#predictive analytics solutions
Explore tagged Tumblr posts
Text
Business Analytics Services | Connect Infosoft | Data-Driven Solutions
Discover the transformative potential of your data with Connect Infosoft's Business Analytics services. Our expert team offers comprehensive solutions to unlock actionable insights and drive informed decision-making. From analytics consulting to advanced tools and visualization services, we empower businesses to harness the full power of their data. Partner with us for predictive analytics, data visualization, and business intelligence tools that pave the way for success.
For more info: Click Here
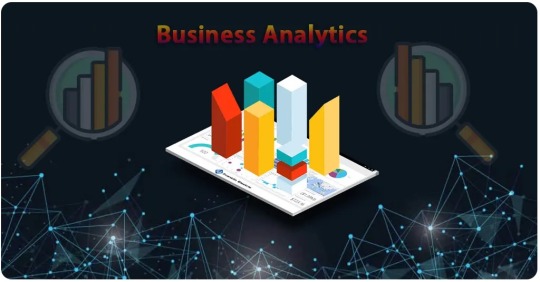
#Business analytics solutions#Data-driven insights#Analytics consulting services#Business intelligence tools#Advanced analytics services#Data visualization services#Predictive analytics solutions#Data analysis consulting#Business data analytics#Data-driven decision making
0 notes
Text
Why Do Brands Invest in Predictive Analytics Services?
Forecasting business performance can go wrong if data quality management (DQM) has flaws. While in-house professionals might know suitable data processing methods, selecting established predictive analytics services ensures maximum efficiency. This page lists what a company will get from a data partner specializing in predictive and prescriptive insight discovery.
0 notes
Text
Know Everything About Predictive Analytics
Predictive analytics is the process of using data, statistical algorithms, and machine learning techniques to analyze historical data and make predictions about future events or outcomes. It involves extracting meaningful patterns and relationships from past data to forecast what might happen in the future.
What is Predictive Analytics
Predictive analytics is a field of data analysis that involves using historical data and statistical algorithms to make predictions about future events or outcomes. It’s a form of advanced analytics that goes beyond describing what happened in the past or analyzing the present but instead focuses on forecasting what might happen in the future.
The main goal of predictive analytics is to identify patterns, trends, and relationships in historical data and use this information to make informed predictions about potential future scenarios. By leveraging these insights, businesses, and organizations can make better decisions, anticipate risks, optimize processes, and gain a competitive advantage.
The process of predictive analytics involves several steps, including data collection, data preprocessing, data exploration, model selection, model training, model evaluation, model deployment, and ongoing monitoring and maintenance.
Predictive analytics is widely used in various industries and applications. For example, it can be applied to predict customer churn in businesses, forecast sales and demand for products, detect fraudulent activities in financial transactions, optimize supply chain operations, predict equipment failures in maintenance processes, and even forecast disease outbreaks in healthcare.
Predictive Analytics Techniques
Predictive analytics employs a variety of techniques and methods to make accurate predictions based on historical data. Here are some commonly used techniques in predictive analytics:
Regression Analysis: Regression models are used to establish relationships between dependent and independent variables. It helps in predicting a continuous numeric value, such as predicting sales or estimating a person’s age based on various factors.
Classification: Classification techniques are used to predict categorical outcomes, such as whether a customer will buy a product (yes/no) or whether an email is spam or not. Common classification algorithms include Decision Trees, Random Forests, Support Vector Machines (SVM), and Logistic Regression.
Time Series Analysis: Time series models are used when dealing with sequential data points that are collected over time. They are useful for predicting future values based on past observations and trends. Time series forecasting is widely used in finance, economics, and various other fields.
Machine Learning Algorithms: Various machine learning algorithms, such as k-Nearest Neighbors (k-NN), Naive Bayes, and Neural Networks, can be used for predictive analytics tasks depending on the problem at hand and the nature of the data.
Clustering Analysis: Clustering techniques are used to group similar data points together. While clustering itself is not a prediction task, it can help identify patterns within the data that may aid in making predictions or segmenting customers based on behavior.
Text Mining and Natural Language Processing (NLP): These techniques are used to analyze and extract insights from unstructured text data. Sentiment analysis, for instance, can predict the sentiment of customer reviews or social media comments.
Ensemble Methods: Ensemble techniques combine the predictions of multiple models to improve accuracy and reduce overfitting. Bagging (e.g., Random Forest) and boosting (e.g., Gradient Boosting Machines) are popular ensemble methods.
Anomaly Detection: Anomaly detection techniques are used to identify unusual patterns or outliers in data, which can be useful for predicting rare events or detecting anomalies in real time.
Collaborative Filtering: This technique is widely used in recommendation systems. It predicts a user’s preference for a product or service based on the preferences of similar users.
Survival Analysis: Survival analysis is used to predict the time to an event’s occurrence, such as predicting customer churn or the lifespan of a product.
The choice of predictive analytics technique depends on the specific problem, the type of data available, and the desired outcome. Often, a combination of different techniques and data preprocessing steps is necessary to create an accurate predictive model.
How to Implement Predictive Analytics in Your Business
Implementing predictive analytics in your business involves several key steps to ensure its successful integration and utilization. Here’s a general guide to help you get started:
Define Your Objectives: Clearly outline the business objectives you want to achieve with predictive analytics. Identify the specific problems you want to solve or the opportunities you want to explore. Having well-defined goals will help you focus your efforts and resources effectively.
Collect and Prepare Data: Gather relevant data from various sources within your organization. This data could include historical records, customer information, sales data, website activity, and any other data that is pertinent to your objectives. Clean and preprocess the data to ensure its accuracy and consistency.
Select the Right Tools and Techniques: Choose the appropriate predictive analytics tools and techniques that align with your objectives and data. Consider the nature of your data (numeric, categorical, sequential), the type of predictions you want to make (regression, classification, time series forecasting), and the available resources (software, hardware, expertise).
Build Predictive Models: Train and build predictive models using the preprocessed data and selected techniques. This step involves splitting your data into training and testing sets to assess the model’s performance. Experiment with various algorithms to find the best fit for your data.
Validate and Evaluate Models: Validate the models using testing data to ensure they perform well and generalize to unseen data. Evaluate the models using appropriate metrics such as accuracy, precision, recall, or mean squared error, depending on the nature of your predictive task.
Implement Models in Your Business Processes: Once you have validated and selected the best-performing models, integrate them into your business processes. This could involve deploying the models into existing software systems, creating APIs for real-time predictions, or building dashboards for decision-makers.
Train Your Team: Provide training to your team members who will be working with predictive analytics. Ensure they understand the models, their implications, and how to interpret the results. This will help your team effectively use predictive analytics insights to make data-driven decisions.
Monitor and Update: Continuously monitor the performance of your predictive models and update them as needed. As new data becomes available, retrain the models to keep them accurate and up-to-date.
Start Small and Scale: Consider starting with smaller pilot projects to test the feasibility and effectiveness of predictive analytics in your business. Once you gain confidence and see positive results, you can gradually scale up and apply predictive analytics to more areas of your business.
Stay Compliant with Data Regulations: Ensure that you comply with data privacy and security regulations when implementing predictive analytics. Protect sensitive data and obtain necessary permissions from customers or stakeholders, as required.
Remember that implementing predictive analytics is an iterative process. It’s essential to continuously learn from your results, refine your models, and adapt to changing business needs. With time and effort, predictive analytics can become a valuable asset in making informed decisions and gaining a competitive advantage in your industry.
#predictive analytics services#predictive analytics consulting#predictive analytics solutions#predictive analytics consulting services
0 notes
Text
Top 5 DeepSeek AI Features Powering Industry Innovation
Table of Contents1. The Problem: Why Legacy Tools Can’t Keep Up2. What Makes DeepSeek AI Unique?3. 5 Game-Changing DeepSeek AI Features (with Real Stories)3.1 Adaptive Learning Engine3.2 Real-Time Anomaly Detection3.3 Natural Language Reports3.4 Multi-Cloud Sync3.5 Ethical AI Auditor4. How These Features Solve Everyday Challenges5. Step-by-Step: Getting Started with DeepSeek AI6. FAQs: Your…
#affordable AI solutions#AI automation#AI for educators#AI for entrepreneurs#AI for non-techies#AI for small business#AI in manufacturing#AI innovation 2024#AI time management#business growth tools#data-driven decisions#DeepSeek AI Features#ethical AI solutions#healthcare AI tools#no-code AI tools#Predictive Analytics#real-time analytics#remote work AI#retail AI features#startup AI tech
2 notes
·
View notes
Text
Boost E-commerce in Saudi Arabia with ML-Powered Apps
In today's digital era, the e-commerce industry in Saudi Arabia is rapidly expanding, fueled by increasing internet penetration and a tech-savvy population. To stay competitive, businesses are turning to advanced technologies, particularly Machine Learning (ML), to enhance user experiences, optimize operations, and drive growth. This article explores how ML is transforming the e-commerce landscape in Saudi Arabia and how businesses can leverage this technology to boost their success.
The Current E-commerce Landscape in Saudi Arabia
The e-commerce market in Saudi Arabia has seen exponential growth over the past few years. With a young population, widespread smartphone usage, and supportive government policies, the Kingdom is poised to become a leading e-commerce hub in the Middle East. Key players like Noon, Souq, and Jarir have set the stage, but the market is ripe for innovation, especially with the integration of Machine Learning.
The Role of Machine Learning in E-commerce
Machine Learning, a subset of Artificial Intelligence (AI), involves the use of algorithms to analyze data, learn from it, and make informed decisions. In e-commerce, ML enhances various aspects, from personalization to fraud detection. Machine Learning’s ability to analyze large datasets and identify trends is crucial for businesses aiming to stay ahead in a competitive market.
Personalized Shopping Experiences
Personalization is crucial in today’s e-commerce environment. ML algorithms analyze user data, such as browsing history and purchase behavior, to recommend products that align with individual preferences. This not only elevates the customer experience but also drives higher conversion rates. For example, platforms that leverage ML for personalization have seen significant boosts in sales, as users are more likely to purchase items that resonate with their interests.
Optimizing Inventory Management
Effective inventory management is critical for e-commerce success. ML-driven predictive analytics can forecast demand with high accuracy, helping businesses maintain optimal inventory levels. This minimizes the chances of overstocking or running out of products, ensuring timely availability for customers. E-commerce giants like Amazon have successfully implemented ML to streamline their inventory management processes, setting a benchmark for others to follow.
Dynamic Pricing Strategies
Price is a major factor influencing consumer decisions. Machine Learning enables real-time dynamic pricing by assessing market trends, competitor rates, and customer demand. This allows businesses to adjust their prices to maximize revenue while remaining competitive. Dynamic pricing, powered by ML, has proven effective in attracting price-sensitive customers and increasing overall profitability.
Enhanced Customer Support
Customer support is another area where ML shines. AI-powered chatbots and virtual assistants can handle a large volume of customer inquiries, providing instant responses and resolving issues efficiently. This not only improves customer satisfaction but also reduces the operational costs associated with maintaining a large support team. E-commerce businesses in Saudi Arabia can greatly benefit from incorporating ML into their customer service strategies.
Fraud Detection and Security
With the rise of online transactions, ensuring the security of customer data and payments is paramount. ML algorithms can detect fraudulent activities by analyzing transaction patterns and identifying anomalies. By implementing ML-driven security measures, e-commerce businesses can protect their customers and build trust, which is essential for long-term success.
Improving Marketing Campaigns
Effective marketing is key to driving e-commerce success. ML can analyze customer data to create targeted marketing campaigns that resonate with specific audiences. It enhances the impact of marketing efforts, leading to improved customer engagement and higher conversion rates. Successful e-commerce platforms use ML to fine-tune their marketing strategies, ensuring that their messages reach the right people at the right time.
Case Study: Successful E-commerce Companies in Saudi Arabia Using ML
Several e-commerce companies in Saudi Arabia have already begun leveraging ML to drive growth. For example, Noon uses ML to personalize the shopping experience and optimize its supply chain, leading to increased customer satisfaction and operational efficiency. These companies serve as examples of how ML can be a game-changer in the competitive e-commerce market.
Challenges of Implementing Machine Learning in E-commerce
While the benefits of ML are clear, implementing this technology in e-commerce is not without challenges. Technical hurdles, such as integrating ML with existing systems, can be daunting. Additionally, there are concerns about data privacy, particularly in handling sensitive customer information. Businesses must address these challenges to fully harness the power of ML.
Future Trends in Machine Learning and E-commerce
As ML continues to evolve, new trends are emerging that will shape the future of e-commerce. For instance, the integration of ML with augmented reality (AR) offers exciting possibilities, such as virtual try-ons for products. Businesses that stay ahead of these trends will be well-positioned to lead the market in the coming years.
Influence of Machine Learning on Consumer Behavior in Saudi Arabia
ML is already influencing consumer behavior in Saudi Arabia, with personalized experiences leading to increased customer loyalty. As more businesses adopt ML, consumers can expect even more tailored shopping experiences, further enhancing their satisfaction and engagement.
Government Support and Regulations
The Saudi government is proactively encouraging the integration of cutting-edge technologies, including ML, within the e-commerce industry. Through initiatives like Vision 2030, the government aims to transform the Kingdom into a global tech hub. However, businesses must also navigate regulations related to data privacy and AI to ensure compliance.
Conclusion
Machine Learning is revolutionizing e-commerce in Saudi Arabia, offering businesses new ways to enhance user experiences, optimize operations, and drive growth. By embracing ML, e-commerce companies can not only stay competitive but also set new standards in the industry. The future of e-commerce in Saudi Arabia is bright, and Machine Learning will undoubtedly play a pivotal role in shaping its success.
FAQs
How does Machine Learning contribute to the e-commerce sector? Machine Learning enhances e-commerce by improving personalization, optimizing inventory, enabling dynamic pricing, and enhancing security.
How can Machine Learning improve customer experiences in e-commerce? ML analyzes user data to provide personalized recommendations, faster customer support, and tailored marketing campaigns, improving overall satisfaction.
What are the challenges of integrating ML in e-commerce? Challenges include technical integration, data privacy concerns, and the need for skilled professionals to manage ML systems effectively.
Which Saudi e-commerce companies are successfully using ML? Companies like Noon and Souq are leveraging ML for personalized shopping experiences, inventory management, and customer support.
What is the future of e-commerce with ML in Saudi Arabia? The future looks promising with trends like ML-driven AR experiences and more personalized
#machine learning e-commerce#Saudi Arabia tech#ML-powered apps#e-commerce growth#AI in retail#customer experience Saudi Arabia#digital transformation Saudi#ML app benefits#AI-driven marketing#predictive analytics retail#Saudi digital economy#e-commerce innovation#smart retail solutions#AI tech adoption#machine learning in business
2 notes
·
View notes
Text
Migrating Legacy Contact Centers to Smart AI Solutions

Introduction
In an era dominated by digital transformation, businesses are rapidly shifting from traditional, on-premise contact center systems to smart, AI-powered platforms. This migration is not merely a trend—it’s a strategic imperative. Legacy contact centers, while once reliable, often struggle to keep up with the demands of modern customers who expect seamless, real-time, omnichannel support. Smart AI solutions offer a scalable, efficient, and intelligent approach to managing customer interactions while significantly improving the overall customer experience (CX).
Why Legacy Contact Centers Fall Short
Legacy contact centers were built to handle voice calls through physical infrastructure and manual workflows. These systems are rigid, expensive to maintain, and lack the flexibility needed for today’s fast-paced digital environment. Some key limitations include:
Limited scalability
High operational costs
Minimal integration with digital channels
Lack of real-time data analytics
Inability to support remote agents effectively
Moreover, legacy systems are often siloed, making it difficult to provide a unified customer experience across channels such as email, chat, social media, and messaging apps.
The Case for AI-Powered Contact Centers
AI contact centers leverage technologies like machine learning, natural language processing (NLP), and robotic process automation (RPA) to enhance and automate customer interactions. These platforms can intelligently route queries, provide self-service options, and analyze customer sentiment in real time.
Key benefits of migrating to a smart AI solution include:
Enhanced customer experience (CX) with personalized, context-aware interactions
24/7 availability through AI-powered virtual agents and chatbots
Omnichannel support that unifies communication across voice, email, chat, SMS, and social platforms
Cost savings through intelligent automation and reduced reliance on live agents
AI-driven analytics for better decision-making and performance optimization
Key Technologies Powering Smart AI Contact Centers
Natural Language Processing (NLP) NLP enables AI to understand and respond to human language more effectively. It powers chatbots, virtual assistants, and intelligent IVRs, making interactions more human-like and intuitive.
Machine Learning and Predictive Analytics Machine learning models analyze historical data to predict customer behavior, enabling proactive service and intelligent routing of interactions to the right agents or systems.
AI-Driven Automation Robotic process automation (RPA) handles repetitive tasks such as data entry, verification, and ticket generation, allowing agents to focus on complex issues.
Cloud-Based Infrastructure Modern AI contact centers are built on the cloud, enabling easy scalability, remote agent support, and seamless updates without downtime.
Speech Recognition and Sentiment Analysis These tools analyze tone and emotion during voice interactions, helping organizations adapt responses in real time to improve outcomes.
The Migration Journey: Key Steps and Best Practices
Migrating to a smart AI contact center requires strategic planning and execution. Here’s a high-level roadmap:
1. Assess Your Current State
Begin with a comprehensive audit of your existing contact center infrastructure, workflows, customer pain points, and technology stack. Identify gaps in CX, agent productivity, and system performance.
2. Define Your Objectives
Clearly define your goals—whether it's improving response times, enabling omnichannel support, or reducing costs through automation. These objectives will guide technology selection and implementation strategy.
3. Choose the Right AI Contact Center Platform
Look for platforms that offer:
Seamless cloud migration
Integration with your existing CRM and support systems
AI-powered virtual agents and intelligent routing
Real-time dashboards and AI-driven analytics
Security and compliance features
Top vendors include Amazon Connect, Google Cloud Contact Center AI, Genesys Cloud, and Five9.
4. Plan for Integration and Data Migration
Ensure that customer data, interaction history, and knowledge bases are migrated securely and accurately. APIs and middleware tools can help integrate legacy systems during the transition phase.
5. Train AI Models and Agents
Leverage historical interaction data to train your virtual assistants and automation tools. Concurrently, provide your human agents with training on new tools and AI-assisted workflows.
6. Monitor, Optimize, and Iterate
Post-migration, continuously monitor system performance, customer feedback, and agent productivity. Use AI-driven analytics to identify areas for improvement and adapt quickly.
Addressing Common Challenges
Data Privacy and Compliance: Ensure your new platform adheres to regulations such as GDPR, HIPAA, or PCI-DSS. AI systems should handle sensitive information responsibly.
Change Management: Prepare your team for the cultural shift. AI is meant to augment—not replace—human agents. Empower them with AI tools to work more efficiently.
Integration Complexity: Work with experienced technology partners or consultants who specialize in cloud migration and AI implementation to reduce friction during integration.
Real-World Impact: AI in Action
A leading telecom company replaced its legacy call center with a cloud-based AI solution. The results included:
35% reduction in average handling time (AHT)
50% increase in first contact resolution (FCR)
40% improvement in customer satisfaction (CSAT)
60% of queries handled by AI-powered virtual agents
This transformation not only enhanced operational efficiency but also empowered agents with real-time insights and support tools, allowing them to focus on high-value interactions.
The Future of AI Contact Centers
As generative AI and real-time voice synthesis continue to evolve, smart contact centers will become even more sophisticated. We can expect:
Hyper-personalized customer journeys driven by behavioral analytics
Real-time agent assist tools offering prompts and next-best actions
Voice bots with near-human conversational capabilities
Deeper integration with enterprise systems like ERP and sales platforms
The AI contact center is no longer a futuristic concept—it is today’s strategic advantage.
Conclusion
Migrating legacy contact centers to smart AI solutions is a transformative move that enables organizations to meet the demands of today’s digital-first customers. By embracing AI-powered tools, businesses can deliver superior customer experiences, improve operational efficiency, and gain a competitive edge.
This transition, while complex, can be managed effectively with the right strategy, technology, and partners. As AI continues to evolve, the future of customer engagement lies in intelligent, adaptive, and scalable contact center platforms.
#AI contact center#legacy contact center#customer experience (CX)#contact center migration#AI-powered contact center#intelligent automation#cloud contact center#natural language processing (NLP)#AI-driven analytics#omnichannel support#virtual agents#chatbots for contact centers#contact center modernization#machine learning in customer service#contact center cloud migration#smart contact center solutions#customer service automation#speech recognition AI#predictive analytics for CX#digital transformation in customer support
0 notes
Text
Beyond Maintenance: Expanding Predictive Analytics Applications in Manufacturing
When most people think of predictive analytics in manufacturing, the first thing that comes to mind is predictive maintenance. And it's true that this is one of the most popular and effective uses of predictive technology. By analyzing machine data, manufacturers can forecast when equipment is likely to fail and fix it before it causes downtime. But predictive analytics can do much more than just keep machines running.

In this blog, we’ll explore how manufacturers can go beyond maintenance and use predictive analytics in new ways to improve efficiency, reduce waste, and make smarter business decisions.
Quality Control and Defect Prevention
Predictive analytics can play a powerful role in improving product quality. By analyzing data from production lines, such as temperature, pressure, speed, or raw material quality, systems can identify patterns that lead to defects. This allows manufacturers to fix problems early, before they turn into major quality issues.
For example, if a slight change in raw material thickness often leads to defects, the system can flag it immediately. This helps maintain consistent product quality and reduces the number of returns or complaints.
Bonus Benefit: Predictive analytics can also help adjust processes in real time to ensure every product meets quality standards, saving time, money, and resources.
Energy Usage Optimization
Factories use a lot of energy, and energy costs can add up quickly. Predictive analytics can help monitor and forecast energy consumption across equipment, shifts, or departments. Over time, the system learns usage patterns and can suggest changes to save energy and lower costs.
For instance, it might identify that certain machines use more power during peak hours and suggest running them at off-peak times instead. It can also detect unusual spikes in energy use that could point to faulty equipment or inefficient processes.
This use of predictive tools supports sustainability efforts and can even help manufacturers meet government regulations on energy use.
Inventory and Supply Chain Forecasting
Another exciting application is in inventory management and supply chain optimization. By looking at past sales data, seasonal trends, and supplier performance, predictive analytics can forecast future demand and supply needs. This helps avoid stockouts or overstocking, both of which can hurt profits.
Imagine knowing in advance that a specific part will be in short supply next month—or predicting that customer demand for a product will spike next quarter. These insights help manufacturers plan better, reduce waste, and keep operations running smoothly.
This is one of the areas where data analytics in the manufacturing industry is truly transforming how businesses operate, moving from reactive to proactive decision-making.
Workforce Planning and Productivity
Predictive analytics can also help with workforce management. It can analyze shift data, productivity reports, and even weather patterns to forecast when more or fewer workers will be needed. For example, during seasonal peaks or holidays, it can suggest staffing adjustments to meet demand.
These insights can also help improve employee safety and satisfaction. If the data shows that injury risks increase after long shifts or during late hours, manufacturers can change schedules or provide extra support during those times.
This approach creates a safer, more productive work environment while ensuring resources are used wisely.
Customer Demand and Product Customization
Consumer preferences are always changing. Predictive analytics can analyze customer data, buying trends, and market conditions to forecast future demand. This allows manufacturers to adapt quickly and offer more personalized or in-demand products.
For example, if customers in a particular region are starting to prefer eco-friendly packaging, predictive models can spot this trend early. Manufacturers can then adjust their product design or packaging accordingly, staying ahead of competitors and meeting customer needs.
Final Thoughts
Predictive analytics in manufacturing is no longer just about preventing machine failure. It’s becoming a valuable tool for improving product quality, saving energy, managing inventory, and understanding both employees and customers better.
As more manufacturers embrace this technology, the possibilities continue to grow. What was once a tool for maintenance has now become a key driver of innovation, efficiency, and growth.
The future of data analytics in manufacturing industry lies in its ability to uncover insights across the entire business—from the shop floor to the supply chain to the customer’s hands.
By going beyond maintenance, manufacturers can unlock new opportunities and stay competitive in a fast-changing world.
0 notes
Text
How Artificial Intelligence Will Transform Healthcare by 2025

Key Areas of Transformation
Enhanced Diagnostics AI-powered tools can analyze medical images, detect patterns, and provide faster, more accurate diagnoses for conditions like cancer, heart disease, and neurological disorders.
Personalized Medicine By leveraging patient data, AI can create tailored treatment plans, optimizing medication and care based on individual needs and genetic profiles.
Remote Monitoring and Telehealth AI-enabled wearable devices and telehealth platforms allow for real-time health monitoring, reducing hospital visits and empowering patients to manage their conditions at home.
Predictive Analytics AI algorithms can predict patient outcomes and disease risks, enabling early intervention and preventive care.
Future Prospects By 2025, AI is expected to play a pivotal role in global healthcare, offering solutions that improve accessibility, affordability, and quality of care. While challenges remain, the potential benefits make AI a cornerstone of modern medicine.
Conclusion The transformative power of AI in healthcare is undeniable. As technology advances, embracing these innovations will be crucial in addressing the ever-evolving challenges of patient care. The future of healthcare is smarter, faster, and more patient-centered, thanks to AI.
#Tradegatestore#artificial intelligence in healthcare#AI healthcare innovations#AI in medical diagnosis#personalized medicine with AI#predictive analytics in healthcare#AI-powered healthcare#robotics in surgery#AI telehealth solutions#healthcare technology 2025#future of AI in medicine#AI in patient care
1 note
·
View note
Text
IoT in Action: Transforming Industries with Intelligent Connectivity
The Power of Connectivity
The Internet of Things (IoT) has become a cornerstone of innovation, as it reimagines industries and redefines the way business is conducted. In bridging the physical and digital worlds, IoT enables seamless connectivity, smarter decision-making, and unprecedented efficiency. Today, in the competitive landscape, intelligent connectivity is no longer just a technology advancement; for businesses wanting to be relevant and continue to thrive, it is now a strategic imperative.
IoT is not simply about connecting devices; it’s about creating ecosystems that work collaboratively to drive value. With industries relying heavily on real-time data and actionable insights, IoT-powered connectivity has become the backbone of operational excellence and growth. Let’s explore how this transformative technology is revolutionizing key sectors, with a focus on how businesses can leverage it effectively.
Applications of IoT in Key Industries
1.Smart Manufacturing: Efficiency Through Connectivity
Manufacturing has embraced IoT as a tool to streamline operations and boost productivity. By embedding sensors in machinery and integrating real-time monitoring systems, manufacturers can:
Predict and Prevent Downtime: IoT-enabled predictive maintenance reduces unplanned outages, saving time and money.
Optimize Resource Allocation: Smart systems track inventory, raw materials, and energy consumption, ensuring optimal usage.
Enhance Quality Control: Real-time data from production lines helps identify defects early, maintaining high-quality standards.
Example: A global automotive manufacturer integrated IoT sensors into its assembly lines, reducing equipment downtime by 25% and improving production efficiency by 30%. The ability to monitor machinery health in real time transformed their operations, delivering significant cost savings.
2.Healthcare: Improve Patient Outcomes
In healthcare, IoT has been a game-changer in enabling connected medical devices and systems that enhance patient care and operational efficiency. The main applications include:
Remote Patient Monitoring: Devices track vital signs in real time, allowing healthcare providers to offer timely interventions.
Smart Hospital Systems: IoT-enabled equipment and sensors optimize resource utilization, from patient beds to medical supplies.
Data-Driven Decisions: IoT integrates patient data across systems, providing actionable insights for personalized treatment plans.
Example: A major hospital has put into operation IoT-enabled wearables for chronic disease management. This solution reduced the number of readmissions to hospitals by 20% and empowered patients to take an active role in their health.
3.Retail: Revolutionizing Customer Experiences
IoT is revolutionizing retail through increased customer interaction and streamlined operations. Connected devices and smart analytics allow retailers to:
Personalize Shopping Experiences: IoT systems track customer preferences, offering tailored recommendations in real time.
Improve Inventory Management: Smart shelves and sensors keep stock levels optimal, reducing wastage and improving availability.
Enable Smooth Transactions: IoT-driven payment systems make checkout easier and much faster, increasing customers’ convenience
Example: A retail chain leveraged IoT to integrate smart shelves that automatically update inventory data. This reduced out-of-stock situations by 40%, improving customer satisfaction and driving higher sales.
Role of Intelligent Connectivity in Business Transformation
Intelligent connectivity lies at the heart of IoT’s transformative potential. By connecting devices, systems, and processes, businesses can:
Accelerate Decision-Making: Real-time data sharing enables faster, more informed decisions, giving companies a competitive edge.
It increases collaboration by allowing smooth communication between departments and teams, making the entire system more efficient.
Adapt to Market Dynamics: IoT enables companies to respond quickly to changes in demand, supply chain disruptions, or operational challenges.
Intelligent connectivity is not just about technology; it’s about creating value by aligning IoT solutions with business objectives. This strategic approach guarantees that IoT investments will deliver measurable outcomes, from cost savings to improved customer loyalty.
How Tudip Technologies Powers Intelligent Connectivity
Tudip Technologies specializes in designing and implementing IoT solutions that drive meaningful transformation for businesses. With a focus on innovation and collaboration, Tudip ensures that its clients achieve operational excellence through intelligent connectivity.
Tailored Solution for Every Business Industry
Tudip understands that no two businesses are alike. By customizing IoT strategies to address specific challenges, Tudip helps clients unlock the full potential of connectivity. Examples include:
Smart Supply Chains: Implementing IoT systems that provide real-time visibility into inventory and logistics, reducing delays and improving efficiency.
Energy Management: Developing IoT frameworks to monitor and optimize energy usage, driving sustainability and cost savings.
Healthcare Innovations: Designing networked medical devices that allow remote patient monitoring and data integration without a hitch.
The Future of Connected Systems
The demand for intelligent connectivity will keep increasing as the industries continue to evolve. Emerging trends in IoT include edge computing, 5G networks, and AI-powered analytics, which promise to redefine possibilities for connected ecosystems.
Businesses that embrace these advancements stand to gain:
Greater Resilience: IoT enables adaptive systems that can withstand market fluctuations and operational challenges.
Enhanced Innovation: Connected technologies open doors to new business models, revenue streams, and customer experiences.
Sustainable Growth: IoT optimizes resources and processes, contributing to long-term environmental and economic sustainability.
The future belongs to those who see connectivity not just as a technological tool but as a strategic enabler of transformation. The right partner will help businesses transform IoT from a concept into a competitive advantage.
Conclusion: Embracing Intelligent Connectivity with Tudip
IoT is not just changing the way businesses operate—it’s redefining what’s possible. From manufacturing and healthcare to retail and beyond, intelligent connectivity is driving innovation, efficiency, and growth across industries.
Tudip Technologies is at the forefront of this transformation, offering customized IoT solutions that deliver real results. By prioritizing collaboration, adaptability, and measurable outcomes, Tudip ensures that its clients stay ahead in an increasingly connected world.
Now is the time to embrace the power of IoT and unlock its potential for your business. With Tudip as your partner, the journey to intelligent connectivity is not just achievable—it’s inevitable.
Click the link below to learn more about the blog IoT in Action: Transforming Industries with Intelligent Connectivity https://tudip.com/blog-post/iot-in-action-transforming-industries-with-intelligent-connectivity/
#Tudip#IoT#intelligent connectivity#real-time data#predictive maintenance#smart manufacturing#remote patient monitoring#healthcare IoT#retail IoT#smart shelves#supply chain optimization#edge computing#AI-powered analytics#5G networks#industrial IoT#connected devices#digital transformation#operational efficiency#business intelligence#automation#data-driven decision-making#IoT solutions#smart systems#enterprise IoT#IoT-powered connectivity#sustainable growth#technology innovation#machine learning#cloud computing#smart sensors
0 notes
Text
How Artificial Intelligence is Transforming Business
Introduction:
As businesses strive to stay competitive in today's rapidly evolving market landscape, Artificial Intelligence (AI) solutions have become invaluable assets. AI is revolutionizing the business world, with companies innovating to maintain their edge. By leveraging AI, businesses can generate significant value through predictive analytics, task automation, and data analysis. Implementing AI applications grants enterprises enhanced access to data across various functions, including customer relationship management, enterprise resource planning, fraud detection, finance, human resources, and IT management. This comprehensive technology suite is reshaping the business industry.
What is AI and How Does It Work?
Artificial Intelligence (AI) encompasses machine learning techniques designed to address problems traditionally requiring human intelligence, such as pattern recognition and decision-making. Initially developed to assist humans with complex tasks, AI now spans a broad spectrum—from simple automation to sophisticated learning algorithms. Today, AI is utilized across various sectors, including retail, customer service, manufacturing, and logistics.
Solving real-world challenges using Computer Vision (CV) and Digital Image Processing (DIP) is pivotal in the current landscape. Processing images and videos from surveillance systems to extract summarized outputs for critical decision-making exemplifies this focus, particularly in image and video analytics. Advancements in Natural Language Processing (NLP) within AI research have led to innovative solutions enhancing human life quality. Technologies such as conversational AI chatbots, digital assistants, self-driving vehicles, and fraud detection systems are now integral to various industries.
There are tremendous opportunities in the market for AI based solutions because machines are good at performing repetitive and tedious tasks without making errors. Thus, making it a Robust technology, which has specific USP’s
Unique Customer Experience
Precise Outcome
Streamline Operational Tasks
State of the Art performance
Customized algorithms for object detection
Wide range of applications using the end output

Potential Benefits of Artificial Intelligence in Business
For businesses considering AI adoption, the potential benefits are extensive. AI can enhance profitability, drive innovation, and create new growth opportunities.
Improved Decision-Making: Artificial Intelligence in business enables companies to make faster and more accurate decisions by analyzing large data sets and identifying patterns. By leveraging AI transformation, businesses can better identify AI trends, predict customer behavior, and optimize marketing strategies. This enhances AI decision-making, leading to improved customer relations and higher sales.
Enhanced Customer Service: AI in customer service, such as Conversational AI chatbots and virtual assistants, ensures quick and consistent support 24/7. This results in faster issue resolution, higher customer satisfaction, and improved retention rates, contributing to the AI economic impact.
Efficiency and Productivity Gains: AI automates time-consuming tasks like data entry and report generation, boosting AI operational efficiency. By streamlining processes, businesses can enhance productivity and focus on strategic goals, making operations more effective. Notably, AI is projected to improve employee productivity by up to 40% by 2035.
Personalized Shopping Experiences: AI enables businesses to upsell and cross-sell by analyzing customer data and tailoring experiences to individual preferences. This strengthens customer relationships, builds loyalty, and maximizes revenue opportunities. For example, Netflix's AI-driven recommendation system contributes approximately $1 billion in annual revenue by personalizing content for users.
Improved Quality and Reduced Human Error: AI enhances quality control, reduces defects, and provides employees with insightful feedback, helping businesses maintain high standards and minimize human error.
Increased Forecasting Accuracy: AI helps businesses identify hidden patterns in data, improving forecasting in sales, marketing, supply chain management, and inventory planning. This enhances decision-making and drives business growth.
Read Also: The Benefits of Adding Conversational AI Chatbot to Your E-commerce Store During Festive Seasons
Conclusion & Future Outlook of AI in Business Today
As AI technologies continue to advance, businesses are increasingly adopting AI to enhance efficiency and effectiveness. AI facilitates process automation, improved customer service, and informed decision-making. From chatbots to predictive analytics, AI is reshaping the contemporary business landscape. Hire the best AI Experts from Rydot Infotech!
Looking ahead, AI's role in business is poised to expand. As AI technologies become more sophisticated, companies can leverage their capabilities to gain a competitive advantage. The coming years are expected to witness a surge in AI-driven services and products, making it an opportune time for businesses to embrace AI.
For expert guidance on integrating AI into your business, consider consulting with AI specialists to navigate this transformative journey effectively.
#Artificial Intelligence#AI solutions#AI in business#AI applications#machine learning#predictive analytics
0 notes
Text
Case Study: Predicting Equipment Failure Using Vibration & Wear Data
Background: An international manufacturing company, operating across multiple locations, faced significant challenges in predicting equipment failures. Despite having access to extensive data from equipment sensors, ERP systems, IoT devices, and third-party vendor systems, the company struggled to accurately forecast when equipment would fail due to vibration and associated wear. This lack of predictive maintenance capabilities led to unexpected downtime, increased maintenance costs, and potential disruptions in production. The company sought a more intelligent solution to leverage its existing data and improve its maintenance strategies.
Problem: International Manufacturing has data from equipment, ERP, IoT and from 3rd party vendor systems, but did not have a good sense of when equipment would fail due to vibration and associated wear data.
Objectives:
1. Collect and analyze sensor data (vibration, wear, etc.) to detect early signs of equipment failure. 2. Implement predictive maintenance to prevent unexpected breakdowns. 3. Enhance equipment reliability and extend machinery lifespan.
Solution:
1. Data Collection:
- Sensors and IoT Devices: Use sensor data from critical equipment to monitor vibration, temperature, wear, and other relevant metrics. - Data Logging: Use IoT platforms to collect and store real-time sensor data for analysis (use available data).
2. Data Preprocessing:
- Cleaning and Filtering: Remove noise and irrelevant data from the sensor readings. - Normalization: Standardize data to ensure consistency and accuracy in analysis.
3. Feature Extraction:
- Time-Series Analysis: Extract features from the time-series data, such as mean, standard deviation, and frequency components. - Anomaly Detection: Identify patterns and anomalies that indicate potential equipment failure.
4. Predictive Modeling:
- Machine Learning Algorithms: Use supervised learning algorithms (e.g., Random Forest, SVM) to train predictive models on historical data. - AI Agent: Implement deep learning models (e.g., LSTM, CNN) for more complex pattern recognition and prediction with an AI Agent.
5. Model Training and Evaluation:
- Training: Train the models using historical failure data and sensor readings. - Evaluation: Evaluate model performance using metrics like accuracy, precision, recall, and F1 score.
6. Real-Time Monitoring and Prediction:
- Deployment: Deploy the predictive models in a real-time monitoring system. - Alerts and Notifications: Set up automated alerts for maintenance teams when the model predicts potential failures.
7. Maintenance Scheduling:
- Dynamic Scheduling: Adjust maintenance schedules based on prediction results to address potential issues before they lead to failures. - Resource Allocation: Optimize the allocation of maintenance resources based on predicted needs.
8. Reporting and Analysis:
- Dashboards: Develop dashboards to visualize sensor data, predictions, and maintenance schedules. - Continuous Improvement: Use feedback from maintenance activities to continually improve model accuracy and reliability.
Business Insights:
Vibration Anomaly: AI detects an unusual vibration pattern in a critical machine, predicting a potential bearing failure within the next week. An alert is sent to the maintenance team for immediate inspection.
Wear Analysis: Sensor data shows accelerated wear on a component. Predictive models suggest replacing the component during the next scheduled maintenance to avoid unplanned downtime.
Temperature Spike: An increase in temperature readings indicates a potential overheating issue. The maintenance team is alerted to check the cooling system before it fails.
Outcomes & Results:
- Reduced unplanned downtime and extended equipment lifespan. - Lower maintenance costs due to timely interventions. - Enhanced operational efficiency with reliable and well-maintained machinery.
Conclusion:
The implementation of a predictive maintenance solution using vibration and wear data has significantly improved the company's ability to manage equipment reliability. By leveraging advanced data collection, preprocessing, and AI algorithms, the company achieved remarkable results:
•Reduced Unplanned Downtime: The solution led to a 35% reduction in unplanned downtime by accurately predicting equipment failures and enabling timely interventions. •Extended Equipment Lifespan: Predictive maintenance extended the lifespan of critical machinery by 25%, reducing the need for frequent replacements. •Lower Maintenance Costs: Timely interventions and optimized maintenance schedules resulted in a 20% reduction in overall maintenance costs. •Enhanced Operational Efficiency: Real-time monitoring and predictive modelling improved operational efficiency by 30%, ensuring smooth and uninterrupted production processes. •Improved Decision-Making: The implementation of real-time alerts and detailed dashboards enabled better decision-making and proactive management, leading to a 15% increase in overall operational efficiency.
Overall, the intelligent solution not only streamlined the company's maintenance processes but also provided valuable business insights that supported continuous improvement and strategic planning. The company is now better equipped to predict and prevent equipment failures, ensuring reliable and efficient operations across its multiple locations.
Ready to Unlock the Full Power of Your Data?
Learn more about DataPeak:
#agentic ai#ai#artificial intelligence#machine learning#saas#technology#DataPeak#FactR#ai solutions for data driven decision making#datadrivendecisions#dataanalytics#data driven decision making#digital transformation#digital trends#cloud computing#SMBs#smbsuccess#predictive analytics tools#machine learning for workflow#AI-driven business solutions#no code
0 notes
Text
The Need for Predictive Analytics Services
Forecasting industry trends is vital to enterprise risk management and service innovation. After all, consumers in different markets respond to each product idea in unique ways. Meanwhile, specific macroeconomic conditions might favor a brand in one business area, but social stability concerns might make its market penetration strategy less viable. Therefore, managers and business leaders must leverage predictive analytics services and relevant insights into customers' behavioral patterns. Doing so will increase the success rate of growth strategies.
0 notes
Text
Consulting and solutions for predictive analytics - Predictive analytics services enable you to proactively address unforeseen difficulties and make wise choices. We are a dependable partner for combining ML & AI with predictive analytics to advance your company.
#predictive analytics services#predictive analytics consulting#predictive analytics solutions#predictive analytics consulting services
0 notes
Text
Ultimate Guide to DeepSeek AI for Business Growth
Table of Contents of DeepSeek AI for Business Growth1. Introduction: Why AI is Essential for Modern Business Growth2. What Is DeepSeek AI?3. Top 5 DeepSeek AI Tools for Scaling Businesses3.1 Demand Forecasting Engine3.2 Customer Lifetime Value (CLV) Predictor3.3 Automated Supply Chain Optimizer3.4 Dynamic Pricing Module3.5 Sentiment Analysis Hub4. How DeepSeek AI Reduces Costs and Boosts…
#AI automation 2024#AI budgeting#AI business growth#AI for non-tech teams#AI for startups#AI implementation guide#AI in retail#AI supply chain#Business Intelligence#cost reduction strategies#data-driven decisions#DeepSeek AI#enterprise AI adoption#fintech AI solutions#generative AI for business#Predictive Analytics#ROI optimization#scaling with AI#SME AI tools#startup scaling
2 notes
·
View notes
Text
How AI Is Revolutionizing Contact Centers in 2025
As contact centers evolve from reactive customer service hubs to proactive experience engines, artificial intelligence (AI) has emerged as the cornerstone of this transformation. In 2025, modern contact center architectures are being redefined through AI-based technologies that streamline operations, enhance customer satisfaction, and drive measurable business outcomes.
This article takes a technical deep dive into the AI-powered components transforming contact centers—from natural language models and intelligent routing to real-time analytics and automation frameworks.
1. AI Architecture in Modern Contact Centers
At the core of today’s AI-based contact centers is a modular, cloud-native architecture. This typically consists of:
NLP and ASR engines (e.g., Google Dialogflow, AWS Lex, OpenAI Whisper)
Real-time data pipelines for event streaming (e.g., Apache Kafka, Amazon Kinesis)
Machine Learning Models for intent classification, sentiment analysis, and next-best-action
RPA (Robotic Process Automation) for back-office task automation
CDP/CRM Integration to access customer profiles and journey data
Omnichannel orchestration layer that ensures consistent CX across chat, voice, email, and social
These components are containerized (via Kubernetes) and deployed via CI/CD pipelines, enabling rapid iteration and scalability.
2. Conversational AI and Natural Language Understanding
The most visible face of AI in contact centers is the conversational interface—delivered via AI-powered voice bots and chatbots.
Key Technologies:
Automatic Speech Recognition (ASR): Converts spoken input to text in real time. Example: OpenAI Whisper, Deepgram, Google Cloud Speech-to-Text.
Natural Language Understanding (NLU): Determines intent and entities from user input. Typically fine-tuned BERT or LLaMA models power these layers.
Dialog Management: Manages context-aware conversations using finite state machines or transformer-based dialog engines.
Natural Language Generation (NLG): Generates dynamic responses based on context. GPT-based models (e.g., GPT-4) are increasingly embedded for open-ended interactions.
Architecture Snapshot:
plaintext
CopyEdit
Customer Input (Voice/Text)
↓
ASR Engine (if voice)
↓
NLU Engine → Intent Classification + Entity Recognition
↓
Dialog Manager → Context State
↓
NLG Engine → Response Generation
↓
Omnichannel Delivery Layer
These AI systems are often deployed on low-latency, edge-compute infrastructure to minimize delay and improve UX.
3. AI-Augmented Agent Assist
AI doesn’t only serve customers—it empowers human agents as well.
Features:
Real-Time Transcription: Streaming STT pipelines provide transcripts as the customer speaks.
Sentiment Analysis: Transformers and CNNs trained on customer service data flag negative sentiment or stress cues.
Contextual Suggestions: Based on historical data, ML models suggest actions or FAQ snippets.
Auto-Summarization: Post-call summaries are generated using abstractive summarization models (e.g., PEGASUS, BART).
Technical Workflow:
Voice input transcribed → parsed by NLP engine
Real-time context is compared with knowledge base (vector similarity via FAISS or Pinecone)
Agent UI receives predictive suggestions via API push
4. Intelligent Call Routing and Queuing
AI-based routing uses predictive analytics and reinforcement learning (RL) to dynamically assign incoming interactions.
Routing Criteria:
Customer intent + sentiment
Agent skill level and availability
Predicted handle time (via regression models)
Customer lifetime value (CLV)
Model Stack:
Intent Detection: Multi-label classifiers (e.g., fine-tuned RoBERTa)
Queue Prediction: Time-series forecasting (e.g., Prophet, LSTM)
RL-based Routing: Models trained via Q-learning or Proximal Policy Optimization (PPO) to optimize wait time vs. resolution rate
5. Knowledge Mining and Retrieval-Augmented Generation (RAG)
Large contact centers manage thousands of documents, SOPs, and product manuals. AI facilitates rapid knowledge access through:
Vector Embedding of documents (e.g., using OpenAI, Cohere, or Hugging Face models)
Retrieval-Augmented Generation (RAG): Combines dense retrieval with LLMs for grounded responses
Semantic Search: Replaces keyword-based search with intent-aware queries
This enables agents and bots to answer complex questions with dynamic, accurate information.
6. Customer Journey Analytics and Predictive Modeling
AI enables real-time customer journey mapping and predictive support.
Key ML Models:
Churn Prediction: Gradient Boosted Trees (XGBoost, LightGBM)
Propensity Modeling: Logistic regression and deep neural networks to predict upsell potential
Anomaly Detection: Autoencoders flag unusual user behavior or possible fraud
Streaming Frameworks:
Apache Kafka / Flink / Spark Streaming for ingesting and processing customer signals (page views, clicks, call events) in real time
These insights are visualized through BI dashboards or fed back into orchestration engines to trigger proactive interventions.
7. Automation & RPA Integration
Routine post-call processes like updating CRMs, issuing refunds, or sending emails are handled via AI + RPA integration.
Tools:
UiPath, Automation Anywhere, Microsoft Power Automate
Workflows triggered via APIs or event listeners (e.g., on call disposition)
AI models can determine intent, then trigger the appropriate bot to complete the action in backend systems (ERP, CRM, databases)
8. Security, Compliance, and Ethical AI
As AI handles more sensitive data, contact centers embed security at multiple levels:
Voice biometrics for authentication (e.g., Nuance, Pindrop)
PII Redaction via entity recognition models
Audit Trails of AI decisions for compliance (especially in finance/healthcare)
Bias Monitoring Pipelines to detect model drift or demographic skew
Data governance frameworks like ISO 27001, GDPR, and SOC 2 compliance are standard in enterprise AI deployments.
Final Thoughts
AI in 2025 has moved far beyond simple automation. It now orchestrates entire contact center ecosystems—powering conversational agents, augmenting human reps, automating back-office workflows, and delivering predictive intelligence in real time.
The technical stack is increasingly cloud-native, model-driven, and infused with real-time analytics. For engineering teams, the focus is now on building scalable, secure, and ethical AI infrastructures that deliver measurable impact across customer satisfaction, cost savings, and employee productivity.
As AI models continue to advance, contact centers will evolve into fully adaptive systems, capable of learning, optimizing, and personalizing in real time. The revolution is already here—and it's deeply technical.
#AI-based contact center#conversational AI in contact centers#natural language processing (NLP)#virtual agents for customer service#real-time sentiment analysis#AI agent assist tools#speech-to-text AI#AI-powered chatbots#contact center automation#AI in customer support#omnichannel AI solutions#AI for customer experience#predictive analytics contact center#retrieval-augmented generation (RAG)#voice biometrics security#AI-powered knowledge base#machine learning contact center#robotic process automation (RPA)#AI customer journey analytics
0 notes
Text
"Economy Redefined: Bridgera's Support Makes Predictive Maintenance Implementations Easier"
Suppose that each lost minute in your operations is costing thousands. "Predictive maintenance," fueled by "IoT" and "advanced analytics," is set to revolutionize asset management and operational effectiveness. However, the path ahead is not free of hurdles. With total assistance from Bridgera and the industry's top-of-the-line "IoT solutions," the implementation is as seamless as a drive to the future of "predictive maintenance" with the least trouble or disruption.
#iot#iot solutions#iot platform#iot integration#iot development#iot asset tracking#iot software development services#predictive maintenance#advanced analytics
0 notes