#data warehousing solution
Explore tagged Tumblr posts
Text
#data warehousing consulting services and solutions#data warehousing solution#data warehousing solutions#data warehousing solutions for digital agencies
0 notes
Text
Deep Learning Solutions for Real-World Applications: Trends and Insights
Deep learning is revolutionizing industries by enabling machines to process and analyze vast amounts of data with unprecedented accuracy. As AI-powered solutions continue to advance, deep learning is being widely adopted across various sectors, including healthcare, finance, manufacturing, and retail. This article explores the latest trends in deep learning, its real-world applications, and key insights into its transformative potential.
Understanding Deep Learning in Real-World Applications
Deep learning, a subset of machine learning, utilizes artificial neural networks (ANNs) to mimic human cognitive processes. These networks learn from large datasets, enabling AI systems to recognize patterns, make predictions, and automate complex tasks.
The adoption of deep learning is driven by its ability to:
Process unstructured data such as images, text, and speech.
Improve accuracy with more data and computational power.
Adapt to real-world challenges with minimal human intervention.
With these capabilities, deep learning is shaping the future of AI across industries.
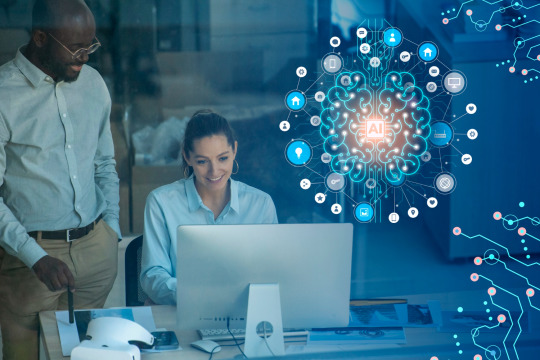
Key Trends in Deep Learning Solutions
1. AI-Powered Automation
Deep learning is driving automation by enabling machines to perform tasks that traditionally required human intelligence. Industries are leveraging AI to optimize workflows, reduce operational costs, and improve efficiency.
Manufacturing: AI-driven robots are enhancing production lines with automated quality inspection.
Customer Service: AI chatbots and virtual assistants are improving customer engagement.
Healthcare: AI automates medical imaging analysis for faster diagnosis.
2. Edge AI and On-Device Processing
Deep learning models are increasingly deployed on edge devices, reducing dependence on cloud computing. This trend enhances:
Real-time decision-making in autonomous systems.
Faster processing in mobile applications and IoT devices.
Privacy and security by keeping data local.
3. Explainable AI (XAI)
As deep learning solutions become integral to critical applications like finance and healthcare, explainability and transparency are essential. Researchers are developing Explainable AI (XAI) techniques to make deep learning models more interpretable, ensuring fairness and trustworthiness.
4. Generative AI and Creative Applications
Generative AI models, such as GPT (text generation) and DALL·E (image synthesis), are transforming creative fields. Businesses are leveraging AI for:
Content creation (automated writing and design).
Marketing and advertising (personalized campaigns).
Music and video generation (AI-assisted production).
5. Self-Supervised and Few-Shot Learning
AI models traditionally require massive datasets for training. Self-supervised learning and few-shot learning are emerging to help AI learn from limited labeled data, making deep learning solutions more accessible and efficient.
Real-World Applications of Deep Learning Solutions
1. Healthcare and Medical Diagnostics
Deep learning is transforming healthcare by enabling AI-powered diagnostics, personalized treatments, and drug discovery.
Medical Imaging: AI detects abnormalities in X-rays, MRIs, and CT scans.
Disease Prediction: AI models predict conditions like cancer and heart disease.
Telemedicine: AI chatbots assist in virtual health consultations.
2. Financial Services and Fraud Detection
Deep learning enhances risk assessment, automated trading, and fraud detection in the finance sector.
AI-Powered Fraud Detection: AI analyzes transaction patterns to prevent cyber threats.
Algorithmic Trading: Deep learning models predict stock trends with high accuracy.
Credit Scoring: AI evaluates creditworthiness based on financial behavior.
3. Retail and E-Commerce
Retailers use deep learning for customer insights, inventory optimization, and personalized shopping experiences.
AI-Based Product Recommendations: AI suggests products based on user behavior.
Automated Checkout Systems: AI-powered cameras and sensors enable cashier-less stores.
Demand Forecasting: Deep learning predicts inventory needs for efficient supply chain management.
4. Smart Manufacturing and Industrial Automation
Deep learning improves quality control, predictive maintenance, and process automation in manufacturing.
Defect Detection: AI inspects products for defects in real-time.
Predictive Maintenance: AI predicts machine failures, reducing downtime.
Robotic Process Automation (RPA): AI automates repetitive tasks in production lines.
5. Transportation and Autonomous Vehicles
Self-driving cars and smart transportation systems rely on deep learning for real-time decision-making and navigation.
Autonomous Vehicles: AI processes sensor data to detect obstacles and navigate safely.
Traffic Optimization: AI analyzes traffic patterns to improve city traffic management.
Smart Logistics: AI-powered route optimization reduces delivery costs.
6. Cybersecurity and Threat Detection
Deep learning strengthens cybersecurity defenses by detecting anomalies and preventing cyber attacks.
AI-Powered Threat Detection: Identifies suspicious activities in real time.
Biometric Authentication: AI enhances security through facial and fingerprint recognition.
Malware Detection: Deep learning models analyze patterns to identify potential cyber threats.
7. Agriculture and Precision Farming
AI-driven deep learning is improving crop monitoring, yield prediction, and pest detection.
Automated Crop Monitoring: AI analyzes satellite images to assess crop health.
Smart Irrigation Systems: AI optimizes water usage based on weather conditions.
Disease and Pest Detection: AI detects plant diseases early, reducing crop loss.
Key Insights into the Future of Deep Learning Solutions
1. AI Democratization
With the rise of open-source AI frameworks like TensorFlow and PyTorch, deep learning solutions are becoming more accessible to businesses of all sizes. This democratization of AI is accelerating innovation across industries.
2. Ethical AI Development
As AI adoption grows, concerns about bias, fairness, and privacy are increasing. Ethical AI development will focus on creating fair, transparent, and accountable deep learning solutions.
3. Human-AI Collaboration
Rather than replacing humans, deep learning solutions will enhance human capabilities by automating repetitive tasks and enabling AI-assisted decision-making.
4. AI in Edge Computing and 5G Networks
The integration of AI with edge computing and 5G will enable faster data processing, real-time analytics, and enhanced connectivity for AI-powered applications.
Conclusion
Deep learning solutions are transforming industries by enhancing automation, improving efficiency, and unlocking new possibilities in AI. From healthcare and finance to retail and cybersecurity, deep learning is solving real-world problems with remarkable accuracy and intelligence.
As technology continues to advance, businesses that leverage deep learning solutions will gain a competitive edge, driving innovation, efficiency, and smarter decision-making. The future of AI is unfolding rapidly, and deep learning remains at the heart of this transformation.
Stay ahead in the AI revolution—explore the latest trends and insights in deep learning today!
#Deep learning solutions#Big Data and Data Warehousing service#Data visualization#Predictive Analytics#Data Mining#Deep Learning
1 note
·
View note
Text
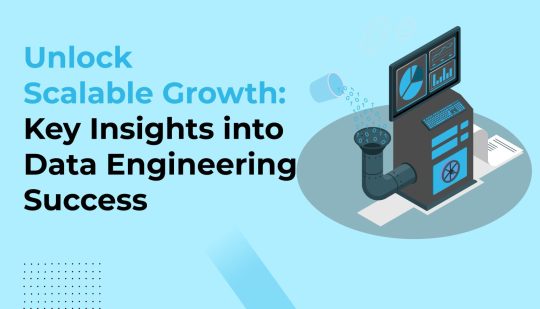
Data Engineering services have become a growing necessity as organizations struggle to manage exponentially increasing volumes of data. Efficient Data Engineering services empower companies to leverage data for strategic decision-making, ensuring they maintain a competitive edge.
By implementing the right frameworks, businesses can continuously innovate and stay ahead of industry challenges.
#data solutions#business insights#advanced analytics#data driven decisions#it services#data engineering#data warehousing
0 notes
Text
Why Your Business Needs SAP Data Analytics Consulting in 2024
Unlock the full potential of your SAP data! Explore how SAP Data Analytics Consulting can help businesses in 2024 drive better insights, optimize processes, and make data-driven decisions. Discover key strategies and benefits tailored for modern business challenges. Read more by clicking on the link
0 notes
Text
Implementing a robust Warehouse Management System (WMS) is pivotal for businesses engaged in logistics and warehouse operations. A sophisticated WMS streamlines processes and enhances logistic optimization, ensuring that goods move smoothly from suppliers to customers. This integration of technology in modern logistics transforms traditional warehousing into a seamless, efficient, and highly productive component of the supply chain.
0 notes
Text
Improve the accessibility and quality of your data with Scalable Data Warehousing Solutions. Outrank your competitors by making smarter data-driven decisions.
0 notes
Text
Our qualified and experienced Data Engineers will optimize and transform your data to help you make better business decisions.
0 notes
Text
Navigating the Future with Cloud-Based Data Warehousing: Empowering Enterprises with Atgeir Solution
Atgeir Solution pioneers modern data warehousing, empowering enterprises with expert Cloud Data Warehouse solutions. We tackle challenges such as slow analytics, unreasonable costs, and poor data pipelines, leveraging our rich experience in traditional data warehouses. Our expertise lies in seamlessly migrating traditional warehouses to the cloud, unifying data from multiple sources, improving performance, enhancing disaster recovery, and ensuring scalable and flexible solutions. Experience the ease and power of cloud-based data warehousing with Atgeir Solution!
0 notes
Text
This blog covers the latest trends in Data Warehousing, including the role of new technologies like AI, data streaming, and machine learning. We aim to help data professionals stay ahead in the field by embracing these advancements. Read this full blog.
1 note
·
View note
Text
In today’s fast-paced world of logistics innovation and evolution have become the driving forces behind success. As we step into 2024, the logistics sector will undergo extensive disruptions, fueled by game-changing innovations that promise to revolutionize supply chain management as we know it.
At iFour, we take immense pride in our expertise in the logistics industry, and we are excited to share with you the trends that are currently transforming this dynamic sector in Australia. As a leading custom Logistics software development company, we understand the unique challenges and opportunities facing businesses in the Australian market.
Here are the key trends that are reshaping the logistics landscape and how our solutions can help your company stay ahead of the curve.
#Supply Chain Technology#E-commerce Logistics#Automation and Robotics#Blockchain in Logistics#Sustainable and Green Logistics#Last-Mile Delivery Solutions#Data Analytics and Predictive Insights#3D Printing in Logistics#IoT (Internet of Things) in Supply Chain#Artificial Intelligence in Transportation#On-Demand and Sharing Economy Logistics#Digital Twins in Warehousing#Autonomous Vehicles and Drones#Climate Change and Resilience Planning#Cross-Border Trade and Customs#Cybersecurity in Supply Chain#Inventory Management Strategies#Reverse Logistics and Returns#Multi-Modal Transportation#software outsourcing#software development company#.net development
0 notes
Text
Google Cloud’s BigQuery Autonomous Data To AI Platform

BigQuery automates data analysis, transformation, and insight generation using AI. AI and natural language interaction simplify difficult operations.
The fast-paced world needs data access and a real-time data activation flywheel. Artificial intelligence that integrates directly into the data environment and works with intelligent agents is emerging. These catalysts open doors and enable self-directed, rapid action, which is vital for success. This flywheel uses Google's Data & AI Cloud to activate data in real time. BigQuery has five times more organisations than the two leading cloud providers that just offer data science and data warehousing solutions due to this emphasis.
Examples of top companies:
With BigQuery, Radisson Hotel Group enhanced campaign productivity by 50% and revenue by over 20% by fine-tuning the Gemini model.
By connecting over 170 data sources with BigQuery, Gordon Food Service established a scalable, modern, AI-ready data architecture. This improved real-time response to critical business demands, enabled complete analytics, boosted client usage of their ordering systems, and offered staff rapid insights while cutting costs and boosting market share.
J.B. Hunt is revolutionising logistics for shippers and carriers by integrating Databricks into BigQuery.
General Mills saves over $100 million using BigQuery and Vertex AI to give workers secure access to LLMs for structured and unstructured data searches.
Google Cloud is unveiling many new features with its autonomous data to AI platform powered by BigQuery and Looker, a unified, trustworthy, and conversational BI platform:
New assistive and agentic experiences based on your trusted data and available through BigQuery and Looker will make data scientists, data engineers, analysts, and business users' jobs simpler and faster.
Advanced analytics and data science acceleration: Along with seamless integration with real-time and open-source technologies, BigQuery AI-assisted notebooks improve data science workflows and BigQuery AI Query Engine provides fresh insights.
Autonomous data foundation: BigQuery can collect, manage, and orchestrate any data with its new autonomous features, which include native support for unstructured data processing and open data formats like Iceberg.
Look at each change in detail.
User-specific agents
It believes everyone should have AI. BigQuery and Looker made AI-powered helpful experiences generally available, but Google Cloud now offers specialised agents for all data chores, such as:
Data engineering agents integrated with BigQuery pipelines help create data pipelines, convert and enhance data, discover anomalies, and automate metadata development. These agents provide trustworthy data and replace time-consuming and repetitive tasks, enhancing data team productivity. Data engineers traditionally spend hours cleaning, processing, and confirming data.
The data science agent in Google's Colab notebook enables model development at every step. Scalable training, intelligent model selection, automated feature engineering, and faster iteration are possible. This agent lets data science teams focus on complex methods rather than data and infrastructure.
Looker conversational analytics lets everyone utilise natural language with data. Expanded capabilities provided with DeepMind let all users understand the agent's actions and easily resolve misconceptions by undertaking advanced analysis and explaining its logic. Looker's semantic layer boosts accuracy by two-thirds. The agent understands business language like “revenue” and “segments” and can compute metrics in real time, ensuring trustworthy, accurate, and relevant results. An API for conversational analytics is also being introduced to help developers integrate it into processes and apps.
In the BigQuery autonomous data to AI platform, Google Cloud introduced the BigQuery knowledge engine to power assistive and agentic experiences. It models data associations, suggests business vocabulary words, and creates metadata instantaneously using Gemini's table descriptions, query histories, and schema connections. This knowledge engine grounds AI and agents in business context, enabling semantic search across BigQuery and AI-powered data insights.
All customers may access Gemini-powered agentic and assistive experiences in BigQuery and Looker without add-ons in the existing price model tiers!
Accelerating data science and advanced analytics
BigQuery autonomous data to AI platform is revolutionising data science and analytics by enabling new AI-driven data science experiences and engines to manage complex data and provide real-time analytics.
First, AI improves BigQuery notebooks. It adds intelligent SQL cells to your notebook that can merge data sources, comprehend data context, and make code-writing suggestions. It also uses native exploratory analysis and visualisation capabilities for data exploration and peer collaboration. Data scientists can also schedule analyses and update insights. Google Cloud also lets you construct laptop-driven, dynamic, user-friendly, interactive data apps to share insights across the organisation.
This enhanced notebook experience is complemented by the BigQuery AI query engine for AI-driven analytics. This engine lets data scientists easily manage organised and unstructured data and add real-world context—not simply retrieve it. BigQuery AI co-processes SQL and Gemini, adding runtime verbal comprehension, reasoning skills, and real-world knowledge. Their new engine processes unstructured photographs and matches them to your product catalogue. This engine supports several use cases, including model enhancement, sophisticated segmentation, and new insights.
Additionally, it provides users with the most cloud-optimized open-source environment. Google Cloud for Apache Kafka enables real-time data pipelines for event sourcing, model scoring, communications, and analytics in BigQuery for serverless Apache Spark execution. Customers have almost doubled their serverless Spark use in the last year, and Google Cloud has upgraded this engine to handle data 2.7 times faster.
BigQuery lets data scientists utilise SQL, Spark, or foundation models on Google's serverless and scalable architecture to innovate faster without the challenges of traditional infrastructure.
An independent data foundation throughout data lifetime
An independent data foundation created for modern data complexity supports its advanced analytics engines and specialised agents. BigQuery is transforming the environment by making unstructured data first-class citizens. New platform features, such as orchestration for a variety of data workloads, autonomous and invisible governance, and open formats for flexibility, ensure that your data is always ready for data science or artificial intelligence issues. It does this while giving the best cost and decreasing operational overhead.
For many companies, unstructured data is their biggest untapped potential. Even while structured data provides analytical avenues, unique ideas in text, audio, video, and photographs are often underutilised and discovered in siloed systems. BigQuery instantly tackles this issue by making unstructured data a first-class citizen using multimodal tables (preview), which integrate structured data with rich, complex data types for unified querying and storage.
Google Cloud's expanded BigQuery governance enables data stewards and professionals a single perspective to manage discovery, classification, curation, quality, usage, and sharing, including automatic cataloguing and metadata production, to efficiently manage this large data estate. BigQuery continuous queries use SQL to analyse and act on streaming data regardless of format, ensuring timely insights from all your data streams.
Customers utilise Google's AI models in BigQuery for multimodal analysis 16 times more than last year, driven by advanced support for structured and unstructured multimodal data. BigQuery with Vertex AI are 8–16 times cheaper than independent data warehouse and AI solutions.
Google Cloud maintains open ecology. BigQuery tables for Apache Iceberg combine BigQuery's performance and integrated capabilities with the flexibility of an open data lakehouse to link Iceberg data to SQL, Spark, AI, and third-party engines in an open and interoperable fashion. This service provides adaptive and autonomous table management, high-performance streaming, auto-AI-generated insights, practically infinite serverless scalability, and improved governance. Cloud storage enables fail-safe features and centralised fine-grained access control management in their managed solution.
Finaly, AI platform autonomous data optimises. Scaling resources, managing workloads, and ensuring cost-effectiveness are its competencies. The new BigQuery spend commit unifies spending throughout BigQuery platform and allows flexibility in shifting spend across streaming, governance, data processing engines, and more, making purchase easier.
Start your data and AI adventure with BigQuery data migration. Google Cloud wants to know how you innovate with data.
#technology#technews#govindhtech#news#technologynews#BigQuery autonomous data to AI platform#BigQuery#autonomous data to AI platform#BigQuery platform#autonomous data#BigQuery AI Query Engine
2 notes
·
View notes
Text
Data warehousing solution
Unlocking the Power of Data Warehousing: A Key to Smarter Decision-Making
In today's data-driven world, businesses need to make smarter, faster, and more informed decisions. But how can companies achieve this? One powerful tool that plays a crucial role in managing vast amounts of data is data warehousing. In this blog, we’ll explore what data warehousing is, its benefits, and how it can help organizations make better business decisions.
What is Data Warehousing?
At its core, data warehousing refers to the process of collecting, storing, and managing large volumes of data from different sources in a central repository. The data warehouse serves as a consolidated platform where all organizational data—whether from internal systems, third-party applications, or external sources—can be stored, processed, and analyzed.
A data warehouse is designed to support query and analysis operations, making it easier to generate business intelligence (BI) reports, perform complex data analysis, and derive insights for better decision-making. Data warehouses are typically used for historical data analysis, as they store data from multiple time periods to identify trends, patterns, and changes over time.
Key Components of a Data Warehouse
To understand the full functionality of a data warehouse, it's helpful to know its primary components:
Data Sources: These are the various systems and platforms where data is generated, such as transactional databases, CRM systems, or external data feeds.
ETL (Extract, Transform, Load): This is the process by which data is extracted from different sources, transformed into a consistent format, and loaded into the warehouse.
Data Warehouse Storage: The central repository where cleaned, structured data is stored. This can be in the form of a relational database or a cloud-based storage system, depending on the organization’s needs.
OLAP (Online Analytical Processing): This allows for complex querying and analysis, enabling users to create multidimensional data models, perform ad-hoc queries, and generate reports.
BI Tools and Dashboards: These tools provide the interfaces that enable users to interact with the data warehouse, such as through reports, dashboards, and data visualizations.
Benefits of Data Warehousing
Improved Decision-Making: With data stored in a single, organized location, businesses can make decisions based on accurate, up-to-date, and complete information. Real-time analytics and reporting capabilities ensure that business leaders can take swift action.
Consolidation of Data: Instead of sifting through multiple databases or systems, employees can access all relevant data from one location. This eliminates redundancy and reduces the complexity of managing data from various departments or sources.
Historical Analysis: Data warehouses typically store historical data, making it possible to analyze long-term trends and patterns. This helps businesses understand customer behavior, market fluctuations, and performance over time.
Better Reporting: By using BI tools integrated with the data warehouse, businesses can generate accurate reports on key metrics. This is crucial for monitoring performance, tracking KPIs (Key Performance Indicators), and improving strategic planning.
Scalability: As businesses grow, so does the volume of data they collect. Data warehouses are designed to scale easily, handling increasing data loads without compromising performance.
Enhanced Data Quality: Through the ETL process, data is cleaned, transformed, and standardized. This means the data stored in the warehouse is of high quality—consistent, accurate, and free of errors.
Types of Data Warehouses
There are different types of data warehouses, depending on how they are set up and utilized:
Enterprise Data Warehouse (EDW): An EDW is a central data repository for an entire organization, allowing access to data from all departments or business units.
Operational Data Store (ODS): This is a type of data warehouse that is used for storing real-time transactional data for short-term reporting. An ODS typically holds data that is updated frequently.
Data Mart: A data mart is a subset of a data warehouse focused on a specific department, business unit, or subject. For example, a marketing data mart might contain data relevant to marketing operations.
Cloud Data Warehouse: With the rise of cloud computing, cloud-based data warehouses like Google BigQuery, Amazon Redshift, and Snowflake have become increasingly popular. These platforms allow businesses to scale their data infrastructure without investing in physical hardware.
How Data Warehousing Drives Business Intelligence
The purpose of a data warehouse is not just to store data, but to enable businesses to extract valuable insights. By organizing and analyzing data, businesses can uncover trends, customer preferences, and operational inefficiencies. Some of the ways in which data warehousing supports business intelligence include:
Customer Segmentation: Companies can analyze data to segment customers based on behavior, demographics, or purchasing patterns, leading to better-targeted marketing efforts.
Predictive Analytics: By analyzing historical data, businesses can forecast trends and predict future outcomes, such as sales, inventory needs, and staffing levels.
Improved Operational Efficiency: With data-driven insights, businesses can streamline processes, optimize supply chains, and reduce costs. For example, identifying inventory shortages or surplus can help optimize stock levels.
Challenges in Data Warehousing
While the benefits of data warehousing are clear, there are some challenges to consider:
Complexity of Implementation: Setting up a data warehouse can be a complex and time-consuming process, requiring expertise in database management, ETL processes, and BI tools.
Data Integration: Integrating data from various sources with differing formats can be challenging, especially when dealing with legacy systems or unstructured data.
Cost: Building and maintaining a data warehouse can be expensive, particularly when managing large volumes of data. However, the investment is often worth it in terms of the business value generated.
Security: With the consolidation of sensitive data in one place, data security becomes critical. Organizations need robust security measures to prevent unauthorized access and ensure compliance with data protection regulations.
The Future of Data Warehousing
The world of data warehousing is constantly evolving. With advancements in cloud technology, machine learning, and artificial intelligence, businesses are now able to handle larger datasets, perform more sophisticated analyses, and automate key processes.
As companies increasingly embrace the concept of a "data-driven culture," the need for powerful data warehousing solutions will continue to grow. The integration of AI-driven analytics, real-time data processing, and more intuitive BI tools will only further enhance the value of data warehouses in the years to come.
Conclusion
In today’s fast-paced, data-centric world, having access to accurate, high-quality data is crucial for making informed business decisions. A robust data warehousing solution enables businesses to consolidate, analyze, and extract valuable insights from their data, driving smarter decision-making across all departments. While building a data warehouse comes with challenges, the benefits—improved efficiency, better decision-making, and enhanced business intelligence—make it an essential tool for modern organizations.
You said:
blog for Data warehousing
ChatGPT said:
Title: Data Warehousing: The Backbone of Data-Driven Decision Making
In today’s fast-paced business environment, the ability to make data-driven decisions quickly is paramount. However, to leverage data effectively, companies need more than just raw data. They need a centralized, structured system that allows them to store, manage, and analyze data seamlessly. This is where data warehousing comes into play.
Data warehousing has become the cornerstone of modern business intelligence (BI) systems, enabling organizations to unlock valuable insights from vast amounts of data. In this blog, we’ll explore what data warehousing is, why it’s important, and how it drives smarter decision-making.
What is Data Warehousing?
At its core, data warehousing refers to the process of collecting and storing data from various sources into a centralized system where it can be easily accessed and analyzed. Unlike traditional databases, which are optimized for transactional operations (i.e., data entry, updating), data warehouses are designed specifically for complex queries, reporting, and data analysis.
A data warehouse consolidates data from various sources—such as customer information systems, financial systems, and even external data feeds—into a single repository. The data is then structured and organized in a way that supports business intelligence (BI) tools, enabling organizations to generate reports, create dashboards, and gain actionable insights.
Key Components of a Data Warehouse
Data Sources: These are the different systems or applications that generate data. Examples include CRM systems, ERP systems, external APIs, and transactional databases.
ETL (Extract, Transform, Load): This is the process by which data is pulled from different sources (Extract), cleaned and converted into a usable format (Transform), and finally loaded into the data warehouse (Load).
Data Warehouse Storage: The actual repository where structured and organized data is stored. This could be in traditional relational databases or modern cloud-based storage platforms.
OLAP (Online Analytical Processing): OLAP tools enable users to run complex analytical queries on the data warehouse, creating reports, performing multidimensional analysis, and identifying trends.
Business Intelligence Tools: These tools are used to interact with the data warehouse, generate reports, visualize data, and help businesses make data-driven decisions.
Benefits of Data Warehousing
Improved Decision Making: By consolidating data into a single repository, decision-makers can access accurate, up-to-date information whenever they need it. This leads to more informed, faster decisions based on reliable data.
Data Consolidation: Instead of pulling data from multiple systems and trying to make sense of it, a data warehouse consolidates data from various sources into one place, eliminating the complexity of handling scattered information.
Historical Analysis: Data warehouses are typically designed to store large amounts of historical data. This allows businesses to analyze trends over time, providing valuable insights into long-term performance and market changes.
Increased Efficiency: With a data warehouse in place, organizations can automate their reporting and analytics processes. This means less time spent manually gathering data and more time focusing on analyzing it for actionable insights.
Better Reporting and Insights: By using data from a single, trusted source, businesses can produce consistent, accurate reports that reflect the true state of affairs. BI tools can transform raw data into meaningful visualizations, making it easier to understand complex trends.
Types of Data Warehouses
Enterprise Data Warehouse (EDW): This is a centralized data warehouse that consolidates data across the entire organization. It’s used for comprehensive, organization-wide analysis and reporting.
Data Mart: A data mart is a subset of a data warehouse that focuses on specific business functions or departments. For example, a marketing data mart might contain only marketing-related data, making it easier for the marketing team to access relevant insights.
Operational Data Store (ODS): An ODS is a database that stores real-time data and is designed to support day-to-day operations. While a data warehouse is optimized for historical analysis, an ODS is used for operational reporting.
Cloud Data Warehouse: With the rise of cloud computing, cloud-based data warehouses like Amazon Redshift, Google BigQuery, and Snowflake have become popular. These solutions offer scalable, cost-effective, and flexible alternatives to traditional on-premises data warehouses.
How Data Warehousing Supports Business Intelligence
A data warehouse acts as the foundation for business intelligence (BI) systems. BI tools, such as Tableau, Power BI, and QlikView, connect directly to the data warehouse, enabling users to query the data and generate insightful reports and visualizations.
For example, an e-commerce company can use its data warehouse to analyze customer behavior, sales trends, and inventory performance. The insights gathered from this analysis can inform marketing campaigns, pricing strategies, and inventory management decisions.
Here are some ways data warehousing drives BI and decision-making:
Customer Insights: By analyzing customer purchase patterns, organizations can better segment their audience and personalize marketing efforts.
Trend Analysis: Historical data allows companies to identify emerging trends, such as seasonal changes in demand or shifts in customer preferences.
Predictive Analytics: By leveraging machine learning models and historical data stored in the data warehouse, companies can forecast future trends, such as sales performance, product demand, and market behavior.
Operational Efficiency: A data warehouse can help identify inefficiencies in business operations, such as bottlenecks in supply chains or underperforming products.
2 notes
·
View notes
Text
Why Modernizing Data Warehousing Solutions is Significance for Today’s Business
Unlock efficiency and real-time insights with GrayMatter's modern data warehousing solutions. Our expert approach transforms data processes, empowering smarter decisions and giving your business a competitive edge in today’s data-driven world. Read the blog to learn more.
0 notes
Text
Empowering Businesses with Comprehensive Data Analytics Services

In today’s digital landscape, the significance of data-driven decision-making cannot be overstated. As organizations grapple with an overwhelming influx of data, the ability to harness, manage, and analyze this information effectively is key to gaining a competitive advantage. **Data analytics services** have emerged as essential tools that enable companies to transform raw data into valuable insights, driving strategic growth and operational efficiency.
The Role of Data Management in Modern Enterprises
Effective data management lies at the heart of any successful data analytics strategy. It involves systematically organizing, storing, and protecting data to ensure its quality and accessibility. A robust data management framework not only supports data compliance but also allows for the seamless integration of data from various sources, making it possible for businesses to have a unified view of their operations. This foundational layer is critical for maximizing the potential of data analytics services.
Turning Raw Data into Strategic Insights
Raw data, on its own, has little value unless it is processed and analyzed to reveal trends and patterns that can inform business strategies. Data analytics services help organizations unlock the true value of their data by converting it into actionable insights. These services leverage sophisticated techniques such as predictive analytics, data mining, and statistical modeling to deliver deeper insights into customer behavior, market trends, and operational efficiencies.
By employing data analytics, companies can optimize their decision-making processes, anticipate market changes, and enhance their products or services based on customer needs and feedback. This approach ensures that businesses stay ahead of the curve in an ever-evolving market landscape.
Driving Innovation Through Advanced Analytics
Data analytics services are not only about analyzing past performance; they are also instrumental in shaping the future. By integrating advanced analytics techniques, such as machine learning and artificial intelligence, businesses can identify emerging patterns and predict future outcomes with higher accuracy. This predictive capability enables organizations to mitigate risks, identify new revenue streams, and innovate more effectively.
The integration of real-time analytics further enhances a company’s ability to respond promptly to changing market conditions. It empowers decision-makers to take immediate actions based on the latest data insights, ensuring agility and resilience in dynamic environments.
Implementing a Data-Driven Culture
For businesses to truly benefit from data analytics services, it is crucial to foster a data-driven culture. This involves training teams to understand the value of data and encouraging data-centric decision-making across all levels of the organization. A culture that prioritizes data-driven insights helps break down silos, promotes transparency, and supports continuous improvement.
Organizations that embrace a data-driven mindset are better positioned to leverage analytics to drive strategic growth and deliver superior customer experiences.
Partnering for Success
Choosing the right partner for data management and analytics is vital. Companies like SG Analytics offer a range of tailored solutions designed to help organizations manage their data more effectively and gain valuable insights. From data warehousing and data integration to advanced analytics, these services provide end-to-end support, ensuring businesses can make the most of their data assets.
By leveraging data analytics services from experienced partners, companies can focus on their core objectives while simultaneously evolving into data-driven enterprises that thrive in the digital age.
Conclusion
In the era of big data, the ability to transform information into insights is a crucial differentiator. With comprehensive data analytics services, businesses can harness the power of their data to drive informed decisions, innovate, and maintain a competitive edge. The key lies in effective data management, advanced analytics, and fostering a culture that values data-driven insights.
2 notes
·
View notes
Text
AWS Security 101: Protecting Your Cloud Investments
In the ever-evolving landscape of technology, few names resonate as strongly as Amazon.com. This global giant, known for its e-commerce prowess, has a lesser-known but equally influential arm: Amazon Web Services (AWS). AWS is a powerhouse in the world of cloud computing, offering a vast and sophisticated array of services and products. In this comprehensive guide, we'll embark on a journey to explore the facets and features of AWS that make it a driving force for individuals, companies, and organizations seeking to utilise cloud computing to its fullest capacity.
Amazon Web Services (AWS): A Technological Titan
At its core, AWS is a cloud computing platform that empowers users to create, deploy, and manage applications and infrastructure with unparalleled scalability, flexibility, and cost-effectiveness. It's not just a platform; it's a digital transformation enabler. Let's dive deeper into some of the key components and features that define AWS:
1. Compute Services: The Heart of Scalability
AWS boasts services like Amazon EC2 (Elastic Compute Cloud), a scalable virtual server solution, and AWS Lambda for serverless computing. These services provide users with the capability to efficiently run applications and workloads with precision and ease. Whether you need to host a simple website or power a complex data-processing application, AWS's compute services have you covered.
2. Storage Services: Your Data's Secure Haven
In the age of data, storage is paramount. AWS offers a diverse set of storage options. Amazon S3 (Simple Storage Service) caters to scalable object storage needs, while Amazon EBS (Elastic Block Store) is ideal for block storage requirements. For archival purposes, Amazon Glacier is the go-to solution. This comprehensive array of storage choices ensures that diverse storage needs are met, and your data is stored securely.
3. Database Services: Managing Complexity with Ease
AWS provides managed database services that simplify the complexity of database management. Amazon RDS (Relational Database Service) is perfect for relational databases, while Amazon DynamoDB offers a seamless solution for NoSQL databases. Amazon Redshift, on the other hand, caters to data warehousing needs. These services take the headache out of database administration, allowing you to focus on innovation.
4. Networking Services: Building Strong Connections
Network isolation and robust networking capabilities are made easy with Amazon VPC (Virtual Private Cloud). AWS Direct Connect facilitates dedicated network connections, and Amazon Route 53 takes care of DNS services, ensuring that your network needs are comprehensively addressed. In an era where connectivity is king, AWS's networking services rule the realm.
5. Security and Identity: Fortifying the Digital Fortress
In a world where data security is non-negotiable, AWS prioritizes security with services like AWS IAM (Identity and Access Management) for access control and AWS KMS (Key Management Service) for encryption key management. Your data remains fortified, and access is strictly controlled, giving you peace of mind in the digital age.
6. Analytics and Machine Learning: Unleashing the Power of Data
In the era of big data and machine learning, AWS is at the forefront. Services like Amazon EMR (Elastic MapReduce) handle big data processing, while Amazon SageMaker provides the tools for developing and training machine learning models. Your data becomes a strategic asset, and innovation knows no bounds.
7. Application Integration: Seamlessness in Action
AWS fosters seamless application integration with services like Amazon SQS (Simple Queue Service) for message queuing and Amazon SNS (Simple Notification Service) for event-driven communication. Your applications work together harmoniously, creating a cohesive digital ecosystem.
8. Developer Tools: Powering Innovation
AWS equips developers with a suite of powerful tools, including AWS CodeDeploy, AWS CodeCommit, and AWS CodeBuild. These tools simplify software development and deployment processes, allowing your teams to focus on innovation and productivity.
9. Management and Monitoring: Streamlined Resource Control
Effective resource management and monitoring are facilitated by AWS CloudWatch for monitoring and AWS CloudFormation for infrastructure as code (IaC) management. Managing your cloud resources becomes a streamlined and efficient process, reducing operational overhead.
10. Global Reach: Empowering Global Presence
With data centers, known as Availability Zones, scattered across multiple regions worldwide, AWS enables users to deploy applications close to end-users. This results in optimal performance and latency, crucial for global digital operations.
In conclusion, Amazon Web Services (AWS) is not just a cloud computing platform; it's a technological titan that empowers organizations and individuals to harness the full potential of cloud computing. Whether you're an aspiring IT professional looking to build a career in the cloud or a seasoned expert seeking to sharpen your skills, understanding AWS is paramount.
In today's technology-driven landscape, AWS expertise opens doors to endless opportunities. At ACTE Institute, we recognize the transformative power of AWS, and we offer comprehensive training programs to help individuals and organizations master the AWS platform. We are your trusted partner on the journey of continuous learning and professional growth. Embrace AWS, embark on a path of limitless possibilities in the world of technology, and let ACTE Institute be your guiding light. Your potential awaits, and together, we can reach new heights in the ever-evolving world of cloud computing. Welcome to the AWS Advantage, and let's explore the boundless horizons of technology together!
8 notes
·
View notes
Text
Learn how AI-enabled data warehouses can enhance your analytics capabilities. This article explores the benefits of automated data integration, optimization and insights for better business decision making.
0 notes