#incorrect df
Explore tagged Tumblr posts
Text
Bose (to Mika): We should have a little romantic getaway, just you and me.
August (at the breakfast table): Guys please, we’re trying to eat here.
9 notes
·
View notes
Text
The Hero: You’re the bad guy in someone else’s story.
Sepulchure: Pal, I’m the bad guy in MY story.
#dragonfable#incorrect quotes#df hero#the hero#sepulchure#valen pulchure#valen the pure#source: tumblr
22 notes
·
View notes
Text
Some DF Incorrect Quotes
Mika: Why are your tongues purple?
Chapa: We had slushies. I had a red one.
Miles: I had a blue one.
Mika: oh
Mika:
Mika: OH
Bose:
Bose: You drank each other’s slushies?
~~~~~~~~~~~~
Mika: Can I be frank with you guys?
Bose: Sure, but I don’t see how changing your name is gonna help.
Chapa: Can I still be Chapa?
Miles: Shh, let Frank speak.
~~~~~~~~~~~~
Chapa, banging on the door: Bose! Open up!
Bose: Well, it all started when I was a kid…
Mika: No, she meant-
Miles: Let him finish.
~~~~~~~~~~~~
Bose: Is stabbing someone immoral?
Miles: Not if they consent to it.
Chapa: Depends on who you’re stabbing.
Mika: YES?!?
~~~~~~~~~~~~
Mika: Bose, I’m sad.
Bose: *Holds out arms for a hug* It’s going to be okay.
Miles: Chapa, I’m sad.
Chapa, nodding: mood.
~~~~~~~~~~~~
Bose: Oh just so you know, it’s very muggy outside
Mika:
Mika: Bose, I swear, if I step outside and all of our mugs are on the front lawn…
Bose: *Sips coffee from bowl*
~~~~~~~~~~~~
Chapa: I prevented a murder today.
Miles: Really? How’d you do that?
Chapa: self control.
#DF incorrect quotes#danger force#dangerverse#bose o'brien#mika macklin#bomika#danger force season 3#nickelodeon#mika danger force#chapa de silva#miles macklin
26 notes
·
View notes
Text
Shoutmon: Excuse you!
King Drasil: Excuse me?
Shoutmon: You’ve been ignoring someone this entire time!
King Drasil: Me?
Shoutmon: No, you mouth-breather! You’ve been ignoring your king!
King Drasil: What is a king to a god?
Shoutmon: What is a god… to a nonbeliever?!
#digimon fusion#digital pets#digimon xros wars#digimon digital monsters#digital monsters#digital pet#digimon#v pets#v pet#xros wars#fusion#df#dxw#source: dbz abridged#incorrect quotations#incorrect quotes#iq#quotes#quote#quotations#incorrect#source: dbza#source: dragon ball z abridged#virtual pets#virtual pet#xw#tamagotchi
2 notes
·
View notes
Text
doing another gender presentation (ha) at another church that is going to pay me to be transgender for an hour. awesome
#blah blah blah#the last iteration of this presentation was very yikes#i was trying to pack 300-level 90 minute nuance into a 000-level 20 minute class because i myself am a phd in being queer#and trying to put all that nuance meant i got choppy and awkward and accidentally gave Incorrect messages because of the incoherent flow#oopsie#but i'm good now#*this presentation is different from the one i did a few weeks ago. that one was fine. this is an edit of one i did a few years ago and whe#all the over-clarification and granular definitions was ACCIDENTALLY giving r*df*m vibes. yikesaroonie glad i'm fixing this shit#this iteration is much more 'words are whatever. be nice about em' and that is MUCH healthier and much closer to my actual beliefs#smh embarrassed about how the last version of this was. my apologies to pastor e about the accidental disservice i did to his group#my resident cis(?)het(?) (affectionate) is my proofreader tonight
5 notes
·
View notes
Text
Aldereyes/star: MYRTLEWING LIKES ME?? NO WAY WE’RE JUST FRIENDS ALWAYS HAVE BEEN AND OH I CAN’T BELIEVE MYRTLEWING IS A MURDERER! OR THAT HE LIKES ME--ROMANTICALLY! WE’RE JUST FRIENDS BUT NOW WE CAN’T BE BECAUSE APPARENTLY HE’S A DERANGED KILLER HE’S LITTERALLY A MONSTER HOW CAN HE FEEL LOVE ANYWAY
Aldereyes/star some time later, to Myrtlewing: babe, watch this! *punches through a cat’s throat* :D
#sprouting thorns#wc#aldereyes#alderstar#myrtlewing#eye-out poly#eye-out family#warriors#warriors oc#wc incorrect quotes#warriors incorrect quotes#warrior cats#warrior cats oc#df oc#dark forest oc
2 notes
·
View notes
Text
Ollie: Ugh, can't move in your body, why do you wear such tight clothes.
Javi: Why does your wardrobe look like the bargain bin from a store. It's too warm for this stuff.
Aiyon: Now this is fun. *bites lip*
Izzy: You guys are weird.
#DF ep 19#the makeover#power rangers#dino fury#power rangers dino fury#prdf#incorrect power rangers#incorrect quotes#incorrect power ranger quotes#javi garcia#ollie akana#izzy garcia#aiyon#aiyon has no filter
6 notes
·
View notes
Text
Snowbrook and Lani quotes but it's based on random posts I find
Snowbrook: of course I have a praise kink, I was ignored as a child
--
Snowbrook: just learned my entire personality is a trauma response lol
--
Lani: no rizz just brown eyes and really fucking stupid
--
Lani: not to sound like a total whore but oh boy it would be so nice to have a hug and some reassurance
--
Lani: every time I make a mistake I think the only solution is being put down like a sick dog
--
Snowbrook: I get so affectionate when I'm sleepy, it's disgusting
--
Snowbrook: if a child asked me nicely I would for sure give one a cigarette, no questions asked
--
Snowbrook: inside, I am still the little girl on the playground with no friends
--
Snowbrook: all I ask is for you to know me on a deep, intimate level while I resist and obstruct your every attempt to do so
--
Snowbrook: I hate when people ask you to open up like bro I met you 4 years ago give it time
--
Lani: ok wow so I guess these friendship bracelets I made for us mean nothing
--
Lani: I'm not gullible enough to be lured into a cult but I am nosy enough
--
Lani: why is gorgeous the only thing you can be drop-dead? I wanna be drop-dead silly. Let my enemies crumble before me, overcome by the depths of my whimsy. And I will be gorgeous also
1 note
·
View note
Text
Dismissed Manifestations
wtf just realised i manifested something without realising.
technically that isn’t true.
so i’ve been wanting to manifest my sp to make moves and actually interact with me and my db and df
then i saw a post in passing about how manifesting small things shows that manifesting is true
i also made a post about that
i was like hmm i should do that since i’ve been losing faith
so i was like hmm i really want some fries rn but then realised ugh that’s not possible since there’s food at home (smh🤦♀️ im my own limiting belief)
so i kinda forgot about it just expecting to eat the food at home. then nearly 7 hours later my sister told me that the food was finished. i was like oh ok ill just find something to eat.
my other sister(who also hasn’t eaten) came up to me and hour later and said there’s nothing at home.
so what did we do? ask my sister to order food
GUESS WHO JUST ORDERES FRIES!!!!
this made me realise that manifesting is not immediate and can happen even if the thought was in passing and if u want it so bad.
i thought of having fries once and here i am having it‼️‼️‼️
ignore incorrect grammar pls i didn’t read it
#manifesation#manifesting#shifting#law of assumption#loa success#success story#loablr#neville goddard#reality shift
18 notes
·
View notes
Text
Now let's talk about how Cooper liked a flirty Bomika incorrect quote he wasn't even tagged in😭 in that live where he acknowledged a question abt what qas his favorite df ship he def wanted to say bomika, DON'T PLAY WITH HIM.
#COOPER CHOSE A SIDE#on the right side of history#danger force#dangerverse#ray Manchester#cooper barnes
30 notes
·
View notes
Text
Mika: Bose honey, wanna take a shower with me?
Bose: I have a gun in that nightstand beside the bed. If I ever say no to that question, I want you to take it out and shot me because I’ve obviously gone crazy.
#danger force#bomika#otp: we are in danger of falling in love#bose x mika#mika x bose#het ship#incorrect df
6 notes
·
View notes
Text
[At Warlic’s Funeral]
Xan: I hate funerals. I never know what to say.
The Hero: Just say “I’m sorry for your loss” and move on.
Xan, to the crowd: I’m sorry for your loss. Move on.
18 notes
·
View notes
Text
Step-by-Step Guide with Code Example Replacing NA Values with Zeros in R: A Simple Guide Handling missing data is a common task in data analysis. In R, missing values are represented as NA, and one common way to deal with them is by replacing them with a value like zero. This can be useful when you want to maintain the dataset's integrity without introducing biases. In this article, we'll go over a simple method to replace all NA values in a dataframe with zeros using base R functions. Let’s dive in with a code example you can easily copy and paste into your R environment. Why Replace NA Values? When dealing with datasets that contain missing values, many functions in R might fail or return incorrect results. Replacing missing values with zeros ensures you can continue your analysis without errors. In some scenarios, zero can represent a meaningful value, such as a measurement of no activity or a missing count. Step-by-Step Example Let’s walk through an example of how to replace NA values in an R dataframe with zeros. Sample Dataframe Here’s a small dataframe with some missing values (NA): RCopy code# Sample dataframe with NA values df
0 notes
Text
Data Cleaning Techniques Every Data Scientist Should Know
Data Cleaning Techniques Every Data Scientist Should Know
Data cleaning is a vital step in any data analysis or machine learning workflow. Raw data is often messy, containing inaccuracies, missing values, and inconsistencies that can impact the quality of insights or the performance of models.
Below, we outline essential data cleaning techniques that every data scientist should know.
Handling Missing Values
Missing data is a common issue in datasets and needs to be addressed carefully.
Common approaches include:
Removing Missing Data:
If a row or column has too many missing values, it might be better to drop it entirely.
python
df.dropna(inplace=True)
# Removes rows with any missing values Imputing Missing Data:
Replace missing values with statistical measures like mean, median, or mode.
python
df[‘column’].fillna(df[‘column’].mean(),
inplace=True)
Using Predictive Imputation:
Leverage machine learning models to predict and fill missing values.
2. Removing Duplicates Duplicates can skew your analysis and result in biased models.
Identify and remove them efficiently:
python
df.drop_duplicates(inplace=True)
3. Handling Outliers
Outliers can distort your analysis and lead to misleading conclusions.
Techniques to handle them include:
Visualization: Use boxplots or scatter plots to identify outliers.
Clipping: Cap values that exceed a specific threshold.
python
df[‘column’] = df[‘column’].clip(lower=lower_limit, upper=upper_limit)
Transformation: Apply logarithmic or other transformations to normalize data.
4. Standardizing and Normalizing
Data To ensure consistency, particularly for machine learning algorithms, data should often be standardized or normalized:
Standardization: Converts data to a mean of 0 and a standard deviation of
python from sklearn.preprocessing
import StandardScaler scaler = StandardScaler()
df_scaled = scaler.fit_transform(df)
Normalization:
Scales values to a range between 0 and 1.
pythonfrom sklearn.preprocessing
import MinMaxScaler scaler = MinMaxScaler()
df_normalized = scaler.fit_transform(df)
5. Fixing Structural Errors
Structural errors include inconsistent naming conventions, typos, or incorrect data types.
Correct these issues by: Renaming columns for uniformity:
python df.rename(columns={‘OldName’: ‘NewName’}, inplace=True)
Correcting data types:
python
df[‘column’] = df[‘column’].astype(‘int’)
6. Encoding Categorical Data
Many algorithms require numeric input, so categorical variables must be encoded:
One-Hot Encoding:
python
pd.get_dummies(df, columns=[‘categorical_column’], drop_first=True)
Label Encoding:
python
from sklearn.preprocessing
import LabelEncoder encoder = LabelEncoder()
df[‘column’] = encoder.fit_transform(df[‘column’])
7. Addressing Multicollinearity
Highly correlated features can confuse models.
Use correlation matrices or Variance Inflation Factor (VIF) to identify and reduce multicollinearity.
8. Scaling Large Datasets For datasets with varying scales, scaling ensures all features contribute equally to the model:
python
from sklearn.preprocessing
import StandardScaler scaler = StandardScaler()
df_scaled = scaler.fit_transform(df)
Tools for Data Cleaning
Python Libraries:
Pandas, NumPy, OpenRefine Automation:
Libraries like dataprep or pyjanitor streamline the cleaning process.
Visual Inspection:
Tools like Tableau and Power BI help spot inconsistencies visually. Conclusion Data cleaning is the foundation of accurate data analysis and successful machine learning projects.
Mastering these techniques ensures that your data is reliable, interpretable, and actionable.
As a data scientist, developing an efficient data cleaning workflow is an investment in producing quality insights and impactful results.
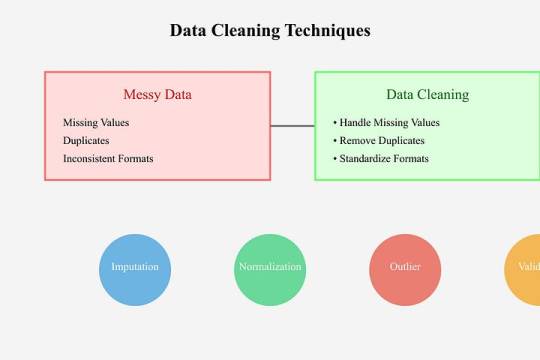
0 notes
Text
Hi everyone,
I'm sharing my code and output here. I'd greatly appreciate any recommendations or suggestions for improvement. If you notice any errors or incorrect interpretations in my code, please feel free to point them out—I'd be very grateful for your feedback. As someone learning decision trees for the first time, your insights would be incredibly valuable to me.
Thank you!
import pandas as pd import os import matplotlib.pylab as plt from sklearn.model_selection import train_test_split from sklearn.tree import DecisionTreeClassifier import sklearn.metrics
Load dataset (a built-in datasets from Scikit-learn)
from sklearn.datasets import load_breast_cancer AH_data = load_breast_cancer(as_frame=True)
remove NA
data_clean = AH_data.frame.dropna()
print(load_breast_cancer()) print(data_clean.head())
data_clean.dtypes data_clean.describe()
print(data_clean.columns)
df = AH_data.frame
Predictors uses all columns except "target"
Predictors = df.drop(columns="target")
targets = df["target"]
pred_train, pred_test, tar_train, tar_test = train_test_split(Predictors, targets, test_size=.4)
pred_train.shape pred_test.shape tar_train.shape tar_test.shape
classifier = DecisionTreeClassifier() classifier = classifier.fit(pred_train, tar_train)
predictions = classifier.predict(pred_test)
Shows correct and incorrect classifications
sklearn.metrics.confusion_matrix(tar_test, predictions)
diagonal represents no. of true negatives and no. of true positives
bottom left represents no. of false negatives
upper right no. of false positives
sklearn.metrics.accuracy_score(tar_test, predictions)
eg. If output is (0.899), it will suggest decesion tree model has classify 90% correctly
Display the decision tree
from sklearn import tree from io import StringIO from IPython.display import Image out = StringIO() tree.export_graphviz(classifier, out_file=out)
import pydotplus graph=pydotplus.graph_from_dot_data(out.getvalue()) Image(graph.create_png())
1 note
·
View note
Text
ANOVA Analysis
Course "Data Analysis Tools" on the Coursera platform corresponding to week 01. The objective is to execute an Analysis of Variance using the ANOVA statistical test, this analysis evaluates the measurements of two or more groups that are statistically different from each other. It is mainly used when you want to compare the measurements (quantitative variables) of groups (categorical variables). The hypotheses are the following: H0="There is no difference in the mean of the quantitative variable between groups (categorical variable)" H1= While the alternatives there is a difference.
The Code in Phython
import numpy as ns import pandas as pd import statsmodels.api as sm import statsmodels.formula.api as smf import statsmodels.stats.multicomp as multi import matplotlib.pyplot as plt import seaborn as sns import researchpy as rp import pycountry_convert as pc
df = pd.read_csv('gapminder.csv')
df = df[['lifeexpectancy', 'incomeperperson']]
df['lifeexpectancy'] = df['lifeexpectancy'].apply(pd.to_numeric, errors='coerce')
def income_categories(row): if row["incomeperperson"]>15000: return "A" elif row["incomeperperson"]>8000: return "B" elif row["incomeperperson"]>4000: return "C" elif row["incomeperperson"]>1000: return "D" else: return "E"
df=df[(df['lifeexpectancy']>=1) & (df['lifeexpectancy']<=120) & (df['incomeperperson'] > 0) ]
df["Income_category"]=df.apply(income_categories, axis=1)
df = df[["Income_category","incomeperperson","lifeexpectancy"]].dropna()
df["Income_category"]=df.apply(income_categories, axis=1)
print (rp.summary_cont(df['lifeexpectancy']))
fig1, ax1 = plt.subplots() df_new = [df[df['Income_category']=='A']['lifeexpectancy'], df[df['Income_category']=='B']['lifeexpectancy'], df[df['Income_category']=='C']['lifeexpectancy'], df[df['Income_category']=='D']['lifeexpectancy'], df[df['Income_category']=='E']['lifeexpectancy']] ax1.set_title('life expectancy') ax1.boxplot(df_new) plt.show()
results = smf.ols('lifeexpectancy ~ C(Income_category)', data=df).fit() print (results.summary())
print ("Tukey") mc1 = multi.MultiComparison(df['lifeexpectancy'], df['Income_category']) print (mc1) res1 = mc1.tukeyhsd() print (res1.summary())
print ('means for for life expectancy by Income') m1= df.groupby('Income_category').mean() print (m1)
print ('Results') print ('standard deviations for life expectancy by Income') sd1 = df.groupby('Income_category').std() print (sd1)
Conclusions – ANOVA Analysis
An ANOVA test is carried out, integrated by 176 rows, 171 will be used for the test. A filter is applied to eliminate some incorrect values, such as non-numeric, negative, etc., reducing the rows of the original data set.
The ANOVA analysis shows a graph for each category (at the top) and, as can be seen, the life expectancy of class A, has a life expectancy of 80.39 years while the E class has a life expectancy of 59, 15 years
1 note
·
View note