#unstructured web scraping
Explore tagged Tumblr posts
Text
How to reduce product returns with Digital shelf analytics
Discover how Digital shelf analytics can help minimize product returns to transform your retail success. Dive in for actionable strategies. Read more https://xtract.io/blog/how-to-reduce-product-returns-with-digital-shelf-analytics/
0 notes
Text
In the subject of data analytics, this is the most important concept that everyone needs to understand. The capacity to draw insightful conclusions from data is a highly sought-after talent in today's data-driven environment. In this process, data analytics is essential because it gives businesses the competitive edge by enabling them to find hidden patterns, make informed decisions, and acquire insight. This thorough guide will take you step-by-step through the fundamentals of data analytics, whether you're a business professional trying to improve your decision-making or a data enthusiast eager to explore the world of analytics.
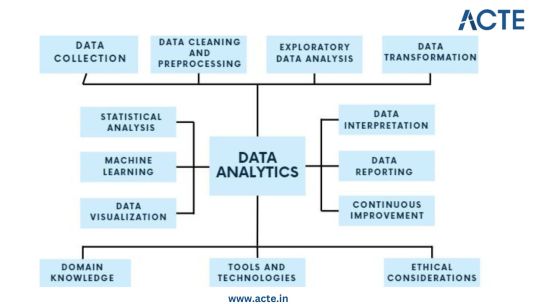
Step 1: Data Collection - Building the Foundation
Identify Data Sources: Begin by pinpointing the relevant sources of data, which could include databases, surveys, web scraping, or IoT devices, aligning them with your analysis objectives. Define Clear Objectives: Clearly articulate the goals and objectives of your analysis to ensure that the collected data serves a specific purpose. Include Structured and Unstructured Data: Collect both structured data, such as databases and spreadsheets, and unstructured data like text documents or images to gain a comprehensive view. Establish Data Collection Protocols: Develop protocols and procedures for data collection to maintain consistency and reliability. Ensure Data Quality and Integrity: Implement measures to ensure the quality and integrity of your data throughout the collection process.
Step 2: Data Cleaning and Preprocessing - Purifying the Raw Material
Handle Missing Values: Address missing data through techniques like imputation to ensure your dataset is complete. Remove Duplicates: Identify and eliminate duplicate entries to maintain data accuracy. Address Outliers: Detect and manage outliers using statistical methods to prevent them from skewing your analysis. Standardize and Normalize Data: Bring data to a common scale, making it easier to compare and analyze. Ensure Data Integrity: Ensure that data remains accurate and consistent during the cleaning and preprocessing phase.
Step 3: Exploratory Data Analysis (EDA) - Understanding the Data
Visualize Data with Histograms, Scatter Plots, etc.: Use visualization tools like histograms, scatter plots, and box plots to gain insights into data distributions and patterns. Calculate Summary Statistics: Compute summary statistics such as means, medians, and standard deviations to understand central tendencies. Identify Patterns and Trends: Uncover underlying patterns, trends, or anomalies that can inform subsequent analysis. Explore Relationships Between Variables: Investigate correlations and dependencies between variables to inform hypothesis testing. Guide Subsequent Analysis Steps: The insights gained from EDA serve as a foundation for guiding the remainder of your analytical journey.
Step 4: Data Transformation - Shaping the Data for Analysis
Aggregate Data (e.g., Averages, Sums): Aggregate data points to create higher-level summaries, such as calculating averages or sums. Create New Features: Generate new features or variables that provide additional context or insights. Encode Categorical Variables: Convert categorical variables into numerical representations to make them compatible with analytical techniques. Maintain Data Relevance: Ensure that data transformations align with your analysis objectives and domain knowledge.
Step 5: Statistical Analysis - Quantifying Relationships
Hypothesis Testing: Conduct hypothesis tests to determine the significance of relationships or differences within the data. Correlation Analysis: Measure correlations between variables to identify how they are related. Regression Analysis: Apply regression techniques to model and predict relationships between variables. Descriptive Statistics: Employ descriptive statistics to summarize data and provide context for your analysis. Inferential Statistics: Make inferences about populations based on sample data to draw meaningful conclusions.
Step 6: Machine Learning - Predictive Analytics
Algorithm Selection: Choose suitable machine learning algorithms based on your analysis goals and data characteristics. Model Training: Train machine learning models using historical data to learn patterns. Validation and Testing: Evaluate model performance using validation and testing datasets to ensure reliability. Prediction and Classification: Apply trained models to make predictions or classify new data. Model Interpretation: Understand and interpret machine learning model outputs to extract insights.
Step 7: Data Visualization - Communicating Insights
Chart and Graph Creation: Create various types of charts, graphs, and visualizations to represent data effectively. Dashboard Development: Build interactive dashboards to provide stakeholders with dynamic views of insights. Visual Storytelling: Use data visualization to tell a compelling and coherent story that communicates findings clearly. Audience Consideration: Tailor visualizations to suit the needs of both technical and non-technical stakeholders. Enhance Decision-Making: Visualization aids decision-makers in understanding complex data and making informed choices.
Step 8: Data Interpretation - Drawing Conclusions and Recommendations
Recommendations: Provide actionable recommendations based on your conclusions and their implications. Stakeholder Communication: Communicate analysis results effectively to decision-makers and stakeholders. Domain Expertise: Apply domain knowledge to ensure that conclusions align with the context of the problem.
Step 9: Continuous Improvement - The Iterative Process
Monitoring Outcomes: Continuously monitor the real-world outcomes of your decisions and predictions. Model Refinement: Adapt and refine models based on new data and changing circumstances. Iterative Analysis: Embrace an iterative approach to data analysis to maintain relevance and effectiveness. Feedback Loop: Incorporate feedback from stakeholders and users to improve analytical processes and models.
Step 10: Ethical Considerations - Data Integrity and Responsibility
Data Privacy: Ensure that data handling respects individuals' privacy rights and complies with data protection regulations. Bias Detection and Mitigation: Identify and mitigate bias in data and algorithms to ensure fairness. Fairness: Strive for fairness and equitable outcomes in decision-making processes influenced by data. Ethical Guidelines: Adhere to ethical and legal guidelines in all aspects of data analytics to maintain trust and credibility.
Data analytics is an exciting and profitable field that enables people and companies to use data to make wise decisions. You'll be prepared to start your data analytics journey by understanding the fundamentals described in this guide. To become a skilled data analyst, keep in mind that practice and ongoing learning are essential. If you need help implementing data analytics in your organization or if you want to learn more, you should consult professionals or sign up for specialized courses. The ACTE Institute offers comprehensive data analytics training courses that can provide you the knowledge and skills necessary to excel in this field, along with job placement and certification. So put on your work boots, investigate the resources, and begin transforming.
24 notes
·
View notes
Text
Healthcare Market Research: Why Does It Matter?
Healthcare market research (MR) providers interact with several stakeholders to discover and learn about in-demand treatment strategies and patients’ requirements. Their insightful reports empower medical professionals, insurance companies, and pharma businesses to engage with patients in more fulfilling ways. This post will elaborate on the growing importance of healthcare market research.
What is Healthcare Market Research?
Market research describes consumer and competitor behaviors using first-hand or public data collection methods, like surveys and web scraping. In medicine and life sciences, clinicians and accessibility device developers can leverage it to improve patient outcomes. They grow faster by enhancing their approaches as validated MR reports recommend.
Finding key opinion leaders (KOL), predicting demand dynamics, or evaluating brand recognition efforts becomes more manageable thanks to domain-relevant healthcare market research consulting. Although primary MR helps with authority-building, monitoring how others in the target field innovate their business models is also essential. So, global health and life science enterprises value secondary market research as much as primary data-gathering procedures.
The Importance of Modern Healthcare Market Research
1| Learning What Competitors Might Do Next
Businesses must beware of market share fluctuations due to competitors’ expansion strategies. If your clients are more likely to seek help from rival brands, this situation suggests failure to compete.
Companies might provide fitness products, over-the-counter (OTC) medicines, or childcare facilities. However, they will always lose to a competitor who can satisfy the stakeholders’ demands more efficiently. These developments evolve over the years, during which you can study and estimate business rivals’ future vision.
You want to track competing businesses’ press releases, public announcements, new product launches, and marketing efforts. You must also analyze their quarter-on-quarter market performance. If the data processing scope exceeds your tech capabilities, consider using healthcare data management services offering competitive intelligence integrations.
2| Understanding Patients and Their Needs for Unique Treatment
Patients can experience unwanted bodily changes upon consuming a medicine improperly. Otherwise, they might struggle to use your accessibility technology. If healthcare providers implement a user-friendly feedback and complaint collection system, they can reduce delays. As a result, patients will find a cure for their discomfort more efficiently.
However, processing descriptive responses through manual means is no longer necessary. Most market research teams have embraced automated unstructured data processing breakthroughs. They can guess a customer’s emotions and intentions from submitted texts without frequent human intervention. This era of machine learning (ML) offers ample opportunities to train ML systems to sort patients’ responses quickly.
So, life science companies can increase their employees’ productivity if their healthcare market research providers support ML-based feedback sorting and automation strategies.
Besides, hospitals, rehabilitation centers, and animal care facilities can incorporate virtual or physical robots powered by conversational artificial intelligence (AI). Doing so is one of the potential approaches to addressing certain patients’ loneliness problems throughout hospitalization. Utilize MR to ask your stakeholders whether such integrations improve their living standards.
3| Improving Marketing and Sales
Healthcare market research aids pharma and biotechnology corporations to categorize customer preferences according to their impact on sales. It also reveals how brands can appeal to more people when introducing a new product or service. One approach is to shut down or downscale poorly performing ideas.
If a healthcare facility can reduce resources spent on underperforming promotions, it can redirect them to more engaging campaigns. Likewise, MR specialists let patients and doctors directly communicate their misgivings about such a medicine or treatment via online channels. The scale of these surveys can extend to national, continental, or global markets. It is more accessible as cloud platforms flexibly adjust the resources a market research project may need.
With consistent communication involving doctors, patients, equipment vendors, and pharmaceutical brands, the healthcare industry will be more accountable. It will thrive sustainably.
Healthcare Market Research: Is It Ethical?
Market researchers in healthcare and life sciences will rely more on data-led planning as competition increases and customers demand richer experiences like telemedicine. Remember, it is not surprising how awareness regarding healthcare infrastructure has skyrocketed since 2020. At the same time, life science companies must proceed with caution when handling sensitive data in a patient’s clinical history.
On one hand, universities and private research projects need more healthcare data. Meanwhile, threats of clinical record misuse are real, having irreparable financial and psychological damage potential.
Ideally, hospitals, laboratories, and pharmaceutical firms must inform patients about the use of health records for research or treatment intervention. Today, reputed data providers often conduct MR surveys, use focus groups, and scan scholarly research publications. They want to respect patients’ choice in who gets to store, modify, and share the data.
Best Practices for Healthcare Market Research Projects
Legal requirements affecting healthcare data analysis, market research, finance, and ethics vary worldwide. Your data providers must recognize and respect this reality. Otherwise, gathering, storing, analyzing, sharing, or deleting a patient’s clinical records can increase legal risks.
Even if a healthcare business has no malicious intention behind extracting insights, cybercriminals can steal healthcare data. Therefore, invest in robust IT infrastructure, partner with experts, and prioritize data governance.
Like customer-centricity in commercial market research applications, dedicate your design philosophy to patient-centricity.
Incorporating health economics and outcomes research (HEOR) will depend on real-world evidence (RWE). Therefore, protect data integrity and increase quality management standards. If required, find automated data validation assistance and develop or rent big data facilities.
Capture data on present industry trends while maintaining a grasp on long-term objectives. After all, a lot of data is excellent for accuracy, but relevance is the backbone of analytical excellence and business focus.
Conclusion
Given this situation, transparency is the key to protecting stakeholder faith in healthcare data management. As such, MR consultants must act accordingly. Healthcare market research is not unethical. Yet, this statement stays valid only if a standardized framework specifies when patients’ consent trumps medical researchers’ data requirements. Healthcare market research is not unethical. Yet, this statement stays valid only if a standardized framework specifies when patients’ consent trumps medical researchers’ data requirements.
Market research techniques can help fix the long-standing communication and ethics issues in doctor-patient relationships if appropriately configured, highlighting their importance in the healthcare industry’s progress. When patients willingly cooperate with MR specialists, identifying recovery challenges or clinical devices’ ergonomic failures is quick. No wonder that health and life sciences organizations want to optimize their offerings by using market research.
3 notes
·
View notes
Text
Web Scraping 101: Everything You Need to Know in 2025
🕸️ What Is Web Scraping? An Introduction
Web scraping—also referred to as web data extraction—is the process of collecting structured information from websites using automated scripts or tools. Initially driven by simple scripts, it has now evolved into a core component of modern data strategies for competitive research, price monitoring, SEO, market intelligence, and more.
If you’re wondering “What is the introduction of web scraping?” — it’s this: the ability to turn unstructured web content into organized datasets businesses can use to make smarter, faster decisions.
💡 What Is Web Scraping Used For?
Businesses and developers alike use web scraping to:
Monitor competitors’ pricing and SEO rankings
Extract leads from directories or online marketplaces
Track product listings, reviews, and inventory
Aggregate news, blogs, and social content for trend analysis
Fuel AI models with large datasets from the open web
Whether it’s web scraping using Python, browser-based tools, or cloud APIs, the use cases are growing fast across marketing, research, and automation.
🔍 Examples of Web Scraping in Action
What is an example of web scraping?
A real estate firm scrapes listing data (price, location, features) from property websites to build a market dashboard.
An eCommerce brand scrapes competitor prices daily to adjust its own pricing in real time.
A SaaS company uses BeautifulSoup in Python to extract product reviews and social proof for sentiment analysis.
For many, web scraping is the first step in automating decision-making and building data pipelines for BI platforms.
⚖️ Is Web Scraping Legal?
Yes—if done ethically and responsibly. While scraping public data is legal in many jurisdictions, scraping private, gated, or copyrighted content can lead to violations.
To stay compliant:
Respect robots.txt rules
Avoid scraping personal or sensitive data
Prefer API access where possible
Follow website terms of service
If you’re wondering “Is web scraping legal?”—the answer lies in how you scrape and what you scrape.
🧠 Web Scraping with Python: Tools & Libraries
What is web scraping in Python? Python is the most popular language for scraping because of its ease of use and strong ecosystem.
Popular Python libraries for web scraping include:
BeautifulSoup – simple and effective for HTML parsing
Requests – handles HTTP requests
Selenium – ideal for dynamic JavaScript-heavy pages
Scrapy – robust framework for large-scale scraping projects
Puppeteer (via Node.js) – for advanced browser emulation
These tools are often used in tutorials like “Web scraping using Python BeautifulSoup” or “Python web scraping library for beginners.”
⚙️ DIY vs. Managed Web Scraping
You can choose between:
DIY scraping: Full control, requires dev resources
Managed scraping: Outsourced to experts, ideal for scale or non-technical teams
Use managed scraping services for large-scale needs, or build Python-based scrapers for targeted projects using frameworks and libraries mentioned above.
🚧 Challenges in Web Scraping (and How to Overcome Them)
Modern websites often include:
JavaScript rendering
CAPTCHA protection
Rate limiting and dynamic loading
To solve this:
Use rotating proxies
Implement headless browsers like Selenium
Leverage AI-powered scraping for content variation and structure detection
Deploy scrapers on cloud platforms using containers (e.g., Docker + AWS)
🔐 Ethical and Legal Best Practices
Scraping must balance business innovation with user privacy and legal integrity. Ethical scraping includes:
Minimal server load
Clear attribution
Honoring opt-out mechanisms
This ensures long-term scalability and compliance for enterprise-grade web scraping systems.
🔮 The Future of Web Scraping
As demand for real-time analytics and AI training data grows, scraping is becoming:
Smarter (AI-enhanced)
Faster (real-time extraction)
Scalable (cloud-native deployments)
From developers using BeautifulSoup or Scrapy, to businesses leveraging API-fed dashboards, web scraping is central to turning online information into strategic insights.
📘 Summary: Web Scraping 101 in 2025
Web scraping in 2025 is the automated collection of website data, widely used for SEO monitoring, price tracking, lead generation, and competitive research. It relies on powerful tools like BeautifulSoup, Selenium, and Scrapy, especially within Python environments. While scraping publicly available data is generally legal, it's crucial to follow website terms of service and ethical guidelines to avoid compliance issues. Despite challenges like dynamic content and anti-scraping defenses, the use of AI and cloud-based infrastructure is making web scraping smarter, faster, and more scalable than ever—transforming it into a cornerstone of modern data strategies.
🔗 Want to Build or Scale Your AI-Powered Scraping Strategy?
Whether you're exploring AI-driven tools, training models on web data, or integrating smart automation into your data workflows—AI is transforming how web scraping works at scale.
👉 Find AI Agencies specialized in intelligent web scraping on Catch Experts,
📲 Stay connected for the latest in AI, data automation, and scraping innovation:
💼 LinkedIn
🐦 Twitter
📸 Instagram
👍 Facebook
▶️ YouTube
#web scraping#what is web scraping#web scraping examples#AI-powered scraping#Python web scraping#web scraping tools#BeautifulSoup Python#web scraping using Python#ethical web scraping#web scraping 101#is web scraping legal#web scraping in 2025#web scraping libraries#data scraping for business#automated data extraction#AI and web scraping#cloud scraping solutions#scalable web scraping#managed scraping services#web scraping with AI
0 notes
Link
[ad_1] Web scraping and data extraction are crucial for transforming unstructured web content into actionable insights. Firecrawl Playground streamlines this process with a user-friendly interface, enabling developers and data practitioners to explore and preview API responses through various extraction methods easily. In this tutorial, we walk through the four primary features of Firecrawl Playground: Single URL (Scrape), Crawl, Map, and Extract, highlighting their unique functionalities. Single URL Scrape In the Single URL mode, users can extract structured content from individual web pages by providing a specific URL. The response preview within the Firecrawl Playground offers a concise JSON representation, including essential metadata such as page title, description, main content, images, and publication dates. The user can easily evaluate the structure and quality of data returned by this single-page scraping method. This feature is useful for cases where focused, precise data from individual pages, such as news articles, product pages, or blog posts, is required. The user accesses the Firecrawl Playground and enters the URL www.marktechpost.com under the Single URL (/scrape) tab. They select the FIRE-1 model and write the prompt: “Get me all the articles on the homepage.” This sets up Firecrawl’s agent to retrieve structured content from the MarkTechPost homepage using an LLM-powered extraction approach. The result of the single-page scrape is displayed in a Markdown view. It successfully extracts links to various sections, such as “Natural Language Processing,” “AI Agents,” “New Releases,” and more, from the homepage of MarkTechPost. Below these links, a sample article headline with introductory text is also displayed, indicating accurate content parsing. Crawl The Crawl mode significantly expands extraction capabilities by allowing automated traversal through multiple interconnected web pages starting from a given URL. Within the Playground’s preview, users can quickly examine responses from the initial crawl, observing JSON-formatted summaries of page content alongside URLs discovered during crawling. The Crawl feature effectively handles broader extraction tasks, including retrieving comprehensive content from entire websites, category pages, or multi-part articles. Users benefit from the ability to assess crawl depth, page limits, and response details through this preview functionality. In the Crawl (/crawl) tab, the same site ( www.marktechpost.com ) is used. The user sets a crawl limit of 10 pages and configures path filters to exclude pages such as “blog” or “about,” while including only URLs under the “/articles/” path. Page options are customized to extract only the main content, avoiding tags such as scripts, ads, and footers, thereby optimizing the crawl for relevant information. The platform shows results for 10 pages scraped from MarkTechPost. Each tile in the results grid presents content extracted from different sections, such as “Sponsored Content,” “SLD Dashboard,” and “Embed Link.” Each page has both Markdown and JSON response tabs, offering flexibility in how the extracted content is viewed or processed. Map The Map feature introduces an advanced extraction mechanism by applying user-defined mappings across crawled data. It enables users to specify custom schema structures, such as extracting particular text snippets, authors’ names, or detailed product descriptions from multiple pages simultaneously. The Playground preview clearly illustrates how mapping rules are applied, presenting extracted data in a neatly structured JSON format. Users can quickly confirm the accuracy of their mappings and ensure that the extracted content aligns precisely with their analytical requirements. This feature significantly streamlines complex data extraction workflows requiring consistency across multiple webpages. In the Map (/map) tab, the user again targets www.marktechpost.com but this time uses the Search (Beta) feature with the keyword “blog.” Additional options include enabling subdomain searches and respecting the site’s sitemap. This mode aims to retrieve a large number of relevant URLs that match the search pattern. The mapping operation returns a total of 5000 matched URLs from the MarkTechPost website. These include links to categories and articles under themes such as AI, machine learning, knowledge graphs, and others. The links are displayed in a structured list, with the option to view results as JSON or download them for further processing. Currently available in Beta, the Extract feature further refines Firecrawl’s capabilities by facilitating tailored data retrieval through advanced extraction schemas. With Extract, users design highly granular extraction patterns, such as isolating specific data points, including author metadata, detailed product specifications, pricing information, or publication timestamps. The Playground’s Extract preview displays real-time API responses that reflect user-defined schemas, providing immediate feedback on the accuracy and completeness of the extraction. As a result, users can iterate and fine-tune extraction rules seamlessly, ensuring data precision and relevance. Under the Extract (/extract) tab (Beta), the user enters the URL and defines a custom extraction schema. Two fields are specified: company_mission as a string and is_open_source as a boolean. The prompt guides the extraction to ignore details such as partners or integrations, focusing instead on the company’s mission and whether it is open-source. The final formatted JSON output shows that MarkTechPost is identified as an open-source platform, and its mission is accurately extracted: “To provide the latest news and insights in the field of Artificial Intelligence and technology, focusing on research, tutorials, and industry developments.” In conclusion, Firecrawl Playground provides a robust and user-friendly environment that significantly simplifies the complexities of web data extraction. Through intuitive previews of API responses across Single URL, Crawl, Map, and Extract modes, users can effortlessly validate and optimize their extraction strategies. Whether working with isolated web pages or executing intricate, multi-layered extraction schemas across entire sites, Firecrawl Playground empowers data professionals with powerful, versatile tools essential for effective and accurate web data retrieval. Also, don’t forget to follow us on Twitter and join our Telegram Channel and LinkedIn Group. Don’t Forget to join our 90k+ ML SubReddit. 🔥 [Register Now] miniCON Virtual Conference on AGENTIC AI: FREE REGISTRATION + Certificate of Attendance + 4 Hour Short Event (May 21, 9 am- 1 pm PST) + Hands on Workshop Asif Razzaq is the CEO of Marktechpost Media Inc.. As a visionary entrepreneur and engineer, Asif is committed to harnessing the potential of Artificial Intelligence for social good. His most recent endeavor is the launch of an Artificial Intelligence Media Platform, Marktechpost, which stands out for its in-depth coverage of machine learning and deep learning news that is both technically sound and easily understandable by a wide audience. The platform boasts of over 2 million monthly views, illustrating its popularity among audiences. [ad_2] Source link
0 notes
Text
ow do leading industries turn the web into a source of competitive advantage?
What do e-commerce giants, financial firms, real estate platforms, and pharma leaders have in common? They all rely on web scraping to fuel growth, innovation, and competitive advantage.
Here’s how industries are turning unstructured web data into strategic insights:
♦ Track real-time pricing, trends, and customer sentiment
♦ Extract market intelligence at scale ♦ Make faster, data-backed decisions across teams Explore how different sectors are using web scraping to fuel smarter decisions and faster growth : https://bit.ly/4jq0vzu #WebScraping #MarketResearch #FinancialAnalytics #HealthcareData #RealEstateInsights #JobMarketAnalytics #TravelData #PromptCloud
0 notes
Text
What Is Web Scraping? A Complete Guide to Data Extraction
Web scraping is an automated technique for extracting large volumes of data from websites, transforming unstructured web data into structured, usable formats. For businesses, it has become a key tool for gathering valuable insights and powering operations like market research, lead generation, price monitoring, and more.
0 notes
Text
Intro to Web Scraping
Chances are, if you have access to the internet, you have heard of Data Science. Aside from the buzz generated by the title ‘Data Scientist’, only a few in relevant fields can claim to understand what data science is. The majority of people think, if at all, that a data scientist is a mad scientist type able to manipulate statistics and computers to magically generate crazy visuals and insights seemingly out of thin air.
Looking at the plethora of definitions to be found in numerous books and across the internet of what data science is, the layman’s image of a data scientist may not be that far off.
While the exact definition of ‘data science’ is still a work in progress, most in the know would agree that the data science universe encompasses fields such as:
Big Data
Analytics
Machine Learning
Data Mining
Visualization
Deep Learning
Business Intelligence
Predictive Modeling
Statistics
Data Source: Top keywords

Image Source – Michael Barber
Further exploration of the skillset that goes into what makes a data scientist, consensus begins to emerge around the following:
Statistical Analysis
Programming/Coding Skills: - R Programming; Python Coding
Structured Data (SQL)
Unstructured Data (3-5 top NoSQL DBs)
Machine Learning/Data Mining Skills
Data Visualization
Big Data Processing Platforms: Hadoop, Spark, Flink, etc.
Structured vs unstructured data
Structured data refers to information with a high degree of organization, such that inclusion in a relational database is seamless and readily searchable by simple, straightforward search engine algorithms or other search operation
Examples of structured data include numbers, dates, and groups of words and numbers called strings.
Unstructured data (or unstructured information) is information that either does not have a pre-defined data model or is not organized in a pre-defined manner. Unstructured information is typically text-heavy, but may contain data such as dates, numbers, and facts as well. This results in irregularities and ambiguities that make it difficult to understand using traditional programs as compared to data stored in fielded form in databases or annotated (semantically tagged) in documents.
Examples of "unstructured data" may include books, journals, documents, metadata, health records, audio, video, analog data, images, files, and unstructured text such as the body of an e-mail message, Web pages, or word-processor document. Source: Unstructured data - Wikipedia
Implied within the definition of unstructured data is the fact that it is very difficult to search. In addition, the vast amount of data in the world is unstructured. A key skill when it comes to mining insights out of the seeming trash that is unstructured data is web scraping.
What is web scraping?
Everyone has done this: you go to a web site, see an interesting table and try to copy it over to Excel so you can add some numbers up or store it for later. Yet this often does not really work, or the information you want is spread across a large number of web sites. Copying by hand can quickly become very tedious.
You’ve tried everything else, and you haven’t managed to get your hands on the data you want. You’ve found the data on the web, but, alas — no download options are available and copy-paste has failed you. Fear not, there may still be a way to get the data out. Source: Data Journalism Handbook
As a data scientist, the more data you collect, the better your models, but what if the data you want resides on a website? This is the problem of social media analysis when the data comes from users posting content online and can be extremely unstructured. While there are some websites who support data collection from their web pages and have even exposed packages and APIs (such as Twitter), most of the web pages lack the capability and infrastructure for this. If you are a data scientist who wants to capture data from such web pages then you wouldn’t want to be the one to open all these pages manually and scrape the web pages one by one. Source: Perceptive Analytics
Web scraping, web harvesting, or web data extraction is data scraping used for extracting data from websites. Web scraping software may access the World Wide Web directly using the Hypertext Transfer Protocol, or through a web browser. While web scraping can be done manually by a software user, the term typically refers to automated processes implemented using a bot or web crawler. It is a form of copying, in which specific data is gathered and copied from the web, typically into a central local database or spreadsheet, for later retrieval or analysis. Source: Wikipedia
Web Scraping is a method to convert the data from websites, whether structured or unstructured, from HTML into a form on which analysis can be performed.
The advantage of scraping is that you can do it with virtually any web site — from weather forecasts to government spending, even if that site does not have an API for raw data access. While this method is very powerful and can be used in many places, it requires a bit of understanding about how the web works.
There are a variety of ways to scrape a website to extract information for reuse. In its simplest form, this can be achieved by copying and pasting snippets from a web page, but this can be unpractical if there is a large amount of data to be extracted, or if it spread over a large number of pages. Instead, specialized tools and techniques can be used to automate this process, by defining what sites to visit, what information to look for, and whether data extraction should stop once the end of a page has been reached, or whether to follow hyperlinks and repeat the process recursively. Automating web scraping also allows to define whether the process should be run at regular intervals and capture changes in the data.
https://librarycarpentry.github.io/lc-webscraping/
Web Scraping with R
Atop any data scientist’s toolkit lie Python and R. While python is a general purpose coding language used in a variety of situations; R was built from the ground up to mold statistics and data. From data extraction, to clean up, to visualization to publishing; R is in use. Unlike packages such as tableau, Stata or Matlab which are skewed either towards data manipulation or visualization, R is a general purpose statistical language with functionality cutting across all data management operations. R is also free and open source which contributes to making it even more popular.
To extend the boundaries limiting data scientists from accessing data from web pages, there are packages based on ‘Web scraping’ available in R. Let us look into web scraping technique using R.
Harvesting Data with RVEST
R. Hadley Wickham authored the rvest package for web scraping using R which will be demonstrated in this tutorial. Although web scraping with R is a fairly advanced topic it is possible to dive in with a few lines of code within a few steps and appreciate its utility, versatility and power.
We shall use 2 examples inspired by Julia Silge in her series cool things you can do with R in a tweet:
Scraping the list of districts of Uganda
Getting the list of MPs of the Republic of Rwanda
0 notes
Text
Five Things You Didn’t Know About Unstructured Web
Get the most out of web data with our custom web scraping and crawling solutions. Our intelligent data extraction capabilities help in fetching data from complex websites with ease. Read more https://www.scrape.works/infographics/BigData/five-things-you-didnt-know-about-unstructured-web
0 notes
Text
Web Scraping Liquor Prices by Location for Market Trends

Introduction
In today's digital landscape, Web Scraping Liquor Prices by Location is essential for businesses, researchers, and consumers seeking data-driven insights. The liquor industry faces dynamic pricing influenced by regional variations, competition, and promotions. By utilizing Web Scraping for Liquor Market Analysis, businesses can extract real-time pricing data across different geographic areas, enabling them to track price fluctuations, analyze demand trends, and adjust strategies accordingly.
With the ability to Track Liquor Prices Across Regions with Data Scraping, stakeholders can gain a competitive edge by monitoring market trends and optimizing pricing strategies. This technique helps businesses stay informed about competitor pricing, identify emerging patterns, and make strategic decisions based on data-driven insights. Whether for retailers, wholesalers, or researchers, web scraping empowers informed decision-making in an evolving market. Businesses can efficiently collect and analyze liquor pricing data by leveraging automation, ensuring they remain competitive and responsive to market shifts.
Importance of Web Scraping Liquor Prices

Liquor pricing varies significantly by location due to taxation policies, regional demand, supply chain factors, and retailer pricing strategies. Traditional data collection methods are time-consuming and prone to errors, whereas Real-Time Liquor Price Data Extraction automates the process, ensuring accuracy and efficiency.
Competitive Analysis: One primary reason for Web Scraping Alcohol and liquor Data is to perform competitive analysis. Liquor retailers and distributors can track pricing trends among competitors, identify overpriced or underpriced products, and adjust their strategies accordingly to stay competitive.
Regional Price Variation Insights: Extract Regional Liquor Pricing Data to understand how liquor prices fluctuate due to state regulations and taxation. For instance, a bottle of whiskey may cost significantly more in one state than another due to excise duties, impacting both businesses and consumers.
Market Trend Identification: Scraping Regional Alcohol Pricing Trends helps track liquor prices over time, revealing seasonal trends, such as price spikes during holidays or significant events. Retailers can use this insight to optimize stock levels and pricing strategies.
Consumer Price Awareness: Liquor price datasets benefit consumers by enabling them to compare prices across retailers and find the best deals. Many price comparison platforms rely on web scraping for accurate and updated pricing information.
Challenges in Scraping Liquor Price Data

While web scraping is a powerful tool for collecting liquor price data, several challenges need to be addressed.
Anti-Scraping Measures by Websites: Many liquor retailers and e-commerce platforms employ anti-scraping techniques, such as CAPTCHA, IP blocking, and JavaScript rendering, to prevent automated data extraction. Overcoming these barriers requires sophisticated scraping techniques, including proxy servers, headless browsers, and CAPTCHA-solving mechanisms.
Dynamic Pricing: Liquor prices on online platforms may change frequently due to promotions, discounts, or dynamic pricing algorithms. Capturing accurate data requires setting up scheduled scraping operations that collect information at regular intervals to ensure up-to-date insights.
Data Structure Variability: Different websites display pricing information in various formats, making standardizing the data challenging. Scrapers must be designed to handle structured and unstructured data effectively, extracting relevant information such as brand name, bottle size, price, discounts, and location.
Legal and Ethical Considerations: Web scraping laws vary by country, and some websites prohibit data extraction in their terms of service. Businesses must comply with legal regulations by using ethical scraping practices, such as obtaining permission from website owners or relying on publicly available APIs.
Applications of Scraped Liquor Price Data

Once liquor price data has been successfully scraped, it can be used in various ways to drive business intelligence, improve consumer decision-making, and enhance market research.
Price Optimization Strategies: Retailers and liquor brands can use scraped data to optimize pricing strategies based on market demand, competitor pricing, and regional variations. Dynamic pricing models can be developed to adjust prices in real-time based on demand and supply conditions.
Inventory Management: Access to real-time pricing data allows liquor retailers to manage their inventory efficiently. If a specific liquor brand experiences a sudden price hike in a particular region, businesses can allocate stock accordingly to maximize profits and meet demand.
Business Intelligence for Manufacturers: Liquor manufacturers can use scraped pricing data to analyze their products' prices across different markets. This helps formulate better distribution and promotional strategies to ensure brand visibility and profitability.
Consumer-Facing Price Comparison Platforms: Many online platforms provide consumers with price comparison tools for alcoholic beverages. These platforms rely on scraped data to update users on their region's latest liquor prices, discounts, and availability.
Get accurate, real-time liquor data with our advanced web scraping solutions—boost your business today!
Contact Us Today!
Future of Web Scraping in the Liquor Industry

Web scraping is becoming increasingly vital in the liquor industry as businesses and consumers rely more on data-driven insights. Future AI and machine learning advancements will enhance data extraction efficiency, allowing for faster and more accurate analysis. Additionally, the rise of structured APIs may reduce the need for extensive web scraping by providing direct access to pricing data.
As e-commerce platforms integrate AI-driven recommendations and dynamic pricing, web scraping will be crucial in tracking real-time changes. This will enable businesses to refine marketing strategies, optimize pricing models, and enhance customer engagement through competitive pricing and personalized promotions. By leveraging web scraping, liquor retailers and distributors can stay ahead of market trends, monitor competitor strategies, and adjust their offerings to effectively meet consumer demand. As data drives business decisions, web scraping will remain essential in ensuring competitive advantage in the evolving liquor industry.
How Product Data Scrape Can Help You?

1. Price Monitoring & Competitor Analysis – Extract real-time pricing data from liquor e-commerce websites and marketplaces to track competitor pricing, discounts, and promotional strategies.
2. Product Catalog & Inventory Tracking – Scrape detailed liquor product listings, including brand, category, alcohol content, and availability, to maintain up-to-date inventory insights.
3. Consumer Sentiment & Review Analysis – Collect and analyze customer reviews and ratings to understand consumer preferences, emerging trends, and brand perception.
4. Market Trend Insights – Extract sales data, regional availability, and seasonal demand patterns to help businesses optimize marketing campaigns and product offerings.
5. Regulatory Compliance & Labeling Information – Scrape data on liquor regulations, labeling requirements, and tax policies across different regions to ensure compliance and informed decision-making.
Conclusion
Web scraping liquor prices by location is a game-changer for businesses, researchers, and consumers seeking real-time pricing insights. The benefits of extracting liquor price data are vast, from competitive analysis to price optimization. However, challenges such as anti-scraping measures, dynamic pricing, and legal considerations must be navigated carefully to ensure compliance and efficiency.
By leveraging advanced scraping techniques, businesses can unlock valuable market intelligence, optimize pricing strategies, and stay ahead of industry trends. As technology evolves, web scraping will play a pivotal role in transforming how liquor prices are monitored and analyzed worldwide.
At Product Data Scrape, we strongly emphasize ethical practices across all our services, including Competitor Price Monitoring and Mobile App Data Scraping. Our commitment to transparency and integrity is at the heart of everything we do. With a global presence and a focus on personalized solutions, we aim to exceed client expectations and drive success in data analytics. Our dedication to ethical principles ensures that our operations are both responsible and effective.
Know More>> https://www.productdatascrape.com/web-scraping-liquor-prices-track-market-trends.php
#WebScrapingLiquorPricesByLocationHelpTrackMarketTrends#WebScrapingForLiquorMarketAnalysis#TrackLiquorPricesAcrossRegionsWithDataScraping#RealTimeLiquorPriceDataExtraction#WebScrapingAlcoholAndLiquorData#ExtractRegionalLiquorPricingData
0 notes
Text
```markdown
Cryptocurrency Web Scraping: Unlocking Insights from the Digital Frontier
In the ever-evolving landscape of digital currencies, staying ahead of the game requires more than just a keen eye for trends. It demands access to real-time data and insights that can only be unlocked through web scraping.
The Power of Data in Cryptocurrency
Cryptocurrencies are inherently volatile, with prices fluctuating based on a myriad of factors including market sentiment, regulatory changes, and technological advancements. One powerful tool that has emerged as a key player in this arena is web scraping. By harnessing the power of web scraping, enthusiasts and investors alike can unlock a wealth of information that was previously inaccessible or difficult to obtain.
What is Web Scraping?
Web scraping, in its simplest form, involves using automated tools to extract data from websites. This process allows users to gather large amounts of structured and unstructured data from various sources, which can then be analyzed to derive meaningful insights. In the context of cryptocurrencies, web scraping can be used to collect data on everything from price movements and trading volumes to social media sentiment and news articles.
Why Web Scrape for Cryptocurrencies?
The cryptocurrency market is notoriously unpredictable, with prices swinging wildly in response to a variety of factors. To make informed decisions, it's crucial to have access to up-to-date and comprehensive data. Web scraping provides a way to automate the collection of this data, allowing users to:
Monitor Market Trends: Stay ahead of the curve by tracking real-time price movements and trading volumes.
Analyze Sentiment: Understand how public opinion and news events impact the value of different coins.
Identify Patterns: Use historical data to identify patterns and predict future trends.
Stay Informed: Keep abreast of the latest developments in the crypto world, from new coin launches to major partnerships.
Tools and Techniques
There are several tools available for web scraping, ranging from simple scripts written in Python using libraries like BeautifulSoup and Scrapy to more advanced platforms designed specifically for financial data collection. These tools allow users to scrape data from a variety of sources, including:
Cryptocurrency Exchanges: Gather data on trading volumes, order books, and price movements.
News Websites: Track breaking news and announcements that could affect the market.
Social Media Platforms: Monitor discussions and sentiment around specific coins or technologies.
Ethical Considerations
While web scraping offers a wealth of opportunities, it's important to approach it ethically and responsibly. Always ensure that you're scraping data in compliance with website terms of service and privacy policies. Additionally, consider the potential impact of your scraping activities on the servers of the sites you're scraping from.
Conclusion
Web scraping is a powerful tool that can provide valuable insights into the cryptocurrency market. However, it's important to use this tool responsibly and ethically. As the landscape continues to evolve, those who can effectively leverage web scraping will be better positioned to navigate the complexities of the digital frontier.
What are your thoughts on the role of web scraping in the cryptocurrency space? Do you think it's an essential tool for investors and enthusiasts, or does it raise ethical concerns? Share your thoughts in the comments below!
```
加飞机@yuantou2048
負面刪除
Google外链代发
0 notes
Text
Web Scraping
Stay Ahead with Custom Web Scraping Services by DxMinds
In today’s competitive landscape, data is a powerful asset that drives strategic decision-making and business growth. Web scraping enables businesses to extract and analyze valuable information from various online sources, helping them gain a competitive edge. Here’s why web scraping is crucial:
Why is Web Scraping Essential for Businesses?
🔹 Efficient Data Extraction – Instead of manually gathering information, web scraping automates the process, collecting structured data quickly and accurately.
🔹 Instant Access to Real-Time Data – Businesses can retrieve the latest insights from multiple sources, ensuring they make informed decisions based on up-to-date market conditions.
🔹 Comprehensive Market Research – Web scraping allows companies to monitor industry trends, customer preferences, and competitor strategies, providing valuable insights for product development and marketing.
🔹 Boosting Lead Generation & Sales – Extracting contact details, emails, and business information from websites helps organizations improve their outreach efforts and identify potential customers.
🔹 Competitive Pricing & Product Monitoring – E-commerce companies can track product prices, stock availability, and discount strategies of competitors to optimize their own pricing and inventory management.
🔹 SEO & Digital Marketing Enhancement – Marketers can use web scraping to gather data on search engine rankings, trending keywords, and competitor content strategies, refining their digital marketing campaigns for better performance.
By leveraging custom web scraping solutions, businesses can streamline operations, gain valuable business intelligence, and stay ahead in the market.
DxMinds Advanced Web Scraping Capabilities
At DxMinds, we specialize in developing customized web scraping solutions designed to meet your industry-specific needs. Our expertise ensures that businesses can extract, process, and utilize data efficiently for strategic decision-making. Here’s how our capabilities make a difference:
✅ AI-Powered Scraping – Our advanced AI-driven algorithms intelligently extract relevant data with high accuracy, reducing errors and eliminating irrelevant information. This ensures businesses receive only meaningful and structured insights.
✅ Secure & Scalable Solutions – Whether you need to scrape data from a single website or multiple platforms, our solutions are highly scalable and designed to handle large-scale data extraction securely. We implement robust security measures to prevent unauthorized access and ensure data integrity.
✅ Data Cleaning & Processing – Raw data can often be incomplete, unstructured, or duplicated. We use automated data-cleaning techniques to refine and format information into structured insights that can be easily analyzed and integrated into business workflows.
✅ Cloud-Based Storage & Automation – Our web scraping solutions are cloud-integrated, allowing businesses to store, access, and analyze scraped data in real time. Automated processes ensure that data is regularly updated and available for decision-making without manual intervention.
✅ Ethical & Compliance-Based Scraping – At DxMinds, we adhere to legal and ethical standards while performing web scraping. Our techniques ensure compliance with data privacy laws, website terms of service, and industry regulations, making our solutions safe and legally sound.
With DxMinds’ powerful web scraping capabilities, businesses can harness real-time, accurate, and structured data to optimize operations, enhance marketing strategies, track competitors, and improve decision-making.
Why Choose DxMinds for Web Scraping?
At DxMinds, we provide custom web scraping solutions designed to extract real-time, accurate, and structured data from multiple sources. Our expert team ensures:
✅ High-Performance Scraping – Fast, scalable, and efficient data extraction. ✅ AI-Driven Accuracy – Intelligent algorithms for precise data collection. ✅ Secure & Compliant – Ethical scraping practices that follow legal guidelines. ✅ Automated & Cloud-Based – Access and process data seamlessly in real-time.
With DxMinds, your business can stay ahead of the competition by leveraging valuable insights for market research, pricing strategies, lead generation, and more. 🚀
SOCIAL MEDIA URL'S
https://www.linkedin.com/company/dxminds
youtube
0 notes
Text
Enhance Data Extraction with AI for Smarter Insights
In today’s data-driven world, businesses need efficient ways to gather and process vast amounts of information. Traditional data extraction methods can be time-consuming and prone to errors, making AI-powered solutions a game-changer. By integrating artificial intelligence, companies can enhance data extraction with AI, leading to faster, more accurate, and scalable data collection processes.
The Power of AI in Data Extraction
Artificial intelligence is transforming how data is collected and analyzed. Unlike conventional scraping techniques, AI-powered extraction adapts to changes in website structures, understands unstructured data, and ensures high accuracy. Businesses leveraging AI can:
Automate Data Collection: Reduce manual efforts and speed up data processing.
Improve Accuracy: AI algorithms minimize errors by intelligently recognizing patterns.
Scale Efficiently: Handle large datasets with ease, making data extraction more reliable.
To explore how AI enhances web scraping, check out our detailed insights on web scraping with AI.
Why Businesses Need AI-Driven Data Extraction
Industries across various sectors rely on high-quality data for decision-making. Whether tracking competitor prices, monitoring customer sentiment, or extracting market trends, AI-powered data extraction offers unmatched efficiency. Here are some use cases:
1. E-commerce and Retail
Companies can extract real-time promotions data to stay ahead of market trends and offer competitive pricing. Learn more about how AI can help businesses extract real-time promotions data.
2. Real Estate Market Insights
AI-driven tools streamline real estate data scraping services, helping businesses gather property listings, pricing trends, and investment opportunities. Find out how our real estate data scraping services provide valuable insights.
3. Grocery and Retail Analytics
AI-powered scraping solutions can track grocery sales trends and consumer behavior. Check out how we analyze the Blinkit sales dataset for deeper business insights.
How Professional Web Scraping Enhances AI Integration
A key aspect of AI-driven data extraction is leveraging professional web scraping solutions. These services ensure seamless data retrieval, improved data quality, and real-time analytics, enabling businesses to make data-backed decisions efficiently.
Conclusion
Enhancing data extraction with AI is no longer a luxury but a necessity for businesses looking to stay competitive. AI-powered solutions offer automation, accuracy, and scalability, transforming raw data into actionable insights. Ready to leverage AI for your data needs? Explore our web scraping solutions today!
#web scraping with AI#extract real-time promotions data#real estate data scraping services#Blinkit sales dataset#professional web scraping
0 notes
Text
Must-Have Skills for Job in Data Science Career at the Best Data Science Institute in Laxmi Nagar
Understanding Data Science and Its Growing Scope
Data science is transforming industries by enabling organizations to make informed decisions based on data-driven insights. From healthcare to finance, e-commerce to entertainment, every sector today relies on data science for better efficiency and profitability.
With the rise of artificial intelligence and machine learning, the demand for skilled data scientists is at an all-time high. According to reports from IBM and World Economic Forum, data science is among the fastest-growing fields, with millions of new job openings expected in the coming years. Companies worldwide are looking for professionals who can analyze complex data and provide actionable solutions.
If you are planning to enter this dynamic field, choosing the best data science institute in Laxmi Nagar is crucial. Modulation Digital offers a structured and job-oriented program that ensures deep learning and hands-on experience. With 100% job assurance and real-world exposure through live projects in our in-house internship, students gain practical expertise that sets them apart in the job market.
5 Essential Skills Needed to Excel in Data Science
1. Mastering Programming Languages for Data Science
Programming is the backbone of data science. A strong command over programming languages like Python and R is essential, as they provide a wide range of libraries and frameworks tailored for data manipulation, analysis, and machine learning.
Key Aspects to Focus On:
Python: Used for data analysis, web scraping, and deep learning applications with libraries like NumPy, Pandas, Matplotlib, and Scikit-learn.
R: Preferred for statistical computing and visualization.
SQL: Essential for querying databases and handling structured data.
Version Control (Git): Helps track changes in code and collaborate effectively with teams.
At Modulation Digital, students receive intensive hands-on training in Python, R, and SQL, ensuring they are job-ready with practical knowledge and coding expertise.
2. Understanding Statistics and Mathematics
A strong foundation in statistics, probability, and linear algebra is crucial for analyzing patterns in data and developing predictive models. Many data science problems involve statistical analysis and mathematical computations to derive meaningful insights.
Core Mathematical Concepts:
Probability and Distributions: Understanding normal, binomial, and Poisson distributions helps in making statistical inferences.
Linear Algebra: Essential for working with vectors, matrices, and transformations in machine learning algorithms.
Calculus: Helps in optimizing machine learning models and understanding gradient descent.
Hypothesis Testing: Used to validate assumptions and make data-driven decisions.
Students at Modulation Digital get hands-on practice with statistical methods and problem-solving exercises, ensuring they understand the theoretical concepts and apply them effectively.
3. Data Wrangling and Preprocessing
Real-world data is often incomplete, inconsistent, and unstructured. Data wrangling refers to the process of cleaning and structuring raw data for effective analysis.
Key Techniques in Data Wrangling:
Handling Missing Data: Using imputation techniques like mean, median, or predictive modeling.
Data Normalization and Transformation: Ensuring consistency across datasets.
Feature Engineering: Creating new variables from existing data to improve model performance.
Data Integration: Merging multiple sources of data for a comprehensive analysis.
At Modulation Digital, students work on live datasets, learning how to clean, structure, and prepare data efficiently for analysis.
4. Machine Learning and AI Integration
Machine learning enables computers to learn patterns and make predictions. Understanding supervised, unsupervised, and reinforcement learning is crucial for building intelligent systems.
Important Machine Learning Concepts:
Regression Analysis: Linear and logistic regression models for prediction.
Classification Algorithms: Decision trees, SVM, and random forests.
Neural Networks and Deep Learning: Understanding CNNs, RNNs, and GANs.
Natural Language Processing (NLP): Used for text analysis and chatbots.
At Modulation Digital, students get hands-on experience in building AI-driven applications with frameworks like TensorFlow and PyTorch, preparing them for industry demands.
5. Data Visualization and Storytelling
Data visualization is essential for presenting insights in a clear and compelling manner. Effective storytelling through data helps businesses make better decisions.
Key Visualization Tools:
Tableau and Power BI: Business intelligence tools for interactive dashboards.
Matplotlib and Seaborn: Used in Python for statistical plotting.
D3.js: JavaScript library for creating dynamic data visualizations.
Dash and Streamlit: Tools for building web-based analytical applications.
At Modulation Digital, students learn how to create interactive dashboards and compelling data reports, ensuring they can communicate their findings effectively.
Support from Leading Organizations in Data Science
Global tech giants such as Google, Amazon, and IBM invest heavily in data science and continuously shape industry trends. Harvard Business Review has called data science the "sexiest job of the 21st century," highlighting its importance in today’s world.
Modulation Digital ensures that its curriculum aligns with these global trends. Additionally, our program prepares students for globally recognized certifications, increasing their credibility in the job market.
Why the Right Training Matters
A successful career in data science requires the right mix of technical knowledge, hands-on experience, and industry insights. Choosing the best data science institute in Laxmi Nagar ensures that you get a structured and effective learning environment.
Why Modulation Digital is the Best Choice for Learning Data Science
Selecting the right institute can define your career trajectory. Modulation Digital stands out as the best data science institute in Laxmi Nagar for several reasons:
1. Industry-Relevant Curriculum
Our program is designed in collaboration with industry experts and follows the latest advancements in data science, artificial intelligence, and machine learning.
2. Hands-on Learning with Live Projects
We believe in practical education. Students work on real-world projects during their in-house internship, which strengthens their problem-solving skills.
3. 100% Job Assurance
We provide placement support with top organizations, ensuring that every student gets a strong start in their career.
4. Expert Faculty and Mentorship
Data Science Trainer
Mr. Prem Kumar
Mr. Prem Kumar is a seasoned Data Scientist with over 6+ years of professional experience in data analytics, machine learning, and artificial intelligence. With a strong academic foundation and practical expertise, he has mastered a variety of tools and technologies essential for data science, including Microsoft Excel, Python, SQL, Power BI, Tableau, and advanced AI concepts like machine learning and deep learning.
As a trainer, Mr. Prem is highly regarded for his engaging teaching style and his knack for simplifying complex data science concepts. He emphasizes hands-on learning, ensuring students gain practical experience to tackle real-world challenges confidently.
5. Certifications and Career Support
Get certified by Modulation Digital, along with guidance for global certifications from IBM, Coursera, and Harvard Online, making your resume stand out.
If you are ready to kickstart your data science career, enroll at Modulation Digital today and gain the skills that top companies demand!
#DataScience#CareerInDataScience#ModulationDigital#DataScienceInstitute#JobAssurance#LiveProjectExperience#DataScienceSkills#IndustryInsights#HighPayingJobs#UnlockYourFuture#digital marketing
0 notes
Text
A Comprehensive Handbook on Datasets for Machine Learning Initiatives
Introduction:
Datasets in Machine Learning is fundamentally dependent on data. Whether you are a novice delving into predictive modeling or a seasoned expert developing deep learning architectures, the selection of an appropriate dataset is vital for achieving success. This detailed guide will examine the various categories of datasets, sources for obtaining them, and criteria for selecting the most suitable ones for your machine learning endeavors.
The Importance of Datasets in Machine Learning
A dataset serves as the foundation for any machine learning model. High-quality and well-organized datasets enable models to identify significant patterns, whereas subpar data can result in inaccurate and unreliable outcomes. Datasets impact several aspects, including:
Model accuracy and efficiency
Feature selection and engineering
Generalizability of models
Training duration and computational requirements
Selecting the appropriate dataset is as critical as choosing the right algorithm. Let us now investigate the different types of datasets and their respective applications.
Categories of Machine Learning Datasets
Machine learning datasets are available in various formats and serve multiple purposes. The primary categories include:
1. Structured vs. Unstructured Datasets
Structured data: Arranged in a tabular format consisting of rows and columns (e.g., Excel, CSV files, relational databases).
Unstructured data: Comprises images, videos, audio files, and text that necessitate preprocessing prior to being utilized in machine learning models.
2. Supervised vs. Unsupervised Datasets
Supervised datasets consist of labeled information, where input-output pairs are clearly defined, and are typically employed in tasks related to classification and regression.
Unsupervised datasets, on the other hand, contain unlabeled information, allowing the model to independently identify patterns and structures, and are utilized in applications such as clustering and anomaly detection.
3. Time-Series and Sequential Data
These datasets are essential for forecasting and predictive analytics, including applications like stock market predictions, weather forecasting, and data from IoT sensors.
4. Text and NLP Datasets
Text datasets serve various natural language processing functions, including sentiment analysis, the development of chatbots, and translation tasks.
5. Image and Video Datasets
These datasets are integral to computer vision applications, including facial recognition, object detection, and medical imaging.
Having established an understanding of the different types of datasets, we can now proceed to examine potential sources for obtaining them.
Domain-Specific Datasets
Healthcare and Medical Datasets
MIMIC-III – ICU patient data for medical research.
Chest X-ray Dataset – Used for pneumonia detection.
Finance and Economics Datasets
Yahoo Finance API – Financial market and stock data.
Quandl – Economic, financial, and alternative data.
Natural Language Processing (NLP) Datasets
Common Crawl – Massive web scraping dataset.
Sentiment140 – Labeled tweets for sentiment analysis.
Computer Vision Datasets
ImageNet – Large-scale image dataset for object detection.
COCO (Common Objects in Context) – Image dataset for segmentation and captioning tasks.
Custom Dataset Generation
When publicly available datasets do not fit your needs, you can:
Web Scraping: Use BeautifulSoup or Scrapy to collect custom data.
APIs: Utilize APIs from Twitter, Reddit, and Google Maps to generate unique datasets.
Synthetic Data: Create simulated datasets using libraries like Faker or Generative Adversarial Networks (GANs).
Selecting an Appropriate Dataset
The choice of an appropriate dataset is influenced by various factors:
Size and Diversity – A dataset that is both large and diverse enhances the model's ability to generalize effectively.
Data Quality – High-quality data that is clean, accurately labeled, and devoid of errors contributes to improved model performance.
Relevance – It is essential to select a dataset that aligns with the specific objectives of your project.
Legal and Ethical Considerations – Ensure adherence to data privacy laws and regulations, such as GDPR and HIPAA.
In Summary
Datasets serve as the cornerstone of any machine learning initiative. Regardless of whether the focus is on natural language processing, computer vision, or financial forecasting, the selection of the right dataset is crucial for the success of your model. Utilize platforms such as GTS.AI to discover high-quality datasets, or consider developing your own through web scraping and APIs.
With the appropriate data in hand, your machine learning project is already significantly closer to achieving success.
0 notes
Text
Data Collection Services
Fusion Digitech is a leading provider of data collection services in the US, offering accurate, reliable, and efficient data-gathering solutions for businesses across various industries. With a commitment to quality and innovation, Fusion Digitech ensures that organizations receive high-quality, real-time data to make informed decisions and drive business growth.
Our data collection services include survey data collection, web scraping, market research, field data gathering, and customer feedback analysis. We use advanced technologies, AI-driven automation, and manual verification methods to ensure the accuracy and integrity of the collected data. Whether your business needs structured or unstructured data, we tailor our services to meet your unique requirements.
At Fusion Digitech, we prioritize data security, compliance, and confidentiality, adhering to industry standards and regulations. Our expert team specializes in gathering, processing, and analyzing data from multiple sources, helping businesses improve operations, optimize marketing strategies, and enhance customer experiences.
With years of experience and a client-centric approach, Fusion Digitech is the trusted partner for businesses seeking cost-effective and scalable data collection solutions. Contact us today to discover how our services can help your organization achieve data-driven success.

0 notes