#Data engineering tools
Explore tagged Tumblr posts
Text
The Importance of Data Engineering in Today’s Data-Driven World

In today’s fast-paced, technology-driven world, data has emerged as a critical asset for businesses across all sectors. It serves as the foundation for strategic decisions, drives innovation, and shapes competitive advantage. However, extracting meaningful insights from data requires more than just access to information; it necessitates well-designed systems and processes for efficient data management and analysis. This is where data engineering steps in. A vital aspect of data science and analytics, data engineering is responsible for building, optimizing, and maintaining the systems that collect, store, and process data, ensuring it is accessible and actionable for organizations.
Let's explore how Data Engineering is important in today's world:
1. What is Data Engineering
2. Why is Data Engineering Important
3. Key Components of Data Engineering
4. Trends in Data Engineering
5. The Future of Data Engineering
Let’s examine each one in detail below.
What is Data Engineering?
Data engineering involves creating systems that help collect, store, and process data effectively.It involves creating data pipelines that transport data from its source to storage and analysis systems, implementing ETL processes (Extract, Transform, Load), and maintaining data management systems to ensure data is accessible and secure. It enables organizations to make better use of their data resources for data-driven decision-making.
Why is Data Engineering Important?
Supports Data-Driven Decision-Making: In a competitive world, decisions need to be based on facts and insights. Data engineering ensures that clean, reliable, and up-to-date data is available to decision-makers. From forecasting market trends to optimizing operations, data engineering helps businesses stay ahead.
Manages Big Data Effectively: Big data engineering focuses on handling large and complex datasets, making it possible to process and analyze them efficiently. Industries like finance, healthcare, and e-commerce rely heavily on big data solutions to deliver better results.
Enables Modern Technologies: Technologies like machine learning, artificial intelligence, and predictive analytics depend on well-prepared data. Without a solid modern data infrastructure, these advanced technologies cannot function effectively. Data engineering ensures these systems have the data they need to perform accurately.
Key Components of Data Engineering:
Data Pipelines: Data pipelines move data automatically between systems.They take data from one source, change it into a useful format, and then store it or prepare it for analysis.
ETL Processes: ETL (Extract, Transform, Load) processes are crucial in preparing raw data for analysis. They clean, organize, and format data, ensuring it is ready for use.
Data Management Systems:
These systems keep data organized and make it easy to access. Examples of these systems are databases, data warehouses, and data lakes.
Data Engineering Tools: From tools like Apache Kafka for real-time data streaming to cloud platforms like AWS and Azure, data engineering tools are essential for managing large-scale data workflows.
Trends in Data Engineering:
The field of data engineering is changing quickly, and many trends are shaping its future:
Cloud-Based Infrastructure: More businesses are moving to the cloud for scalable and flexible data storage.
Real-Time Data Processing: The need for instant insights is driving the adoption of real-time data systems.
Automation in ETL: Automating repetitive ETL tasks is becoming a standard practice to improve efficiency.
Focus on Data Security: With increasing concerns about data privacy, data engineering emphasizes building secure systems.
Sustainability: Energy-efficient systems are gaining popularity as companies look for greener solutions.
The Future of Data Engineering:
The future of data engineering looks bright. As data grows in size and complexity, more skilled data engineers will be needed.Innovations in artificial intelligence and machine learning will further integrate with data engineering, making it a critical part of technological progress. Additionally, advancements in data engineering tools and methods will continue to simplify and enhance workflows.
Conclusion:
Data engineering is the backbone of contemporary data management and analytics. It provides the essential infrastructure and frameworks that allow organizations to efficiently process and manage large volumes of data. By focusing on data quality, scalability, and system performance, data engineers ensure that businesses can unlock the full potential of their data, empowering them to make informed decisions and drive innovation in an increasingly data-driven world.
Tudip Technologies has been a pioneering force in the tech industry for over a decade, specializing in AI-driven solutions. Our innovative solutions leverage GenAI capabilities to enhance real-time decision-making, identify opportunities, and minimize costs through seamless processes and maintenance.
If you're interested in learning more about the Data Engineering related courses offered by Tudip Learning please visit: https://tudiplearning.com/course/essentials-of-data-engineering/.
#Data engineering trends#Importance of data engineering#Data-driven decision-making#Big data engineering#Modern data infrastructure#Data pipelines#ETL processes#Data engineering tools#Future of data engineering#Data Engineering
1 note
·
View note
Text
Data Engineering Tools for 2024 by SG Analytics Blog Post
Today, data engineering tools are the most popular and in-demand in the ever-evolving big data domain across the globe. Data engineering tools are critical to building, monitoring, and refining complex data models, enabling organizations to enhance their business outcomes by harnessing data power. The critical role of data engineering services in today's data-driven landscape outlines the key functionalities essential to business growth.
Data Engineering - Brief Overview
Data engineering is the backbone of every successful data-driven organization. It is the discipline accountable for transforming raw and messy data into a clean, structured, and readily available format. The impact of data engineering consulting on businesses includes:
Informed decision-making: By making data readily available and organized, data engineering enables organizations to make data-driven decisions. This involves optimizing marketing campaigns and streamlining product development based on customer insights.
Enhanced efficiency: Data engineering automates monotonous tasks like data collection and transformation, freeing up valuable time and resources for other activities. Streamlined workflows help increase efficiency and cost savings.
Improved innovation: Data engineering helps unlock the potential for discovering hidden patterns and trends within data. This enables businesses to innovate by recognizing new market opportunities and developing data-driven solutions.
Integrating the right data engineering tools is critical for organizations to maximize these benefits. The wrong data engineering tools can lead to bottlenecks and data quality issues, thereby hindering the organization's ability to extract value from its data.
What are Data Engineering Tools?
Data engineering tools function as the bridge between raw data and actionable insights. Today, organizations are constantly bombarded with data from customer interactions, transactions, and different social media activities. This data deluge holds immense potential to discover critical insights, optimize data operations, and make informed decisions. However, raw data stored in isolated systems has immense untapped potential.
These tools allow data engineers to transform raw data into an accessible format ready for analysis and strategic decision-making. By streamlining data ingestion, transformation, and management, data engineering tools help organizations discover critical insights.
https://www.sganalytics.com/blog/data-engineering-tools/
#data#engineering#data engineering tools#tools#blog#post#analytics#solutions#software#services#business#USA
0 notes
Text
What is Data Profiling?
Profiling is a crucial part of data preparation programs. It's the process of examining and analyzing data sets to gain more insights into their quality. Having mountains of data is the norm in modern business. But accuracy can make or break what you do with it.
Profiling helps you learn more about how accurate and accessible your data is while giving your teams more knowledge about its structure, content, interrelationships and more. This process can also unveil potential data projects, highlighting ways you can use your data assets to boost the bottom line.
Types of Data Profiling
There are three primary forms of profiling.
The first is structure discovery. This process is about formatting data to ensure everything is uniform and consistent. Statistical analysis can give you more insight into your data's validity.
The second type of profiling is content discovery. With the content discovery, the goal is to determine the quality of the data. It helps identify anything incomplete, ambiguous or otherwise null.
Finally, we have relationship discovery. As the name implies, it's about determining how data sources connect. The process highlights similarities, differences and associations.
Why Profiling is Necessary
There are many benefits to profiling data. Ultimately, the biggest reason to include it in your data preparation program is to ensure you work with credible, high-quality data. Errors and inconsistencies will only set your organization back. They can misguide your strategy and force you to make decisions that don't provide the desired results.
Another benefit is that it helps with predictive analysis and core decision-making. When you profile data, you're learning more about the assets you hold. You can use this process to make predictions about sales, revenue, etc. That information can guide you in the right direction, making critical decisions that help generate growth and success.
Organizations also use profiling to spot potential issues within their data stream. For example, the content discovery phase highlights errors and inconsistencies. Chronic problems may point to a glaring issue within your system, helping you spot quality data issues at their source.
Read a similar article about data glossary here at this page.
0 notes
Text
As someone with a passing knowledge of how AI/computer image generation works, I really wouldn't have minded my stuff being plugged into an engine before a handful of months ago.
That isn't to say that artists shouldn't have control over whether their content is used as training data (the general lack of discretion in the data sets is a whole issue of its own), but up until recently these programs were a lot more of a novelty, and also commonly acknowledged to be very bad at what they were trying to do. While stable diffusion has made the outputs scan better, they're clearly still lacking a lot. Of course, I've never been personally at risk, because I don't have a singular distinctive visual art style - I have a good handful which I apply depending on my mood or what the project demands. My writing, also, is geared toward clear and concise communication, and that simply can't be imitated if the engine doesn't understand the concept behind what it's trying to communicate.
But moreso, it seemed absurd to me that image generation in its current state could compete with actual artists, and I still don't really see that as being as much of a concern as some people think. The people who understand the value of human-made art will still want it regardless of replicability. Anyone willing to substitute it for something that gives them much less flexibility with the final output probably either wasn't compensating artists fairly before, or wasn't buying art at all. I'm not really convinced this hypothetical guy exists who previously would have paid full price for a work by a popular digital artist, but won't anymore because they can generate a facsimile of the artist's style. I think the problem is way more with the general devaluation of art and design work.
There certainly may be less commercial work, but the survival of artists shouldn't depend on their commercial viability. In our capitalist hellscape those corners are liable to be cut when any substitute is available. That could be computer image generation, or it could be a guy who can whip out a barely-passable Photoshop job in 20 minutes where an artist (or a designer who cared and was paid enough to make it more than barely-passable) would spend hours.
Anyone who's argued for modern art before - or on the other end of the spectrum, for photorealism - knows that art shouldn't be valued only by how easy the image is to replicate, and I feel like the emphasis on commercial viability really flattens the discussion on how this technology actually has the capability for harm.
My concern is not about the artists not getting paid for jobs in industries they were already being cut out of. My concern is about how our world might get measurably worse because some people have decided that "actively garbage but scans ok at a first glance" is good enough. Or they don't know enough about what they're doing to discern whether the output is good quality, which is uh, horrifying in some of these contexts. But again, those people were already cutting those corners - it's just gotten way easier to point and laugh at them.
So really, if we want to protect ourselves, I think the main thing that we can do is point and laugh. This won't guarantee that they'll change anything meaningfully, but it'll at least show them they can't get away with making these changes without anyone noticing. It'll hopefully teach people who have not figured it out that these tools are not smart and essays are not an appropriate use for them. And it'll give us some pretty guilt-free schadenfreude to tide us over.
#these engines have a lot of other issues that need to be addressed#but i have zero issue with the *concept* of these tools when used appropriately#a lot of the issues currently are that people aren't using them appropriately#and are thereby making themselves look very stupid#everyone should have vastly more control over their data than is currently given to us regardless of its use#but even if a computer or a five year old or anyone else can replicate your style your art has value#and you must sell to people who know that because those who see art as only a commodity will always treat you poorly#anyway i genuinely put a lot of work into this mini-essay please reblog it
8 notes
·
View notes
Text
i don't really get the assumption that everyone who uses chatgpt is telling it to generate prose or w/e. if i want good fiction or poetry i know where to go for that and it isn't to a bot.
i use it when i need to complain about my emotional problems which are too embarrassing to tell a real person, and also ask it questions too specific for google, like about particular chemical elements or certain planetary placements in astrology or "recommend me music with sounds like [timestamp] in [song title]" lol
#random rambles#honestly i think it has potential to be a good learning tool because it allows for a higher level of detail than a search engine#maybe this is a hot take but i think its general premise is good actually#of course its no–holds–barred method of harvesting data is Not good#but i think with enough demand they will be forced to change how it works once first–world govts start pressuring them about it#idk i think we're in the same phase that dawns on humans every time a new bit of modern tech is made available to the average person#there's a lot of panic about what it 'could' do and what it means for Us and some of the fear is absolutely warranted#because heaven knows the first several versions of any major invention are flawed in multiple ways#but with time it gets more refined and less 'threatening' and we become familiar with it and eventually it becomes just another Thing lol
3 notes
·
View notes
Text
https://www.teepublic.com/t-shirt/46350021-future-machine-learning-engineer
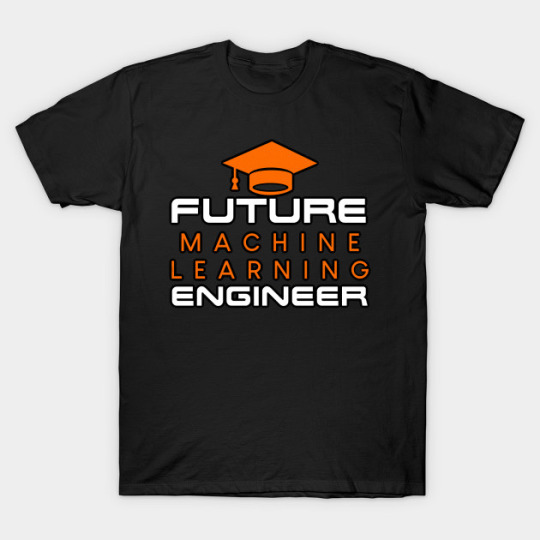

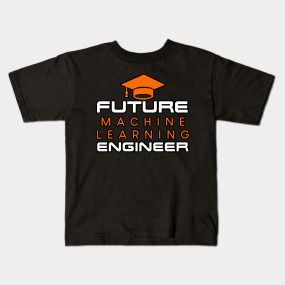
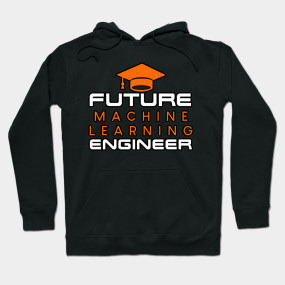
#chatbot#ai#ai tools#ai artwork#artificalintelligence#machinelearning#machine learning#data science#prompt engineering#artificial intelligence#hbculove#black history month#hbcu#black history#merch by amazon
3 notes
·
View notes
Text
How iceDQ Ensures Reliable Data Migration to Databricks?
iceDQ helped a pharmaceutical company automate the entire migration testing process from Azure Synapse to Databricks. By validating schema, row counts, and data consistency, they reduced manual work and improved accuracy. The integration with CI/CD pipelines made delivery faster and audit-ready. Avoid migration headaches—discover iceDQ’s Databricks migration testing.
#data migration testing#data warehouse testing#data migration testing tools#data reliability engineering#production data monitoring#icedq#etl testing
0 notes
Text
Explore IGMPI’s Big Data Analytics program, designed for professionals seeking expertise in data-driven decision-making. Learn advanced analytics techniques, data mining, machine learning, and business intelligence tools to excel in the fast-evolving world of big data.
#Big Data Analytics#Data Science#Machine Learning#Predictive Analytics#Business Intelligence#Data Visualization#Data Mining#AI in Analytics#Big Data Tools#Data Engineering#IGMPI#Online Analytics Course#Data Management#Hadoop#Python for Data Science
0 notes
Text
The Future of Digital Marketing: Trends to Watch in 2025
Introduction The digital marketing landscape is evolving faster than ever. With advancements in artificial intelligence, changing consumer behaviors, and new regulations shaping the industry, businesses must stay ahead of the curve. To remain competitive, marketers need to adapt to the latest trends that will define digital marketing in 2025. In this article, we will explore the key digital…
#AI in digital marketing#AI-powered content creation tools#Best marketing automation tools 2025#Best video marketing platforms#Brand awareness through influencer marketing#content-marketing#Data privacy in digital advertising#Digital Marketing#Digital marketing trends 2025#Future of digital marketing#Future of paid advertising#How AI is changing digital marketing#How to optimize for Google search in 2025#Influencer marketing strategies#Interactive ads for higher conversions#Interactive content marketing#marketing#Marketing automation and AI#Micro-influencers vs macro-influencers#Omnichannel digital marketing strategy#Privacy-first marketing#Search engine optimization strategies#SEO#SEO trends 2025#Short-form video marketing#Social media engagement tactics#Social media marketing trends#social-media#The impact of AI on consumer behavior#Video marketing trends
0 notes
Text

Our data engineering solutions are designed to grow with your business, ensuring your systems can efficiently handle increasing data volumes, and support expansion without compromising performance or reliability. We integrate data from multiple sources, providing a unified view that makes it easier to manage, analyze, and leverage, improving decision-making, strategic planning, and overall business outcomes.
#data engineering services#data analytics services#data analysis tools#data analysis software#data engineering#data analysis
0 notes
Text
Ask On Data: A Comprehensive Guide to Using AI Chat for Data Engineering Tasks
In today’s fast-paced world of data engineering, businesses are continuously seeking ways to streamline their data processes. One revolutionary tool making waves is Ask On Data, the world’s first Open Source GenAI powered chat based Data Engineering tool. Whether you're a novice or a seasoned data professional, Ask On Data simplifies complex tasks and makes data transformations as easy as typing a message.
What is Ask On Data?
Ask On Data is a cutting-edge tool designed to take the hassle out of data engineering. Built using advanced AI and Large Language Models (LLM), it allows users to manage data transformations with ease. It offers two versions: a free open-source version that can be downloaded and deployed on your own servers, and an enterprise version, which functions as a fully managed service. The open-source version makes the tool highly accessible for smaller businesses or those wishing to have control over their data processes, while the enterprise version provides additional features, support, and scalability for larger organizations.
Key Advantages of Using Ask On Data
No Learning Curve: Traditional data engineering tools often require technical expertise, coding knowledge, and significant training. However, with Ask On Data, there is no learning curve. Its AI-powered chat interface simplifies complex data tasks by allowing users to simply type commands in natural language. Whether it’s cleaning, wrangling, or transforming data, Ask On Data understands your commands and executes them accurately and efficiently.
Empowers Non-Technical Users: A major advantage of Ask On Data is its ability to eliminate the need for technical resources. Users no longer need to rely on developers or data engineers to complete everyday data tasks. Whether you're a business analyst or someone without a technical background, you can directly interact with the tool to perform tasks such as data transformations or loading. This significantly reduces bottlenecks and increases productivity across teams.
Quick and Easy Implementation: One of the standout features of Ask On Data is its speed of implementation. Unlike traditional data engineering tools that can take weeks or even months to set up, Ask On Data allows users to perform complex data operations in real-time. Since the tool operates via simple chat commands, the process of integrating it into existing workflows is as fast as typing.
No Technical Knowledge Required: Another compelling advantage of Ask On Data is that it requires no technical knowledge to use. The platform is designed with a user-friendly interface that makes data engineering tasks accessible to anyone, regardless of their technical background. You don’t need to worry about mastering coding languages, understanding databases, or learning complex ETL processes. Instead, you can type your requests in plain language, and Ask On Data’s AI will take care of the rest.
How Ask On Data Works
Ask On Data works by processing natural language input and transforming it into executable actions. When you type a request such as “clean the sales data,” the AI-powered backend interprets the command, analyzes your data, and performs the necessary operations. This could include removing duplicates, handling missing values, or applying specific business rules for data transformation.
Moreover, the tool’s ability to perform data wrangling (like joining datasets or aggregating values) and data loading (to different destinations) makes it an all-in-one solution for various data engineering needs.
Use Cases
Data Transformation: Ask On Data can handle a range of transformation tasks, from simple data cleaning to more complex operations like pivoting, normalizing, and aggregating data.
Data Integration: It supports integration with various data sources and destinations, helping businesses move and merge data across platforms seamlessly.
Data Monitoring and Validation: The platform can be used to set up monitoring and validation rules to ensure that the data is in the expected format and meets quality standards.
Conclusion
Ask On Data is a game-changer in the world of data engineering. Its chat-based interface, combined with AI-powered capabilities, makes it an invaluable tool for anyone who needs to work with data—whether you're a non-technical user or a seasoned professional. With the freedom to use the open-source version or opt for the enterprise-managed service, Ask On Data provides flexibility, ease of use, and rapid deployment. Say goodbye to complex, time-consuming data workflows, and say hello to a new, simpler way to manage your data transformations with Ask On Data.
0 notes
Text
Introduction to Prompt Engineering Applications
Discover how prompt engineering transforms AI applications—from content creation to coding and education. Learn its real-world uses, challenges, and future trends. Perfect for AI enthusiasts and professionals. Dive in now! #AI #PromptEngineering #ArtificialIntelligence #AITools #Innovation
Prompt engineering is the art and science of crafting inputs to guide AI models toward desired outputs. It’s like giving clear instructions to a highly skilled assistant. But instead of a person, you’re working with advanced AI systems like ChatGPT, GPT-4, or Bard. The applications of prompt engineering are vast and transformative. From creating content to solving complex problems, it’s changing…
0 notes
Text
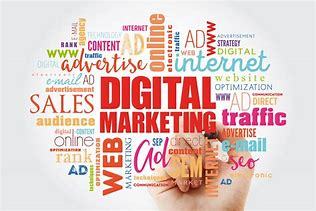
Digital Marketing Services
#Digital marketing services in Hyderabad cater to businesses of all sizes#helping them establish a strong online presence. These services include SEO (Search Engine Optimization)#PPC (Pay-Per-Click) advertising#social media marketing#content marketing#email marketing#and web development. Leading agencies in Hyderabad leverage data-driven strategies#creative campaigns#and the latest tools to boost brand visibility#generate leads#and drive business growth in both local and global markets.
1 note
·
View note
Text
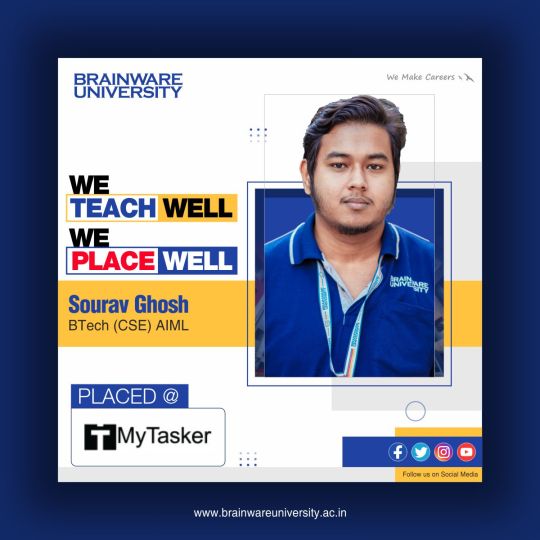
BTech CSE: Your Gateway to High-Demand Tech Careers
Apply now for admission and avail the Early Bird Offer
In the digital age, a BTech in Computer Science & Engineering (CSE) is one of the most sought-after degrees, offering unmatched career opportunities across industries. From software development to artificial intelligence, the possibilities are endless for CSE graduates.
Top Job Opportunities for BTech CSE Graduates
Software Developer: Design and develop innovative applications and systems.
Data Scientist: Analyze big data to drive business decisions.
Cybersecurity Analyst: Safeguard organizations from digital threats.
AI/ML Engineer: Lead the way in artificial intelligence and machine learning.
Cloud Architect: Build and maintain cloud-based infrastructure for global organizations.
Why Choose Brainware University for BTech CSE?
Brainware University provides a cutting-edge curriculum, hands-on training, and access to industry-leading tools. Our dedicated placement cell ensures you’re job-ready, connecting you with top recruiters in tech.
👉 Early Bird Offer: Don’t wait! Enroll now and take the first step toward a high-paying, future-ready career in CSE.
Your journey to becoming a tech leader starts here!
#n the digital age#a BTech in Computer Science & Engineering (CSE) is one of the most sought-after degrees#offering unmatched career opportunities across industries. From software development to artificial intelligence#the possibilities are endless for CSE graduates.#Top Job Opportunities for BTech CSE Graduates#Software Developer: Design and develop innovative applications and systems.#Data Scientist: Analyze big data to drive business decisions.#Cybersecurity Analyst: Safeguard organizations from digital threats.#AI/ML Engineer: Lead the way in artificial intelligence and machine learning.#Cloud Architect: Build and maintain cloud-based infrastructure for global organizations.#Why Choose Brainware University for BTech CSE?#Brainware University provides a cutting-edge curriculum#hands-on training#and access to industry-leading tools. Our dedicated placement cell ensures you’re job-ready#connecting you with top recruiters in tech.#👉 Early Bird Offer: Don’t wait! Enroll now and take the first step toward a high-paying#future-ready career in CSE.#Your journey to becoming a tech leader starts here!#BTechCSE#BrainwareUniversity#TechCareers#SoftwareEngineering#AIJobs#EarlyBirdOffer#DataScience#FutureOfTech#Placements
1 note
·
View note
Text
#Digital marketing is the practice of leveraging online channels such as search engines#social media#email#websites#and mobile apps to promote products#services#or brands. It combines data-driven strategies and creative content to engage target audiences#build brand awareness#and drive conversions. With its ability to reach global audiences and provide measurable results#digital marketing is an essential tool for businesses to thrive in the digital age.
1 note
·
View note
Text
AI strategies for cybersecurity press releases that get coverage
New Post has been published on https://thedigitalinsider.com/ai-strategies-for-cybersecurity-press-releases-that-get-coverage/
AI strategies for cybersecurity press releases that get coverage


If you’ve ever tried to get your cybersecurity news picked up by media outlets, you’ll know just how much of a challenge (and how disheartening) it can be. You pour hours into what you think is an excellent announcement about your new security tool, threat research, or vulnerability discovery, only to watch it disappear into journalists’ overflowing inboxes without a trace.
The cyber PR space is brutally competitive. Reporters at top publications receive tens, if not hundreds, of pitches each day, and they have no choice but to be highly selective about which releases they choose to cover and which to discard. Your challenge then isn’t just creating a good press release, it’s making one that grabs attention and stands out in an industry drowning in technical jargon and “revolutionary” solutions.
Why most cybersecurity press releases fall flat
Let’s first look at some of the main reasons why many cyber press releases fail:
They’re too complex from the start, losing non-technical reporters
They bury the actual news under corporate marketing speak.
They focus on product features rather than the real-world impact or problems they solve.
They lack credible data or specific research findings that journalists can cite as support.
Most of these problems have one main theme: Journalists aren’t interested in promoting your product or your business. They are looking after their interests and seeking newsworthy stories their audiences care about. Keep this in mind and make their job easier by showing them exactly why your announcement matters.
Learning how to write a cybersecurity press release
What does a well-written press release look like? Alongside the reasons listed above, many companies make the mistake of submitting poorly formatted releases that journalists will be unlikely to spend time reading.
It’s worth learning how to write a cybersecurity press release properly, including the preferred structure (headline, subheader, opening paragraph, boilerplate, etc). And, be sure to review some examples of high-quality press releases as well.
AI strategies that transform your press release process
Let’s examine how AI tools can significantly enhance your cyber PR at every stage.
1. Research Enhancement
Use AI tools to track media coverage patterns and identify emerging trends in cybersecurity news. You can analyse which types of security stories gain traction, and this can help you position your announcement in that context.
Another idea is to use LLMs (like Google’s Gemini or OpenAI’s ChatGPT) to analyse hundreds of successful cybersecurity press releases in a niche similar to yours. Ask it to identify common elements in those that generated significant coverage, and then use these same features in your cyber PR efforts.
To take this a step further, AI-powered sentiment analysis can help you understand how different audience segments receive specific cybersecurity topics. The intelligence can help you tailor your messaging to address current concerns and capitalise on positive industry momentum.
2. Writing assistance
If you struggle to convey complex ideas and terminology in more accessible language, consider asking the LLM to help simplify your messaging. This can help transform technical specifications into clear, accessible language that non-technical journalists can understand.
Since the headline is the most important part of your release, use an LLM to generate a handful of options based on your core announcement, then select the best one based on clarity and impact. Once your press release is complete, run it through an LLM to identify and replace jargon that might be second nature to your security team but may be confusing to general tech reporters.
3. Visual storytelling
If you are struggling to find ways to explain your product or service in accessible language, visuals can help. AI image generation tools, like Midjourney, create custom visuals based on prompts that help illustrate your message. The latest models can handle highly complex tasks.
With a bit of prompt engineering (and by incorporating the press release you want help with), you should be able to create accompanying images and infographics that bring your message to life.
4. Video content
Going one step further than a static image, a brief AI-generated explainer video can sit alongside your press release, providing journalists with ready-to-use content that explains complex security concepts. Some ideas include:
Short Explainer Videos: Use text-to-video tools to turn essential sections of your press release into a brief (60 seconds or less) animated or stock-footage-based video. You can usually use narration and text overlays directly on the AI platforms as well.
AI Avatar Summaries: Several tools now enable you to create a brief video featuring an AI avatar that presents the core message of the press release. A human-looking avatar reads out the content and delivers an audio and video component for your release.
Data Visualisation Videos: Use AI tools to animate key statistics or processes described in the release for enhanced clarity.
Final word
Even as you use the AI tools you have at your disposal, remember that the most effective cybersecurity press releases still require that all-important human insight and expertise. Your goal isn’t to automate the entire process. Instead, use AI to enhance your cyber PR efforts and make your releases stand out from the crowd.
AI should help emphasise, not replace, the human elements that make security stories so engaging and compelling. Be sure to shine a spotlight on the researchers who made the discovery, the real-world implications of any threat vulnerabilities you uncover, and the people security measures ultimately protect.
Combine this human-focused storytelling with the power of AI automation, and you’ll ensure that your press releases and cyber PR campaigns get the maximum mileage.
#ai#ai automation#AI image#AI image generation#AI platforms#ai tools#AI-powered#Analysis#Artificial Intelligence#attention#audio#automation#avatar#Business#challenge#chatGPT#Companies#content#cyber#cybersecurity#data#engineering#explainer videos#Features#focus#gemini#Google#how#how to#human
0 notes