#AI Data Processing
Explore tagged Tumblr posts
Text
0 notes
Text
New aerial surveillance footage obtained by the Southern Environmental Law Center has found that Musk's artificial intelligence company, xAI, is using 35 methane gas generators to power its "Colossus" supercomputer facility, the backbone of its flagship Grok. That's 20 more generators than the 15 xAI filed permits for, and 35 more than it was approved to use.
Generators like xAI's emit huge amounts of nitrogen dioxide, a highly reactive gas which causes irreversible respiratory damage over time. And that's before you consider its effects on the ozone layer, or its contribution to acid rain, smog, and nutrient pollution in local soil and waterways. With 35 generators now chugging along, that's a whole chorus of turbines spewing the toxic gas into low-income, minority-led communities 24/7.
0 notes
Text
"Understanding Grok Technology Behind AI Databases: Revolutionizing Data Processing and Intelligence" 2025
Grok Technology: In the ever-evolving world of artificial intelligence (AI), various technologies are being developed to improve the way we process and manage data. Among the many innovations, Grok technology stands out as one of the most transformative tools, especially in the context of AI-driven databases. Grok, an advanced AI model designed to comprehend and process information deeply, has…
#AI data processing#AI databases#AI systems#AI technology#AI-powered databases#Artificial Intelligence#data processing#future of AI#Grok AI#Grok AI technology#Grok database#Grok in AI#Grok technology#Grok technology explained#Machine Learning
0 notes
Text
Enhance your business operations with AI-based data processing. This technology optimizes data management, automates repetitive tasks, and enables smarter decision-making. By leveraging AI, organizations can improve accuracy, reduce costs, and boost efficiency. Stay ahead in the competitive landscape by integrating AI-driven solutions for streamlined workflows and actionable insights. Embrace the future of data processing today!
0 notes
Text
#cogito ai#data annotation#cogitotech usa#machine learning#data collection#data quality#AI Data Processing
0 notes
Text
Nothing to do with anything else, but one of the most annoying aspects of autism is being hypersensitive to touch. I feel compelled to change into a very loose nightgown every evening because otherwise the sensation of anything against my skin by that time makes me want to claw my flesh from my body.
But, simultaneously, there's part of my mind that responds to ... like, a hug or something very small with "I yearn for human connection and some kind of touch however minimal, yay! a hug!" And meanwhile the other part is screaming "BAD BAD BAD RED ALERT BAD," like having a green and red light simultaneously flashing in my head.
Doesn't feel great, Bob!
#okay i /can/ make this about star trek: i have a tinyyyy bit of compassion for the ai monstrosities kirk talks to death#more for rayna though! (i guess he also talked her to death from a certain pov ... but you know what i mean)#but it's the same end of forcing them to run mutually exclusive systems until they start smoking. relatable#j is like 'i thought you'd relate to data' but no he is sweet and communicative and cruelty towards him is like kicking a puppy#but data contains multitudes and not easily reconcilable thought processes. rayna or m-5 or whatever frying their wiring though!#anghraine babbles#rare breed of attack unicorn#anghraine whines#star peace#autism
22 notes
·
View notes
Text
i hate gen AI so much i wish crab raves upon it
#genuinely this shit is like downfall of humanity to me#what do you mean you have a compsci degree and are having chatgpt write basic code for you#what do you mean you are using it to come up with recipes#what do you mean you are talking to it 24/7 like it’s your friend#what do you mean you are RPing with it#what do you mean you use it instead of researching anything for yourself#what do you mean you’re using it to write your essays instead of just writing your essays#i feel crazy i feel insane on god on GOD#i would have gotten a different degree if i knew that half the jobs that exist now for my degree are all feeding into the fucking gen AI#slop machine#what’s worse is my work experience is very much ‘automation engineering’ which is NOT AI but#using coding/technology/databases to improve existing processes and make them easier and less tedious for people#to free them up to do things that involve more brainpower than tedious data entry/etc#SO ESPECIALLY so many of the jobs i would have been able to take with my work experience is now very gen AI shit and i just refuse to fuckin#do that shit?????
2 notes
·
View notes
Text

Chiques fíjense de activar la opción de no compartir datos en el apartado "Visibilidad" en Ajustes ‼️‼️
#Tumblr#ai#ai generated#argie tumblr#español#artificial intelligence#consent#no sé q poner acá#cuidado#caution#data protection#data privacy#online privacy#internet privacy#invasion of privacy#data processing#anti ai#fuck ai
5 notes
·
View notes
Text
getting a samsung phone was probably one of the worst mistakes ive made tech wise in years its like having a iphone all over again except worse somehow
#at least when i had an iphone i could turn off updates indefinitely#try our new galaxy ai assistant we've already opted you in for automatic data collection and processing! fuck you!#ree talks
4 notes
·
View notes
Text
"spotify wrapped was clearly AI"
Two questions. What, exactly, do you think AI is? And did you think spotify had people HAND PICKING your top songs before this???? be for real
#like ??? it's always been computer generated#and this is such simple data analysis you would never need AI to process it#its LITERALLY just ranking songs and artists by playtime#YOU CAN CODE THAT RN BESTIE#absolutely be critical of AI but you look stupid
4 notes
·
View notes
Text
I feel like, with the uproar over Nanowrimo right now, we have an opportunity to really push back at shitty AI, but I feel like we also need to be smart about it.
Just saying "Generative AI is bad! Fuck you!" is not going to make a huge dent in shitty ai practices, because they'll just dismiss us out of hand. But if we ask the really hard hitting questions, then we might be able to start making some level of progress.
Mozilla is actually doing a ton of good work towards this very goal.
They've been working to try to shift industry goals towards more transparent, conscientious, and sustainable practices, and I think their approach has a lot of promise.
AI is not inherently bad or harmful (hells, even generative AI isn't. It's just a tool, thus neutral at its core), but harmful practices and a lack of transparency make it to where we can not fucking trust them, at least in their current iterations.
But the cat is out of the fucking bag, and its not going back in even if we do point out all the harm. Too many people like the idea of making their lives easier, and you can't deny the overwhelming potential that AI offers.
But that doesn't mean we have to tolerate the harm it currently causes.
#nanowrimo#ai#artificial intelligence#generative ai#But no. for real#I can think of a ton of ways AI can be used ethically to help the creative process without completely undermining everything it stands for#But I wouldn't dare fucking try it because who fucking knows where they're getting their training data from#and even beyond that#its abundantly clear that the people who are pushing AI use in creative endeavors (be that writing or art or whatever)#are not doing so with actual creatives in mind. they aren't trying to uplift authors or artists. they're trying to replace them#so the only people willing to use the AI are people who aren't creatives at all#which just feeds into the shitty feelings we creatives feel when looking at art or writing that utilized ai in any way
3 notes
·
View notes
Text
Deep Learning, Deconstructed: A Physics-Informed Perspective on AI’s Inner Workings
Dr. Yasaman Bahri’s seminar offers a profound glimpse into the complexities of deep learning, merging empirical successes with theoretical foundations. Dr. Bahri’s distinct background, weaving together statistical physics, machine learning, and condensed matter physics, uniquely positions her to dissect the intricacies of deep neural networks. Her journey from a physics-centric PhD at UC Berkeley, influenced by computer science seminars, exemplifies the burgeoning synergy between physics and machine learning, underscoring the value of interdisciplinary approaches in elucidating deep learning’s mysteries.
At the heart of Dr. Bahri’s research lies the intriguing equivalence between neural networks and Gaussian processes in the infinite width limit, facilitated by the Central Limit Theorem. This theorem, by implying that the distribution of outputs from a neural network will approach a Gaussian distribution as the width of the network increases, provides a probabilistic framework for understanding neural network behavior. The derivation of Gaussian processes from various neural network architectures not only yields state-of-the-art kernels but also sheds light on the dynamics of optimization, enabling more precise predictions of model performance.
The discussion on scaling laws is multifaceted, encompassing empirical observations, theoretical underpinnings, and the intricate dance between model size, computational resources, and the volume of training data. While model quality often improves monotonically with these factors, reaching a point of diminishing returns, understanding these dynamics is crucial for efficient model design. Interestingly, the strategic selection of data emerges as a critical factor in surpassing the limitations imposed by power-law scaling, though this approach also presents challenges, including the risk of introducing biases and the need for domain-specific strategies.
As the field of deep learning continues to evolve, Dr. Bahri’s work serves as a beacon, illuminating the path forward. The imperative for interdisciplinary collaboration, combining the rigor of physics with the adaptability of machine learning, cannot be overstated. Moreover, the pursuit of personalized scaling laws, tailored to the unique characteristics of each problem domain, promises to revolutionize model efficiency. As researchers and practitioners navigate this complex landscape, they are left to ponder: What unforeseen synergies await discovery at the intersection of physics and deep learning, and how might these transform the future of artificial intelligence?
Yasaman Bahri: A First-Principle Approach to Understanding Deep Learning (DDPS Webinar, Lawrence Livermore National Laboratory, November 2024)
youtube
Sunday, November 24, 2024
#deep learning#physics informed ai#machine learning research#interdisciplinary approaches#scaling laws#gaussian processes#neural networks#artificial intelligence#ai theory#computational science#data science#technology convergence#innovation in ai#webinar#ai assisted writing#machine art#Youtube
3 notes
·
View notes
Text
Why Quantum Computing Will Change the Tech Landscape
The technology industry has seen significant advancements over the past few decades, but nothing quite as transformative as quantum computing promises to be. Why Quantum Computing Will Change the Tech Landscape is not just a matter of speculation; it’s grounded in the science of how we compute and the immense potential of quantum mechanics to revolutionise various sectors. As traditional…
#AI#AI acceleration#AI development#autonomous vehicles#big data#classical computing#climate modelling#complex systems#computational power#computing power#cryptography#cybersecurity#data processing#data simulation#drug discovery#economic impact#emerging tech#energy efficiency#exponential computing#exponential growth#fast problem solving#financial services#Future Technology#government funding#hardware#Healthcare#industry applications#industry transformation#innovation#machine learning
2 notes
·
View notes
Text
How Large Language Models (LLMs) are Transforming Data Cleaning in 2024
Data is the new oil, and just like crude oil, it needs refining before it can be utilized effectively. Data cleaning, a crucial part of data preprocessing, is one of the most time-consuming and tedious tasks in data analytics. With the advent of Artificial Intelligence, particularly Large Language Models (LLMs), the landscape of data cleaning has started to shift dramatically. This blog delves into how LLMs are revolutionizing data cleaning in 2024 and what this means for businesses and data scientists.
The Growing Importance of Data Cleaning
Data cleaning involves identifying and rectifying errors, missing values, outliers, duplicates, and inconsistencies within datasets to ensure that data is accurate and usable. This step can take up to 80% of a data scientist's time. Inaccurate data can lead to flawed analysis, costing businesses both time and money. Hence, automating the data cleaning process without compromising data quality is essential. This is where LLMs come into play.
What are Large Language Models (LLMs)?
LLMs, like OpenAI's GPT-4 and Google's BERT, are deep learning models that have been trained on vast amounts of text data. These models are capable of understanding and generating human-like text, answering complex queries, and even writing code. With millions (sometimes billions) of parameters, LLMs can capture context, semantics, and nuances from data, making them ideal candidates for tasks beyond text generation—such as data cleaning.
To see how LLMs are also transforming other domains, like Business Intelligence (BI) and Analytics, check out our blog How LLMs are Transforming Business Intelligence (BI) and Analytics.

Traditional Data Cleaning Methods vs. LLM-Driven Approaches
Traditionally, data cleaning has relied heavily on rule-based systems and manual intervention. Common methods include:
Handling missing values: Methods like mean imputation or simply removing rows with missing data are used.
Detecting outliers: Outliers are identified using statistical methods, such as standard deviation or the Interquartile Range (IQR).
Deduplication: Exact or fuzzy matching algorithms identify and remove duplicates in datasets.
However, these traditional approaches come with significant limitations. For instance, rule-based systems often fail when dealing with unstructured data or context-specific errors. They also require constant updates to account for new data patterns.
LLM-driven approaches offer a more dynamic, context-aware solution to these problems.

How LLMs are Transforming Data Cleaning
1. Understanding Contextual Data Anomalies
LLMs excel in natural language understanding, which allows them to detect context-specific anomalies that rule-based systems might overlook. For example, an LLM can be trained to recognize that “N/A” in a field might mean "Not Available" in some contexts and "Not Applicable" in others. This contextual awareness ensures that data anomalies are corrected more accurately.
2. Data Imputation Using Natural Language Understanding
Missing data is one of the most common issues in data cleaning. LLMs, thanks to their vast training on text data, can fill in missing data points intelligently. For example, if a dataset contains customer reviews with missing ratings, an LLM could predict the likely rating based on the review's sentiment and content.
A recent study conducted by researchers at MIT (2023) demonstrated that LLMs could improve imputation accuracy by up to 30% compared to traditional statistical methods. These models were trained to understand patterns in missing data and generate contextually accurate predictions, which proved to be especially useful in cases where human oversight was traditionally required.
3. Automating Deduplication and Data Normalization
LLMs can handle text-based duplication much more effectively than traditional fuzzy matching algorithms. Since these models understand the nuances of language, they can identify duplicate entries even when the text is not an exact match. For example, consider two entries: "Apple Inc." and "Apple Incorporated." Traditional algorithms might not catch this as a duplicate, but an LLM can easily detect that both refer to the same entity.
Similarly, data normalization—ensuring that data is formatted uniformly across a dataset—can be automated with LLMs. These models can normalize everything from addresses to company names based on their understanding of common patterns and formats.
4. Handling Unstructured Data
One of the greatest strengths of LLMs is their ability to work with unstructured data, which is often neglected in traditional data cleaning processes. While rule-based systems struggle to clean unstructured text, such as customer feedback or social media comments, LLMs excel in this domain. For instance, they can classify, summarize, and extract insights from large volumes of unstructured text, converting it into a more analyzable format.
For businesses dealing with social media data, LLMs can be used to clean and organize comments by detecting sentiment, identifying spam or irrelevant information, and removing outliers from the dataset. This is an area where LLMs offer significant advantages over traditional data cleaning methods.
For those interested in leveraging both LLMs and DevOps for data cleaning, see our blog Leveraging LLMs and DevOps for Effective Data Cleaning: A Modern Approach.

Real-World Applications
1. Healthcare Sector
Data quality in healthcare is critical for effective treatment, patient safety, and research. LLMs have proven useful in cleaning messy medical data such as patient records, diagnostic reports, and treatment plans. For example, the use of LLMs has enabled hospitals to automate the cleaning of Electronic Health Records (EHRs) by understanding the medical context of missing or inconsistent information.
2. Financial Services
Financial institutions deal with massive datasets, ranging from customer transactions to market data. In the past, cleaning this data required extensive manual work and rule-based algorithms that often missed nuances. LLMs can assist in identifying fraudulent transactions, cleaning duplicate financial records, and even predicting market movements by analyzing unstructured market reports or news articles.
3. E-commerce
In e-commerce, product listings often contain inconsistent data due to manual entry or differing data formats across platforms. LLMs are helping e-commerce giants like Amazon clean and standardize product data more efficiently by detecting duplicates and filling in missing information based on customer reviews or product descriptions.

Challenges and Limitations
While LLMs have shown significant potential in data cleaning, they are not without challenges.
Training Data Quality: The effectiveness of an LLM depends on the quality of the data it was trained on. Poorly trained models might perpetuate errors in data cleaning.
Resource-Intensive: LLMs require substantial computational resources to function, which can be a limitation for small to medium-sized enterprises.
Data Privacy: Since LLMs are often cloud-based, using them to clean sensitive datasets, such as financial or healthcare data, raises concerns about data privacy and security.

The Future of Data Cleaning with LLMs
The advancements in LLMs represent a paradigm shift in how data cleaning will be conducted moving forward. As these models become more efficient and accessible, businesses will increasingly rely on them to automate data preprocessing tasks. We can expect further improvements in imputation techniques, anomaly detection, and the handling of unstructured data, all driven by the power of LLMs.
By integrating LLMs into data pipelines, organizations can not only save time but also improve the accuracy and reliability of their data, resulting in more informed decision-making and enhanced business outcomes. As we move further into 2024, the role of LLMs in data cleaning is set to expand, making this an exciting space to watch.
Large Language Models are poised to revolutionize the field of data cleaning by automating and enhancing key processes. Their ability to understand context, handle unstructured data, and perform intelligent imputation offers a glimpse into the future of data preprocessing. While challenges remain, the potential benefits of LLMs in transforming data cleaning processes are undeniable, and businesses that harness this technology are likely to gain a competitive edge in the era of big data.
#Artificial Intelligence#Machine Learning#Data Preprocessing#Data Quality#Natural Language Processing#Business Intelligence#Data Analytics#automation#datascience#datacleaning#large language model#ai
2 notes
·
View notes
Text
Watched a video about these "AI assistants" that Meta has launched with celebrity faces (Kendall Jenner, Snoop Dogg etc.). Somebody speculated/mentioned in the comments that eventually Meta wants to sell assistant apps to companies, but that makes ... no sense.
If they mean in the sense of a glorified search engine that gives you subtly wrong answers half the time and can't do math, sure - not that that's any different than the stuff that already exists (????)
But if they literally mean assistant, that's complete bogus. The bulk of an assistant's job is organizing things - getting stuff purchased, herding a bunch of hard-to-reach people into the same meeting, booking flights and rides, following up on important conversations. Yes, for some of these there's already an app that has automated the process to a degree. But if these processes were sufficiently automated, companies would already have phased out assistant positions. Sticking a well-read chat bot on top of Siri won't solve this.
If I ask my assistant to get me the best flight to New York, I don't want it to succeed 80 % of time and the rest of the time, book me a flight at 2 a.m. or send me to New York, Florida or put me on a flight that's 8 hours longer than necessary. And yes, you can probably optimize an app + chat bot for this specific task so it only fails 2 % of the time. But you cannot optimize a program to be good at everything–booking flights, booking car rentals, organizing catering, welcoming people at the front desk and basically any other request a human could think off. What you're looking for is a human brain and body. Humans can improvise, prioritize, make decisions, and, very importantly, interact freely with the material world. Developing a sufficiently advanced assistant is a pipe dream.
#now i understand that part of it might just be another round of hype to avoid shares dropping because it looks worse to write 'we got some#videos of kendall jenner and hope to make money off of it someday'#funnily enough no matter what complexity these assistant apps reach#it will be human assistants who use them#because the crux of having an assistant is that you DON'T have to deal with the nitty-gritty (like did the app understand my request) or#follow-up#meta#ai#post#ai assistant#the other thing to consider is when you let an app interact with a service for you that concerns spending money (like booking a flight but#really anything where money will be spent in the process) you lose power as a consumer#because you will hand over data about what you want and have to deal with the intransparency of the service#are you getting suggested the best/fastest/cheapest flight or the one from the airline that has a contract with your assistant app?#we are already seeing this with the enshittification of uber or other food or ride share apps#the company has the power to manipulate consumers and 'contractors' alike because they program the app
11 notes
·
View notes
Photo
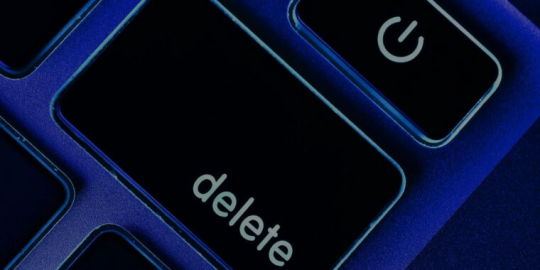
(via It’s a “fake PR stunt”: Artists hate Meta’s AI data deletion process | Ars Technica)
This August, when Meta began allowing people to submit requests to delete personal data from third parties used to train Meta’s generative AI models, many artists and journalists interpreted this new process as Meta’s very limited version of an opt-out program. CNBC explicitly referred to the request form as an “opt-out tool.”
This is a misconception. In reality, there is no functional way to opt out of Meta’s generative AI training.
Artists who have tried to use Meta’s data deletion request form have learned this the hard way and have been deeply frustrated with the process. “It was horrible,” illustrator Mignon Zakuga says. Over a dozen artists shared with WIRED an identical form letter they received from Meta in response to their queries. In it, Meta says it is “unable to process the request” until the requester submits evidence that their personal information appears in responses from Meta’s generative AI.
so - this looks like the best way to go currently...
...The tool, called Nightshade, is intended as a way to fight back against AI companies that use artists’ work to train their models without the creator’s permission.
9 notes
·
View notes