy the image is to replicate, and I feel like the emphasis on commercial viability really flattens the discussion on how this technology actually has the capability for harm.
My concern is not about the artists not getting paid for jobs in industries they were already being cut out of. My concern is about how our world might get measurably worse because some people have decided that "actively garbage but scans ok at a first glance" is good enough. Or they don't know enough about what they're doing to discern whether the output is good quality, which is uh, horrifying in some of these contexts. But again, those people were already cutting those corners - it's just gotten way easier to point and laugh at them.
So really, if we want to protect ourselves, I think the main thing that we can do is point and laugh. This won't guarantee that they'll change anything meaningfully, but it'll at least show them they can't get away with making these changes without anyone noticing. It'll hopefully teach people who have not figured it out that these tools are not smart and essays are not an appropriate use for them. And it'll give us some pretty guilt-free schadenfreude to tide us over.
#these engines have a lot of other issues that need to be addressed#but i have zero issue with the *concept* of these tools when used appropriately#a lot of the issues currently are that people aren't using them appropriately#and are thereby making themselves look very stupid#everyone should have vastly more control over their data than is currently given to us regardless of its use#but even if a computer or a five year old or anyone else can replicate your style your art has value#and you must sell to people who know that because those who see art as only a commodity will always treat you poorly#anyway i genuinely put a lot of work into this mini-essay please reblog it
8 notes
·
View notes
Text
i don't really get the assumption that everyone who uses chatgpt is telling it to generate prose or w/e. if i want good fiction or poetry i know where to go for that and it isn't to a bot.
i use it when i need to complain about my emotional problems which are too embarrassing to tell a real person, and also ask it questions too specific for google, like about particular chemical elements or certain planetary placements in astrology or "recommend me music with sounds like [timestamp] in [song title]" lol
#random rambles#honestly i think it has potential to be a good learning tool because it allows for a higher level of detail than a search engine#maybe this is a hot take but i think its general premise is good actually#of course its no–holds–barred method of harvesting data is Not good#but i think with enough demand they will be forced to change how it works once first–world govts start pressuring them about it#idk i think we're in the same phase that dawns on humans every time a new bit of modern tech is made available to the average person#there's a lot of panic about what it 'could' do and what it means for Us and some of the fear is absolutely warranted#because heaven knows the first several versions of any major invention are flawed in multiple ways#but with time it gets more refined and less 'threatening' and we become familiar with it and eventually it becomes just another Thing lol
3 notes
·
View notes
Text
https://www.teepublic.com/t-shirt/46350021-future-machine-learning-engineer
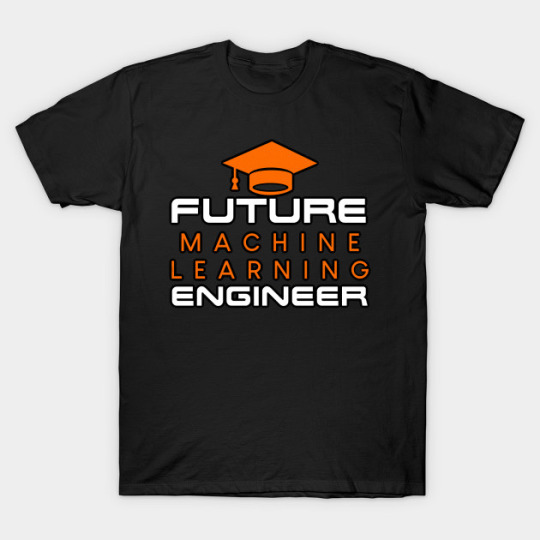

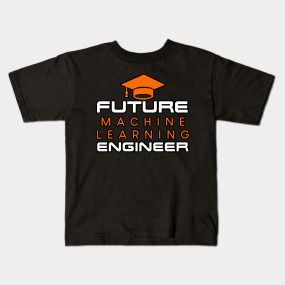
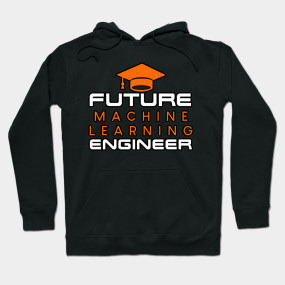
#chatbot#ai#ai tools#ai artwork#artificalintelligence#machinelearning#machine learning#data science#prompt engineering#artificial intelligence#hbculove#black history month#hbcu#black history#merch by amazon
3 notes
·
View notes
Text
Boost Your Data Testing Skills with Practical SQL Training
Want to feel more confident writing SQL queries for your data validation work? The SQL Essentials for Data Testing course by iceDQ helps QA engineers and testers get hands-on with SQL, specifically for testing purposes. You won’t waste time on concepts you won’t use — every module is crafted around how SQL is used in real testing environments. From comparing source and target systems to spotting mismatches and understanding transformations, you’ll get everything you need to validate data correctly. The course is beginner-friendly and packed with practical tips that make SQL easy to learn and apply. 👉 Start learning here
#icedq#data testing automation tools#data warehouse testing#etl testing tools#bi testing#etl testing tool#data migration testing#data reliability engineering#etl testing#production data monitoring#data migration testing tools
0 notes
Text
Explore IGMPI’s Big Data Analytics program, designed for professionals seeking expertise in data-driven decision-making. Learn advanced analytics techniques, data mining, machine learning, and business intelligence tools to excel in the fast-evolving world of big data.
#Big Data Analytics#Data Science#Machine Learning#Predictive Analytics#Business Intelligence#Data Visualization#Data Mining#AI in Analytics#Big Data Tools#Data Engineering#IGMPI#Online Analytics Course#Data Management#Hadoop#Python for Data Science
0 notes
Text
The Future of Digital Marketing: Trends to Watch in 2025
Introduction The digital marketing landscape is evolving faster than ever. With advancements in artificial intelligence, changing consumer behaviors, and new regulations shaping the industry, businesses must stay ahead of the curve. To remain competitive, marketers need to adapt to the latest trends that will define digital marketing in 2025. In this article, we will explore the key digital…
#AI in digital marketing#AI-powered content creation tools#Best marketing automation tools 2025#Best video marketing platforms#Brand awareness through influencer marketing#content-marketing#Data privacy in digital advertising#Digital Marketing#Digital marketing trends 2025#Future of digital marketing#Future of paid advertising#How AI is changing digital marketing#How to optimize for Google search in 2025#Influencer marketing strategies#Interactive ads for higher conversions#Interactive content marketing#marketing#Marketing automation and AI#Micro-influencers vs macro-influencers#Omnichannel digital marketing strategy#Privacy-first marketing#Search engine optimization strategies#SEO#SEO trends 2025#Short-form video marketing#Social media engagement tactics#Social media marketing trends#social-media#The impact of AI on consumer behavior#Video marketing trends
0 notes
Text

Our data engineering solutions are designed to grow with your business, ensuring your systems can efficiently handle increasing data volumes, and support expansion without compromising performance or reliability. We integrate data from multiple sources, providing a unified view that makes it easier to manage, analyze, and leverage, improving decision-making, strategic planning, and overall business outcomes.
#data engineering services#data analytics services#data analysis tools#data analysis software#data engineering#data analysis
0 notes
Text
Ask On Data: A Comprehensive Guide to Using AI Chat for Data Engineering Tasks
In today’s fast-paced world of data engineering, businesses are continuously seeking ways to streamline their data processes. One revolutionary tool making waves is Ask On Data, the world’s first Open Source GenAI powered chat based Data Engineering tool. Whether you're a novice or a seasoned data professional, Ask On Data simplifies complex tasks and makes data transformations as easy as typing a message.
What is Ask On Data?
Ask On Data is a cutting-edge tool designed to take the hassle out of data engineering. Built using advanced AI and Large Language Models (LLM), it allows users to manage data transformations with ease. It offers two versions: a free open-source version that can be downloaded and deployed on your own servers, and an enterprise version, which functions as a fully managed service. The open-source version makes the tool highly accessible for smaller businesses or those wishing to have control over their data processes, while the enterprise version provides additional features, support, and scalability for larger organizations.
Key Advantages of Using Ask On Data
No Learning Curve: Traditional data engineering tools often require technical expertise, coding knowledge, and significant training. However, with Ask On Data, there is no learning curve. Its AI-powered chat interface simplifies complex data tasks by allowing users to simply type commands in natural language. Whether it’s cleaning, wrangling, or transforming data, Ask On Data understands your commands and executes them accurately and efficiently.
Empowers Non-Technical Users: A major advantage of Ask On Data is its ability to eliminate the need for technical resources. Users no longer need to rely on developers or data engineers to complete everyday data tasks. Whether you're a business analyst or someone without a technical background, you can directly interact with the tool to perform tasks such as data transformations or loading. This significantly reduces bottlenecks and increases productivity across teams.
Quick and Easy Implementation: One of the standout features of Ask On Data is its speed of implementation. Unlike traditional data engineering tools that can take weeks or even months to set up, Ask On Data allows users to perform complex data operations in real-time. Since the tool operates via simple chat commands, the process of integrating it into existing workflows is as fast as typing.
No Technical Knowledge Required: Another compelling advantage of Ask On Data is that it requires no technical knowledge to use. The platform is designed with a user-friendly interface that makes data engineering tasks accessible to anyone, regardless of their technical background. You don’t need to worry about mastering coding languages, understanding databases, or learning complex ETL processes. Instead, you can type your requests in plain language, and Ask On Data’s AI will take care of the rest.
How Ask On Data Works
Ask On Data works by processing natural language input and transforming it into executable actions. When you type a request such as “clean the sales data,” the AI-powered backend interprets the command, analyzes your data, and performs the necessary operations. This could include removing duplicates, handling missing values, or applying specific business rules for data transformation.
Moreover, the tool’s ability to perform data wrangling (like joining datasets or aggregating values) and data loading (to different destinations) makes it an all-in-one solution for various data engineering needs.
Use Cases
Data Transformation: Ask On Data can handle a range of transformation tasks, from simple data cleaning to more complex operations like pivoting, normalizing, and aggregating data.
Data Integration: It supports integration with various data sources and destinations, helping businesses move and merge data across platforms seamlessly.
Data Monitoring and Validation: The platform can be used to set up monitoring and validation rules to ensure that the data is in the expected format and meets quality standards.
Conclusion
Ask On Data is a game-changer in the world of data engineering. Its chat-based interface, combined with AI-powered capabilities, makes it an invaluable tool for anyone who needs to work with data—whether you're a non-technical user or a seasoned professional. With the freedom to use the open-source version or opt for the enterprise-managed service, Ask On Data provides flexibility, ease of use, and rapid deployment. Say goodbye to complex, time-consuming data workflows, and say hello to a new, simpler way to manage your data transformations with Ask On Data.
0 notes
Text
Introduction to Prompt Engineering Applications
Discover how prompt engineering transforms AI applications—from content creation to coding and education. Learn its real-world uses, challenges, and future trends. Perfect for AI enthusiasts and professionals. Dive in now! #AI #PromptEngineering #ArtificialIntelligence #AITools #Innovation
Prompt engineering is the art and science of crafting inputs to guide AI models toward desired outputs. It’s like giving clear instructions to a highly skilled assistant. But instead of a person, you’re working with advanced AI systems like ChatGPT, GPT-4, or Bard. The applications of prompt engineering are vast and transformative. From creating content to solving complex problems, it’s changing��
0 notes
Text
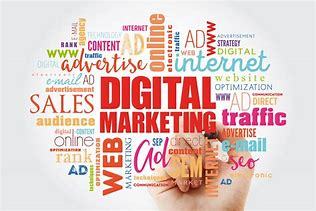
Digital Marketing Services
#Digital marketing services in Hyderabad cater to businesses of all sizes#helping them establish a strong online presence. These services include SEO (Search Engine Optimization)#PPC (Pay-Per-Click) advertising#social media marketing#content marketing#email marketing#and web development. Leading agencies in Hyderabad leverage data-driven strategies#creative campaigns#and the latest tools to boost brand visibility#generate leads#and drive business growth in both local and global markets.
1 note
·
View note
Text
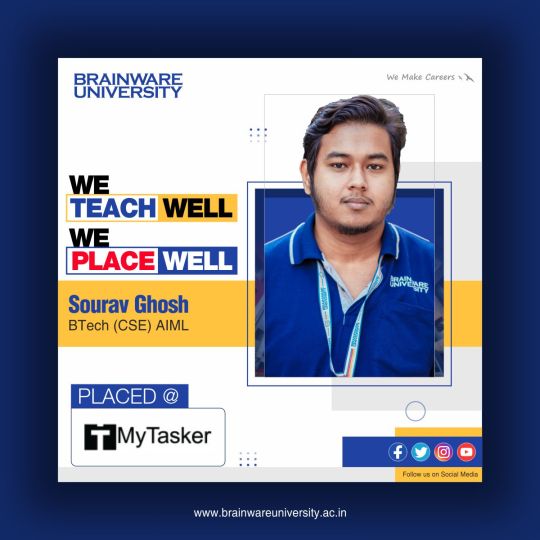
BTech CSE: Your Gateway to High-Demand Tech Careers
Apply now for admission and avail the Early Bird Offer
In the digital age, a BTech in Computer Science & Engineering (CSE) is one of the most sought-after degrees, offering unmatched career opportunities across industries. From software development to artificial intelligence, the possibilities are endless for CSE graduates.
Top Job Opportunities for BTech CSE Graduates
Software Developer: Design and develop innovative applications and systems.
Data Scientist: Analyze big data to drive business decisions.
Cybersecurity Analyst: Safeguard organizations from digital threats.
AI/ML Engineer: Lead the way in artificial intelligence and machine learning.
Cloud Architect: Build and maintain cloud-based infrastructure for global organizations.
Why Choose Brainware University for BTech CSE?
Brainware University provides a cutting-edge curriculum, hands-on training, and access to industry-leading tools. Our dedicated placement cell ensures you’re job-ready, connecting you with top recruiters in tech.
👉 Early Bird Offer: Don’t wait! Enroll now and take the first step toward a high-paying, future-ready career in CSE.
Your journey to becoming a tech leader starts here!
#n the digital age#a BTech in Computer Science & Engineering (CSE) is one of the most sought-after degrees#offering unmatched career opportunities across industries. From software development to artificial intelligence#the possibilities are endless for CSE graduates.#Top Job Opportunities for BTech CSE Graduates#Software Developer: Design and develop innovative applications and systems.#Data Scientist: Analyze big data to drive business decisions.#Cybersecurity Analyst: Safeguard organizations from digital threats.#AI/ML Engineer: Lead the way in artificial intelligence and machine learning.#Cloud Architect: Build and maintain cloud-based infrastructure for global organizations.#Why Choose Brainware University for BTech CSE?#Brainware University provides a cutting-edge curriculum#hands-on training#and access to industry-leading tools. Our dedicated placement cell ensures you’re job-ready#connecting you with top recruiters in tech.#👉 Early Bird Offer: Don’t wait! Enroll now and take the first step toward a high-paying#future-ready career in CSE.#Your journey to becoming a tech leader starts here!#BTechCSE#BrainwareUniversity#TechCareers#SoftwareEngineering#AIJobs#EarlyBirdOffer#DataScience#FutureOfTech#Placements
1 note
·
View note
Text
#Digital marketing is the practice of leveraging online channels such as search engines#social media#email#websites#and mobile apps to promote products#services#or brands. It combines data-driven strategies and creative content to engage target audiences#build brand awareness#and drive conversions. With its ability to reach global audiences and provide measurable results#digital marketing is an essential tool for businesses to thrive in the digital age.
1 note
·
View note
Text
Master Data Modeling Basics with IcedQ University
Dive into core database concepts with IcedQ University’s Data Models and Database Fundamentals course. Covering ER diagrams, dimensional modeling, and critical table types like master and transaction tables, this course is perfect for building a strong foundation in data systems and database design. You’ll also understand how to implement scalable architecture that supports business intelligence, analytics, and data warehousing needs—an essential skill for anyone working in modern data environments.
👉 Start your learning journey today: Enroll Here
#icedq#datamodeling#SQLTraining#DataArchitecture#LearnDataModeling#DatabaseFundamentals#DataAnalyticsBasics#StructuredData#OnlineDataCourse#production data monitoring#data migration testing tools#data reliability engineering
0 notes
Text
The Importance of Data Engineering in Today’s Data-Driven World

In today’s fast-paced, technology-driven world, data has emerged as a critical asset for businesses across all sectors. It serves as the foundation for strategic decisions, drives innovation, and shapes competitive advantage. However, extracting meaningful insights from data requires more than just access to information; it necessitates well-designed systems and processes for efficient data management and analysis. This is where data engineering steps in. A vital aspect of data science and analytics, data engineering is responsible for building, optimizing, and maintaining the systems that collect, store, and process data, ensuring it is accessible and actionable for organizations.
Let's explore how Data Engineering is important in today's world:
1. What is Data Engineering
2. Why is Data Engineering Important
3. Key Components of Data Engineering
4. Trends in Data Engineering
5. The Future of Data Engineering
Let’s examine each one in detail below.
What is Data Engineering?
Data engineering involves creating systems that help collect, store, and process data effectively.It involves creating data pipelines that transport data from its source to storage and analysis systems, implementing ETL processes (Extract, Transform, Load), and maintaining data management systems to ensure data is accessible and secure. It enables organizations to make better use of their data resources for data-driven decision-making.
Why is Data Engineering Important?
Supports Data-Driven Decision-Making: In a competitive world, decisions need to be based on facts and insights. Data engineering ensures that clean, reliable, and up-to-date data is available to decision-makers. From forecasting market trends to optimizing operations, data engineering helps businesses stay ahead.
Manages Big Data Effectively: Big data engineering focuses on handling large and complex datasets, making it possible to process and analyze them efficiently. Industries like finance, healthcare, and e-commerce rely heavily on big data solutions to deliver better results.
Enables Modern Technologies: Technologies like machine learning, artificial intelligence, and predictive analytics depend on well-prepared data. Without a solid modern data infrastructure, these advanced technologies cannot function effectively. Data engineering ensures these systems have the data they need to perform accurately.
Key Components of Data Engineering:
Data Pipelines: Data pipelines move data automatically between systems.They take data from one source, change it into a useful format, and then store it or prepare it for analysis.
ETL Processes: ETL (Extract, Transform, Load) processes are crucial in preparing raw data for analysis. They clean, organize, and format data, ensuring it is ready for use.
Data Management Systems:
These systems keep data organized and make it easy to access. Examples of these systems are databases, data warehouses, and data lakes.
Data Engineering Tools: From tools like Apache Kafka for real-time data streaming to cloud platforms like AWS and Azure, data engineering tools are essential for managing large-scale data workflows.
Trends in Data Engineering:
The field of data engineering is changing quickly, and many trends are shaping its future:
Cloud-Based Infrastructure: More businesses are moving to the cloud for scalable and flexible data storage.
Real-Time Data Processing: The need for instant insights is driving the adoption of real-time data systems.
Automation in ETL: Automating repetitive ETL tasks is becoming a standard practice to improve efficiency.
Focus on Data Security: With increasing concerns about data privacy, data engineering emphasizes building secure systems.
Sustainability: Energy-efficient systems are gaining popularity as companies look for greener solutions.
The Future of Data Engineering:
The future of data engineering looks bright. As data grows in size and complexity, more skilled data engineers will be needed.Innovations in artificial intelligence and machine learning will further integrate with data engineering, making it a critical part of technological progress. Additionally, advancements in data engineering tools and methods will continue to simplify and enhance workflows.
Conclusion:
Data engineering is the backbone of contemporary data management and analytics. It provides the essential infrastructure and frameworks that allow organizations to efficiently process and manage large volumes of data. By focusing on data quality, scalability, and system performance, data engineers ensure that businesses can unlock the full potential of their data, empowering them to make informed decisions and drive innovation in an increasingly data-driven world.
Tudip Technologies has been a pioneering force in the tech industry for over a decade, specializing in AI-driven solutions. Our innovative solutions leverage GenAI capabilities to enhance real-time decision-making, identify opportunities, and minimize costs through seamless processes and maintenance.
If you're interested in learning more about the Data Engineering related courses offered by Tudip Learning please visit: https://tudiplearning.com/course/essentials-of-data-engineering/.
#Data engineering trends#Importance of data engineering#Data-driven decision-making#Big data engineering#Modern data infrastructure#Data pipelines#ETL processes#Data engineering tools#Future of data engineering#Data Engineering
1 note
·
View note
Text
LangChain: Components, Benefits & Getting Started

Understanding the Core Components of LangChain
LangChain is a revolutionary framework designed to enhance the capabilities of Large Language Models (LLMs) by enabling them to process and comprehend real-time data more efficiently. At its core, LangChain is built on foundational components that support its robust architecture. These components include: - Data Connectors: These facilitate seamless integration with various data sources, allowing LLMs to access diverse datasets in real-time. - Processing Pipelines: LangChain employs sophisticated pipelines that preprocess and transform raw data into structured formats suitable for consumption by LLMs. - Semantic Parsers: These components help interpret and extract meaningful information from text inputs, providing LLMs with context-rich data. - Inference Engines: At the heart of LangChain, inference engines leverage advanced algorithms to derive insights from the processed data, enhancing the decision-making capabilities of LLMs. Together, these components form an integrated ecosystem that empowers developers to build dynamic, AI-driven applications.
How LangChain Enhances LLM Capabilities with Real-Time Data
One of the standout features of this framework is its ability to augment LLM capabilities through real-time data integration. Traditional language models often operate in static environments, relying on pre-trained data sets. However, LangChain breaks this limitation by establishing live connections with dynamic data sources. Using its advanced data connectors, it can pull data from APIs, databases, and streams, ensuring that LLMs are informed by the most current information available. This real-time data ingestion not only increases the relevancy of LLM outputs but also enables adaptive learning. The synchronous feeding of real-time data into LLMs allows applications powered by LangChain to react swiftly to changes, whether they pertain to market trends, news events, or user interactions. By leveraging real-time data, LangChain truly sets itself apart as a tool for modern AI applications, providing both accuracy and agility in decision-making processes.
Streamlining Data Organization for Efficient LLM Access
Efficiency in accessing and processing data is crucial for optimizing the performance of LLMs. LangChain introduces several methodologies to streamline data organization, thereby facilitating quick and efficient data retrieval. Firstly, the framework implements a hierarchical data storage system that categorizes data based on its relevance and frequency of access. This enables the prioritization of data that is most pertinent to ongoing tasks, reducing latency in information retrieval. Secondly, LangChain employs advanced indexing techniques. By creating indices tailored to specific data attributes, LangChain accelerates the search process, enabling LLMs to access necessary data rapidly. Furthermore, the use of semantic tagging enhances this process, allowing for intelligent filtering based on contextually relevant keywords. Lastly, a commitment to data normalization within LangChain ensures that data from disparate sources is harmonized into a uniform format. This standardization minimizes the complexity during data processing stages and allows LLMs to interpret data consistently, leading to more accurate results.
Step-by-Step Guide to Developing LLM-Powered Applications with LangChain
Developing applications powered by LangChain involves a systematic approach that maximizes the potential of LLMs. Here is a step-by-step guide to help developers get started: - Define Application Objectives: Clearly outline the goals of your application, particularly how it will utilize LLMs to achieve these objectives. - Select Appropriate Data Sources: Choose data sources that align with your application’s objectives. LangChain’s data connectors support a wide range of sources, including APIs and databases. - Configure Data Connectors: Set up the data connectors in LangChain to establish live feeds from your chosen data sources, ensuring real-time data availability. - Design the Processing Pipeline: Construct a data processing pipeline within LangChain to handle data transformations and preprocessing requirements specific to your application. - Implement Semantic Parsing: Integrate semantic parsers to enrich your data with contextual meaning and facilitate comprehensive interpretation by the LLMs. - Develop Inference Mechanisms: Build inference mechanisms using LangChain’s inference engines to derive actionable insights from the processed data. - Prototype and Test: Develop a prototype of your application and conduct thorough testing to validate functionality and ensure reliability. - Iterate and Optimize: Continuously iterate on your design, incorporating feedback and optimizing components for improved performance. This structured approach not only streamlines the development process but also ensures that the resulting application harnesses the power of LangChain efficiently.
Maximizing the Potential of LangChain in Modern AI Development
In today’s rapidly evolving technological landscape, the potential of LangChain in modern AI development is immense. Its unique combination of real-time data integration, robust processing capabilities, and compatibility with large language models position it as an indispensable tool for developers. To maximize its potential, developers should focus on tailoring LangChain's capabilities to their specific use cases. By aligning LangChain’s powerful functionalities with the unique requirements of their applications, developers can create highly specialized AI solutions that deliver exceptional value. Additionally, staying abreast of updates and enhancements to LangChain will ensure that developers leverage the latest features and improvements. Engaging with the LangChain community, participating in forums, and accessing documentation can provide valuable insights and support. Finally, experimentation and innovation are key. By exploring novel approaches and pushing the boundaries of what is possible with LangChain, developers can unlock new levels of sophistication in AI-driven applications, driving forward the future of AI technology. In conclusion, LangChain stands out as a transformative framework in AI development, offering a suite of tools and components that empower developers to build intelligent, responsive applications. By understanding and implementing its capabilities strategically, one can fully harness its potential to drive innovation in the field of artificial intelligence. Read the full article
#agent-automation#chain-management#conversationalAI#data-integration#document-processing#LLM-orchestration#memory-systems#prompt-engineering#tool-integration#workflowautomation
0 notes