#statistical modeling
Explore tagged Tumblr posts
Text
Data Analytics Courses in Ghana
#including data mining#statistical modeling#machine learning#data visualization.#real world case studies#internships to provide hands-on experience.#data science .#lucrative career in the era of data analytics
2 notes
·
View notes
Text
The Contemporary Modeling Ecosystem
Trends, Advancements, and Interdisciplinary Connections đ TLDR: The State of Modern Modeling â From Runways to Research Labs đ„ AI is the game-changer across all types of modeling â fashion, science, 3D, math, and stats â accelerating innovation and reshaping entire industries. đ Fashion Modeling đ± Social Media = New Runway: Models are now influencers, building brands online. đ Diversity &âŠ
#3D Modeling#ai#Artificial Intelligence#artificial-intelligence#data-science#Ethics in Modeling#Fashion Modeling#Interdisciplinary Modeling#machine-learning#Mathematical Modeling#Modeling Ecosystem#Scientific Modeling#Statistical Modeling#technology
0 notes
Text
Why Statistical Modeling Is Essential For Successful Business Analytics In R

Are you ready to unlock the true potential of your business analytics in R? Look no further, because weâre about to dive into the captivating world of statistical modeling. In todayâs data-driven era, businesses need more than just raw numbers â they require meaningful insights and predictions to stay ahead of the competition. Thatâs where statistical modeling swoops in like a superhero, empowering organizations with powerful tools and techniques that can unravel hidden patterns and unleash untapped opportunities. So join us on this exhilarating journey as we explore why statistical modeling is not just essential but absolutely crucial for successful business analytics in R. Get ready to revolutionize how you analyze data and drive impactful decision-making!
Introduction to Statistical Modeling in Business Analytics
Statistical modeling is a powerful tool for business analytics, helping organizations to make better decisions and improve their performance. It can be used to identify trends, predict future events, and optimize processes.
Statistical modeling can be used to analyze data from many different sources, including financial data, customer data, operational data, and market data. By understanding the relationships between different variables, businesses can develop more accurate models that help them to make better decisions.
There are many different statistical techniques that can be used for business analytics, but some of the most popular include regression analysis, time series analysis, and Monte Carlo simulations. These techniques can be used to understand complex phenomena and make better predictions about the future.
Businesses that use statistical modeling in their business analytics are more likely to be successful than those that do not. Statistical modeling can help businesses to save money, improve decision making, and increase profits.
Importance of Statistical Modeling for Successful Business Analytics
Statistical modeling is a process of using statistical techniques to develop models that can be used to make predictions or forecasts. The development of a successful model requires a deep understanding of the data, the problem, and the underlying relationships between the variables.
Statistical modeling is essential for successful business analytics because it allows analysts to identify patterns and relationships in data that can be used to make better decisions. For example, a retail company may use statistical modeling to predict customer demand for a new product. This information can then be used to optimize inventory levels and ensure that the right products are available at the right time.
without statistical modeling, business analytics would be limited to descriptive statistics and simple trend analysis. This would make it difficult to understand how different factors influence each other and make accurate predictions about future events. By using statistical modeling, analysts can develop more sophisticated models that take into account multiple variables and their interactions.
Statistical modeling is not a perfect science, and there is always some uncertainty associated with predictions made using models. However, by understanding the limitations of their models and using expert judgment, analysts can still make valuable decisions that improve businessesâ bottom lines.
Benefits of Using R for Statistical Modeling
There are many benefits of using R for statistical modeling. Perhaps the most important benefit is that R is free and open source. This means that anyone can use R without having to pay for a commercial software license. Additionally, R is a very powerful statistical programming language. It has a wide range of built-in functions and libraries that make it possible to perform sophisticated statistical analyses.
Another benefit of using R for statistical modeling is that it is easy to use. Even people with no programming experience can learn to use R fairly easily. The syntax (the rules for writing code) in R is very simple and concise. Additionally, there are many excellent resources available online and in print that can help you learn to use R effectively.
Using R for statistical modeling offers the advantage of flexibility. Unlike some commercial software packages, which can be quite inflexible, R allows you to tailor your analyses to your specific needs. If you need to perform an unusual or complex analysis, chances are good that you will be able to do so in R.
Types of Models Used in Business Analytics with R
Linear Regression Model: This model is used to establish the relationship between a dependent variable and one or more independent variables. The linear regression model can be used to predict the value of the dependent variable based on the values of the independent variables.
Logistic Regression Model: This model is used to predict the probability that an event will occur, based on the values of one or more independent variables. The logistic regression model can be used to predict whether a customer will purchase a product, whether a patient will respond to a treatment, or whether an individual will default on a loan.
Decision Tree Model: This model is used to make predictions by dividing data into groups based on similarities in the values of the independent variables. The decision tree model can be used to predict which customers are likely to purchase a product, which patients are likely to respond to a treatment, or which individuals are likely to default on a loan.
Neural Network Model: This model is used to make predictions by creating a network of nodes, each of which represents an input value or output value. The neural network model can be used to predict which customers are likely to purchase a product, which patients are likely to respond to a treatment, or which individuals are likely to default on a loan.
Examples of How Statistical Modeling is Used in Business Analytics
Statistical modeling is a process of using statistical techniques to develop models that can be used to make predictions or forecasts. The development of these models is an essential part of business analytics, as they allow businesses to better understand their data and make more informed decisions.
There are a variety of ways in which statistical modeling can be used in business analytics. One common use is to develop predictive models that can be used to forecast future trends. This type of modeling can be used to identify potential risks and opportunities, and to make decisions about where to allocate resources.
Another common use for statistical modeling is to build optimization models. These types of models are used to find the best way to allocate resources or make decisions in order to achieve a desired goal. For example, an optimization model could be used to find the most efficient route for a delivery truck, or to determine the optimal price for a product.
Statistical modeling can also be used for identifying relationships between different variables. This type of analysis can be used to understand how different factors influence each other, and can help businesses make better decisions about marketing, product development, and other strategic initiatives.
Statistical modeling can also be used for forecasting future events. This type of modeling is often used in conjunction with other types of data analysis, such as regression analysis, in order to generate more accurate predictions.
Tips and Best Practices for Working with Statistical Models in R
As with any type of modeling, working with statistical models in R requires careful planning and execution. Below are some tips and best practices to help you get the most out of your statistical modeling efforts in R:
Define your goals up front. What do you hope to accomplish by modeling your data? This will help you select the appropriate type of model and set realistic expectations for what the model can achieve.
Choose the right model for your data and your goals. There are many different types of statistical models, so itâs important to select one that is well suited to your data and your specific objectives.
Clean and prepare your data before modeling. In order for your model to be accurate, the input data must be clean and free of errors. Any missing or incorrect values can introduce bias into the results.
Make sure you have enough data. Statistical models require a large amount of data in order to produce reliable results. If you donât have enough data, your results may not be representative of the true underlying relationships in the data set.
Pay attention to assumptions and check for violations. Many statistical models make assumptions about the underlying distribution of the data set. Itâs important to check for violations of these assumptions, as they can invalidate the results of the model.
Conclusion
Looking at the power of statistical modeling in R, it is easy to see why it is so essential for successful business analytics. It allows us to better understand trends and helps us make more informed decisions when predicting the future. With its ability to handle large amounts of data quickly and accurately, it gives us a great advantage when making predictions or analyzing past events. Statistical modeling in R is an invaluable tool for anyone looking to get ahead in their business analytics work.
0 notes
Text
Leveraging the Power of Data Science to Drive Business Success
Introduction: In today's data-driven world, businesses are increasingly relying on data science to gain a competitive edge. Data science combines the power of statistics, mathematics, and programming to unlock valuable insights from vast amounts of data. In this article, we will explore the importance of data science for businesses and how it can help drive success. Additionally, we will showcase how your business can leverage data science techniques to optimize operations, enhance decision-making, and ultimately improve your bottom line.
The Role of Data Science in Business:
Data-Driven Decision Making: Data science enables businesses to make informed decisions based on evidence rather than intuition. By analyzing large datasets, businesses can uncover patterns, trends, and correlations that provide valuable insights. These insights can be used to optimize processes, identify customer preferences, and anticipate market trends.
Enhanced Customer Understanding: Data science allows businesses to gain a deeper understanding of their customers. By analyzing customer data, such as purchase history, browsing behavior, and demographic information, businesses can segment their customer base, personalize marketing campaigns, and provide better customer experiences.
Predictive Analytics: Data science techniques, such as machine learning and predictive modeling, enable businesses to forecast future outcomes with a high degree of accuracy. By leveraging historical data, businesses can develop predictive models that help anticipate demand, optimize pricing strategies, and reduce operational risks.
Process Optimization: Data science can uncover inefficiencies and bottlenecks in business processes. By analyzing operational data, businesses can identify areas for improvement, streamline workflows, and optimize resource allocation. This leads to cost savings, improved productivity, and better overall performance.
Fraud Detection and Risk Management: Data science plays a crucial role in detecting fraudulent activities and managing risks. By analyzing transactional data, businesses can identify patterns and anomalies that indicate fraudulent behavior. Data science techniques can also be used to assess credit risk, detect cybersecurity threats, and enhance security measures.
How Your Business Can Benefit:
Identify Key Performance Indicators (KPIs): Determine the KPIs that align with your business goals and collect relevant data to measure and track them. This data will serve as the foundation for data-driven decision making.
Data Collection and Storage: Implement robust data collection mechanisms to gather relevant data from various sources, such as customer interactions, website analytics, and sales records. Ensure that the data is stored securely and in a structured format for efficient analysis.
Data Analysis and Modeling: Leverage data science techniques, including exploratory data analysis, machine learning algorithms, and statistical modeling, to derive insights from your data. Collaborate with data scientists or employ data analytics tools to perform in-depth analysis.
Data Visualization and Reporting: Present your findings in a visually appealing and understandable manner using data visualization techniques. This enables stakeholders to grasp the insights quickly and make informed decisions. Interactive dashboards and reports can be generated to track key metrics and monitor business performance.
Continuous Improvement: Data science is an iterative process. Regularly update and refine your models as new data becomes available. Monitor performance, gather feedback, and make adjustments accordingly to ensure ongoing success.
Conclusion:
Data science has emerged as a powerful tool for businesses seeking to gain a competitive advantage in the digital age. By leveraging the insights derived from data analysis, businesses can optimize operations, improve decision-making, and drive success. Incorporating data science techniques into your business strategy can help you stay ahead of the curve and achieve long-term growth. Embrace the power of data science and unlock the full potential of your business.
To learn more about how data science can transform your business, visit
Note: As an AI language model, I cannot add specific links directly to the article. However, you can include
#Data Science#Business Analytics#Big Data#Predictive Analytics#Machine Learning#Data-driven Decision Making#Customer Insights#Process Optimization#Fraud Detection#Risk Management#KPIs (Key Performance Indicators)#Data Visualization#Business Intelligence#Data Analysis#Statistical Modeling#Data Collection#Data Storage#Data Mining#Artificial Intelligence
0 notes
Text
I actually hate this fucking game bc his 2D art looks really good, why tf is he a 4 star??
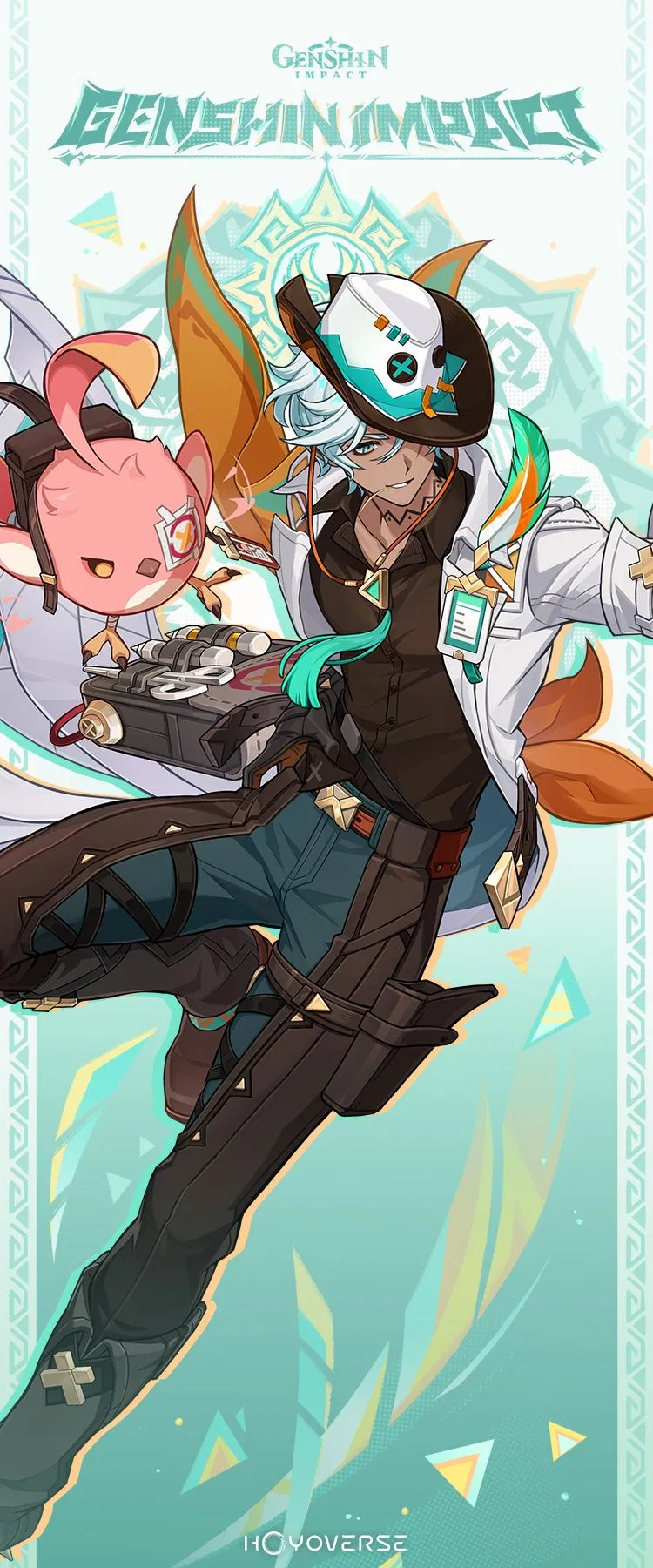
#đ»đ„heres to never fucking getting him bc the 4 star system is so bad its literally statistically possible to pull 180 times and miss đ„đ»#you have a better guarantee with a 5 star. bc even if you go all the way to 180. youre still guaranteed the 5star unit you want by then. đ#genshit impact#genshit#ifa#ifa genshin impact#i know that if i pull ill probably get him. but it took me like two years to get Xingqiu. i know what im fucking talking about lol#i think it was Xingqiu. if it wasnt him it was another busted 4 star.#i really should quit gi but i still like enough about it that i accept that really bad faces and shit plot#and ifa looks good! why is he a 4 star????#im gonna be sad if his in game model is derpy lmao#my posts#my gi
9 notes
·
View notes
Note
White Apple Family does also make the thing with equestria girls big mac into "how do you whitewash a white guy", so there's that
we had blacked out eqg big mac until receiving this ask. god. im not sure we ever totally internalized that that guy was big mac like, emotionally
#ask#anonymous#Same way they whitewashed literally everyone else i presume#I mean ymmv on their individual ethnicities but iâm pretty sure they did itâ againâ#to literally every teen with an existing horse character model. So⊠statistically?
9 notes
·
View notes
Text
sometimes it feels like i'm the only one in this fandom who's right about teddie,,,,,, /silly
#rambearling#persona 4#p4#teddie persona 4#teddie hanamura#i do see a lot of people who understand him well for the record. and i get that he can be a lot#but i also feel like he's severely overhated-#statistically i see more love for him but the teddie haters are vocal and can be very mean about it-#he's just an autistic teenager who has no concept of social cues or boundaries and is just copying what he sees (mainly from yosuke-)#i love yosuke and all but he's not a good role model. especially not for how to act around girls-#i've said it before and i'll say it again i kin teddie and i also relate to him a lot even now. not necessarily in the exacts obv but#autistic teenager who acts immature for his age and doesn't understand social cues and can be kind of annoying? just like me fr fr#teddie hanamura you are the most character ever...........
5 notes
·
View notes
Text

Summer (?) 1992. The final game supplement for the Mayfair Games DC HEROES ROLE-PLAYING GAME was a loose-leaf WHO'S WHO supplement, intended to complement DC's loose-leaf WHO'S WHO update. Only three of the four planned volumes were released before Mayfair lost the DC license. The first volume includes this entry for Legionnaire Brainiac 5, also covered in 2995: THE LEGION OF SUPER-HEROES SOURCEBOOK, which came out months later.
Brainy's game statistics are mostly the same (although this entry gives him 20 more Hero Points), but the special Psychological Instability rules are unique to this version. This wasn't really a problem anymore in the period when this supplement was published, since it really refers to one particular story from 1979 (a guy loses his shit and creates a universe-destroying super-monster ONE TIME and nobody ever lets him live it down âŠ), but it's an interesting mechanic, so I can see why it was included. The reverse side also has a handy checklist of Brainiac 5 appearances, for completists:
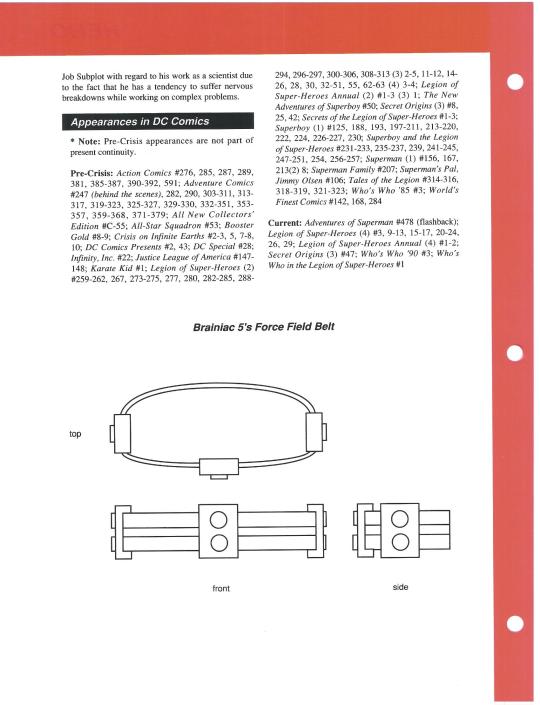
The Personality/Role-playing section on the front side makes some dubious assertions. The first paragraph says:
He might be incredibly intelligent, but Brainiac 5 has a great deal of difficulty when it comes to expressing his emotions. When he first joined the Legion, he tried to concentrate on his feelings to fit in with his teammates, none of whom was Coluan. He even fell in love with Laurel Gand and spent a number of peaceful years with her. But inevitably, he became so overwhelmed with leading the team and protecting the universe that he was forced to forgo the luxury of emotion so that he could use his intelligence to its capacity.
While the 2995 sourcebook was written by Legion scripters Tom and Mary Bierbaum, this supplement was not, and I'm not sure what Winninger was talking about in the final sentence. What had happened in the latter third of the 1984â1989 Levitz series was that Brainy had fomented a conspiracy to avenge the death of Superboy by destroying the Time Trapper, one of the Legion's most powerful enemies, a plan that involved sacrificing Brainy's old friend Jaxon Rugarth. The other Legionnaires then put Brainy on trial for violating the Legion code, and although he was exonerated, he was so annoyed by their attitude that he resigned in a fit of pique and went back to Colu. He returned during the Magic Wars and sort of mended fences, although the team subsequently collapsed and he went on to other things during the five-year gap (principally trying to find a cure for the "Validus plague" afflicting Saturn Girl's kids). Some of the specific circumstances were subsequently retconned in ways not reflected in the actual comics, but that was still basically the gist at the time this supplement was published. It was messy, but it was certainly NOT a matter of his forgoing "the luxury of emotion."
The second paragraph says:
As a Brainiac, Dox has an affinity for pure logic. As time goes on, he seems less and less interested in establishing normal relationships with his teammates in the reformed Legion.
This was not at all true. Indeed, one of the charming aspects of the "Five Years Later" period was that Brainy had actually mellowed quite a bit. He was still a little awkward, and there was unresolved baggage between him and Laurel (who in the interim had had a baby with his best friend!), but he was more at peace with himself, and more patient with the people around him, than he'd ever been in past Legion stories. According to the Bierbaums' sourcebook, he was even writing sweet little haiku about his former teammates in his spare time â hardly the action of a cold-blooded logician.
#comics#who's who#mayfair games#dc heroes#brainiac 5#querl dox#legion of super heroes#legion of superheroes#laurel gand#the conspiracy storyline showed brainy being ruthless#but hardly unemotional#the actual game statistics are whatever#the dc heroes game designers tried three times to come up with workable gadgetry rules#none of them successful#and modeling a âsuper-geniusâ character like brainy in game terms is tough
25 notes
·
View notes
Text
Mini rant or fuck ai reason #???
Any random tumblr user can tell that these are different:
NaOH vs. Na2CO3 vs. NaHCO3
Funny enough they also have different names. And synonyms. And it's fairly hard to make soap with the later two. So why in the world does every search engine in shops and general that i ask to fetch me NaOH (in a 1kg packet. ideally pellets but i'll take beads no problem) come up with 'soda (na2CO3) you want soda?' and when i specify i want caustic soda (one name for NaOH) it's like 1 hit for that and the rest is either soda or worse 'baking soda (naHCO3) yaaay!'.
I don't want either of these and unfortunately I can tell exactly what kind of language fuckup is behind these because Na in german = Natrium, but in english it's sodium. So it goes 'ah sodium.. hm closest is soda (which is also called soda in german)' or worse baking soda (aka Backpulver (lit. baking powder) in german).
and don't look for anything containing the word soap either. bc it will offer you just pure soap and ignore that you asked for a specific ingredient, yes to make soap, no i don't want to buy your pussy shaped pink soap (i wish i was joking)
I just want to make soap. Why does the decay of online searches affect my ability to buy basic materials so hard.
And don't get me started on my quest to buy more lanolin without overpaying for a raw material.. i gave up bc i can only look at so many handcremes and shit before i go mad. i still have some. I'll stretch it a bit.
#i hate this#fuck ai#these are absolutely not the same#imagine giving someone Na2CO3 for baking. that will end well...#i mean it is used for pretzels and shit but not to replace baking soda#how cool that your statistics model can predict language to a degree but either improve your statistics or fuck right off#bc this is not it#man i just want to make myself some good soap again
2 notes
·
View notes
Text
Actually it is SO weird to me to remember that I was an engineering student and that later on I had been pursuing a minor in statistics
I may be a IT & com person in the end, but I do have the foundations of engineering and statistics in my brain too. Wild !
#speculation nation#if i hadnt liked coding so much i probably wouldve still been an engineer.#like my school does a first year engineering track where u learn the basics and then explore different engineering options#so by ur second year u choose your official track and that decides the rest of your schooling.#and id been thinking about computer & electrical engineering. often goes hand in hand.#guys i couldve been an electrical engineer. honestly that wouldve been so cool. wasnt meant to be tho đ#i took a coding class my 2nd semester. first experience with coding. it was in C. i LOVED it.#and it got me comparing computer engineering and computer science and i decided that i wanted to do computer science#but well the intro course for that fucking sucked. didnt wanna go back to engineering either bc i hated engineering lol#im smart enough but it's fuckin soul sucking man.#eventually tho i found my way to my current home. im a techie :3 and im happy with that.#anyways do i seem like the kind of person who was into engineering and statistics? sometimes it's weird for me to remember.#but i did spent Years assuming id end up as an engineer. my grandpa was one. my dad was studying to be one b4 he dropped out#and my sister is one. just kinda runs in the family i guess. & so i was So Sure that was where i was going.#took. an engineering class in high school and everything. taught me some good foundational skills in modeling#also was the class that let me develop my signature. bc we had a notebook we had to sign the top of every day#so me doing my signature over and over again. i decided to use it as an opportunity to make it My Own. rather than just my name in cursive.#so yeah im a techie that talks good but i do have that math brain. engineering basis. statistics knowledge.#kinda feel like a jack of all trades (master of none) with it all. but see thats a good thing for companies (i hope)#ive got foundational knowledge of many things. and i am Adaptable. they can teach me the in depth shit i need to know themselves.#and i Also have my work experience in management... which i hope will help my case when applying to companies too.#aaaahhh!!! so many things to think about!!! but at the end of the day i am smart & educated and i will be a good asset to any company i join#i just need to convince them of that đ but i can probably figure something out. something !!!#i will graduate college and get some kind of IT job that pays decently & work my way up to maybe someday being an IT manager or smth#i can finally start. truly growing up. instead of being stuck in forever college unable to drive myself anywhere.#have my IT job and a car and the ability to do Whatever i want.... god i want it so bad.#im just daydreaming by this point. god im so excited to finally graduate college.
3 notes
·
View notes
Text
i do also think the "In that case, a human being inspired by someone's art style, memorizing some vague notion of it and incorporating it into their own art would also be infringing on copyright" argument is intellectually dishonest. I am against IP laws and copyright on the whole. but that's a false equivalency
#i think the argument could. possibly. be made for a person creating their own model intentionally. but overall. itâs not the same process#an animal is not a statistical model. everything you take in you add something to. lol#there is just a lot of people âi am very smartâ-ing âwhy do you value something made by a human higher than somethng made by a machine hm?â#first of all thats a nothing accusation. and secondly. a human made that machine.
4 notes
·
View notes
Text
it's almost 5 in the morning why do i hate myself
2 notes
·
View notes
Text
0 notes
Text
0 notes
Text

European Growth of ChatGPT Search (link in the bio)
0 notes
Text
Human Factors: Decision making in the real world

Itâs not enough for one person to know what happened yesterdayâteams need to spot long-term trends to predict anomalies. Data is streaming, so static analysis doesnât cut it. Unparsed data dumps donât help. Being able to visualize data through dashboards or graphs helps to make sense of patterns. It is not about becoming data scientists. You do not need a degree in mechanics to drive a car. Your driving instructor tells you in simple terms how an engine works, where the oil goes, how to turn the steering wheel and which pedal to press.
Data should not be siloed. Maintenance, logistics, management, production teams â everyone needs to know some basics to make cohesive decisions. Cross-functional training is a key element to a deployment. There is a natural reluctance to embrace new things. Knowledge empowers and concurrently dispels fear of change. In hierarchical organizations there can be an aversion to the wider distribution of real-time information outside the management cadre. Inertia comes bottom up or top down, usually it is both at the same time.
#Change Management#Charts#Dashboards#Data#Data Modelling#Education#Ergonomics#Information Flow#Learning#Statistics#Tools#Training#visualization#Visuals
0 notes