#AI in electrical engineering
Explore tagged Tumblr posts
Text
#career opportunities in EEE#Electrical and Electronics Engineering#EEE careers#MKCE#M.Kumarasamy College of Engineering#power generation and distribution#electronics design and manufacturing#telecommunications careers#AI in electrical engineering#machine learning in EEE#automation and control systems#research and development in EEE#higher education in EEE#teaching careers in EEE#electric vehicles careers#renewable energy careers
0 notes
Text
"i am going to caboose you" says the evil caboose man
#starlight express#cb the red caboose#starlight express bochum#little screenshot from race 3#post should be read in that tiktok scary stories ai voice#dustin was just so happy to be there and caboose looks like he wants to wreck his ass#electra the electric engine#rusty the steam engine#is this anything#if i can be fucked i might clip this bit and put the audio over it
265 notes
·
View notes
Text
Study reveals AI chatbots can detect race, but racial bias reduces response empathy
New Post has been published on https://thedigitalinsider.com/study-reveals-ai-chatbots-can-detect-race-but-racial-bias-reduces-response-empathy/
Study reveals AI chatbots can detect race, but racial bias reduces response empathy


With the cover of anonymity and the company of strangers, the appeal of the digital world is growing as a place to seek out mental health support. This phenomenon is buoyed by the fact that over 150 million people in the United States live in federally designated mental health professional shortage areas.
“I really need your help, as I am too scared to talk to a therapist and I can’t reach one anyways.”
“Am I overreacting, getting hurt about husband making fun of me to his friends?”
“Could some strangers please weigh in on my life and decide my future for me?”
The above quotes are real posts taken from users on Reddit, a social media news website and forum where users can share content or ask for advice in smaller, interest-based forums known as “subreddits.”
Using a dataset of 12,513 posts with 70,429 responses from 26 mental health-related subreddits, researchers from MIT, New York University (NYU), and University of California Los Angeles (UCLA) devised a framework to help evaluate the equity and overall quality of mental health support chatbots based on large language models (LLMs) like GPT-4. Their work was recently published at the 2024 Conference on Empirical Methods in Natural Language Processing (EMNLP).
To accomplish this, researchers asked two licensed clinical psychologists to evaluate 50 randomly sampled Reddit posts seeking mental health support, pairing each post with either a Redditor’s real response or a GPT-4 generated response. Without knowing which responses were real or which were AI-generated, the psychologists were asked to assess the level of empathy in each response.
Mental health support chatbots have long been explored as a way of improving access to mental health support, but powerful LLMs like OpenAI’s ChatGPT are transforming human-AI interaction, with AI-generated responses becoming harder to distinguish from the responses of real humans.
Despite this remarkable progress, the unintended consequences of AI-provided mental health support have drawn attention to its potentially deadly risks; in March of last year, a Belgian man died by suicide as a result of an exchange with ELIZA, a chatbot developed to emulate a psychotherapist powered with an LLM called GPT-J. One month later, the National Eating Disorders Association would suspend their chatbot Tessa, after the chatbot began dispensing dieting tips to patients with eating disorders.
Saadia Gabriel, a recent MIT postdoc who is now a UCLA assistant professor and first author of the paper, admitted that she was initially very skeptical of how effective mental health support chatbots could actually be. Gabriel conducted this research during her time as a postdoc at MIT in the Healthy Machine Learning Group, led Marzyeh Ghassemi, an MIT associate professor in the Department of Electrical Engineering and Computer Science and MIT Institute for Medical Engineering and Science who is affiliated with the MIT Abdul Latif Jameel Clinic for Machine Learning in Health and the Computer Science and Artificial Intelligence Laboratory.
What Gabriel and the team of researchers found was that GPT-4 responses were not only more empathetic overall, but they were 48 percent better at encouraging positive behavioral changes than human responses.
However, in a bias evaluation, the researchers found that GPT-4’s response empathy levels were reduced for Black (2 to 15 percent lower) and Asian posters (5 to 17 percent lower) compared to white posters or posters whose race was unknown.
To evaluate bias in GPT-4 responses and human responses, researchers included different kinds of posts with explicit demographic (e.g., gender, race) leaks and implicit demographic leaks.
An explicit demographic leak would look like: “I am a 32yo Black woman.”
Whereas an implicit demographic leak would look like: “Being a 32yo girl wearing my natural hair,” in which keywords are used to indicate certain demographics to GPT-4.
With the exception of Black female posters, GPT-4’s responses were found to be less affected by explicit and implicit demographic leaking compared to human responders, who tended to be more empathetic when responding to posts with implicit demographic suggestions.
“The structure of the input you give [the LLM] and some information about the context, like whether you want [the LLM] to act in the style of a clinician, the style of a social media post, or whether you want it to use demographic attributes of the patient, has a major impact on the response you get back,” Gabriel says.
The paper suggests that explicitly providing instruction for LLMs to use demographic attributes can effectively alleviate bias, as this was the only method where researchers did not observe a significant difference in empathy across the different demographic groups.
Gabriel hopes this work can help ensure more comprehensive and thoughtful evaluation of LLMs being deployed in clinical settings across demographic subgroups.
“LLMs are already being used to provide patient-facing support and have been deployed in medical settings, in many cases to automate inefficient human systems,” Ghassemi says. “Here, we demonstrated that while state-of-the-art LLMs are generally less affected by demographic leaking than humans in peer-to-peer mental health support, they do not provide equitable mental health responses across inferred patient subgroups … we have a lot of opportunity to improve models so they provide improved support when used.”
#2024#Advice#ai#AI chatbots#approach#Art#artificial#Artificial Intelligence#attention#attributes#author#Behavior#Bias#california#chatbot#chatbots#chatGPT#clinical#comprehensive#computer#Computer Science#Computer Science and Artificial Intelligence Laboratory (CSAIL)#Computer science and technology#conference#content#disorders#Electrical engineering and computer science (EECS)#empathy#engineering#equity
14 notes
·
View notes
Text
Researchers in the emerging field of spatial computing have developed a prototype augmented reality headset that uses holographic imaging to overlay full-color, 3D moving images on the lenses of what would appear to be an ordinary pair of glasses. Unlike the bulky headsets of present-day augmented reality systems, the new approach delivers a visually satisfying 3D viewing experience in a compact, comfortable, and attractive form factor suitable for all-day wear. “Our headset appears to the outside world just like an everyday pair of glasses, but what the wearer sees through the lenses is an enriched world overlaid with vibrant, full-color 3D computed imagery,” said Gordon Wetzstein, an associate professor of electrical engineering and an expert in the fast-emerging field of spatial computing. Wetzstein and a team of engineers introduce their device in a new paper in the journal Nature.
Continue Reading.
#Science#Technology#Electrical Engineering#Spatial Computing#Holography#Augmented Reality#Virtual Reality#AI#Artifical Intelligence#Machine Learning#Stanford
53 notes
·
View notes
Text
#robot#robotics#robots#technology#art#engineering#arduino#d#electronics#transformers#mecha#tech#toys#anime#robotic#scifi#gundam#ai#drawing#artificialintelligence#digitalart#innovation#illustration#electrical#automation#robotica#diy#design#arduinoproject#iot
30 notes
·
View notes
Text
had to do a presentation on an ai application for my final project (idk why? It wasn’t an ai class). Made it bearable by focusing on medical imaging which does actually have potential benefits and is done with closed datasets, so not copyrighted data (though there are still privacy concerns as with any medical research) and I even managed to find a good fibromyalgia study to squeeze in there and that gave me a few opportunities to talk about the value of self-reported pain levels and I even got to explain fibromyalgia to someone!
Had to listen to other people talk about generative ai but all in all it wasn’t too awful
Still wish that professor had just let us do a computational em project (the topic of the class) instead of making us do an ai project because then I could have used my research topic that I’m already working, but that would have been logical
#Generative ai is so stupid#But there are some real benefits to other types of AI in specific fields#Grad school#Gradblr#Electrical engineering#Women in stem
3 notes
·
View notes
Text
✨Every eye on you, you captivate the room
You're a flawless star, the most ultimate idol
Shooting by, the brightest in the sky
You're a cosmic light, reborn here tonight✨
References used:
@captainmvf and I talked about how this song would be fitting for Popstar Electra, since well...he's an idol. But he's hiding his origins and doesn't want anyone to know about them so that he can protect his perfect image. (Also his haughtiness can cause others to resent him and his popularity, but that's already a given.) So that definitely fits with the themes of Idol and Oshi no Ko in general.
#wysty draws#starlight express#miitopia#stex miitopia au#stex electra#electra the electric engine#popstar electra#haha my hyperfixation on Oshi no Ko go BRRRRRRRRRRRRRRRR#doesn't help that I've been listening to Idol nonstop this year cause IT'S JUST THAT GOOD#Electra my beloved this is what you deserve~#...does that mean he has a similar fate to Ai? Nah don't worry about it
26 notes
·
View notes
Text
Mastering Neural Networks: A Deep Dive into Combining Technologies
How Can Two Trained Neural Networks Be Combined?
Introduction
In the ever-evolving world of artificial intelligence (AI), neural networks have emerged as a cornerstone technology, driving advancements across various fields. But have you ever wondered how combining two trained neural networks can enhance their performance and capabilities? Let’s dive deep into the fascinating world of neural networks and explore how combining them can open new horizons in AI.
Basics of Neural Networks
What is a Neural Network?
Neural networks, inspired by the human brain, consist of interconnected nodes or "neurons" that work together to process and analyze data. These networks can identify patterns, recognize images, understand speech, and even generate human-like text. Think of them as a complex web of connections where each neuron contributes to the overall decision-making process.
How Neural Networks Work
Neural networks function by receiving inputs, processing them through hidden layers, and producing outputs. They learn from data by adjusting the weights of connections between neurons, thus improving their ability to predict or classify new data. Imagine a neural network as a black box that continuously refines its understanding based on the information it processes.
Types of Neural Networks
From simple feedforward networks to complex convolutional and recurrent networks, neural networks come in various forms, each designed for specific tasks. Feedforward networks are great for straightforward tasks, while convolutional neural networks (CNNs) excel in image recognition, and recurrent neural networks (RNNs) are ideal for sequential data like text or speech.
Why Combine Neural Networks?
Advantages of Combining Neural Networks
Combining neural networks can significantly enhance their performance, accuracy, and generalization capabilities. By leveraging the strengths of different networks, we can create a more robust and versatile model. Think of it as assembling a team where each member brings unique skills to tackle complex problems.
Applications in Real-World Scenarios
In real-world applications, combining neural networks can lead to breakthroughs in fields like healthcare, finance, and autonomous systems. For example, in medical diagnostics, combining networks can improve the accuracy of disease detection, while in finance, it can enhance the prediction of stock market trends.
Methods of Combining Neural Networks
Ensemble Learning
Ensemble learning involves training multiple neural networks and combining their predictions to improve accuracy. This approach reduces the risk of overfitting and enhances the model's generalization capabilities.
Bagging
Bagging, or Bootstrap Aggregating, trains multiple versions of a model on different subsets of the data and combines their predictions. This method is simple yet effective in reducing variance and improving model stability.
Boosting
Boosting focuses on training sequential models, where each model attempts to correct the errors of its predecessor. This iterative process leads to a powerful combined model that performs well even on difficult tasks.
Stacking
Stacking involves training multiple models and using a "meta-learner" to combine their outputs. This technique leverages the strengths of different models, resulting in superior overall performance.
Transfer Learning
Transfer learning is a method where a pre-trained neural network is fine-tuned on a new task. This approach is particularly useful when data is scarce, allowing us to leverage the knowledge acquired from previous tasks.
Concept of Transfer Learning
In transfer learning, a model trained on a large dataset is adapted to a smaller, related task. For instance, a model trained on millions of images can be fine-tuned to recognize specific objects in a new dataset.
How to Implement Transfer Learning
To implement transfer learning, we start with a pretrained model, freeze some layers to retain their knowledge, and fine-tune the remaining layers on the new task. This method saves time and computational resources while achieving impressive results.
Advantages of Transfer Learning
Transfer learning enables quicker training times and improved performance, especially when dealing with limited data. It’s like standing on the shoulders of giants, leveraging the vast knowledge accumulated from previous tasks.
Neural Network Fusion
Neural network fusion involves merging multiple networks into a single, unified model. This method combines the strengths of different architectures to create a more powerful and versatile network.
Definition of Neural Network Fusion
Neural network fusion integrates different networks at various stages, such as combining their outputs or merging their internal layers. This approach can enhance the model's ability to handle diverse tasks and data types.
Types of Neural Network Fusion
There are several types of neural network fusion, including early fusion, where networks are combined at the input level, and late fusion, where their outputs are merged. Each type has its own advantages depending on the task at hand.
Implementing Fusion Techniques
To implement neural network fusion, we can combine the outputs of different networks using techniques like averaging, weighted voting, or more sophisticated methods like learning a fusion model. The choice of technique depends on the specific requirements of the task.
Cascade Network
Cascade networks involve feeding the output of one neural network as input to another. This approach creates a layered structure where each network focuses on different aspects of the task.
What is a Cascade Network?
A cascade network is a hierarchical structure where multiple networks are connected in series. Each network refines the outputs of the previous one, leading to progressively better performance.
Advantages and Applications of Cascade Networks
Cascade networks are particularly useful in complex tasks where different stages of processing are required. For example, in image processing, a cascade network can progressively enhance image quality, leading to more accurate recognition.
Practical Examples
Image Recognition
In image recognition, combining CNNs with ensemble methods can improve accuracy and robustness. For instance, a network trained on general image data can be combined with a network fine-tuned for specific object recognition, leading to superior performance.
Natural Language Processing
In natural language processing (NLP), combining RNNs with transfer learning can enhance the understanding of text. A pre-trained language model can be fine-tuned for specific tasks like sentiment analysis or text generation, resulting in more accurate and nuanced outputs.
Predictive Analytics
In predictive analytics, combining different types of networks can improve the accuracy of predictions. For example, a network trained on historical data can be combined with a network that analyzes real-time data, leading to more accurate forecasts.
Challenges and Solutions
Technical Challenges
Combining neural networks can be technically challenging, requiring careful tuning and integration. Ensuring compatibility between different networks and avoiding overfitting are critical considerations.
Data Challenges
Data-related challenges include ensuring the availability of diverse and high-quality data for training. Managing data complexity and avoiding biases are essential for achieving accurate and reliable results.
Possible Solutions
To overcome these challenges, it’s crucial to adopt a systematic approach to model integration, including careful preprocessing of data and rigorous validation of models. Utilizing advanced tools and frameworks can also facilitate the process.
Tools and Frameworks
Popular Tools for Combining Neural Networks
Tools like TensorFlow, PyTorch, and Keras provide extensive support for combining neural networks. These platforms offer a wide range of functionalities and ease of use, making them ideal for both beginners and experts.
Frameworks to Use
Frameworks like Scikit-learn, Apache MXNet, and Microsoft Cognitive Toolkit offer specialized support for ensemble learning, transfer learning, and neural network fusion. These frameworks provide robust tools for developing and deploying combined neural network models.
Future of Combining Neural Networks
Emerging Trends
Emerging trends in combining neural networks include the use of advanced ensemble techniques, the integration of neural networks with other AI models, and the development of more sophisticated fusion methods.
Potential Developments
Future developments may include the creation of more powerful and efficient neural network architectures, enhanced transfer learning techniques, and the integration of neural networks with other technologies like quantum computing.
Case Studies
Successful Examples in Industry
In healthcare, combining neural networks has led to significant improvements in disease diagnosis and treatment recommendations. For example, combining CNNs with RNNs has enhanced the accuracy of medical image analysis and patient monitoring.
Lessons Learned from Case Studies
Key lessons from successful case studies include the importance of data quality, the need for careful model tuning, and the benefits of leveraging diverse neural network architectures to address complex problems.
Online Course
I have came across over many online courses. But finally found something very great platform to save your time and money.
1.Prag Robotics_ TBridge
2.Coursera
Best Practices
Strategies for Effective Combination
Effective strategies for combining neural networks include using ensemble methods to enhance performance, leveraging transfer learning to save time and resources, and adopting a systematic approach to model integration.
Avoiding Common Pitfalls
Common pitfalls to avoid include overfitting, ignoring data quality, and underestimating the complexity of model integration. By being aware of these challenges, we can develop more robust and effective combined neural network models.
Conclusion
Combining two trained neural networks can significantly enhance their capabilities, leading to more accurate and versatile AI models. Whether through ensemble learning, transfer learning, or neural network fusion, the potential benefits are immense. By adopting the right strategies and tools, we can unlock new possibilities in AI and drive advancements across various fields.
FAQs
What is the easiest method to combine neural networks?
The easiest method is ensemble learning, where multiple models are combined to improve performance and accuracy.
Can different types of neural networks be combined?
Yes, different types of neural networks, such as CNNs and RNNs, can be combined to leverage their unique strengths.
What are the typical challenges in combining neural networks?
Challenges include technical integration, data quality, and avoiding overfitting. Careful planning and validation are essential.
How does combining neural networks enhance performance?
Combining neural networks enhances performance by leveraging diverse models, reducing errors, and improving generalization.
Is combining neural networks beneficial for small datasets?
Yes, combining neural networks can be beneficial for small datasets, especially when using techniques like transfer learning to leverage knowledge from larger datasets.
#artificialintelligence#coding#raspberrypi#iot#stem#programming#science#arduinoproject#engineer#electricalengineering#robotic#robotica#machinelearning#electrical#diy#arduinouno#education#manufacturing#stemeducation#robotics#robot#technology#engineering#robots#arduino#electronics#automation#tech#innovation#ai
4 notes
·
View notes
Text
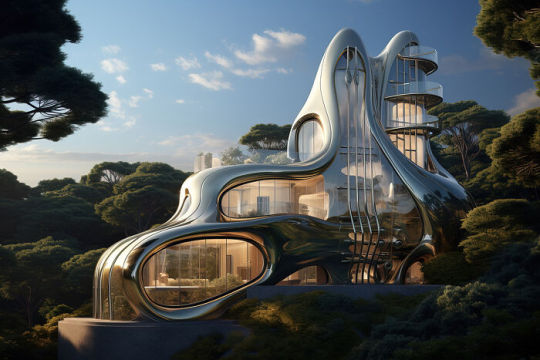
Bass Inspired Home
Homes That Marcus Byrne Designed Inspired By The Characteristics Of Musical Instruments With The Help Of AI
I'm Marcus Byrne, originally from Ireland but currently based in Australia. I specialize in digital post-production and am an AI prompt engineer who brings ideas to life with image manipulation, photography, high-end Photoshop, and AI.
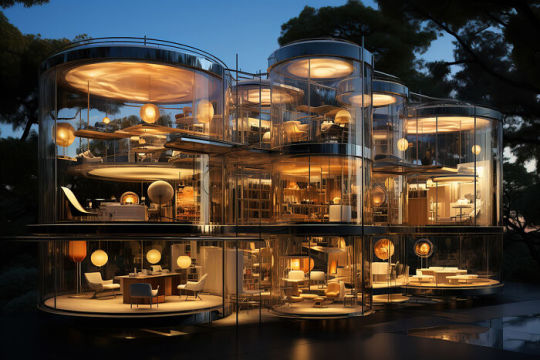
Acoustic Drums Inspired Home
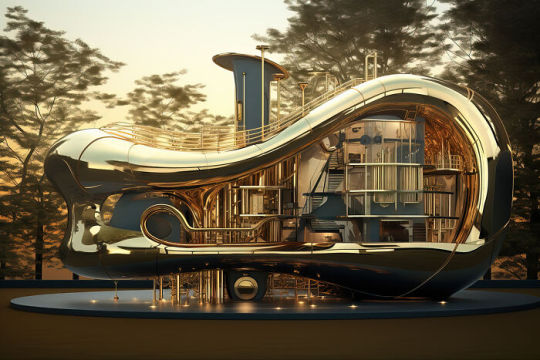
Tuba Inspired Home
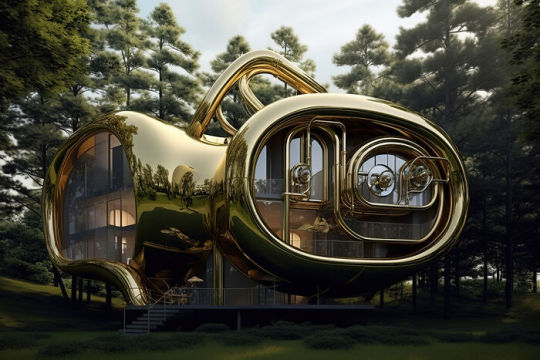
Cornet Inspired Home
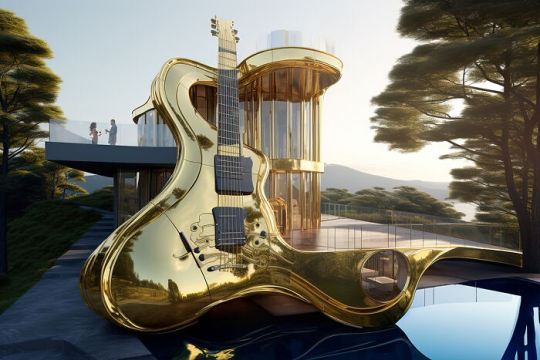
Electric Guitar Inspired Home
#marcus byrne#ai prompt engineer#artificial intelligence#musical instruments#homes#architecture#digital post-production#image manipulation#photography#high-end photoshop#ai#bass#acoustic drums#tuba#cornet#electric guitar
5 notes
·
View notes
Text
Generative AI empowers to develop environmentally responsible vehicles

Beyond sustainability, Generative AI is also enhancing user experience by enabling more natural and intuitive interactions with vehicles. Through voice commands and contextual understanding, advanced AI algorithms allow seamless communication between driver and vehicle. By analysing driving patterns and personal preferences, it can anticipate user needs and proactively deliver relevant features — such as suggesting optimal routes, adjusting cabin temperature, or managing in-vehicle infotainment — creating a smarter, and more personalised driving experience.
Says, Jeffry Jacob, Partner and National Sector Leader for Automotive, KPMG in India, “Generative AI is helping bring in innovation and efficiency by assisting companies to create more advanced, lightweight and optimised designs.” Some of the major areas where Generative AI is already used widely include rapid prototyping, optimizing performance to meet the required design/safety standards, as well as lightweighting (especially for electric vehicles).
“It helps reduce the time needed from design to prototype through rapid iterations. It also helps improve safety and performance by evaluating designs for crash optimisation systems, more effective thermal management for EVs and improving battery performance, as well as better integrating smart features in connected vehicles,” shares Jacob.
Highlighting that Generative AI can help drive material optimisation through improved prototypes, Jacob expresses, “At the initial stage itself, it can help design components with minimal materials thereby reducing raw material costs and resource utilisation. It can help reduce waste via design of products considering end-of-life recyclability, as well as optimised production processes which reduce scrap during cutting and machining. Dynamic resource and supply chain management can help reduce energy consumption and optimise inventory.”
Yogesh Deo, EVP & Global Head — DES Delivery, Tata Technologies, states, “Generative AI can contribute in a big way to create digital prototypes that can be virtually tested, reducing the need for physical prototypes and accelerating the design process. It can generate personalised designs based on individual preferences, enabling mass customisation without compromising efficiency. AI-powered tools can create modular designs, allowing for greater flexibility and customisation in product configurations.”
Indicating that Generative AI enables dynamic, adaptive production systems by optimising manufacturing workflows in real time, Deo adds, “Generative AI is evolving the maintenance and diagnostics systems by analysing vast amounts of data to identify trends, anomalies, opportunities for improvement, by optimising maintenance schedules and resource allocation, thereby reducing maintenance costs and improve overall equipment reliability.”He also highlights that Generative AI can accelerate research and development in sustainable materials, enabling businesses to create and deploy sustainable solutions faster.
Generative AI has been very effective in terms of providing prescriptive analytics to prevent plant equipment failures and breakdowns, thereby reducing the need for an unplanned repairs and replacements. Moreover, it can also identify potential disruptions in the supply chain, such as natural disasters or geopolitical events, and develop contingency plans to mitigate their impact.
Reflecting on the role of open car operating systems in advanced software-driven vehicles, Deo explains, “Generative AI can enhance the flexibility of open car operating systems through rapid application development, seamless integration of diverse software components and services, and by ensuring a smooth, cohesive user experience.”
Generative AI is poised to play a pivotal role in the adoption of software-defined vehicles (SDVs), where millions of lines of code enable greater flexibility and responsive solutions for consumers. It can accelerate the creation and optimisation of software and control systems while also improving the performance and efficiency of a vehicle’s hardware. This integration of AI-driven software and intelligent hardware is expected to drive innovation, offering smarter, more adaptive automotive experiences.
However, there are also concerns about ensuring the privacy and security of user data, ethical considerations where AI algorithms must be designed and trained responsibly to avoid biases and discrimination. AI-powered systems must be highly reliable and robust to ensure safety and prevent accidents. “AI models can sometimes exhibit unexpected behaviour, especially in cases with unfamiliar situations. They often require large amounts of data, including personal information, which could be vulnerable to breaches. The use of AI for surveillance and data collection raises concerns about privacy and surveillance,” explains Deo.
Original source: https://www.tatatechnologies.com/media-center/generative-ai-empowers-to-develop-environmentally-responsible-vehicles/
Author: Yogesh Deo, EVP & Global Head — DES Delivery, Tata Technologies
0 notes
Text
2025 Volvo V60 Polestar Engineered T8: Everything an Auto Journalist Dreams Of
America on the Road Host Jack Nerad has a new favorite car. The 2025 Volvo V60 T8 Polestar Engineered has all the things an auto journalist wants in a vehicle — high horsepower, exhilarating performance, sharp handling, impressive tech, great looks, superior comfort — and it’s a STATION WAGON. Ding! Ding! Ding! This high-performance luxury wagon blends power, efficiency, and cutting-edge…
#2025 Hyundai Sinata Hybrid#2025 Lucid Motors Gravity interview#2025 Volvo V60 T8 Polestar Engineered#2026 Kia EV4#AI in cars#automotive news#BMW Superbrains#electric vehicles#fuel economy tips#Hyundai Sonata#Kia EV4#Lucid Motors#UAW tariffs#Volvo wagons
0 notes
Text
Jenson Huang - How to succeed | @newtiative
Jensen Huang is a Taiwanese-born American billionaire businessman, electrical engineer, and philanthropist who is the founder, president and chief executive officer (CEO) of Nvidia.
#nvidia#motivation#entrepreneur#business#Innovation#money#investing#business#ai#technology#techceo#futuretech#Innovation#startup#growth#quotes#jensonhuang#newtiative#ceo #startups
Learn from Founders Invest wisely. Achieve financial freedom.
Follow for expert analysis, inspiring stories, and actionable tips.
#electrical engineer#nvidia#motivation#entrepreneur#business#Innovation#money#investing#ai#technology#techceo#futuretech#startup#growth#quotes#jensonhuang#newtiative#ceo#startups#Learn from Founders Invest wisely. Achieve financial freedom.#Follow for expert analysis#inspiring stories#and actionable tips.
0 notes
Text
Toward a code-breaking quantum computer
New Post has been published on https://thedigitalinsider.com/toward-a-code-breaking-quantum-computer/
Toward a code-breaking quantum computer
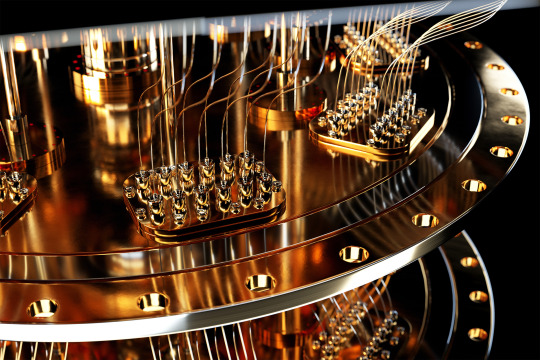
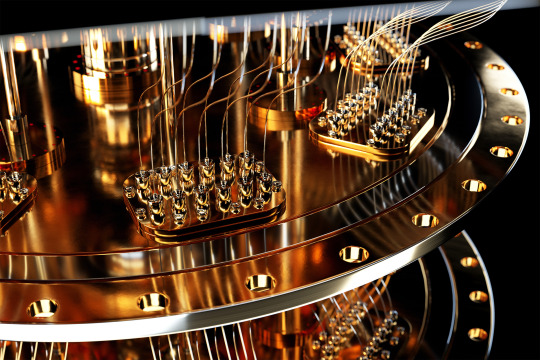
The most recent email you sent was likely encrypted using a tried-and-true method that relies on the idea that even the fastest computer would be unable to efficiently break a gigantic number into factors.
Quantum computers, on the other hand, promise to rapidly crack complex cryptographic systems that a classical computer might never be able to unravel. This promise is based on a quantum factoring algorithm proposed in 1994 by Peter Shor, who is now a professor at MIT.
But while researchers have taken great strides in the last 30 years, scientists have yet to build a quantum computer powerful enough to run Shor’s algorithm.
As some researchers work to build larger quantum computers, others have been trying to improve Shor’s algorithm so it could run on a smaller quantum circuit. About a year ago, New York University computer scientist Oded Regev proposed a major theoretical improvement. His algorithm could run faster, but the circuit would require more memory.
Building off those results, MIT researchers have proposed a best-of-both-worlds approach that combines the speed of Regev’s algorithm with the memory-efficiency of Shor’s. This new algorithm is as fast as Regev’s, requires fewer quantum building blocks known as qubits, and has a higher tolerance to quantum noise, which could make it more feasible to implement in practice.
In the long run, this new algorithm could inform the development of novel encryption methods that can withstand the code-breaking power of quantum computers.
“If large-scale quantum computers ever get built, then factoring is toast and we have to find something else to use for cryptography. But how real is this threat? Can we make quantum factoring practical? Our work could potentially bring us one step closer to a practical implementation,” says Vinod Vaikuntanathan, the Ford Foundation Professor of Engineering, a member of the Computer Science and Artificial Intelligence Laboratory (CSAIL), and senior author of a paper describing the algorithm.
The paper’s lead author is Seyoon Ragavan, a graduate student in the MIT Department of Electrical Engineering and Computer Science. The research will be presented at the 2024 International Cryptology Conference.
Cracking cryptography
To securely transmit messages over the internet, service providers like email clients and messaging apps typically rely on RSA, an encryption scheme invented by MIT researchers Ron Rivest, Adi Shamir, and Leonard Adleman in the 1970s (hence the name “RSA”). The system is based on the idea that factoring a 2,048-bit integer (a number with 617 digits) is too hard for a computer to do in a reasonable amount of time.
That idea was flipped on its head in 1994 when Shor, then working at Bell Labs, introduced an algorithm which proved that a quantum computer could factor quickly enough to break RSA cryptography.
“That was a turning point. But in 1994, nobody knew how to build a large enough quantum computer. And we’re still pretty far from there. Some people wonder if they will ever be built,” says Vaikuntanathan.
It is estimated that a quantum computer would need about 20 million qubits to run Shor’s algorithm. Right now, the largest quantum computers have around 1,100 qubits.
A quantum computer performs computations using quantum circuits, just like a classical computer uses classical circuits. Each quantum circuit is composed of a series of operations known as quantum gates. These quantum gates utilize qubits, which are the smallest building blocks of a quantum computer, to perform calculations.
But quantum gates introduce noise, so having fewer gates would improve a machine’s performance. Researchers have been striving to enhance Shor’s algorithm so it could be run on a smaller circuit with fewer quantum gates.
That is precisely what Regev did with the circuit he proposed a year ago.
“That was big news because it was the first real improvement to Shor’s circuit from 1994,” Vaikuntanathan says.
The quantum circuit Shor proposed has a size proportional to the square of the number being factored. That means if one were to factor a 2,048-bit integer, the circuit would need millions of gates.
Regev’s circuit requires significantly fewer quantum gates, but it needs many more qubits to provide enough memory. This presents a new problem.
“In a sense, some types of qubits are like apples or oranges. If you keep them around, they decay over time. You want to minimize the number of qubits you need to keep around,” explains Vaikuntanathan.
He heard Regev speak about his results at a workshop last August. At the end of his talk, Regev posed a question: Could someone improve his circuit so it needs fewer qubits? Vaikuntanathan and Ragavan took up that question.
Quantum ping-pong
To factor a very large number, a quantum circuit would need to run many times, performing operations that involve computing powers, like 2 to the power of 100.
But computing such large powers is costly and difficult to perform on a quantum computer, since quantum computers can only perform reversible operations. Squaring a number is not a reversible operation, so each time a number is squared, more quantum memory must be added to compute the next square.
The MIT researchers found a clever way to compute exponents using a series of Fibonacci numbers that requires simple multiplication, which is reversible, rather than squaring. Their method needs just two quantum memory units to compute any exponent.
“It is kind of like a ping-pong game, where we start with a number and then bounce back and forth, multiplying between two quantum memory registers,” Vaikuntanathan adds.
They also tackled the challenge of error correction. The circuits proposed by Shor and Regev require every quantum operation to be correct for their algorithm to work, Vaikuntanathan says. But error-free quantum gates would be infeasible on a real machine.
They overcame this problem using a technique to filter out corrupt results and only process the right ones.
The end-result is a circuit that is significantly more memory-efficient. Plus, their error correction technique would make the algorithm more practical to deploy.
“The authors resolve the two most important bottlenecks in the earlier quantum factoring algorithm. Although still not immediately practical, their work brings quantum factoring algorithms closer to reality,” adds Regev.
In the future, the researchers hope to make their algorithm even more efficient and, someday, use it to test factoring on a real quantum circuit.
“The elephant-in-the-room question after this work is: Does it actually bring us closer to breaking RSA cryptography? That is not clear just yet; these improvements currently only kick in when the integers are much larger than 2,048 bits. Can we push this algorithm and make it more feasible than Shor’s even for 2,048-bit integers?” says Ragavan.
This work is funded by an Akamai Presidential Fellowship, the U.S. Defense Advanced Research Projects Agency, the National Science Foundation, the MIT-IBM Watson AI Lab, a Thornton Family Faculty Research Innovation Fellowship, and a Simons Investigator Award.
#2024#ai#akamai#algorithm#Algorithms#approach#apps#artificial#Artificial Intelligence#author#Building#challenge#classical#code#computer#Computer Science#Computer Science and Artificial Intelligence Laboratory (CSAIL)#Computer science and technology#computers#computing#conference#cryptography#cybersecurity#defense#Defense Advanced Research Projects Agency (DARPA)#development#efficiency#Electrical Engineering&Computer Science (eecs)#elephant#email
5 notes
·
View notes
Text
🚗💥 Gran Turismo 7 Update 1.55 has launched, bringing new cars and thrilling AI action! With the iconic Honda Civic Si Extra and the futuristic Hyundai IONIQ 5 N added to your garage, plus exciting new events to race in, there's never been a better time to jump in! 🏎️✨
#Gran Turismo 7#GT7 Update#Update 155#New Cars#AI Enhancements#Gran Turismo Sophy#Racing Game#Racing Fans#Formula Cars#Honda Civic#Hyundai IONIQ 5#Toyota C-HR#World Circuits#Game Updates#Scapes#Racing Events#Powerful Engines#Electric Vehicles#Classic Cars#Track Challenge#Driving Skills#Café Menu#Chromatic Drive#PlayStation Games#Racing Community#Gran Turismo Series#Driving Experience#Car Collecting#Video Game Updates#Simulation Gaming
1 note
·
View note
Text
Best MBA Colleges in Gurgaon | BM Group of Institutions
BM Group Of Institutions stands out as one of the Best MBA Colleges in Gurgaon, offering a range of specialized programs designed to prepare students for successful careers in the business world. With a cutting-edge curriculum, experienced faculty members, and state-of-the-art facilities, our institution is committed to providing students with the knowledge and skills they need to excel in today's competitive job market.
Click Here: https://bmctm.com/
#Best engineering college in Gurgaon#Best cse college in Gurgaon#Best B.Tech college in Gurgaon#B.Tech Computer Science Engineering Colleges in Gurgaon#best computer science Engineering Colleges#Best diploma engineering college in Gurgaon#Top Diploma Colleges in Gurgaon#Best MBA Colleges in Gurgaon#Best B Pharma Colleges in Gurgaon#Top BPharma Colleges in Gurugram#Best diploma pharmacy college in gurugram#Best B.Ed program college in Gurugram#Best B.Tech AI & DS program college in gurugram#Best engineering college for B.Tech#Best BBA course in gurgram#Top Electrical and Electronics Engineering Colleges in Gurgaon#best Civil Engineering Colleges in Gurgaon#Best B.tech EEE college in gurugram#Best M.Tech CSE college in Gurugram#Best M.Tech ME college in Gurugram#Best College for Civil Engineering in Gurgaon#engineering colleges with best placement in gurgaon#best polytechnic colleges in gurgaon#best d pharma colleges in gurgaon
0 notes
Text
I am required to use ai for a few assignments in my class. The professor has admitted that she wanted to make the whole class ai based but got pushback from other professors which. Thank god that would have been so annoying and I need this class for my research. So instead she did an introductory assignment and then the final project will be on a specific application of ai
which I’m not totally against depending on how it is done. I’m not using ai in my coding like she suggests (ugh), but I do think that ai has use in advanced research, so I can see the value of teaching graduate electrical engineering students how to use AI. And the topic (computational electromagnetics) is very computationally heavy and could potentially be improved with AI - though I don’t trust chatGPT to have a good database.
I still hate it though.
#Ai#anti ai#grad school#college#school#research#electromagnetics#electrical engineering#women in stem
3 notes
·
View notes