#data science or data analytics which is better
Explore tagged Tumblr posts
Text
"The Role of Data Analytics in Optimizing Mobile App User Experience?"
"Discover common Google Ads mistakes, learn how to avoid them, and improve your campaign performance to maximize ROI with strategic optimization tips."
#data analysis data analytics difference#data analytics courses#data analytics tools#data analytics process#optimising app#optimizing mobile app#data science or data analytics which is better
0 notes
Text
The Four Horsemen of the Digital Apocalypse
Blockchain. Artificial Intelligence. Internet of Things. Big Data.
Do these terms sound familiar? You have probably been hearing some or all of them non stop for years. "They are the future. You don't want to be left behind, do you?"
While these topics, particularly crypto and AI, have been the subject of tech hype bubbles and inescapable on social media, there is actually something deeper and weirder going on if you scratch below the surface.
I am getting ready to apply for my PhD in financial technology, and in the academic business studies literature (Which is barely a science, but sometimes in academia you need to wade into the trash can.) any discussion of digital transformation or the process by which companies adopt IT seem to have a very specific idea about the future of technology, and it's always the same list, that list being, blockchain, AI, IoT, and Big Data. Sometimes the list changes with additions and substitutions, like the metaverse, advanced robotics, or gene editing, but there is this pervasive idea that the future of technology is fixed, and the list includes tech that goes from questionable to outright fraudulent, so where is this pervasive idea in the academic literature that has been bleeding into the wider culture coming from? What the hell is going on?
The answer is, it all comes from one guy. That guy is Klaus Schwab, the head of the World Economic Forum. Now there are a lot of conspiracies about the WEF and I don't really care about them, but the basic facts are it is a think tank that lobbies for sustainable capitalist agendas, and they famously hold a meeting every year where billionaires get together and talk about how bad they feel that they are destroying the planet and promise to do better. I am not here to pass judgement on the WEF. I don't buy into any of the conspiracies, there are plenty of real reasons to criticize them, and I am not going into that.
Basically, Schwab wrote a book titled the Fourth Industrial Revolution. In his model, the first three so-called industrial revolutions are:
1. The industrial revolution we all know about. Factories and mass production basically didn't exist before this. Using steam and water power allowed the transition from hand production to mass production, and accelerated the shift towards capitalism.
2. Electrification, allowing for light and machines for more efficient production lines. Phones for instant long distance communication. It allowed for much faster transfer of information and speed of production in factories.
3. Computing. The Space Age. Computing was introduced for industrial applications in the 50s, meaning previously problems that needed a specific machine engineered to solve them could now be solved in software by writing code, and certain problems would have been too big to solve without computing. Legend has it, Turing convinced the UK government to fund the building of the first computer by promising it could run chemical simulations to improve plastic production. Later, the introduction of home computing and the internet drastically affecting people's lives and their ability to access information.
That's fine, I will give him that. To me, they all represent changes in the means of production and the flow of information, but the Fourth Industrial revolution, Schwab argues, is how the technology of the 21st century is going to revolutionize business and capitalism, the way the first three did before. The technology in question being AI, Blockchain, IoT, and Big Data analytics. Buzzword, Buzzword, Buzzword.
The kicker though? Schwab based the Fourth Industrial revolution on a series of meetings he had, and did not construct it with any academic rigor or evidence. The meetings were with "numerous conversations I have had with business, government and civil society leaders, as well as technology pioneers and young people." (P.10 of the book) Despite apparently having two phds so presumably being capable of research, it seems like he just had a bunch of meetings where the techbros of the mid 2010s fed him a bunch of buzzwords, and got overly excited and wrote a book about it. And now, a generation of academics and researchers have uncritically taken that book as read, filled the business studies academic literature with the idea that these technologies are inevitably the future, and now that is permeating into the wider business ecosystem.
There are plenty of criticisms out there about the fourth industrial revolution as an idea, but I will just give the simplest one that I thought immediately as soon as I heard about the idea. How are any of the technologies listed in the fourth industrial revolution categorically different from computing? Are they actually changing the means of production and flow of information to a comparable degree to the previous revolutions, to such an extent as to be considered a new revolution entirely? The previous so called industrial revolutions were all huge paradigm shifts, and I do not see how a few new weird, questionable, and unreliable applications of computing count as a new paradigm shift.
What benefits will these new technologies actually bring? Who will they benefit? Do the researchers know? Does Schwab know? Does anyone know? I certainly don't, and despite reading a bunch of papers that are treating it as the inevitable future, I have not seen them offering any explanation.
There are plenty of other criticisms, and I found a nice summary from ICT Works here, it is a revolutionary view of history, an elite view of history, is based in great man theory, and most importantly, the fourth industrial revolution is a self fulfilling prophecy. One rich asshole wrote a book about some tech he got excited about, and now a generation are trying to build the world around it. The future is not fixed, we do not need to accept these technologies, and I have to believe a better technological world is possible instead of this capitalist infinite growth tech economy as big tech reckons with its midlife crisis, and how to make the internet sustainable as Apple, Google, Microsoft, Amazon, and Facebook, the most monopolistic and despotic tech companies in the world, are running out of new innovations and new markets to monopolize. The reason the big five are jumping on the fourth industrial revolution buzzwords as hard as they are is because they have run out of real, tangible innovations, and therefore run out of potential to grow.
#ai#artificial intelligence#blockchain#cryptocurrency#fourth industrial revolution#tech#technology#enshittification#anti ai#ai bullshit#world economic forum
32 notes
·
View notes
Text
weak hero undergrad uni headcanons part 2: union edition
doing this instead of actual uni work lets goooooo! and without further ado...
Jake: medicine/healthcare. i feel like he would become a doctor so he could heal people like his brother. I find it very sweet, like "i'll become a doctor so i can help you get better!" and it would be a nice conclusion to his character arc cos jake struggles with his bloodlust and capacity for violence. turning his energy towards healing would be good for him.
Dean: hotel management. this is because i watched too much hotel del luna and thought it would be funny. dean number 1 hotelier! he's protective over his friends... so being in the hospitality sector isnt too far of a stretch
Timothy: computer science/business analytics. he's really good with data and scouring the internet for info. He could put his skills to good use in the IT sector. alternate path is secret service spy agent...
Eunchan: ngl he'd skip uni and go be a streamer or something but if he HAD to pursue higher education... he'd go to a trade school i think! he seems like a hands-on typa guy.
Jimmy: business. look at him. his inferiority complex ass (affectionate). yeah I think his ambition will take him far with a business degree. jimmy bae ceo of loser inc. (sorry jimmy stans)
Jack: law, specifically commerce law. He'd be a consultant at jimmy's potential company. he gives me law vibes.
Wolf: jewellery design/metalworking. look hear me out on this one... wolfie loves his bling bling, and once he goes to therapy for anger issues, i think he would like working with jewels and gold/silver accessories. he needs a hobby.
Hwangmo: education/ early childhood education/psychology. ok HEAR ME OUT TRUST!!! hwangmo has been dealing with wolf (glorified manchild) for YEARS. and he seems like such a softie once you get past the harsh exterior (bro can befriend anyone). i think it would be really cute if he became a school teacher or therapist since he's good with people and mediating.
Dongha: cosmetology/hairdressing! dude look at those luscious locks tell me he's not gonna save people's haircuts. he'd specialise in high fashion/experimental hairdos, i see him being a stylist for fashion models.
seongmok: honestly... dude would join the military. or if not he'd study communications. which is ironic because he barely speaks.
kingley: law+commerce. he'd totally do a double degree and bust his ass to become a corrupt lawyer for donald's sake. or a secretary.
donald: law / political science / business ... he'd wanna do something that would let him change the world.
#weak hero webtoon#weak hero#headcanon#weak hero headcanons#headcanons#donald na#kingsley kwan#jake ji#eunchan hong#timothy park#dean kwon#jimmy bae#jack kang#wolf keum#hwangmo ju#dongha baek#seongmok do#ugh too many tags
52 notes
·
View notes
Note
That conversation got annoying quickly, I don’t know why people felt such a need to defend the mental health benefits of war, but it seems everyone conflated very different fields? Obviously, “I am depressed because of immutable characteristics of society” isn’t a useful observation, it’s just one of the basic symptoms of depression. But “analyzing the relationship between societal impacts and psychology is useless because it offers no help for the individual” is just as pointless, as if general relativity is useless because it doesn’t teach you to pilot a plane.
Collecting data regarding an entire population is just incomparable to an individual’s psychoanalysis. The latter may be more immediately useful, but it’s also not realistic treating it as a science until a better working model of the human brain is achieved. Until then, comparing various populations’ emotional and psychological reactions along sociological variables is the best system of data collection in this field.
This is a good ask and provides a convenient opportunity for me to summarize my views and then stop talking about it.
it seems everyone conflated very different fields? Obviously, “I am depressed because of immutable characteristics of society” isn’t a useful observation, it’s just one of the basic symptoms of depression.
A number of people said some version of this, that obviously this observation is useless and trite, but to pull out the tweet that prompted this, I don't think I am misrepresenting it:
And I guess this is on me for making my own post instead of just responding to the prior one, but I felt like a lot of people were more or less implying I was strawmanning instead of talking about a very real type of guy.
But “analyzing the relationship between societal impacts and psychology is useless because it offers no help for the individual” is just as pointless, as if general relativity is useless because it doesn’t teach you to pilot a plane.
Again, I'm going to quote myself here:
i guess i don't really understand the utility of the whole "cultural values and class relations" model of mental illness.
I am not saying here that it's useless to analyze the relationship between societal impacts and psychology. I don't believe that. But I also don't believe that's what the "cultural values and class relations" model is doing. I think it's vaguely gesturing at society using leftist-coded terminology with very little actual analysis.
I take it as a given that these aren't serious analytical models in the same way I take it as a given with trads who talk about the decline of marriage as a major negative impact - there's a kernel of truth here (we have research showing that married adults are happier than unmarried adults) but the selective focus on the speaker's political bugbears reveals that as its primary object, not the widespread improvement of people's mental health.
And in particular, both of these are frequently directed at people who talk about being individually mentally ill, which is why I think it's fair to grade them on their individual utility, even though people will often argue that "they aren't meant for that."
Collecting data regarding an entire population is just incomparable to an individual’s psychoanalysis. The latter may be more immediately useful, but it’s also not realistic treating it as a science until a better working model of the human brain is achieved. Until then, comparing various populations’ emotional and psychological reactions along sociological variables is the best system of data collection in this field.
I think you are overstating your argument a bit here. I think dissing psychology/psychiatry in comparison to sociology is missing the mark - it's not like sociology is some shining standard of scientific investigation. We also can and do perform good studies on psychiatric interventions, and indeed, many of these studies have informed my skepticism of clinical psychiatry.
30 notes
·
View notes
Text
Intelligent Data Management in Life Sciences: A Game Changer for the Pharmaceutical Industry
In the fast-paced world of life sciences and pharmaceuticals, data management is crucial for driving innovation, enhancing compliance, and ensuring patient safety. With an ever-growing volume of data being generated across clinical trials, drug development, and regulatory compliance, pharmaceutical companies face the challenge of managing and analyzing this vast amount of data efficiently. Intelligent data management offers a solution to these challenges, ensuring that organizations in the life sciences industry can harness the full potential of their data.
Mastech InfoTrellis is a leader in implementing AI-first data management solutions, enabling pharmaceutical companies to streamline their operations, improve decision-making, and accelerate their research and development efforts. This blog explores the critical role of intelligent data management in the pharmaceutical industry, focusing on how Mastech InfoTrellis helps companies navigate data complexity to enhance business outcomes.
What Is Intelligent Data Management in Life Sciences?
Intelligent data management refers to the use of advanced technologies, such as artificial intelligence (AI), machine learning (ML), and automation, to manage, analyze, and leverage data in a way that improves operational efficiency and decision-making. In the life sciences industry, data is generated from various sources, including clinical trials, electronic health records (EHR), genomic research, and regulatory filings. Intelligent data management solutions help pharmaceutical companies streamline the collection, organization, and analysis of this data, making it easier to extract actionable insights and comply with stringent regulatory requirements.
Mastech InfoTrellis applies cutting-edge data management solutions tailored to the pharmaceutical industry, focusing on improving data accessibility, enhancing data governance, and enabling real-time analytics for better decision-making.
Join - ReimAIgined Intelligence at Informatica World 2025
The Importance of Data Management in the Pharmaceutical Industry
Effective data management is the backbone of the pharmaceutical industry. With the increasing volume of data generated in drug discovery, clinical trials, and regulatory compliance, pharmaceutical companies need intelligent systems to handle this data efficiently. Poor data management can lead to significant challenges, such as:
Regulatory non-compliance: In the pharmaceutical industry, compliance with global regulations, including those from the FDA and EMA, is paramount. Mishandling data or failing to track changes in regulations can lead to severe penalties and delays in product approvals.
Data silos: In many organizations, data is stored in different departments or systems, making it difficult to access and analyze holistically. This leads to inefficiencies and delays in decision-making.
Inaccurate data insights: Inaccurate or incomplete data can hinder the development of new drugs or the identification of critical health trends, affecting the overall success of research and development projects.
Intelligent data management solutions, such as those offered by Mastech InfoTrellis, address these issues by ensuring that data is accurate, accessible, and actionable, helping pharmaceutical companies optimize their workflows and drive better business outcomes.
Key Benefits of Intelligent Data Management in Life Sciences
1. Improved Data Governance and Compliance
In the pharmaceutical industry, data governance is a critical function, particularly when it comes to regulatory compliance. Intelligent data management solutions automate the processes of data validation, audit trails, and reporting, ensuring that all data handling processes comply with industry regulations.
Mastech InfoTrellis provides Informatica CDGC (Cloud Data Governance and Compliance), which ensures that data management processes align with industry standards such as Good Clinical Practice (GCP), Good Manufacturing Practice (GMP), and 21 CFR Part 11. This integration enhances data traceability and ensures that pharmaceutical companies can provide accurate and timely reports to regulatory bodies.
2. Enhanced Data Access and Collaboration
In a complex, multi-departmental organization like a pharmaceutical company, it is essential to have data that is easily accessible to the right stakeholders at the right time. Intelligent data management systems ensure that data from clinical trials, research teams, and regulatory departments is integrated into a unified platform.
With Mastech InfoTrellis's AI-powered Reltio MDM (Master Data Management) solution, pharmaceutical companies can break down data silos and provide a 360-degree view of their operations. This enables seamless collaboration between teams and faster decision-making across departments.
3. Faster Drug Development and Innovation
Pharmaceutical companies must make data-driven decisions quickly to bring new drugs to market efficiently. Intelligent data management accelerates the process by enabling faster access to real-time data, reducing the time spent on data gathering and analysis.
By leveraging AI and machine learning algorithms, Mastech InfoTrellis can automate data analysis, providing real-time insights into clinical trial results and research data. This accelerates the identification of promising drug candidates and speeds up the development process.
4. Real-Time Analytics for Better Decision-Making
In life sciences, every minute counts, especially during clinical trials and regulatory submissions. Intelligent data management systems provide pharmaceutical companies with real-time analytics that can help them make informed decisions faster.
By applying AI-powered analytics, pharmaceutical companies can quickly identify trends, predict outcomes, and optimize clinical trial strategies. This allows them to make data-backed decisions that improve drug efficacy, reduce adverse reactions, and ensure patient safety.
Mastech InfoTrellis: Transforming Data Management in the Pharmaceutical Industry
Mastech InfoTrellis is at the forefront of intelligent data management in the life sciences sector. The company's AI-first approach combines the power of Reltio MDM, Informatica CDGC, and AI-driven analytics to help pharmaceutical companies streamline their data management processes, improve data quality, and accelerate decision-making.
By leveraging Master Data Management (MDM) and Cloud Data Governance solutions, Mastech InfoTrellis empowers pharmaceutical companies to:
Integrate data from multiple sources for a unified view
Enhance data accuracy and integrity for better decision-making
Ensure compliance with global regulatory standards
Optimize the drug development process and improve time-to-market
Real-World Use Case: Improving Clinical Trial Efficiency
One real-world example of how intelligent data management is revolutionizing the pharmaceutical industry is the use of Mastech InfoTrellis's Reltio MDM solution in clinical trials. By integrating data from multiple trial sites, research teams, and regulatory bodies, Mastech InfoTrellis helped a major pharmaceutical company reduce the time spent on data gathering and processing by over 30%, enabling them to focus on analyzing results and making quicker decisions. This improvement led to a faster drug approval process and better patient outcomes.
People Also Ask
How does data management benefit the pharmaceutical industry?
Data management in the pharmaceutical industry ensures that all data, from clinical trials to regulatory filings, is accurate, accessible, and compliant with industry regulations. It helps streamline operations, improve decision-making, and speed up drug development.
What is the role of AI in pharmaceutical data management?
AI enhances pharmaceutical data management by automating data analysis, improving data accuracy, and providing real-time insights. AI-driven analytics allow pharmaceutical companies to identify trends, predict outcomes, and optimize clinical trials.
What are the challenges of data management in the pharmaceutical industry?
The pharmaceutical industry faces challenges such as data silos, regulatory compliance, and the sheer volume of data generated. Intelligent data management solutions help address these challenges by integrating data, automating governance, and providing real-time analytics.
Conclusion: The Future of Data Management in Life Sciences
Intelligent data management is no longer just an option for pharmaceutical companies—it's a necessity. With the power of AI, machine learning, and advanced data integration tools, Mastech InfoTrellis is helping pharmaceutical companies improve efficiency, compliance, and decision-making. By adopting these solutions, life sciences organizations can not only enhance their current operations but also position themselves for future growth and innovation.
As the pharmaceutical industry continues to evolve, intelligent data management will play a critical role in transforming how companies develop and deliver life-changing therapies to the market.
2 notes
·
View notes
Text
What are the skills needed for a data scientist job?
It’s one of those careers that’s been getting a lot of buzz lately, and for good reason. But what exactly do you need to become a data scientist? Let’s break it down.
Technical Skills
First off, let's talk about the technical skills. These are the nuts and bolts of what you'll be doing every day.
Programming Skills: At the top of the list is programming. You’ll need to be proficient in languages like Python and R. These are the go-to tools for data manipulation, analysis, and visualization. If you’re comfortable writing scripts and solving problems with code, you’re on the right track.
Statistical Knowledge: Next up, you’ve got to have a solid grasp of statistics. This isn’t just about knowing the theory; it’s about applying statistical techniques to real-world data. You’ll need to understand concepts like regression, hypothesis testing, and probability.
Machine Learning: Machine learning is another biggie. You should know how to build and deploy machine learning models. This includes everything from simple linear regressions to complex neural networks. Familiarity with libraries like scikit-learn, TensorFlow, and PyTorch will be a huge plus.
Data Wrangling: Data isn’t always clean and tidy when you get it. Often, it’s messy and requires a lot of preprocessing. Skills in data wrangling, which means cleaning and organizing data, are essential. Tools like Pandas in Python can help a lot here.
Data Visualization: Being able to visualize data is key. It’s not enough to just analyze data; you need to present it in a way that makes sense to others. Tools like Matplotlib, Seaborn, and Tableau can help you create clear and compelling visuals.
Analytical Skills
Now, let’s talk about the analytical skills. These are just as important as the technical skills, if not more so.
Problem-Solving: At its core, data science is about solving problems. You need to be curious and have a knack for figuring out why something isn’t working and how to fix it. This means thinking critically and logically.
Domain Knowledge: Understanding the industry you’re working in is crucial. Whether it’s healthcare, finance, marketing, or any other field, knowing the specifics of the industry will help you make better decisions and provide more valuable insights.
Communication Skills: You might be working with complex data, but if you can’t explain your findings to others, it’s all for nothing. Being able to communicate clearly and effectively with both technical and non-technical stakeholders is a must.
Soft Skills
Don’t underestimate the importance of soft skills. These might not be as obvious, but they’re just as critical.
Collaboration: Data scientists often work in teams, so being able to collaborate with others is essential. This means being open to feedback, sharing your ideas, and working well with colleagues from different backgrounds.
Time Management: You’ll likely be juggling multiple projects at once, so good time management skills are crucial. Knowing how to prioritize tasks and manage your time effectively can make a big difference.
Adaptability: The field of data science is always evolving. New tools, techniques, and technologies are constantly emerging. Being adaptable and willing to learn new things is key to staying current and relevant in the field.
Conclusion
So, there you have it. Becoming a data scientist requires a mix of technical prowess, analytical thinking, and soft skills. It’s a challenging but incredibly rewarding career path. If you’re passionate about data and love solving problems, it might just be the perfect fit for you.
Good luck to all of you aspiring data scientists out there!
#artificial intelligence#career#education#coding#jobs#programming#success#python#data science#data scientist#data security
9 notes
·
View notes
Text
Why Tableau is Essential in Data Science: Transforming Raw Data into Insights
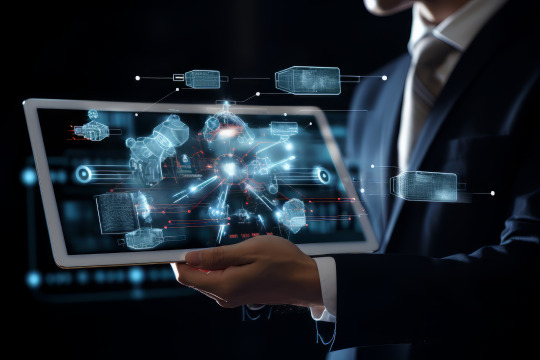
Data science is all about turning raw data into valuable insights. But numbers and statistics alone don’t tell the full story—they need to be visualized to make sense. That’s where Tableau comes in.
Tableau is a powerful tool that helps data scientists, analysts, and businesses see and understand data better. It simplifies complex datasets, making them interactive and easy to interpret. But with so many tools available, why is Tableau a must-have for data science? Let’s explore.
1. The Importance of Data Visualization in Data Science
Imagine you’re working with millions of data points from customer purchases, social media interactions, or financial transactions. Analyzing raw numbers manually would be overwhelming.
That’s why visualization is crucial in data science:
Identifies trends and patterns – Instead of sifting through spreadsheets, you can quickly spot trends in a visual format.
Makes complex data understandable – Graphs, heatmaps, and dashboards simplify the interpretation of large datasets.
Enhances decision-making – Stakeholders can easily grasp insights and make data-driven decisions faster.
Saves time and effort – Instead of writing lengthy reports, an interactive dashboard tells the story in seconds.
Without tools like Tableau, data science would be limited to experts who can code and run statistical models. With Tableau, insights become accessible to everyone—from data scientists to business executives.
2. Why Tableau Stands Out in Data Science
A. User-Friendly and Requires No Coding
One of the biggest advantages of Tableau is its drag-and-drop interface. Unlike Python or R, which require programming skills, Tableau allows users to create visualizations without writing a single line of code.
Even if you’re a beginner, you can:
✅ Upload data from multiple sources
✅ Create interactive dashboards in minutes
✅ Share insights with teams easily
This no-code approach makes Tableau ideal for both technical and non-technical professionals in data science.
B. Handles Large Datasets Efficiently
Data scientists often work with massive datasets—whether it’s financial transactions, customer behavior, or healthcare records. Traditional tools like Excel struggle with large volumes of data.
Tableau, on the other hand:
Can process millions of rows without slowing down
Optimizes performance using advanced data engine technology
Supports real-time data streaming for up-to-date analysis
This makes it a go-to tool for businesses that need fast, data-driven insights.
C. Connects with Multiple Data Sources
A major challenge in data science is bringing together data from different platforms. Tableau seamlessly integrates with a variety of sources, including:
Databases: MySQL, PostgreSQL, Microsoft SQL Server
Cloud platforms: AWS, Google BigQuery, Snowflake
Spreadsheets and APIs: Excel, Google Sheets, web-based data sources
This flexibility allows data scientists to combine datasets from multiple sources without needing complex SQL queries or scripts.
D. Real-Time Data Analysis
Industries like finance, healthcare, and e-commerce rely on real-time data to make quick decisions. Tableau’s live data connection allows users to:
Track stock market trends as they happen
Monitor website traffic and customer interactions in real time
Detect fraudulent transactions instantly
Instead of waiting for reports to be generated manually, Tableau delivers insights as events unfold.
E. Advanced Analytics Without Complexity
While Tableau is known for its visualizations, it also supports advanced analytics. You can:
Forecast trends based on historical data
Perform clustering and segmentation to identify patterns
Integrate with Python and R for machine learning and predictive modeling
This means data scientists can combine deep analytics with intuitive visualization, making Tableau a versatile tool.
3. How Tableau Helps Data Scientists in Real Life
Tableau has been adopted by the majority of industries to make data science more impactful and accessible. This is applied in the following real-life scenarios:
A. Analytics for Health Care
Tableau is deployed by hospitals and research institutions for the following purposes:
Monitor patient recovery rates and predict outbreaks of diseases
Analyze hospital occupancy and resource allocation
Identify trends in patient demographics and treatment results
B. Finance and Banking
Banks and investment firms rely on Tableau for the following purposes:
✅ Detect fraud by analyzing transaction patterns
✅ Track stock market fluctuations and make informed investment decisions
✅ Assess credit risk and loan performance
C. Marketing and Customer Insights
Companies use Tableau to:
✅ Track customer buying behavior and personalize recommendations
✅ Analyze social media engagement and campaign effectiveness
✅ Optimize ad spend by identifying high-performing channels
D. Retail and Supply Chain Management
Retailers leverage Tableau to:
✅ Forecast product demand and adjust inventory levels
✅ Identify regional sales trends and adjust marketing strategies
✅ Optimize supply chain logistics and reduce delivery delays
These applications show why Tableau is a must-have for data-driven decision-making.
4. Tableau vs. Other Data Visualization Tools
There are many visualization tools available, but Tableau consistently ranks as one of the best. Here’s why:
Tableau vs. Excel – Excel struggles with big data and lacks interactivity; Tableau handles large datasets effortlessly.
Tableau vs. Power BI – Power BI is great for Microsoft users, but Tableau offers more flexibility across different data sources.
Tableau vs. Python (Matplotlib, Seaborn) – Python libraries require coding skills, while Tableau simplifies visualization for all users.
This makes Tableau the go-to tool for both beginners and experienced professionals in data science.
5. Conclusion
Tableau has become an essential tool in data science because it simplifies data visualization, handles large datasets, and integrates seamlessly with various data sources. It enables professionals to analyze, interpret, and present data interactively, making insights accessible to everyone—from data scientists to business leaders.
If you’re looking to build a strong foundation in data science, learning Tableau is a smart career move. Many data science courses now include Tableau as a key skill, as companies increasingly demand professionals who can transform raw data into meaningful insights.
In a world where data is the driving force behind decision-making, Tableau ensures that the insights you uncover are not just accurate—but also clear, impactful, and easy to act upon.
#data science course#top data science course online#top data science institute online#artificial intelligence course#deepseek#tableau
3 notes
·
View notes
Text
Clinical Nutrition & Dietetics: Science for Better Health
Clinical Nutrition and Dietetics is a specialized field that uses nutrition to manage and prevent disease, improve health outcomes, and promote overall well-being. Here’s a deep dive into the essential aspects of this field:

What is Clinical Nutrition and Dietetics?
Clinical Nutrition and Dietetics involves assessing, diagnosing, and treating nutrition-related health issues. Dietitians and clinical nutritionists work closely with patients to develop dietary plans tailored to their medical conditions, lifestyle, and specific health goals.
Key Areas of Clinical Nutrition and Dietetics
Medical Nutrition Therapy (MNT): MNT is the cornerstone of clinical dietetics, involving specialized dietary interventions based on scientific evidence. It’s used to treat chronic illnesses like diabetes, cardiovascular disease, cancer, and kidney disease.
Nutritional Assessment: This includes evaluating a patient’s diet, medical history, physical health, and lab results to create personalized nutrition plans. Tools include BMI, body composition analysis, dietary history, and blood tests to assess nutrient levels.
Therapeutic Diets: Dietitians often develop therapeutic diets to manage health conditions, which can include:
Low-sodium diets for hypertension and heart health.
Low-glycemic diets for managing diabetes.
High-protein diets for malnutrition and muscle recovery.
Renal Diets for kidney disease patients to reduce the intake of specific nutrients.
Pediatric and Geriatric Nutrition: Clinical dietitians specialize in creating age-appropriate nutritional plans for children and elderly patients, addressing issues like growth, development, bone health, and cognitive function.
Mental Health and Nutrition: Dietitians are increasingly focusing on the connection between nutrition and mental health, as certain nutrients (e.g., omega-3s, and B vitamins) can impact mood and cognitive function.
Emerging Areas in Clinical Nutrition
Functional Foods and Nutraceuticals: Functional foods (like probiotics) and nutraceuticals (such as dietary supplements) are increasingly used in clinical nutrition to support specific health outcomes, such as immune function or gut health.
Personalized Nutrition and Genomics: Nutrigenomics studies how genes influence individual responses to nutrients, leading to personalized nutrition plans based on a patient’s genetic makeup. This method works especially well for treating chronic illnesses.
Integrative and Holistic Nutrition: Integrative nutrition considers lifestyle factors, stress, and mental health along with diet, promoting a more holistic approach to patient care.
Plant-Based Diets: The use of plant-based diets in clinical settings is becoming popular for their benefits in reducing inflammation, improving heart health, and supporting weight management.
Role of Clinical Dietitians in Healthcare Settings
Hospitals: Clinical dietitians are essential in hospitals, where they design dietary plans for patients recovering from surgeries, dealing with chronic illnesses, or undergoing treatment that affects their nutritional status.
Outpatient Clinics: Many dietitians work in clinics, providing ongoing support for patients with chronic conditions like diabetes or high cholesterol.
Rehabilitation Centers: Nutritionists here help patients with recovery, focusing on high-calorie or high-protein diets to promote healing.
Skills for Clinical Dietitians
Analytical Skills: Strong understanding of biochemistry and physiology to interpret lab data and develop dietary plans. Counseling and Communication: The ability to communicate effectively with patients to promote adherence to dietary plans.
Evidence-Based Practice: Staying updated with the latest research to provide science-backed advice. Career Opportunities
Clinical dietitian: employed by long-term care homes, clinics, or hospitals.
Nutrition Researcher: Contributing to research on disease prevention and dietary interventions.
Consultant Dietitian: Providing freelance or consultancy services for healthcare facilities, wellness centers, or private clients.
Corporate Wellness Programs: Supporting employees’ health and well-being through nutritional guidance in corporate settings.
Conclusion
In conclusion, Clinical Nutrition and Dietetics is a transformative field that bridges the gap between nutrition science and patient care, offering personalized approaches to health and wellness. By understanding the intricate relationship between diet, disease, and overall health, clinical dietitians play a crucial role in improving health outcomes and enhancing quality of life. As new research and innovations in nutrition continue to emerge, this field remains essential in advancing preventive care, supporting disease management, and promoting holistic well-being.
3 notes
·
View notes
Text
"The Role of Data Analytics in Optimizing Mobile App User Experience?"
Google Ads is one of the best tools to expand visibility, traffic, and conversions for any business. However, even the most solid businesses sometimes make mistakes in their Google Ads campaigns, and a massive ad spend gets wasted along with an unsatisfying performance. Being a beginner in Google Ads or a professional who has been running campaigns for some time, you should definitely avoid common mistakes, which means optimizing your campaigns towards better performance.
In this blog, the most common mistakes committed in Google Ads, how to avoid them, and therefore how to improve your campaign's performance, will be discussed. Once some of these issues are corrected, you can surely get cost-effective Google Ads campaigns that result in the intended outcome.
Mistake 1: Ignoring Keyword Match Types
You will have to decide which one of the keywords is to be used for your Google Ads campaign. Most businesses commit the mistake of using broad-match keywords only. This will invite irrelevant clicks on your ad, which will in turn increase the costs too. With broad match keywords, Google will trigger your ad for any search term that it deems related. Your ad would thus be shown for relevant, yet possibly irrelevant queries.
Thus, in order to avoid this, one needs to know various kinds of match types available for keywords.
Broad Match: Your ad is shown to users related to what you are looking for, though there is also the possibility of accidentally clicking on your ads.
Phrase Match: The search term contains the exact phrase or close variant.
Exact Match: Your ad will be shown to the searcher only if their search term exactly matches your keyword.
Mix up your match types and frequently check your search term reports for keywords that are irrelevant and might need to be disallowed. Negative keywords in general are critical to include to stop your ads from appearing for irrelevant searches.
Error 2: Poor Tracking of Conversions
Conversion tracking is one of the most crucial parts of any winning campaign in Google Ads. Without this setting, you'll have no idea how your ads are actually working or where they need to be improved to yield better results. Many businesses either don't activate conversion tracking or set it up wrong, leaving them with incomplete data and missed opportunities.
Avoid it by:
Make sure that you set up conversion tracking correctly for your campaigns. To do this, follow these specific steps.
Determine Your Conversions: Determine what kind of conversion would be taking place for your business. It might be a sale, lead submission, or a call.
Setup Conversion Tracking: Add the Google Ads conversion tracking code to your site so that you ensure it is put on the "thank you" or confirmation page when the conversion takes place.
Test the setup: After installing the tracking code, you must test to ensure it's working correctly.
You'll get a real insight into which ads are generating the most value for your website by accurately tracking conversions. You can then make any necessary adjustments to your campaigns.
Mistake 3: Not Using Ad Extensions
Ad extensions are one of the most accessible ways to enhance your Google Ads performance. Unfortunately, only a few use them. The more ad extensions you use with your ad, the more information about your business is probably going to appear for users—for example, your phone number, your location, or specific products or services. This way, you are more likely to have more people like it enough to click on it. The thing is, if you are not using ad extensions, then you are missing out on an opportunity to expand the click-through rate and then increase ad relevance.
How to Avoid It
In addition to these, Google offers several ad extensions to help in improving the performance of the ads. These include:
Sitelink Extensions: This sends people directly to pages on your website.
Call extensions: Gives your business a number so that potential customers can reach you easily.
Location Extensions: Gives your business address to searchers in your locality
Callout Extensions: Draws more attention to the special features or offers of your business; for instance, "Free Shipping" or "24/7 Support."
Install ad extensions that will help you align your campaigns with your business goals. They will not only stretch the size of your ad but also enhance your CTR and quality score.
Mistake 4: Set and forget campaigns
You begin your campaign on Google Ads and fail to keep track of performance on a regular basis. Google Ads is not a "set and forget" kind of platform. Without daily attention, over time your ads typically become less effective and burn your money performing poorly.
How to Avoid It:
To avoid this, you need to monitor your campaign performance quite often. Here are some of the essential things you should monitor on a regular basis:
Search Terms Report: It would detail which queries trigger your ads, hence refining your keyword list and adding negative keywords.
Ad Performance: Review the CTR, conversion rate, and cost per conversion of your ads to determine which ones are performing well and which areas need to be improved.
Budget and Bids: Also, ensure your budget and bids are properly optimized for your goals. Elevate bids for good-performing keywords and reduce spends on lower-performing keywords.
Campaigns could be optimized regularly to provide better performance and return on the advertisement expenses.
Mistake 5: Not Targeting the Right Audience
Another common mistake in Google Ads is that it often fails to define and target the right audience. Otherwise, without proper targeting of the right audience, the reach of your ads might be receiving clicks from people who are not interested in what you have, hence leading to wasted clicks and minimal conversions.
How to Avoid Mistakes:
To avoid this mistake, one can maximize the audience-targeting features of Google Ads. Some options include:
Demographic Targeting: Target people according to the age group, gender, and income of the household.
Location Targeting: Target the users where your business is geographically located.
Remarketing: Target users who have visited your website but have not yet converted. Advertise to the right audience to improve the efficiency of your campaign, thereby increasing relevant traffic to your website.
Conclusion
Google Ads can be an extremely powerful marketing tool only if campaigns are set up and managed properly. Therefore, it is advisable to avoid common mistakes like ignoring keyword match types, failing to track conversions, underutilizing ad extensions, failing to regularly manage campaigns, and wrong targeting towards the audience, which can significantly improve campaign performance.
Monitoring and using more strategic approaches in your Google Ads campaigns will help you optimize your ads to give you the very best-performing results, cost reduction, and ROI.
Visit TechoSquare for more optimization tips from your digital marketing campaign and discover our range of services that can enable you to take your online presence to the next level!
"Discover common Google Ads mistakes, learn how to avoid them, and improve your campaign performance to maximize ROI with strategic optimization tips."
#digital marketing#data analytics courses#data analytics tools#data analytics process#optimising app#optimizing mobile app#data science or data analytics which is better
0 notes
Text
Re-thinking Marketting 101
They say that companies just can't figure out how to market their toys (and other goods) without the gender divide. I'm here to call them idiots.
"Blue means boy, Pink means girl" has created a decision starting in the toy aisle and making its way all the way to funerals. Which is *ridiculous* considering that caskets aren't inherently a gendered item.
And because of that simple idea, the varying aisles separate everything they possibly can according to gender.
Which, despite literally reducing their customer base; and thus sales: leads to some *really* strange interpretations of data based on the premise rather than their end goal.
And even leads to the Razor aisle where women's razors are branded luxury items. Denoting how *gently* their razors get clogged with the removed hairs.
Despite both colors of razors having essentially the same technological innovation; it seems to have an impact. Considering that "Agender" razors would break the cultural norm and just be "Too Weird" for customers to purchase.
This has real world impacts as well. Consider the toy Aisle again; when Video Games used to be "Boy Toys" before the electronics aisle was invented.
Because if this branding a cultural identity was created around Stem, and video games, that Entertainment was for children; specifically boys. And kitchens were for women.
It persisted to the point that, in America at least; Women *need* special STEM[Science, Technology, Engineering, Math] programs in order to convince them that it's not just an educational path for people that have Stamen.
Despite the fields literally being developed by hyper-intelligent women that were feminized out of the field to begin with.
Similar to how Women in Iran only recently were convinced that they were smart enough to drive cars; a right they had until about a decade ago.
Why do these myths about branding persist? Why do they work? And more importantly, why does it make people think certain genders are smarter than others?
You know; despite a large number of Millennial Parents knowing better these days?
What researchers and trans people have figured out is that the genderfication of items do appeal more to a mental aspect of a person.
The categories exist for a reason; because sometime they work at describing a certain type of person. Despite the label being incredibly incorrect as to what *exactly* it is describing.
A correlation was found, and it seemed to describe a certain data set. Despite... You know; it describing a set of interests and not a set of genitals.
Are men *more* likely to buy Manly gendered things over perceived feminine items? Or are they more likely to buy gener-neutral variants of things that probably shouldn't be gendered?
This is a question for MattPatt's unreleased fifth channel; MarketTheory. Ironically the marketing analytics were not good enough to support the idea.
That companies and marketing specialists; can't figure out how to recategorize their customer bases is precisely the reason why nobodies on YouTube do so much better with the algorithm than offline business.
They're so used to these categorizations that it comes second nature in a way that they can't explain because they don't have the terminology for it.
And yet; seemingly overnight have not only given offline companies a run for their money--but destroyed entire industries without even realizing it.
And that all circles back to the only two marketing categories that companies can seem to understand "Boy and Girl"... Except... For the third category that broke those barriers long ago; The Electronics Aisle, filled to the brim with Agender toys *only* because they don't fit the paradigm set by the other two aisles.
5 notes
·
View notes
Text
Gardening in a Stone Economy
Remake + ○●-Severance Hybrid Bubble: A data science enthusiast is economically compelled to settle for a Go job in her alternate reality, where costs for higher-level computational processing like her original specialty are exorbitant due to resource depletion, AlphaGo and the like have not been invented and organizations resort to mind-control headsets that translate the logic in mundane onscreen work to gameplay logic. These headsets fortuitously use materials not yet in scarcity in that world. The closer the work meets end goals and procedural standards, the better the mentally displayed Go game progresses. The purpose of the translation is two-fold: 1) achieve watertight protection of commercial secrecy and 2) boost employee motivation in a compact, non-graphics-intensive manner.
But since even Go experts may falter on bad-hair days, work protocols limit employees to a small range of moves for each narrowly defined game scenario, which means office jobs still induce yawns regardless of one's fondness of Go. Worse, workers spend years in Go academies only to face potential skill attrition in autonomous analytical and strategic thinking and in solution creativity as they work round the clock in this manner until elderhood.
Intelligence and knowledge perish sooner than one's capacity for altruism, provided the will for the latter lasts. Faced with the same bleak circumstances, some strive to rise above their station in life in a self-determined sense by not only enduring the grind with increasing grit (as far as self-care permits) and rallying around their teams but also extending comradeship and empathy to everyone, whereas some help themselves Misaeng villain-style to what they see as substitute additional compensation: corporate moneys and female playthings.
The heroine stoically sticks to the former approach, Misaeng hero-style. Her spiritual counsel is a set of principles from her data science days:
Garbage in, Garbage out: How much do you trust ethical decision-making founded on empty stomachs, sleep-deprived brains and hatred-consumed memories? Don't ill-treat yourself yet expect to be unfailingly seen as a good grid conqueror. Don't ill-treat your co-workers yet expect unfailing support from them when you slip into a needy position. (But don't expect sympathy either if you cite your reception of ill treatment as justification for your ill treatment of someone. When people are struggling to escape your claws, they do not have the cognitive bandwidth to analyze your personal history.)
Actionable Insights: Endlessly regurgitating negative experiences you are powerless to redress through yourself or through others perpetuates the pain, although perpetual flashbacks are sometimes passive phenomena individuals are powerless to stop. Look out for facts you can act on. For example, do you feel more irritable as the night thickens? How about investing in a cozy LED lamp to boost your enemy-encircling efficiency after dusk? Does your brain come alive during the dull workday only during lunch? How about snacking on colorful berries as you move your stones?
Watch out for Outliers: See beyond immediate gratification and momentary bruises to the ego for the full picture. While do-no-gooders collect future lawsuits, festering grudges, and other ticking time-bombs, you plot your narrowing financial distance to your dreams for each day of hardship or plot your growing insights into multifaceted human nature for each negotiation on fair game allocation.
Mindful Annotation: A small act of kindness can be a quick glow-up and perk-me-up. A small thought for others can be respite from the prison of your own anxieties. In contrast, don't you ever wonder why various screen characters pursue evil as a vocation only to look perpetually stressed and on the guard? What begin as petty comparison and moderate insecurity in those series blow up into messy huge schemes and constant paranoia. Real-life victims may believe, too, that their thirst for justice outlasts perpetrators' feelings of dominion and thrill. Moreover, workplace guidelines and public discourse are increasing on honest stone laborers' side.
Self-care and self-improvement do not imply surrendering to an unhealthy work-life arrangement. The ending reminds us of this as the heroine runs along rooftops above congested streets to submit a labor reform petition on time. We see in parallel a sequence of her leaping between roofs and another of Misaeng's protagonist doing nearly the same, except that a wide anti-suicide net visibly hangs between her roofs. There is no shame in valuing her life.
4 notes
·
View notes
Text
Data mining
1.What's Data mining ?
Datamining is the process of extracting and discovering patterns in large datasets involving methods at the intersection of machine learning, statistics and database systems. Datamining is interdisciplinary subfield of computer-science and statistics with overall goal of extracting information (with intelliegent methods) from a data set and transforming the information into a comprensible structure for further use. Data mining is the analysis step of The KDD process "Knowledge discovery in database".
2.What's KDD process ?
KDD process is known as "Knowledge Discovery in Database".It"s a multi-step process of finding knowledge from large data sets and emphasizes the high-level application of particular datamining methods.It's of interests to researchers in machine learning, pattern recognition, databases, ststistics, artificial intelligence, knowledge aquisition for experts systems and data-visualization. The picture below defines the different steps of KDD process and each of those steps have an input and output entity. The KDD process can't be executed without beginning on data.

3.What are the different steps of the KDD process ?
The overall process of finding and interpretting patterns from data involves the repeated application of the following steps mentioned in the graph above :
Selection : we create a target data set by seecting a part of the overall data set as a sample then focusing on a subset of variables on which discovery is to be performed. The result of these step is a subset of data considered as a sample.
Preprocessing : These step of the KDD process takes the target data set as an input then it applyes data cleaning by removing the noise from the input data set then restucturing the data set. The output of these operation is a preprocessed dataset that can be able to be transformed in the next step.
Data transformation : These step takes the preprocessed data as input and tres to find some useful features depending on the goal of the task and reducing dimension to execute an effective learining datamining.
Data mining : in this phase we will descide whether the goal of KDD process is classification, regression, clustering ...etc. Discover the patterns of interests.
Interpretation : Interpretating mined patterns and consolidating discovered knowledge.
4.What are data mining tasks ?
There are several steps that are defined in the sub-process of KDD especially in datamining steps. In Data mining, there are 02 types of data mining that are :
Predictive mining: predective data mining is the analysis done to predict a future event or other data or trends and to predict something will happen in the near future. Predective data mining offers a better future analysis and to make better decisions to add a value in predective analytics like for example predecting the future customer of a defined service, define the future price of oil and gaz in the world market, define the next ill of an international pandemic, define the future political conflict ... etc. There are 4 types of descriptive data mining tasks which are :
Classification analysis : It is used to retrieve critical and pertinent data and metadata. It categorizes information into various groups. Classification Analysis is best demonstrated by email providers. They use algorithms to determine whether or not a message is legitimate.
Regression Analysis : It tries to express the interdependence of variables. Forecasting and prediction are common applications.
Time Serious Analysis : It is a series of well-defined data points taken at regular intervals.
Prediction Analysis : It is related to time series, but the time isn’t restricted.
Descriptive mining : descriptive data mining is to describe data and make data more readable to human beings, it's used to extract information from previous events and data and to discovering an interesting patterns and association behind data. It's also used to exract correlations, relationships between features and finding new laws and regularities based on data. There are four different types of Descriptive Data Mining tasks. They are as follows :
Clustering analysis : It is the process of determining which data sets are similar to one another. For example, to increase conversion rates, clusters of customers with similar buying habits can be grouped together with similar products.
Summerazation analysis : It entails methods for obtaining a concise description of a dataset. For example, summarising a large number of items related to Christmas season sales provides a general description of the data, which can be extremely useful to sales and marketing managers.
Association rules analysis : This method aids in the discovery of interesting relationships between various variables in large databases. The retail industry is the best example. As the holiday season approaches, retail stores stock up on chocolates, with sales increasing before the holiday, which is accomplished through Data Mining.
Sequence discovery analysis : It's all about how to do something in a specefic order. For instance, a user may frequently purchase shaving gel before purchasing razor in a store.It all comes down to the order in which the user purchases the product, and the store owner can then arrange the items accordingly.
5.Links :
3 notes
·
View notes
Text
Idol's MBTI Types pt 2
A short list of kpop idols and their likely MBTI types. I know most have taken a test by using 16personalities, but it does not actually use MBTI. This half is focused on judging function doms (Te, Ti, Fe, Fi).
These are just brief explainations as I did not want to make this post too long. I will provide more in depth analysis later.
Not an expert. Based on my opinion and observations. May change later.
Pt 1
___
Functions:
Ni (Introverted Intution) Ne (Extroverted Intuition)
Te (Extroverted Thinking) Ti (Introverted Thinking)
Fe (Extroverted Feeling) Fi (Introverted Feeling)
Se (Extroverted Sensing) Si (Introverted Sensing)
___
ExTJ (Te - Pi - Pe - Fi)
Te doms are task and goal oriented, always seeking efficiency and control. ExTJs do whatever it takes to achieve their goals, even if it means disregarding their own needs and wants (inferior Fi). This gives them their cold heartless stereotype. Te doms are solution providers and advice givers rather than emotional comforters. They prefer to use established facts and systems to work with.
ENTJ (Te - Ni - Se - Fi)
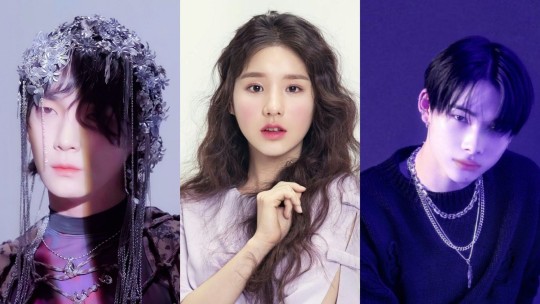
SHINee Key, Loona Heejin, ENHYPEN Ni-Ki
ENTJs value efficiency and accomplishments, completing one goal after another to achieve their desired future. Almost everything they do is for achievement.
Key is very strategic and organized (Te-Ni). He planned his Bad Love album for years, making sure it absolutely matched his vision (Ni).
I am not too familiar with Heejin or Ni-Ki but I needed 2 more examples lol...And from reading a bit about them from others, they seem to utilize ENTJ's functions well.
___
ESTJ (Te - Si - Ne - Fi)
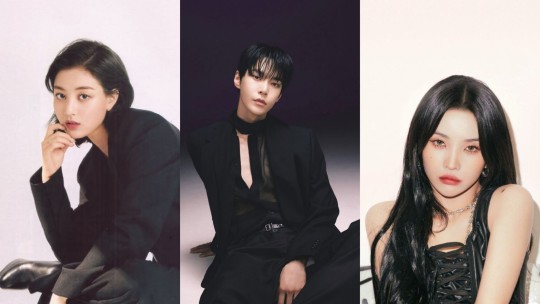
TWICE Jihyo, NCT Doyoung, (G)-IDLE Soyeon
ESTJs are the enforcers and organizers. They have set routines and procedures they prefer for everyone to follow. If needed, they can be creative with solutions for new/unusual situations since they're so determined to push through things.
Jihyo uses her Te to get tasks done. She's always been a natural at organizing her environment and pushing others to reach goals.
Doyoung is straightforward and honest. He always decides what's best for the situation and for the team. He has good leadership skills, which is natural for many Te doms.
Soyeon seems to be very goal oriented, pushing herself and others to achieve new and big things.
___
IxTP (Ti - Pe - Pi - Fe)
Ti doms are analytical and logical, using subjective knowledge to decide what course of action makes the most sense. They look for consistencies in information to put into their own set of data. It's like piecing a puzzle for them. They have a tendency to be honest and blunt, as Ti does not see the need to sugarcoat. This is also due to their inferior Fe - they may want social harmony but do not know how to obtain it. They can also struggle to express their own emotions as they find it awkward. They may also fear the conflict it can bring, which is something Fe dislikes.
INTP (Ti - Ne - Si - Fe)
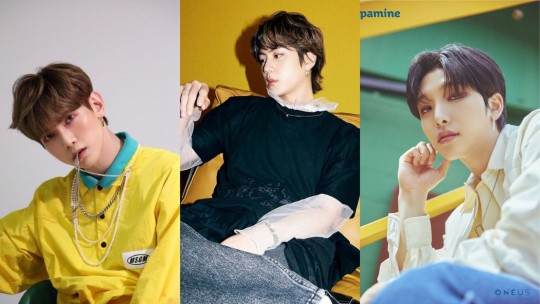
ATEEZ Yeosang, BTS Jin, ONEUS Seoho
INTPs are analytical and logical, with Ne trying to come up with better, more polished ideas. They have a stored system for all the ideas and information they have analyzed. They like to rearrange their framework to what is the most logical.
Yeosang is known for his bluntness and even the members are scared of it. He has the wit and pattern recognition of Ti - Ne. My analysis of him.
Jin has always been quite private and internalized, seemingly a bit awkward in social settings. He's good at deduction and good at such games. He also does not give up easily when arguing.
Seoho has an interest in sciences, was even planning to work in the field. He's blunt and straightforward and does not care enough to contribute to a peaceful environment.
___
ISTP (Ti - Se - Ni - Fe)

ONEUS Leedo, NCT Jeno, GOT7 Mark
ISTPs are often very independent and sensory seeking. They don't really have a preference for people, most often choosing to do activities alone. It allows them to ponder without any interruption.
Leedo does not seem to care much for social harmony - he's always honest and does not hesitate to get mad at his members.
Jeno enjoys complex things such as science and sensory activities. He has said he has no interest in others, showing inferior Fe.
Mark is very rational and logical, always thinks through his decisions. He's quite reactive and does not mind conflict, especially when he was younger (Se-Fe).
___
ExFJ (Fe - Pi - Pe - Ti)
Fe doms seek social harmony and place importance on other's values. They want to bring people together in order to achieve a shared goal. They generally put other people before themselves. Fe doms can influence other's feelings - if not careful, they can be manipulative. They may have hard time expressing their own opinions and arguments, fearing conflict and/or not feeling confident enough to word their analysis correctly.
ENFJ (Fe - Ni - Se - Ti)
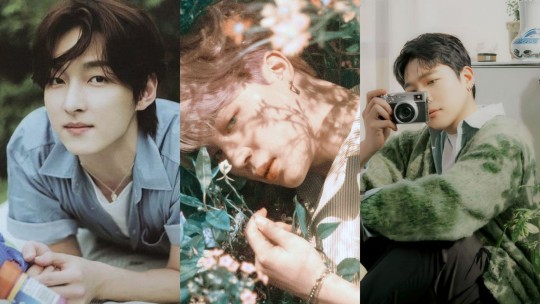
SF9 Zuho, BTS Jimin, DAY6 Sungjin
ENFJs are aware of their emotional environment, always putting a lot of focus into others needs and wants. Their observation of others' feelings can help them use the right words and actions to reach a set goal. They want to be a good influence and make meaningful change for people.
Zuho clearly values being with people, easily connecting with others (Fe). He has the natural charm that is common with Fe doms.
Jimin definitely cares a lot about other's perception of him and even wants to make meaningful change for others (Fe - Ni).
Sungjin is quite people oriented, even saying he wanted to be a counselor. This shows a preference of wanting to connect and understand other's emotions and mind (Fe-Ni).
___
ESFJ (Fe - Si - Ne - Ti)
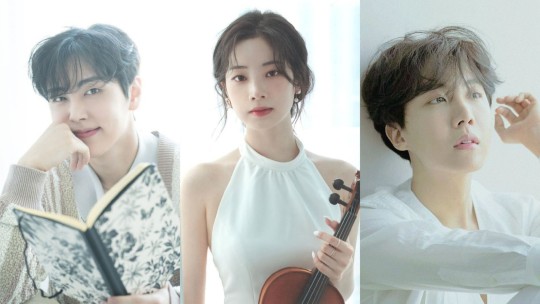
SF9 Jaeyoon, TWICE Dahyun, BTS J-Hope
ESFJs are aware of other's feelings and strive for connection. Fe - Si wants harmony and peace, with Si keeping track of valuable information of people. This helps to keep everyone and everything in check.
Jaeyoon is very caring and attentive towards others, gains energy from people. It's why and how he is very active on socials, always connecting with friends and fans. My analysis of him.
Dahyun is great at using her Fe - Ne to be an entertainer. She's also conflict avoidant and prefers to go with the flow (Fe - Si).
J-Hope is a social chameleon who naturally takes on the energy of others (Fe). He's very detail oriented and perfectionistic due to his Si.
___
IxFP (Fi - Pe - Pi - Te)
Fi doms are very internalized and private. They're concerned with authenticity, placing importance on their own values and morals. They want to be genuine and true to themselves. Deeply value ndividualistism. Because of this, they can be honest and strongly opinionated. They're more concerned with their own feelings and identity as they're the only things IxFPs feel confident controlling.
INFP (Fi - Ne - Si - Te)
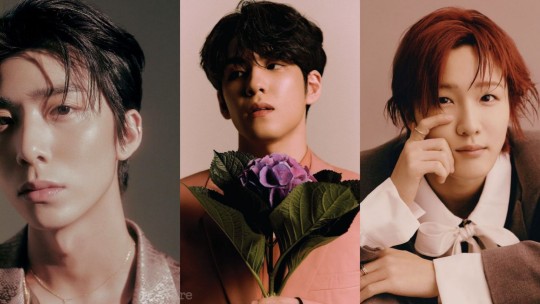
SF9 Hwiyoung, DAY6 Wonpil, P1Harmony Soul
INFPs are often considered daydreamers due to their internalized nature of Fi and idealism of aux Ne. They're prone to reminsicing about their identities and experiences. These experiences serve as lessons though, shaping their characters and beliefs.
Hwiyoung has admitted to being very sensitive and emotional, is strongly opinionated (Fi). He also tends to have his head in the clouds (Ne).
Wonpil places a lot of value on authenticity and is able to create ideas from connections in the environment.
Soul is...unique and is not shy to express it (Fi). He also has some out of the box ideas sometimes (Ne).
___
ISFP (Fi - Se - Ni - Te)

SF9 Youngbin, ATEEZ San, BLACKPINK Jennie
ISFPs are quite down to earth and realistic due to auxiliary Se. They still have a focus on expressing their individuality (Fi) and will often show this through physical means (Se). This can be seen through fashion, different art forms, performing, etc. With aux Se, they're a lot quicker to react and pursue things without hesitation. Passionate people, nothing will stop them from getting what they want.
Youngbin is known for his passionate nature, always giving pep talks with his members. He has even made them stay up very late despite having busy schedules just bc he had so much fiery energy lol. My analysis of him.
San always talks about being genuine and authentic. No matter what the person's status is, he always judges them by their heart, which is very Fi.
Jennie has stated that staying true to herself is the most important thing for her. She also expresses her style through her fashion, makeup, and music.
___
Individual idol profile analysis list
___
Other blog:
Kpop + astrology side blog @rainy-astrology
#mbti#kpop#sf9#typology#cognitive functions#myers briggs type indicator#myers briggs#ateez#shinee#twice#loona#enhypen#bts#nct#(g)i dle#got7#oneus#day6#entj#estj#enfj#esfj#infp#isfp#intp#istp#i hope those 2 anons see this 🫶💙 spite fueled this post#im sure they love the esfj portion#Kpop mbti
16 notes
·
View notes
Text
Python Development Course: Empowering the Future with Softs Solution Service
Python, a high-level programming language, has emerged as a favorite among developers worldwide due to its emphasis on readability and efficiency. Originating in the late 1980s, Python was conceived by Guido van Rossum as a successor to the ABC language. Its design philosophy, encapsulated by the phrase "Beautiful is better than ugly", reflects a commitment to aesthetic code and functionality.
What sets Python apart is its versatile nature. It supports multiple programming paradigms, including procedural, object-oriented, and functional programming. This flexibility allows developers to use Python for a wide range of applications, from web development and software engineering to scientific computing and artificial intelligence.
Python’s standard library is another of its strengths, offering a rich set of modules and tools that enable developers to perform various tasks without the need for additional installations. This extensive library, combined with Python’s straightforward syntax, makes it an excellent language for rapid application development.
One of Python's most significant contributions to the tech world is its role in data science and machine learning. Its easy-to-learn syntax and powerful libraries, like NumPy, Pandas, and Matplotlib, make it an ideal language for data analysis and visualization. Furthermore, frameworks like TensorFlow and PyTorch have solidified Python's position in the development of machine learning models.
Education in Python programming has become crucial due to its growing demand in the industry. Recognizing this, institutions like Softs Solution Service, IT training institute in Ahmedabad, have stepped up to provide comprehensive Python Development Training. Their Online Python Development Course is tailored to meet the needs of both beginners and seasoned programmers. This course offers an in-depth exploration of Python's capabilities, covering everything from basic syntax to advanced programming concepts.
The course structure usually begins with an introduction to Python's basic syntax and programming concepts. It then progressively moves into more complex topics, such as data structures, file operations, error and exception handling, and object-oriented programming principles. Participants also get to work on real-life projects, which is vital for understanding how Python can be applied in practical scenarios.
A significant advantage of online courses like the one offered by Softs Solution Service is their accessibility. Students can learn at their own pace, with access to a wealth of resources and support from experienced instructors. Additionally, these courses often provide community support, where learners can interact with peers, share knowledge, and collaborate on projects.
Python's future seems bright as it continues to evolve with new features and enhancements. Its growing popularity in various fields, including web development, data analytics, artificial intelligence, and scientific research, ensures that Python developers will remain in high demand.
In summary, Python is not just a programming language; it's a tool that opens a world of possibilities for developers, data scientists, and tech enthusiasts. With resources like the Online Python Development Course from Softs Solution Service, mastering Python has become more accessible than ever, promising exciting opportunities in the ever-evolving world of technology.
#IT Training and Internship#Softs Solution Service#IT Training Institute in Ahmedabad#Online Python Development Course#Python Development Training#Python Development Course
3 notes
·
View notes
Text
Empowering Decision-Making: Unlocking the Potential of Data Science Across Industries
In the era of information abundance, data has become a formidable asset. However, the real distinction for successful enterprises lies in their ability to derive meaningful insights from this vast sea of data. Enter data science – a field that transcends mere analysis and offers a transformative lens through which industries can innovate, optimize, and thrive. Opting for the Best Data Science Institute can further expedite your journey into this burgeoning industry. In this blog, we will delve into the diverse applications of data science across various sectors, spotlighting its pivotal role in steering informed decision-making and fostering innovation.
1. Business Intelligence and Analytics: Revealing Patterns for Growth
At the heart of data science is the ability to unravel intricate patterns within extensive datasets. For businesses, this translates into a powerful tool for business intelligence and analytics. By harnessing historical and current data, organizations can gain valuable insights into their performance, identify trends, and make informed decisions that drive growth. Whether optimizing operational processes or uncovering opportunities for expansion, data science serves as a compass for strategic decision-making.
2. Predictive Modeling: Forecasting the Future with Confidence
Predictive modeling stands out as one of the hallmark applications of data science. By analyzing historical data, organizations can develop models that forecast future trends and outcomes. This capability proves invaluable across various domains. In finance, predictive modeling aids in anticipating stock prices; in healthcare, it contributes to predicting patient outcomes. The ability to foresee potential scenarios empowers decision-makers to plan and strategize with confidence.
3. Machine Learning Applications: Infusing Intelligence into Applications
Machine learning, a subset of data science, takes analytical power a step further by enabling intelligent applications. From recommendation systems in e-commerce to fraud detection in finance and image recognition in healthcare, machine learning algorithms bring a layer of automation and adaptability to diverse domains. This not only enhances user experience but also improves the efficiency and effectiveness of various processes.
4. Healthcare and Life Sciences: Revolutionizing Patient Care and Research
In the realm of healthcare, data science acts as a catalyst for transformation. From patient diagnosis to personalized treatment plans and drug discovery, data-driven insights are revolutionizing the industry. Analyzing large datasets allows medical professionals to identify patterns, tailor treatment strategies, and accelerate medical research, ultimately leading to better patient outcomes.
5. Finance and Risk Management: Navigating Uncertainty with Data-Driven Insights
Financial institutions leverage the power of data science for risk assessment, fraud detection, and portfolio optimization. Predictive analytics aids in forecasting market trends, managing risks, and making informed investment decisions. In an industry where every decision carries significant consequences, data science provides a reliable compass for navigating uncertainties. Choosing the finest Data Science Courses in Chennai is a pivotal step in acquiring the necessary expertise for a successful career in the evolving landscape of data science.
6. Supply Chain Optimization: Enhancing Efficiency from End to End
Optimizing supply chain operations is a complex endeavor, but data science offers a clear path forward. By utilizing data to forecast demand, manage inventory effectively, and optimize logistics, organizations can achieve substantial cost savings and improve overall operational efficiency. From manufacturers to retailers, data science is reshaping how businesses approach the end-to-end supply chain process.
7. Marketing and Customer Insights: Tailoring Strategies for Success
In the realm of marketing, data science emerges as a game-changer. Analyzing customer behavior, preferences, and engagement patterns allows marketers to create targeted campaigns that resonate with their audience. The ability to derive actionable insights from data enhances customer experience, improves satisfaction, and maximizes the impact of marketing initiatives.
8. Social Media Analysis: Decoding Trends and Sentiments
The digital era has ushered in an abundance of social media data, and data science plays a crucial role in making sense of this vast landscape. By analyzing social media data, businesses can extract valuable insights into user behavior, sentiment, and trends. This information is instrumental in shaping social media strategies, engaging with the audience effectively, and managing online reputation.
9. Smart Cities and Urban Planning: Paving the Way for Sustainable Living
In the context of urban planning, data science contributes to the development of smart cities. By analyzing data from sensors, traffic cameras, and citizen feedback, urban planners can optimize city infrastructure, improve traffic flow, and enhance overall urban living. Data-driven insights play a pivotal role in creating sustainable and livable urban environments.
10. Education and Personal Development: Shaping the Future of Learning
Data science is making significant inroads into education, where it is utilized to analyze student performance, tailor learning materials, and provide personalized recommendations. This not only enhances the learning experience for students but also facilitates adaptive learning platforms. The application of data science in education is reshaping how we approach teaching and learning, with a focus on individualized and effective educational experiences.
As we delve deeper into the era of big data, data science stands as a beacon of innovation and progress. Its applications span across industries, touching every facet of modern life. From healthcare and finance to education and urban planning, data science is shaping the way we make decisions, solve problems, and envision the future. Embracing the power of data science is not just a choice; it's a necessity for those looking to thrive in a data-driven world.
3 notes
·
View notes
Text
The Significance of Exploratory Data Analysis (EDA) in the Data Analysis Workflow
In the ever-expanding realm of data science, Exploratory Data Analysis (EDA) stands as a cornerstone in the data analysis workflow. It is the preliminary step that sets the stage for more complex analyses, helping analysts and data scientists unravel the mysteries hidden within datasets. In this article, we will delve into the significance of EDA and how it plays a pivotal role in understanding, cleaning, and preparing data for further analysis.
Introduction to Exploratory Data Analysis (EDA)
Exploratory Data Analysis is the process of visually and statistically summarizing, interpreting, and understanding the main characteristics of a dataset. It involves techniques that allow analysts to gain insights into the data's distribution, relationships, and patterns, aiding in the formulation of hypotheses and guiding subsequent analysis.
Data Quality Assessment
One of the primary functions of EDA is to assess the quality of the data. Analysts scrutinize the dataset for missing values, outliers, and inconsistencies. Identifying and addressing these issues early in the analysis process is crucial, as they can significantly impact the accuracy and reliability of subsequent analyses.
Identification of Patterns and Trends
EDA provides a lens through which analysts can identify patterns and trends within the data. Visualizations such as scatter plots, line charts, and histograms offer a comprehensive view of the data distribution and can reveal underlying structures that might not be immediately apparent. Recognizing these patterns can guide the formulation of hypotheses and influence the choice of analytical techniques.
Variable Relationships and Correlation
Understanding the relationships between variables is essential in many analyses. EDA tools enable analysts to explore the correlation between different variables, helping to uncover dependencies and interactions. This insight is invaluable when designing predictive models or making data-driven decisions based on correlated factors.
Outlier Detection and Handling
Outliers, data points significantly different from the rest, can distort analysis results. EDA helps identify outliers, enabling analysts to decide whether to exclude, transform, or investigate these data points further. Addressing outliers at the EDA stage ensures a cleaner dataset for subsequent analyses.
Feature Engineering Guidance
Feature engineering involves transforming raw data into a format suitable for machine learning models. EDA aids in the identification of relevant features and potential transformations. By understanding the distribution and characteristics of variables, analysts can make informed decisions about which features to include, exclude, or engineer for optimal model performance.
Data Visualization for Communication
EDA often involves creating visualizations that not only assist in understanding the data but also serve as powerful communication tools. Visual representations make it easier to convey complex information to stakeholders, facilitating better decision-making. From bar charts to heatmaps, the variety of visualizations generated during EDA enhances the interpretability of the data.
Hypothesis Generation and Testing
EDA serves as a fertile ground for hypothesis generation. Analysts, armed with a deeper understanding of the data, can formulate hypotheses about relationships and trends. Subsequent statistical tests can then be conducted to validate or refute these hypotheses, guiding the direction of more advanced analyses.
Enhancing Model Selection
The insights gained from EDA play a pivotal role in selecting the most appropriate modeling techniques. By understanding the distribution of the target variable and the relationships between features, analysts can make informed decisions about which models are likely to perform well on the given dataset. This ensures that computational resources are allocated efficiently, leading to more accurate and interpretable models.
Time and Resource Efficiency
Lastly, EDA contributes to time and resource efficiency in the data analysis workflow. By thoroughly exploring and understanding the dataset upfront, analysts can avoid unnecessary detours and focus on the most promising avenues of analysis. This iterative and interactive process helps streamline the overall analysis, making the entire workflow more efficient and effective.
Conclusion
Exploratory Data Analysis is not merely a preliminary step in the data analysis workflow; it is a fundamental and integral part of the entire process. From ensuring data quality to guiding feature engineering and model selection, EDA serves as the compass that steers analysts through the intricate landscape of data. Embracing the significance of EDA not only facilitates a deeper understanding of the data but also sets the stage for more robust, accurate, and actionable insights in the dynamic field of data science.
2 notes
·
View notes