#ApacheAirflow
Explore tagged Tumblr posts
Text
The Best Open-Source Tools for Data Science in 2025

Data science in 2025 is thriving, driven by a robust ecosystem of open-source tools that empower professionals to extract insights, build predictive models, and deploy data-driven solutions at scale. This year, the landscape is more dynamic than ever, with established favorites and emerging contenders shaping how data scientists work. Here’s an in-depth look at the best open-source tools that are defining data science in 2025.
1. Python: The Universal Language of Data Science
Python remains the cornerstone of data science. Its intuitive syntax, extensive libraries, and active community make it the go-to language for everything from data wrangling to deep learning. Libraries such as NumPy and Pandas streamline numerical computations and data manipulation, while scikit-learn is the gold standard for classical machine learning tasks.
NumPy: Efficient array operations and mathematical functions.
Pandas: Powerful data structures (DataFrames) for cleaning, transforming, and analyzing structured data.
scikit-learn: Comprehensive suite for classification, regression, clustering, and model evaluation.
Python’s popularity is reflected in the 2025 Stack Overflow Developer Survey, with 53% of developers using it for data projects.
2. R and RStudio: Statistical Powerhouses
R continues to shine in academia and industries where statistical rigor is paramount. The RStudio IDE enhances productivity with features for scripting, debugging, and visualization. R’s package ecosystem—especially tidyverse for data manipulation and ggplot2 for visualization—remains unmatched for statistical analysis and custom plotting.
Shiny: Build interactive web applications directly from R.
CRAN: Over 18,000 packages for every conceivable statistical need.
R is favored by 36% of users, especially for advanced analytics and research.
3. Jupyter Notebooks and JupyterLab: Interactive Exploration
Jupyter Notebooks are indispensable for prototyping, sharing, and documenting data science workflows. They support live code (Python, R, Julia, and more), visualizations, and narrative text in a single document. JupyterLab, the next-generation interface, offers enhanced collaboration and modularity.
Over 15 million notebooks hosted as of 2025, with 80% of data analysts using them regularly.
4. Apache Spark: Big Data at Lightning Speed
As data volumes grow, Apache Spark stands out for its ability to process massive datasets rapidly, both in batch and real-time. Spark’s distributed architecture, support for SQL, machine learning (MLlib), and compatibility with Python, R, Scala, and Java make it a staple for big data analytics.
65% increase in Spark adoption since 2023, reflecting its scalability and performance.
5. TensorFlow and PyTorch: Deep Learning Titans
For machine learning and AI, TensorFlow and PyTorch dominate. Both offer flexible APIs for building and training neural networks, with strong community support and integration with cloud platforms.
TensorFlow: Preferred for production-grade models and scalability; used by over 33% of ML professionals.
PyTorch: Valued for its dynamic computation graph and ease of experimentation, especially in research settings.
6. Data Visualization: Plotly, D3.js, and Apache Superset
Effective data storytelling relies on compelling visualizations:
Plotly: Python-based, supports interactive and publication-quality charts; easy for both static and dynamic visualizations.
D3.js: JavaScript library for highly customizable, web-based visualizations; ideal for specialists seeking full control.
Apache Superset: Open-source dashboarding platform for interactive, scalable visual analytics; increasingly adopted for enterprise BI.
Tableau Public, though not fully open-source, is also popular for sharing interactive visualizations with a broad audience.
7. Pandas: The Data Wrangling Workhorse
Pandas remains the backbone of data manipulation in Python, powering up to 90% of data wrangling tasks. Its DataFrame structure simplifies complex operations, making it essential for cleaning, transforming, and analyzing large datasets.
8. Scikit-learn: Machine Learning Made Simple
scikit-learn is the default choice for classical machine learning. Its consistent API, extensive documentation, and wide range of algorithms make it ideal for tasks such as classification, regression, clustering, and model validation.
9. Apache Airflow: Workflow Orchestration
As data pipelines become more complex, Apache Airflow has emerged as the go-to tool for workflow automation and orchestration. Its user-friendly interface and scalability have driven a 35% surge in adoption among data engineers in the past year.
10. MLflow: Model Management and Experiment Tracking
MLflow streamlines the machine learning lifecycle, offering tools for experiment tracking, model packaging, and deployment. Over 60% of ML engineers use MLflow for its integration capabilities and ease of use in production environments.
11. Docker and Kubernetes: Reproducibility and Scalability
Containerization with Docker and orchestration via Kubernetes ensure that data science applications run consistently across environments. These tools are now standard for deploying models and scaling data-driven services in production.
12. Emerging Contenders: Streamlit and More
Streamlit: Rapidly build and deploy interactive data apps with minimal code, gaining popularity for internal dashboards and quick prototypes.
Redash: SQL-based visualization and dashboarding tool, ideal for teams needing quick insights from databases.
Kibana: Real-time data exploration and monitoring, especially for log analytics and anomaly detection.
Conclusion: The Open-Source Advantage in 2025
Open-source tools continue to drive innovation in data science, making advanced analytics accessible, scalable, and collaborative. Mastery of these tools is not just a technical advantage—it’s essential for staying competitive in a rapidly evolving field. Whether you’re a beginner or a seasoned professional, leveraging this ecosystem will unlock new possibilities and accelerate your journey from raw data to actionable insight.
The future of data science is open, and in 2025, these tools are your ticket to building smarter, faster, and more impactful solutions.
#python#r#rstudio#jupyternotebook#jupyterlab#apachespark#tensorflow#pytorch#plotly#d3js#apachesuperset#pandas#scikitlearn#apacheairflow#mlflow#docker#kubernetes#streamlit#redash#kibana#nschool academy#datascience
0 notes
Text
IBM Watsonx.data Offers VSCode, DBT & Airflow Dataops Tools
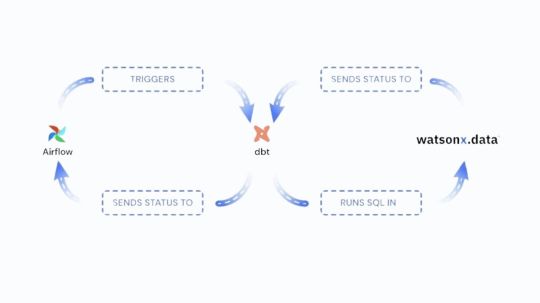
We are happy to inform that VSCode, Apache Airflow, and data-build-tool a potent set of tools for the contemporary dataops stack are now supported by IBM watsonx.data. IBM Watsonx.data delivers a new set of rich capabilities, including data build tool (dbt) compatibility for both Spark and Presto engines, automated orchestration with Apache Airflow, and an integrated development environment via VSCode. These functionalities enable teams to effectively construct, oversee, and coordinate data pipelines.
The difficulty with intricate data pipelines
Building and maintaining complicated data pipelines that depend on several engines and environments is a challenge that organizations must now overcome. Teams must continuously move between different languages and tools, which slows down development and adds complexity.
It can be challenging to coordinate workflows across many platforms, which can result in inefficiencies and bottlenecks. Data delivery slows down in the absence of a smooth orchestration tool, which postpones important decision-making.
A coordinated strategy
Organizations want a unified, efficient solution that manages process orchestration and data transformations in order to meet these issues. Through the implementation of an automated orchestration tool and a single, standardized language for transformations, teams can streamline their workflows, facilitating communication and lowering the difficulty of pipeline maintenance. Here’s where Apache Airflow and DBT come into play.
Teams no longer need to learn more complicated languages like PySpark or Scala because dbt makes it possible to develop modular structured query language (SQL) code for data transformations. The majority of data teams are already familiar with SQL, thus database technology makes it easier to create, manage, and update transformations over time.
Throughout the pipeline, Apache Airflow automates and schedules jobs to minimize manual labor and lower mistake rates. When combined, dbt and Airflow offer a strong framework for easier and more effective management of complicated data pipelines.
Utilizing IBM watsonx.data to tie everything together
Although strong solutions like Apache Airflow and DBT are available, managing a developing data ecosystem calls for more than just a single tool. IBM Watsonx.data adds the scalability, security, and dependability of an enterprise-grade platform to the advantages of these tools. Through the integration of VSCode, Airflow, and DBT within watsonx.data, it has developed a comprehensive solution that makes complex data pipeline management easier:
By making data transformations with SQL simpler, dbt assists teams in avoiding the intricacy of less used languages.
By automating orchestration, Airflow streamlines processes and gets rid of bottlenecks.
VSCode offers developers a comfortable environment that improves teamwork and efficiency.
This combination makes pipeline management easier, freeing your teams to concentrate on what matters most: achieving tangible business results. IBM Watsonx.data‘s integrated solutions enable teams to maintain agility while optimizing data procedures.
Data Build Tool’s Spark adaptor
The data build tool (dbt) adapter dbt-watsonx-spark is intended to link Apache Spark with dbt Core. This adaptor facilitates Spark data model development, testing, and documentation.
FAQs
What is data build tool?
A transformation workflow called dbt enables you to complete more tasks with greater quality. Dbt can help you centralize and modularize your analytics code while giving your data team the kind of checks and balances that are usually seen in software engineering workflows. Before securely delivering data models to production with monitoring and visibility, work together on them, version them, test them, and record your queries.
DBT allows you and your team to work together on a single source of truth for metrics, insights, and business definitions by compiling and running your analytics code against your data platform. Having a single source of truth and the ability to create tests for your data helps to minimize errors when logic shifts and notify you when problems occur.
Read more on govindhtech.com
#IBMWatsonx#dataOffer#VSCode#DBT#data#ApacheSpark#ApacheAirflow#Watsonxdata#DataopsTools#databuildtool#Sparkadaptor#UtilizingIBMwatsonxdata#technology#technews#news#govindhteh
0 notes
Photo

Reposted from @godatadriven Win a ticket for PyData Festival Amsterdam. Leave your comment in this post to secure your chance to win. https://amsterdam.pydata.org/ . . #training #datascience #python #productowner #apacheairflow #apache #virtualclass #aistrategy #techamsterdam #dataplatform #aiapplication #onlinedata #amsterdam #amsterdamfestival #techfestival #pythonprogramming #techandtechie #pydata #techworkshop #techtalk #techinterview #opensource #computing #cloudcomputing #datascientist #dataengineer #dataanalytics #dataanalyst #netherlands (at Amsterdam, Netherlands) https://www.instagram.com/p/CBc5349Jwwe/?igshid=mbym8mqau2ca
#training#datascience#python#productowner#apacheairflow#apache#virtualclass#aistrategy#techamsterdam#dataplatform#aiapplication#onlinedata#amsterdam#amsterdamfestival#techfestival#pythonprogramming#techandtechie#pydata#techworkshop#techtalk#techinterview#opensource#computing#cloudcomputing#datascientist#dataengineer#dataanalytics#dataanalyst#netherlands
0 notes
Text
Data Privacy: A runbook for engineers
#DataPrivacy #Arunbookforengineers #DataPipelines #ApacheAirflow #Kindle
0 notes
Link
The Ultimate Docker Containers Developer Course https://t.co/fK5rghr9uS#devops #kubernetes #docker #serverless #nodejs #apacheairflow #kubecon #deeplearning #CloudNative #csharp #code #Engineering #womenwhocode #udemycoupon pic.twitter.com/aQQlAVP9ax
— BlackfreeNiken (@BlackfreeNiken) March 6, 2020
via: https://ift.tt/1GAs5mb
0 notes
Text
How Can We Operate Airflow Apache On Google Cloud?
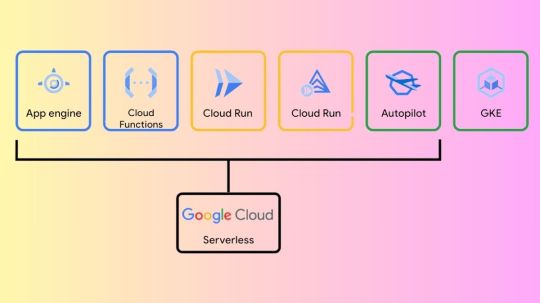
Apache Airflow on Google Cloud
Are you considering utilizing Google Cloud to run Apache Airflow? This is a well-liked option for managing intricate sequences of operations, such Extract, Transform, and Load (ETL) or pipelines for data analytics. Airflow Apache is a sophisticated tool for scheduling and dependency graphing. It employs a Directed Acyclic Graph (DAG) to arrange and relate many tasks for your workflows, including scheduling the required activity to run at a specific time.
What are the various configuration options for Airflow Apache on Google Cloud? Making the incorrect decision could result in lower availability or more expenses. You could need to construct multiple environments, such as dev, staging, and prod, or the infrastructure could fail. It will examine three methods for using Airflow Apache on Google Cloud in this post and go over the benefits and drawbacks of each. It offer Terraform code, which is available on GitHub, for every method so you may give it a try.
It should be noted that this article’s Terraform has a directory structure. The format of the files under modules is the same as that of the Terraform default code. Consider the modules directory to be a type of library if you work as a developer. The real business code is stored in the main.tf file. Assume you are working on development: begin with main.tf and save the shared code in folders such as modules, libraries, etc.)
Apache Airflow best practices
Let’s examine three methods for utilizing Airflow Apache.
Compute Engine
Installing and using Airflow directly on a Compute Engine virtual machine instance is a popular method for using Airflow on Google Cloud. The benefits of this strategy are as follows:
It costs less than the others.
All you need to know about virtual machines.
Nevertheless, there are drawbacks as well:
The virtual computer needs to be maintained by you.
There is less of it available.
Although there can be significant drawbacks, Compute Engine can be used to quickly prove of concept Airflow adoption.
First, use the following terraform code to construct a Compute Engine instance (some code has been eliminated for brevity). Allow is a firewall configuration. Since Airflow Web uses port 8080 by default, it ought to be open. You are welcome to modify the other options.
main.tf
module “gcp_compute_engine” { source = “./modules/google_compute_engine” service_name = local.service_name
region = local.region zone = local.zone machine_type = “e2-standard-4” allow = { … 2 = { protocol = “tcp” ports = [“22”, “8080”] } } }
The code and files that take the variables it handed in before and actually build an instance for it was found in the google_compute_engine directory, which it reference as source in main.tf above. Take note of how it takes in the machine_type.
modules/google_compute_engine/google_compute_instance.tf
resource “google_compute_instance” “default” { name = var.service_name machine_type = var.machine_type zone = var.zone … }
Use Terraform to run the code you wrote above:
$ terraform apply
A Compute Engine instance will be created after a short wait. The next step is to install Airflow by connecting to the instance; Launch Airflow after installation.
You can now use your browser to access Airflow! You will need to take extra precautions with your firewall settings if you intend to run Airflow on Compute Engine. It should only be accessible to authorized users, even in the event that the password is compromised. It has only made the sample accessible with the barest minimum of firewall settings.
You ought to get a screen similar after logging in. Additionally, an example DAG from Airflow is displayed. Examine the contents of the screen.
GKE Autopilot
Using Google Kubernetes Engine (GKE), Google’s managed Kubernetes service, running Airflow Apache on Google Cloud is made extremely simple. Additionally, you have the option to operate in GKE Autopilot mode, which will automatically scale your cluster according to your demands and assist you avoid running out of compute resources. You can manage your own Kubernetes nodes without having to do so because GKE Autopilot is serverless.
GKE Autopilot provides scalability and high availability. Additionally, you may make use of the robust Kubernetes ecosystem. For instance, you can monitor workloads in addition to other business services in your cluster using the kubectl command, which allows for fine-grained control over workloads. However, if you’re not particularly knowledgeable with Kubernetes, utilizing this method may result in you spending a lot of time managing Kubernetes rather than concentrating on Airflow.
Cloud Composer
Using Cloud Composer, a fully managed data workflow orchestration service on Google Cloud, is the third option. As a managed service, Cloud Composer simplifies the Airflow installation process, relieving you of the burden of maintaining the Airflow infrastructure. But it offers fewer choices. One unusual scenario is that storage cannot be shared throughout DAGs. Because you don’t have as much control over CPU and memory utilization, you might also need to make sure you balance those usages.
Conclude
Three considerations must be made if you plan to use Airflow in production: availability, performance, and cost. Three distinct approaches of running Airflow Apache on Google Cloud have been covered in this post; each has advantages and disadvantages of its own.
Remember that these are the requirements at the very least for selecting an Airflow environment. It could be enough to write some Python code to generate a DAG if you’re using Airflow for a side project. But in order to execute Airflow in production, you’ll also need to set up the Executor (LocalExecutor, CeleryExecutor, KubernetesExecutor, etc.), Airflow Core (concurrency, parallelism, SQL Pool size, etc.), and other components as needed.
Read more on Govindhtech.com
#Google#googlecloud#apacheairflow#computeengine#GKEautopilot#cloudcomposer#govindhtech#news#technews#Technology#technologynews#technologytrends
0 notes
Text
Google Cloud Composer Airflow DAG And Task Concurrency

Google Cloud Composer
One well-liked solution for coordinating data operations is Apache Airflow. Authoring, scheduling, and monitoring pipelines is made possible with Google Cloud’s fully managed workflow orchestration solution, Google Cloud Composer, which is based on Apache Airflow.
Apache Airflow DAG
The subtleties of DAG (Directed Acyclic Graph) and task concurrency can be frightening, despite Airflow’s widespread use and ease of use. This is because an Airflow installation involves several different components and configuration settings. Your data pipelines’ fault-tolerance, scalability, and resource utilisation are all improved by comprehending and putting concurrent methods into practice. The goal of this guide is to cover all the ground on Airflow concurrency at four different levels:
The Composer Environment
Installation of Airflow
DAG
Task
You can see which parameters need to be changed to make sure your Airflow tasks function exactly as you intended by referring to the visualisations in each section. Now let’s get going!
The environment level parameters for Cloud Composer 2
This represents the complete Google Cloud service. The managed infrastructure needed to run Airflow is entirely included, and it also integrates with other Google Cloud services like Cloud Monitoring and Cloud Logging. The DAGs, Tasks, and Airflow installation will inherit the configurations at this level.
Minimum and maximum number of workers
You will define the minimum and maximum numbers of Airflow Workers as well as the Worker size (processor, memory, and storage) while creating a Google Cloud Composer environment. The value of worker_concurrency by default will be set by these configurations.
Concurrency among workers
Usually, a worker with one CPU can manage twelve tasks at once. The default worker concurrency value on Cloud Composer 2 is equivalent to:
A minimal value out of 32, 12 * worker_CPU and 8 * worker_memory in Airflow 2.3.3 and later versions.
Versions of Airflow prior to 2.3.3 have 12 * worker_CPU.
For example:
Small Composer environment:
worker_cpu = 0.5
worker_mem = 2
worker_concurrency = min(32, 12*0.5cpu, 8*2gb) = 6
Medium Composer environment:
worker_cpu = 2
worker_mem = 7.5
worker_concurrency = min(32, 12*2cpu, 8*7.5gb) = 24
Large Composer environment:
worker_cpu = 4
worker_mem = 15
worker_concurrency = min(32, 12*4cpu, 8*15gb) = 32
Autoscaling of workers
Two options are related to concurrency performance and the autoscaling capabilities of your environment:
The bare minimum of Airflow employees
The parameter [celery]worker_concurrency
In order to take up any waiting tasks, Google Cloud Composer keeps an eye on the task queue and creates more workers. When [celery]worker_concurrency is set to a high value, each worker can accept a large number of tasks; hence, in some cases, the queue may never fill and autoscaling may never occur.
Each worker would pick up 100 tasks, for instance, in a Google Cloud Composer setup with two Airflow workers, [celery]worker_concurrency set to 100, and 200 tasks in the queue. This doesn’t start autoscaling and leaves the queue empty. Results may be delayed if certain jobs take a long time to finish since other tasks may have to wait for available worker slots.
Taking a different approach, we can see that Composer’s scaling is based on adding up all of the Queued Tasks and Running Tasks, dividing that total by [celery]worker_concurrency, and then ceiling()ing the result. The target number of workers is ceiling((11+8)/6) = 4 if there are 11 tasks in the Running state and 8 tasks in the Queued state with [celery]worker_concurrency set to 6. The composer aims to reduce the workforce to four employees.
Airflow installation level settings
This is the Google Cloud Composer-managed Airflow installation. It consists of every Airflow component, including the workers, web server, scheduler, DAG processor, and metadata database. If they are not already configured, this level will inherit the Composer level configurations.
Worker concurrency ([celery]): For most use scenarios, Google Cloud Composer‘s default defaults are ideal, but you may want to make unique tweaks based on your environment.
core.parallelism: the most jobs that can be executed simultaneously within an Airflow installation. Infinite parallelism=0 is indicated.
Maximum number of active DAG runs per DAG is indicated by core.max_active_runs_per_dag.
Maximum number of active DAG tasks per DAG is indicated by core.max_active_tasks_per_dag.
Queues
It is possible to specify which Celery queues jobs are sent to when utilising the CeleryExecutor. Since queue is a BaseOperator attribute, any job can be assigned to any queue. The celery -> default_queue section of the airflow.cfg defines the environment’s default queue. This specifies which queue Airflow employees listen to when they start as well as the queue to which jobs are assigned in the absence of a specification.
Airflow Pools
It is possible to restrict the amount of simultaneous execution on any given collection of tasks by using airflow pools. Using the UI (Menu -> Admin -> Pools), you can manage the list of pools by giving each one a name and a number of worker slots. Additionally, you may choose there whether the pool’s computation of occupied slots should take postponed tasks into account.
Configuring the DAG level
The fundamental idea behind Airflow is a DAG, which groups tasks together and arranges them according to linkages and dependencies to specify how they should operate.
max_active_runs: the DAG’s maximum number of active runs. Once this limit is reached, the scheduler will stop creating new active DAG runs. backs to the core.If not configured, max_active_runs_per_dag
max_active_tasks: This is the maximum number of task instances that are permitted to run concurrently throughout all active DAG runs. The value of the environment-level option max_active_tasks_per_dag is assumed if this variable is not defined.
Configuring the task level
Concerning Airflow Tasks
A Task Instance may be in any of the following states:
none: Because its dependencies have not yet been satisfied, the task has not yet been queued for execution.
scheduled: The task should proceed because the scheduler has concluded that its dependencies are satisfied.
queued: An Executor has been given the task, and it is awaiting a worker.
running: A worker (or a local/synchronous executor) is performing the task.
success: There were no mistakes in the task’s completion.
restarting: While the job was operating, an external request was made for it to restart.
failed: A task-related fault prevented it from completing.
skipped: Branching, LatestOnly, or a similar reason led to the job being skipped.
upstream_failed: The Trigger Rule indicates that we needed it, but an upstream task failed.
up_for_retry: The job failed, but there are still retries available, and a new date will be set.
up_for_reschedule: A sensor that is in reschedule mode is the task.
deferred: A trigger has been assigned to complete the task.
removed: Since the run began, the task has disappeared from the DAG.
A task should ideally go from being unplanned to being scheduled, queued, running, and ultimately successful. Unless otherwise indicated, tasks will inherit concurrency configurations established at the DAG or Airflow level. Configurations particular to a task comprise:
Pool: the area where the task will be carried out. Pools can be used to restrict the amount of work that can be done in parallel.
The maximum number of concurrently executing task instances across dag_runs per task is controlled by max_active_tis_per_dag.
Deferrable Triggers and Operators
Even when they are idle, Standard Operators and Sensors occupy a full worker slot. For instance, if you have 100 worker slots available for Task execution and 100 DAGs are waiting on an idle but running Sensor, you will not be able to run any other tasks, even though your entire Airflow cluster is effectively idle.
Deferrable operators can help in this situation.
When an operator is constructed to be deferrable, it can recognise when it needs to wait, suspend itself, free up the worker, and assign the task of resuming to something known as a trigger. Because of this, it is not consuming a worker slot while it is suspended (delayed), which means that your cluster will use a lot fewer resources on inactive Operators and Sensors. It should be noted that delayed tasks do not automatically eat up pool slots; however, you can modify the pool in question to make them do so if desired.
Triggers are short, asynchronous Python code segments that are intended to execute concurrently within a single Python session; their asynchrony allows them to coexist effectively. Here’s a rundown of how this procedure operates:
When a task instance, also known as a running operator, reaches a waiting point, it defers itself using a trigger connected to the event that should resume it. This allows the employee to focus on other tasks.
A triggerer process detects and registers the new Trigger instance within Airflow.
The source task of the trigger gets rescheduled after it fires. The trigger is triggered.
The task is queued by the scheduler to be completed on a worker node.
Sensor Modes
Sensors can operate in two separate modes as they are mostly idle, which allows you to use them more effectively:
Poke (default): Throughout its whole duration, the Sensor occupies a worker slot.
Reschedule: The Sensor sleeps for a predetermined amount of time in between checks, only using a worker slot when it is checking. Part of problem is resolved when Sensors are run in rescheduled mode, which restricts their operation to predetermined intervals. However, this mode is rigid and only permits the use of time as justification for resuming operations.
As an alternative, some sensors let you specify deferrable=True, which transfers tasks to a different Triggerer component and enhances resource efficiency even more.
Distinction between deferrable=True and mode=’reschedule’ in sensors
Sensors in Airflow wait for certain requirements to be satisfied before moving on to downstream operations. When it comes to controlling idle times, sensors have two options: mode=’reschedule’ and deferrable=True. If the condition is not met, the sensor can reschedule itself thanks to the mode=’reschedule’ parameter specific to the BaseSensorOperator in Airflow. In contrast, deferrable=True is a convention used by some operators to indicate that the task can be retried (or deferred), but it is not a built-in parameter or mode in the Airflow. The individual operator implementation may cause variations in the behaviour of the task’s retry.
Read more on govindhtech.com
#GoogleCloud#ComposerAirflowDAG#TaskConcurrency#ApacheAirflow#GoogleCloudservices#CloudMonitoring#CPUcanmanage#processor#cpu#GoogleCloudComposer#technology#technews#news#govindhtech
0 notes
Link
Apache NiFi vs Airflow: Overview and Comparison Study
#ksolves#apachenifi#apacheairflow#apachenifivsairflow#apachenifidevelopmentcompany apachenififdevelopmentservices
0 notes
Link
0 notes