#datapipelines
Explore tagged Tumblr posts
Text
#DataEngineering#PipelineMonitoring#AzureDataFactory#DataPipelines#DataManagement#Automation#TechTips
0 notes
Text
Medallion Architecture: A Scalable Framework for Modern Data Management

In the current big data era, companies must effectively manage data to make data-driven decisions. One such well-known data management architecture is the Medallion Architecture. This architecture offers a structured, scalable, modular approach to building data pipelines, ensuring data quality, and optimizing data operations.
What is Medallion Architecture?
Medallion Architecture is a system for managing and organizing data in stages. Each stage, or “medallion,” improves the quality and usefulness of the data, step by step. The main goal is to transform raw data into meaningful data that is ready for the analysis team.
The Three Layers of Medallion Architecture:
Bronze Layer (Raw Data):This layer stores all raw data exactly as it comes in without any changes or cleaning, preserving a copy of the original data for fixing errors or reprocessing when needed. Example: Logs from a website, sensor data, or files uploaded by users.
Silver Layer (Cleaned and Transformed Data):The Silver Layer involves cleaning, organizing, and validating data by fixing errors such as duplicates or missing values, ensuring the data is consistent and reliable for analysis, such as removing duplicate customer records or standardizing dates in a database Example: Removing duplicate customer records or standardizing dates in a database.
Gold Layer (Business-Ready Data):The Gold Layer contains final, polished data optimized for reports, dashboards, and decision-making, providing businesses with exactly the information they need to make informed decisions Example: A table showing the total monthly sales for each region
Advantages:
Improved Data Quality: Incremental layers progressively refine data quality from raw to business-ready datasets
Scalability: Each layer can be scaled independently based on specific business requirements
Security: If you have a large team to handle, you can separate them by their level
Modularity: The layered approach separates responsibilities, simplifying management and debugging
Traceability: Raw data preserved in the Bronze layer ensures traceability and allows reprocessing when issues arise in downstream layers
Adaptability: The architecture supports diverse data sources and formats, making it suitable for various business needs
Challenges:
Takes Time: Processing through multiple layers can delay results
Storage Costs: Storing raw and processed data requires more space
Requires Skills: Implementing this architecture requires skilled data engineers familiar with ETL/ELT tools, cloud platforms, and distributed systems
Best Practices for Medallion Architecture:
Automate ETL/ELT Processes: Use orchestration tools like Apache Airflow or AWS Step Functions to automate workflows between layers
Enforce Data Quality at Each Layer: Validate schemas, apply deduplication rules, and ensure data consistency as it transitions through layers
Monitor and Optimize Performance: Use monitoring tools to track pipeline performance and optimize transformations for scalability
Leverage Modern Tools: Adopt cloud-native technologies like Databricks, Delta Lake, or Snowflake to simplify the implementation
Plan for Governance: Implement robust data governance policies, including access control, data cataloging, and audit trails
Conclusion
Medallion Architecture is a robust framework for building reliable, scalable, and modular data pipelines. Its layered approach allows businesses to extract maximum value from their data by ensuring quality and consistency at every stage. While it comes with its challenges, the benefits of adopting Medallion Architecture often outweigh the drawbacks, making it a cornerstone for modern data engineering practices.
To learn more about this blog, please click on the link below: https://tudip.com/blog-post/medallion-architecture/.
#Tudip#MedallionArchitecture#BigData#DataPipelines#ETL#DataEngineering#CloudData#TechInnovation#DataQuality#BusinessIntelligence#DataDriven#TudipTechnologies
0 notes
Text

Data is at the core of digital transformation, driving innovation and business agility. Future-ready data engineering strategies leverage automation, AI, and cloud technologies to streamline workflows, enhance analytics capabilities, and unlock new revenue opportunities.
A structured approach ensures seamless integration, secure data governance, and high-performance processing pipelines. Scalable architectures future-proof operations, allowing businesses to adapt to evolving market demands.
With #RoundTheClockTechnologies, enterprises gain a strategic partner in navigating the complexities of modern data ecosystems, ensuring sustained growth and competitive advantage in an increasingly data-driven world.
Learn more about our data engineering services at https://rtctek.com/data-engineering-services/
#rtctek#roundtheclocktechnologies#data#dataengineeringservices#dataengineering#dataanalytics#datapipelines#dataworkflows#dataexperties
0 notes
Text
Medallion Architecture: A Scalable Framework for Modern Data Management

In the current big data era, companies must effectively manage data to make data-driven decisions. One such well-known data management architecture is the Medallion Architecture. This architecture offers a structured, scalable, modular approach to building data pipelines, ensuring data quality, and optimizing data operations.
What is Medallion Architecture?
Medallion Architecture is a system for managing and organizing data in stages. Each stage, or “medallion,” improves the quality and usefulness of the data, step by step. The main goal is to transform raw data into meaningful data that is ready for the analysis team.
The Three Layers of Medallion Architecture:
Bronze Layer (Raw Data):This layer stores all raw data exactly as it comes in without any changes or cleaning, preserving a copy of the original data for fixing errors or reprocessing when needed. Example: Logs from a website, sensor data, or files uploaded by users.
Silver Layer (Cleaned and Transformed Data):The Silver Layer involves cleaning, organizing, and validating data by fixing errors such as duplicates or missing values, ensuring the data is consistent and reliable for analysis, such as removing duplicate customer records or standardizing dates in a database Example: Removing duplicate customer records or standardizing dates in a database.
Gold Layer (Business-Ready Data):The Gold Layer contains final, polished data optimized for reports, dashboards, and decision-making, providing businesses with exactly the information they need to make informed decisions Example: A table showing the total monthly sales for each region
Advantages:
Improved Data Quality: Incremental layers progressively refine data quality from raw to business-ready datasets
Scalability: Each layer can be scaled independently based on specific business requirements
Security: If you have a large team to handle, you can separate them by their level
Modularity: The layered approach separates responsibilities, simplifying management and debugging
Traceability: Raw data preserved in the Bronze layer ensures traceability and allows reprocessing when issues arise in downstream layers
Adaptability: The architecture supports diverse data sources and formats, making it suitable for various business needs
Challenges:
Takes Time: Processing through multiple layers can delay results
Storage Costs: Storing raw and processed data requires more space
Requires Skills: Implementing this architecture requires skilled data engineers familiar with ETL/ELT tools, cloud platforms, and distributed systems
Best Practices for Medallion Architecture:
Automate ETL/ELT Processes: Use orchestration tools like Apache Airflow or AWS Step Functions to automate workflows between layers
Enforce Data Quality at Each Layer: Validate schemas, apply deduplication rules, and ensure data consistency as it transitions through layers
Monitor and Optimize Performance: Use monitoring tools to track pipeline performance and optimize transformations for scalability
Leverage Modern Tools: Adopt cloud-native technologies like Databricks, Delta Lake, or Snowflake to simplify the implementation
Plan for Governance: Implement robust data governance policies, including access control, data cataloging, and audit trails
Conclusion
Medallion Architecture is a robust framework for building reliable, scalable, and modular data pipelines. Its layered approach allows businesses to extract maximum value from their data by ensuring quality and consistency at every stage. While it comes with its challenges, the benefits of adopting Medallion Architecture often outweigh the drawbacks, making it a cornerstone for modern data engineering practices.
Click the link below to learn more about Medallion Architecture:
#Tudip#MedallionArchitecture#BigData#DataPipelines#ETL#DataEngineering#CloudData TechInnovation#DataQuality#BusinessIntelligence#DataDriven#TudipTechnologies
1 note
·
View note
Text
Find the Perfect Data Pipeline for Your Business!
Not all data pipelines are created equal, and choosing the right one can make all the difference. 🚀
In our latest blog, we explore 10 types of data pipelines—batch, real-time, ETL, and more—and help you decide which one suits your business needs best. Whether you're managing complex workflows or optimizing operations, this guide has you covered!
📖 Read more

0 notes
Text
Observability data: Secret To Successful Data Integration

Data observability platforms
In the past, creating data pipelines has frequently taken precedence over thorough monitoring and alerting for data engineers. The timely and cost-effective completion of projects frequently took precedence over the long-term integrity of data. Subtle indicators like regular, inexplicable data spikes, slow performance decline, or irregular data quality are typically overlooked by data engineers.
These were perceived as singular occurrences rather than widespread problems. A larger picture becomes visible with improved Observability Data. Uncovered bottlenecks are exposed, resource allocation is optimized, data lineage gaps are found, and firefighting is eventually turned into prevention.
Data engineer
There weren’t many technologies specifically for Data observability accessible until recently. Data engineers frequently turned to creating unique monitoring solutions, which required a lot of time and resources. Although this method worked well in less complicated settings, Observability Data has become an essential part of the data engineering toolbox due to the growing complexity of contemporary data architectures and the growing dependence on data-driven decision-making.
It’s critical to recognize that things are shifting quickly in this situation. According to projections made by Gartner, “by 2026, up from less than 20% in 2024, 50% of enterprises implementing distributed data architectures will have adopted data observability tools toincrease awareness of the current status of the data landscape.”
Data observability is becoming more and more important as data becomes more crucial to company success. Data engineers are now making Observability Data a top priority and a fundamental part of their jobs due to the development of specialized tools and a rising realization of the costs associated with low-quality data.
what is data observability
The process of keeping an eye on and managing data to guarantee its availability, dependability, and quality throughout an organization’s many systems, pipelines, and processes is known as Observability Data. It gives teams a thorough insight of the condition and healthcare of the data, empowering them to see problems early and take preventative action.
Data observability vs Data quality
Dangers lurking in your data pipeline
The following indications indicate whether your data team requires a Observability Data tool:
The high frequency of inaccurate, inconsistent, or missing data can be ascribed to problems with data quality. Finding the source of the data quality problem becomes difficult, even if you can identify the problem. To help ensure data accuracy, data teams frequently need to adhere to a manual method.
Another clue could be long-term outages in data processing operations that keep happening. When data is inaccessible for protracted periods of time, it indicates problems with the reliability of the data pipeline, which undermines trust among downstream consumers and stakeholders.
Understanding data dependencies and relationships presents difficulties for data teams.
If you find yourself using a lot of manual checks and alarms and are unable to handle problems before they affect downstream systems, it may be time to look at observability tools.
The entire data integration process may become more challenging to manage if complex data processing workflows with several steps and a variety of data sources are not well managed.
Another warning flag could be trouble managing the data lifecycle in accordance with compliance guidelines and data privacy and security laws.
A Observability Data tool can greatly enhance your data engineering procedures and the general quality of your data if you’re having any of these problems. Through the provision of data pipeline visibility, anomaly detection, and proactive issue resolution, these technologies can assist you in developing more dependable and effective data systems.
Neglecting the indicators that suggest Observability Data is necessary might have a domino effect on an organization’s undesirable outcomes. Because certain effects are intangible, it might be difficult to accurately estimate these losses; however, they can identify important areas of potential loss.
Data inaccuracies can cause faulty business decisions, lost opportunities, and client attrition, costing money. False data can damage a company’s brand and customers’ trust in its products and services. Although they are hard to measure, the intangible effects on customer trust and reputation can have long-term effects.
Put observability first to prevent inaccurate data from derailing your efforts
Data observability gives data engineers the ability to become data stewards rather than just data movers. You are adopting a more comprehensive, strategic strategy rather than merely concentrating on the technical issues of transferring data from diverse sources into a consolidated repository. You may streamline impact management, comprehend dependencies and lineage, and maximize pipeline efficiency using observability. These advantages all contribute to improved governance, economical resource usage, and reduced expenses.
Data quality becomes a quantifiable indicator that is simple to monitor and enhance with Observability Data. It is possible to anticipate possible problems in your data pipelines and datasets before they become major ones. This methodology establishes a robust and effective data environment.
Observability becomes essential when data complexity increases because it helps engineers to create solid, dependable, and trustworthy data foundations, which ultimately speeds up time-to-value for the entire company. You may reduce these risks and increase the return on investment (ROI) of your data and AI initiatives by making investments in Observability Data.
To put it simply, data observability gives data engineers the ability to create and manage solid, dependable, and high-quality data pipelines that add value to the company.
Read more on govindhtech.com
#Observabilitydata#SuccessfulDataIntegration#datapipelines#Dataengineers#datasyste#datasets#data#DataPrivacy#DataSecurity#technology#Dataobservabilityplatforms#technews#news#govindhtech
0 notes
Text
In today's rapidly evolving digital landscape, we're witnessing a significant shift in how organizations approach data projects. As a solution architect, I've observed a growing trend: the integration of DevOps practices with Business Intelligence (BI) is quickly becoming a top priority, superseding traditional siloed data projects. Let's explore why this convergence is essential for modern solutions.
The Limitations of Siloed Data Projects
Traditionally, data teams operated in isolation, focusing solely on data collection, analysis, and reporting. While this approach had its merits, it also presented several challenges:
1. Slow time-to-insight
2. Limited scalability
3. Difficulty in adapting to changing business requirements
4. Inconsistent data across departments
5. Lack of continuous improvement processes
The DevOps and BI Synergy
By bringing DevOps principles into the BI world, we're addressing these challenges head-on. Here's why this integration is crucial from a solution architecture standpoint:
1. Agile Data Pipelines: DevOps practices enable us to build flexible, automated data pipelines that can quickly adapt to new data sources or changing business needs. This flexibility is essential in today's rapidly changing business landscape.
2. Continuous Integration and Delivery of Insights: With CI/CD practices applied to BI, we can ensure that new data models, reports, and dashboards are tested, validated, and deployed rapidly and reliably.
3. Infrastructure as Code: Treating data infrastructure as code allows for version control, easy replication of environments, and quick scaling of BI systems as data volumes grow.
4. Automated Testing and Quality Assurance: Implementing automated testing for data processes, ETL jobs, and reports significantly improves data quality and reliability of insights.
5. Monitoring and Observability: DevOps principles help in setting up comprehensive monitoring for BI systems, ensuring performance, detecting anomalies, and facilitating quick troubleshooting.
6. Collaboration and Knowledge Sharing: Breaking down silos between data scientists, analysts, and IT ops teams fosters innovation and ensures that BI solutions are both powerful and practical.
Architectural Considerations
When designing solutions that integrate DevOps and BI, consider the following:
1. Modular Architecture: Design your BI system with loosely coupled components that can be independently developed, tested, and deployed.
2. API-First Approach: Implement APIs for data access and integration to enable flexibility and interoperability.
3. Containerization: Use container technologies like Docker to ensure consistency across development, testing, and production environments.
4. Orchestration: Employ orchestration tools like Kubernetes to manage and scale your BI infrastructure efficiently.
5. Version Control: Implement version control for data models, ETL processes, and dashboards, not just for code.
6. Automated Data Governance: Integrate data governance checks into your CI/CD pipeline to ensure compliance and data quality.
Overcoming Challenges
While the benefits are clear, implementing DevOps in BI is not without challenges:
1. Skill Gap: Teams need to develop new competencies spanning both DevOps and BI domains.
2. Cultural Shift: Encouraging collaboration between traditionally separate teams can be difficult.
3. Tool Integration: Ensuring seamless integration between DevOps tools and BI platforms requires careful planning.
4. Data Security: With increased automation and data flow, robust security measures become even more critical.
Conclusion
As solution architects, our role is to design systems that not only meet current needs but are also adaptable to future requirements. The integration of DevOps and BI is no longer just a nice-to-have – it's becoming essential for organizations that want to remain competitive in a data-driven world.
By embracing this convergence, we can create BI solutions that are more agile, reliable, and capable of delivering timely insights. This approach not only improves the technical aspects of data management but also aligns more closely with business objectives, enabling organizations to make data-driven decisions faster and more effectively.
The future of BI lies in breaking down silos, automating processes, and fostering a culture of continuous improvement. As solution architects, it's our responsibility to lead this transformation and help our organizations harness the full potential of their data assets.
Contact Us For More Details Or Email Us @ [email protected]
#xequalto#DevOps#BusinessIntelligence#SolutionArchitect#DataIntegration#AgileData#CI_CD#DataPipelines#InfrastructureAsCode#DataTesting#DataMonitoring#DataGovernance#DataSecurity#DataCulture#DigitalTransformation#DataDriven#ContinuousImprovement#TechInnovation#DataAnalytics#DataStrategy#customerbehavior#businessgrowth#inventorymanagement#datascience#machinelearning#datadrivendecisionmaking#customerinsights
0 notes
Text
Databricks Consulting Services & Partner Solutions | Unlocking the Power of Data
As businesses increasingly rely on data-driven insights to drive their decision-making processes, tools like Databricks have emerged as vital platforms for big data analytics and machine learning. Databricks unifies data engineering, data science, and analytics under one platform, enabling businesses to process vast amounts of data with speed and efficiency. For organizations looking to fully leverage this platform, Databricks consulting services and partner solutions provide the expertise necessary to maximize its capabilities.
What is Databricks?
Databricks is a cloud-based platform built on Apache Spark, offering a unified data analytics workspace that simplifies data workflows. It allows organizations to build and deploy scalable data pipelines, collaborate on big data projects, and run machine learning models with enhanced performance.
Key Benefits of Databricks
Unified Analytics Platform: Databricks combines data engineering, data science, and business analytics into a single workspace. This allows different teams to collaborate seamlessly on data projects, reducing time-to-insight and fostering innovation.
Scalable Data Processing: Built on Apache Spark, Databricks enables businesses to process and analyze large volumes of data in real-time, allowing for the swift processing of complex datasets.
Machine Learning at Scale: Databricks comes equipped with built-in machine learning tools, empowering organizations to develop, train, and deploy models across a scalable infrastructure. This accelerates the development of AI and ML solutions.
Seamless Integration: Databricks easily integrates with cloud platforms such as Microsoft Azure, AWS, and Google Cloud, enabling businesses to work within their preferred cloud ecosystems.
Why Databricks Consulting Services are Essential
While Databricks is a powerful platform, its full potential is unlocked with the help of expert guidance. Databricks consulting services provide the necessary skills and knowledge to ensure a smooth and effective implementation, helping companies get the most out of their data infrastructure.
Here are the key benefits of working with Databricks consultants:
Tailored Implementations: Databricks consulting partners assess your current data architecture and customize the platform to suit your unique business needs. Whether you’re looking to streamline data workflows or accelerate analytics, consultants develop tailored solutions that align with your goals.
Data Engineering Expertise: Implementing Databricks requires deep knowledge of data engineering best practices. Consulting services ensure that your data pipelines are built efficiently, delivering clean, reliable data to stakeholders.
Optimized Machine Learning Workflows: Databricks consultants help businesses optimize their machine learning models, from data preparation to deployment. This reduces errors and accelerates time to market for AI-driven solutions.
End-to-End Support: From initial setup to post-deployment support, consulting services provide end-to-end guidance. This includes everything from cloud integration to data security and governance, ensuring that your Databricks environment is optimized for performance.
Training and Enablement: Beyond implementation, consultants offer training programs to upskill your internal teams. This ensures your staff can efficiently manage and expand Databricks capabilities as your business grows.
Partner Solutions for Seamless Databricks Integration
In addition to consulting services, partner solutions play a crucial role in maximizing the potential of Databricks. These solutions enhance Databricks’ functionality by providing complementary services and tools, including:
Cloud Integrations: Seamless integration with cloud providers such as AWS, Microsoft Azure, and Google Cloud helps businesses manage their data lakes with improved scalability and cost-efficiency.
Data Security: Partners provide robust security solutions that protect sensitive data and ensure compliance with industry regulations.
Advanced Analytics: Partner solutions enhance Databricks’ capabilities by integrating advanced analytics tools and AI frameworks for deeper insights and automation.
Why Choose Databricks Consulting Services?
With Databricks consulting services, businesses gain access to a wealth of expertise and resources that enable them to harness the full power of the Databricks platform. Whether it’s optimizing big data workflows, improving collaboration across teams, or accelerating machine learning initiatives, consulting partners provide the strategic guidance needed to succeed.
When choosing a Databricks consulting partner, it’s important to look for:
Proven Experience: Ensure the partner has a track record of successful Databricks implementations across multiple industries.
Technical Expertise: Consultants should have deep knowledge of Apache Spark, machine learning, and cloud platforms.
Comprehensive Services: Choose a partner that offers a full range of services, from implementation and support to training and optimization.
Conclusion
Databricks consulting services and partner solutions provide businesses with the expertise and tools needed to unlock the full potential of their data. By collaborating with skilled consultants, companies can enhance their data management processes, build scalable data solutions, and achieve actionable insights faster than ever before.
If you're ready to elevate your data strategy with Databricks consulting services, contact Feathersoft Inc Solutions today for expert guidance.
#Databricks#BigData#DataAnalytics#MachineLearning#DataEngineering#DataScience#DatabricksConsulting#ApacheSpark#CloudComputing#DataPipelines#AnalyticsSolutions#DataStrategy#CloudData#BusinessIntelligence
0 notes
Text
Introduction to Data Engineering Concepts and Tools

Introduction to Data Engineering: Concepts and Tools thoroughly grounding the fundamental principles and technologies underpinning current data infrastructure. This course teaches students how to design, develop, and maintain strong data pipelines, ensuring efficient data movement and storage. Participants acquire hands-on experience using industry-standard technologies while learning fundamental topics like ETL (Extract, Transform, Load) procedures, data warehousing, and cloud computing. The Data Engineer Course at London School of Emerging Technology (LSET)builds on this expertise through practical projects and expert-led sessions. Collaborate with peers and industry professionals to gain skills that will help shape the future of data-driven organisations.
Enrol @ https://lset.uk/ for admission.
0 notes
Text
#DataEngineers#DataEngineering#Infrastructure#DataSystems#DataAvailability#DataAccessibility#ProgrammingLanguages#Python#Java#Scala#DataPipelines#DataWorkflows#Databases#SQL#NoSQL#RelationalDatabases#DistributedDataStores#DataWarehousing#AmazonRedshift#GoogleBigQuery#Snowflake#BigDataTechnologies#Hadoop#Spark#ETLTools#ApacheNiFi#Talend#Informatica#DataModelingTools#DataIntegrationTools
0 notes
Text
What sets Konnect Insights apart from other data orchestration and analysis tools available in the market for improving customer experiences in the aviation industry?
I can highlight some general factors that may set Konnect Insights apart from other data orchestration and analysis tools available in the market for improving customer experiences in the aviation industry. Keep in mind that the competitive landscape and product offerings may have evolved since my last knowledge update. Here are some potential differentiators:
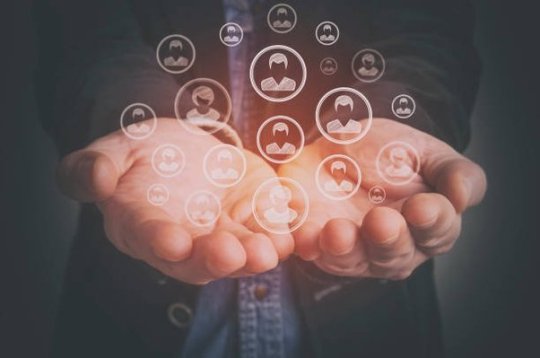
Aviation Industry Expertise: Konnect Insights may offer specialized features and expertise tailored to the unique needs and challenges of the aviation industry, including airports, airlines, and related businesses.
Multi-Channel Data Integration: Konnect Insights may excel in its ability to integrate data from a wide range of sources, including social media, online platforms, offline locations within airports, and more. This comprehensive data collection can provide a holistic view of the customer journey.
Real-Time Monitoring: The platform may provide real-time monitoring and alerting capabilities, allowing airports to respond swiftly to emerging issues or trends and enhance customer satisfaction.
Customization: Konnect Insights may offer extensive customization options, allowing airports to tailor the solution to their specific needs, adapt to unique workflows, and focus on the most relevant KPIs.
Actionable Insights: The platform may be designed to provide actionable insights and recommendations, guiding airports on concrete steps to improve the customer experience and operational efficiency.
Competitor Benchmarking: Konnect Insights may offer benchmarking capabilities that allow airports to compare their performance to industry peers or competitors, helping them identify areas for differentiation.
Security and Compliance: Given the sensitive nature of data in the aviation industry, Konnect Insights may include robust security features and compliance measures to ensure data protection and adherence to industry regulations.
Scalability: The platform may be designed to scale effectively to accommodate the data needs of large and busy airports, ensuring it can handle high volumes of data and interactions.
Customer Support and Training: Konnect Insights may offer strong customer support, training, and consulting services to help airports maximize the value of the platform and implement best practices for customer experience improvement.
Integration Capabilities: It may provide seamless integration with existing airport systems, such as CRM, ERP, and database systems, to ensure data interoperability and process efficiency.
Historical Analysis: The platform may enable airports to conduct historical analysis to track the impact of improvements and initiatives over time, helping measure progress and refine strategies.
User-Friendly Interface: Konnect Insights may prioritize a user-friendly and intuitive interface, making it accessible to a wide range of airport staff without requiring extensive technical expertise.
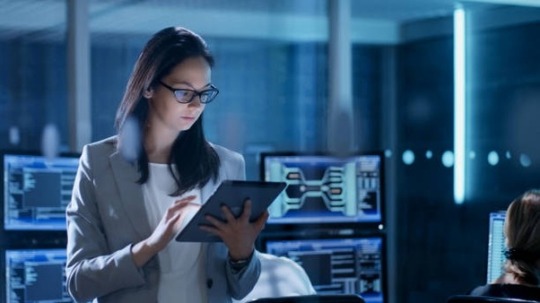
It's important for airports and organizations in the aviation industry to thoroughly evaluate their specific needs and conduct a comparative analysis of available solutions to determine which one aligns best with their goals and requirements. Additionally, staying updated with the latest developments and customer feedback regarding Konnect Insights and other similar tools can provide valuable insights when making a decision.
#DataOrchestration#DataManagement#DataOps#DataIntegration#DataEngineering#DataPipeline#DataAutomation#DataWorkflow#ETL (Extract#Transform#Load)#DataIntegrationPlatform#BigData#CloudComputing#Analytics#DataScience#AI (Artificial Intelligence)#MachineLearning#IoT (Internet of Things)#DataGovernance#DataQuality#DataSecurity
2 notes
·
View notes
Text

Every business has unique data needs, requiring customized solutions rather than one-size-fits-all approaches. Bespoke data engineering strategies align with organizational goals, ensuring optimal data architecture, integration, and processing workflows.
Whether building scalable data lakes, implementing advanced analytics, or optimizing cloud infrastructure, a tailored approach ensures maximum efficiency. Industry-specific compliance measures guarantee data security, while automated processes enhance operational agility.
Learn more about our data engineering services at https://rtctek.com/data-engineering-services/
#rtctek#roundtheclocktechnologies#data#dataengineeringservices#dataengineering#dataanalytics#datapipelines#dataworkflows#dataexperties
0 notes
Text
Beyond the Pipeline: Choosing the Right Data Engineering Service Providers for Long-Term Scalability
Introduction: Why Choosing the Right Data Engineering Service Provider is More Critical Than Ever
In an age where data is more valuable than oil, simply having pipelines isn’t enough. You need refineries, infrastructure, governance, and agility. Choosing the right data engineering service providers can make or break your enterprise’s ability to extract meaningful insights from data at scale. In fact, Gartner predicts that by 2025, 80% of data initiatives will fail due to poor data engineering practices or provider mismatches.
If you're already familiar with the basics of data engineering, this article dives deeper into why selecting the right partner isn't just a technical decision—it’s a strategic one. With rising data volumes, regulatory changes like GDPR and CCPA, and cloud-native transformations, companies can no longer afford to treat data engineering service providers as simple vendors. They are strategic enablers of business agility and innovation.
In this post, we’ll explore how to identify the most capable data engineering service providers, what advanced value propositions you should expect from them, and how to build a long-term partnership that adapts with your business.
Section 1: The Evolving Role of Data Engineering Service Providers in 2025 and Beyond
What you needed from a provider in 2020 is outdated today. The landscape has changed:
📌 Real-time data pipelines are replacing batch processes
📌 Cloud-native architectures like Snowflake, Databricks, and Redshift are dominating
📌 Machine learning and AI integration are table stakes
📌 Regulatory compliance and data governance have become core priorities
Modern data engineering service providers are not just builders—they are data architects, compliance consultants, and even AI strategists. You should look for:
📌 End-to-end capabilities: From ingestion to analytics
📌 Expertise in multi-cloud and hybrid data ecosystems
📌 Proficiency with data mesh, lakehouse, and decentralized architectures
📌 Support for DataOps, MLOps, and automation pipelines
Real-world example: A Fortune 500 retailer moved from Hadoop-based systems to a cloud-native lakehouse model with the help of a modern provider, reducing their ETL costs by 40% and speeding up analytics delivery by 60%.
Section 2: What to Look for When Vetting Data Engineering Service Providers
Before you even begin consultations, define your objectives. Are you aiming for cost efficiency, performance, real-time analytics, compliance, or all of the above?
Here’s a checklist when evaluating providers:
📌 Do they offer strategic consulting or just hands-on coding?
📌 Can they support data scaling as your organization grows?
📌 Do they have domain expertise (e.g., healthcare, finance, retail)?
📌 How do they approach data governance and privacy?
📌 What automation tools and accelerators do they provide?
📌 Can they deliver under tight deadlines without compromising quality?
Quote to consider: "We don't just need engineers. We need architects who think two years ahead." – Head of Data, FinTech company
Avoid the mistake of over-indexing on cost or credentials alone. A cheaper provider might lack scalability planning, leading to massive rework costs later.
Section 3: Red Flags That Signal Poor Fit with Data Engineering Service Providers
Not all providers are created equal. Some red flags include:
📌 One-size-fits-all data pipeline solutions
📌 Poor documentation and handover practices
📌 Lack of DevOps/DataOps maturity
📌 No visibility into data lineage or quality monitoring
📌 Heavy reliance on legacy tools
A real scenario: A manufacturing firm spent over $500k on a provider that delivered rigid ETL scripts. When the data source changed, the whole system collapsed.
Avoid this by asking your provider to walk you through previous projects, particularly how they handled pivots, scaling, and changing data regulations.
Section 4: Building a Long-Term Partnership with Data Engineering Service Providers
Think beyond the first project. Great data engineering service providers work iteratively and evolve with your business.
Steps to build strong relationships:
📌 Start with a proof-of-concept that solves a real pain point
📌 Use agile methodologies for faster, collaborative execution
📌 Schedule quarterly strategic reviews—not just performance updates
📌 Establish shared KPIs tied to business outcomes, not just delivery milestones
📌 Encourage co-innovation and sandbox testing for new data products
Real-world story: A healthcare analytics company co-developed an internal patient insights platform with their provider, eventually spinning it into a commercial SaaS product.
Section 5: Trends and Technologies the Best Data Engineering Service Providers Are Already Embracing
Stay ahead by partnering with forward-looking providers who are ahead of the curve:
📌 Data contracts and schema enforcement in streaming pipelines
📌 Use of low-code/no-code orchestration (e.g., Apache Airflow, Prefect)
📌 Serverless data engineering with tools like AWS Glue, Azure Data Factory
📌 Graph analytics and complex entity resolution
📌 Synthetic data generation for model training under privacy laws
Case in point: A financial institution cut model training costs by 30% by using synthetic data generated by its engineering provider, enabling robust yet compliant ML workflows.
Conclusion: Making the Right Choice for Long-Term Data Success
The right data engineering service providers are not just technical executioners—they’re transformation partners. They enable scalable analytics, data democratization, and even new business models.
To recap:
📌 Define goals and pain points clearly
📌 Vet for strategy, scalability, and domain expertise
📌 Watch out for rigidity, legacy tools, and shallow implementations
📌 Build agile, iterative relationships
📌 Choose providers embracing the future
Your next provider shouldn’t just deliver pipelines—they should future-proof your data ecosystem. Take a step back, ask the right questions, and choose wisely. The next few quarters of your business could depend on it.
#DataEngineering#DataEngineeringServices#DataStrategy#BigDataSolutions#ModernDataStack#CloudDataEngineering#DataPipeline#MLOps#DataOps#DataGovernance#DigitalTransformation#TechConsulting#EnterpriseData#AIandAnalytics#InnovationStrategy#FutureOfData#SmartDataDecisions#ScaleWithData#AnalyticsLeadership#DataDrivenInnovation
0 notes
Text
Data Ingestion: 12 Essential Tools and Processes for Efficient Data Management
Data Ingestion is the first step in building a robust data pipeline, involving the collection and import of data from various sources into storage or analytics systems. This guide highlights 12 essential tools and processes—like Apache NiFi, Kafka, and Airbyte—that ensure efficient, scalable, and real-time data ingestion. Mastering these tools is key to unlocking timely insights and streamlined data workflows Read More...

0 notes
Text
Making Flink Apache Available Across Your Enterprise Data

Making Flink Apache consumable in every aspect of your company: Apache Flink for all.
In this age of fast technological development, adaptability is essential. Event-driven enterprises in every industry need real-time data to respond to events as they happen. By satisfying consumers, these adaptable companies identify requirements, meet them, and take the lead in the market.
What is Apache Flink?
Here’s where Flink Apache really shines, providing a strong way to fully utilize the processing and computational power of an event-driven business architecture. This is made feasible in large part by Flink tasks, which are built to process continuous data streams.
How Apache Flink improves enterprises that are event-driven in real time
Envision a retail business that has the ability to rapidly modify its inventory by utilizing real-time sales data pipelines. In order to take advantage of new opportunities, they can quickly adjust to shifting demands. Alternatively, think about a FinTech company that can identify and stop fraudulent transactions right away. Threats are neutralized, saving the company money and averting unhappy customers. Any business hoping to be a market leader in 2018 must have these real-time capabilities, they are no longer optional.
By processing raw events, Flink Apache increases their relevance within a larger business context. When events are joined, aggregated, and enriched during event processing, deeper insights are obtained and a wide range of use cases are made possible, including:
By tracking user behavior, financial transactions, or data from Internet of Things devices, data analytics: Assists in performing analytics on data processing on streams.
From continuously streaming data streams, pattern detection makes it possible to recognize and extract complicated event patterns.
Anomaly detection: Rapidly locates anomalous activities by identifying odd patterns or outliers in streaming data.
Data aggregation makes ensuring that continuous data flows are efficiently summarized and processed so that timely insights and decisions may be made.
Stream joins: These techniques combine information from several data sources and streaming platforms to enhance event correlation and analysis.
Data filtering: This process takes streaming data and applies certain conditions to extract pertinent data.
Data manipulation: Uses data mapping, filtering, and aggregation to transform and modify data streams.
Apache Flink’s distinct benefits
In order to help organizations respond to events more effectively in real time, Flink Apache enhances event streaming solutions such as Apache Kafka. Both Flink and Kafka are strong tools, however Flink has a few more special benefits:
Data stream processing uses efficient computing to provide stately, time-based processing of data streams for use cases including predictive maintenance, transaction analysis, and client customization.
Integration: Has little trouble integrating with other platforms and data systems, such as Apache Kafka, Spark, Hadoop, and different databases.
Scalability: Manages big datasets among dispersed computers, guaranteeing performance even in the most taxing Flink tasks.
Fault tolerance ensures dependability by recovering from faults without losing data.
IBM gives users more power and enhances Apache Kafka and Flink
The de-facto standard for real-time event streaming is Apache Kafka, which should come as no surprise. But that’s only the start. A single raw stream is insufficient for most applications, and many programs can utilize the same stream in different ways.
Events can be distilled using Flink Apache, allowing them to do even more for your company. Each event stream’s value can increase dramatically when combined in this way. Leverage advanced ETL procedures, improve your event analytics, and react faster and more effectively to growing business demands. With your fingertips, you can harness the power to provide real-time automation and insights.
IBM is leading the way in stream processing and event streaming, enhancing Apache Flink’s functionality. They want to address these significant industry challenges by offering an open and modular solution for event streaming and streaming applications. Any Kafka topic can be used with Flink Apache, making it accessible to everyone.
By enhancing what clients already have, IBM technology avoids vendor lock-in. Regardless of their role, users may exploit events to supplement their data streams with real-time context, even if they lack extensive knowledge of SQL, Java, or Python, thanks to its user-friendly and no-code style. Users can increase the number of projects that can be delivered by decreasing their reliance on highly qualified technicians and freeing up developers’ time. Enabling them to concentrate on business logic, create incredibly responsive Flink apps, and reduce application workloads are the objectives.
Proceed to the next action
Companies can take the lead in their endeavors no matter where they are in their journey thanks to IBM Event Automation, an entirely modular event-driven solution. Unlocking the value of events requires an event-driven architecture, which is made possible by the event streams, event processing capabilities, and event endpoint management. In order to promote smooth integration and control, you can also manage your events similarly to APIs.
With Flink Apache and IBM Event Automation, you can move closer to a competitive, responsive, and agile IT ecosystem.
Read more on govindhtech.com
#MakingFlinkapache#AvailableAcross#EnterpriseData#realtimedata#datapipelines#realtimecapabilities#dataprocessing#datastreams#databases#sql#IBMtechnology#api#ibm#data#technology#technews#news#govindhtch
0 notes