#Natural Language Generation (NLG)
Explore tagged Tumblr posts
Text
Explore Natural Language Generation (NLG) Benefits
In the age of digital transformation, the way we generate content is evolving faster than ever. One of the key driving forces behind this revolution is Natural Language Generation (NLG), a subfield of Artificial Intelligence (AI) that turns structured data into human-like language.
From automating financial reports to powering chatbots and personalizing marketing messages, NLG is no longer just a futuristic concept, it’s an operational reality for many businesses.
This article explores the top benefits of Natural Language Generation, how it's used in real-world scenarios, and why it’s becoming a must-have technology in modern data-driven organizations.
What is Natural Language Generation (NLG)?
NLG is a branch of AI that converts structured data into natural language. In simple terms, it’s the tech that enables machines to write or speak in a way that resembles human communication.
Think of NLG as the opposite of Natural Language Processing (NLP). While NLP helps machines understand human language, NLG helps machines produce it.
Some well-known tools powered by NLG include:
AI writing assistants like ChatGPT and Jasper
Automated weather or sports reports
Customer service chatbots
Business intelligence dashboards with narrative insights
Why NLG Matters: Benefits That Go Beyond Speed
Natural Language Generation isn't just about producing text fast—it’s about transforming how businesses communicate, scale, and make decisions. Below are the core benefits:
1. Efficiency and Scalability
NLG automates time-consuming writing tasks that previously required hours of human effort. Whether you're generating 10 or 10,000 reports, NLG can scale with zero drop in quality.
NOTE: Companies using NLG for report generation have seen content production time drop by up to 80% (Source: Gartner, 2023).
2. Data-to-Insight Transformation
NLG transforms complex data into understandable narratives. For example, a sales dashboard filled with numbers becomes a readable paragraph: "Revenue increased by 15% in Q1, mainly driven by strong performance in the US market."
This is especially useful in:
Finance (automated earnings reports)
Retail (sales summaries)
Healthcare (patient summaries)
3. Consistency in Communication
Unlike human writers, NLG tools never get tired or distracted. This means businesses get consistent tone, language, and structure—critical for brand messaging, compliance, and customer trust.
4. Multilingual Support
Need the same report in five different languages? NLG can generate content across multiple languages at scale, breaking down communication barriers and opening new global markets.
5. Hyper-Personalization
With the rise of personalization in marketing, NLG allows brands to tailor content at an individual level using user data. Think product descriptions that adjust based on browsing history or emails written uniquely for each recipient.
NOTE: Personalized content using NLG can increase conversion rates by 20-30% (Source: McKinsey Digital, 2022).
6. Reduced Operational Costs
By automating repetitive and high-volume tasks, companies reduce the need for manual labor while improving output. Writers can focus on strategy and creativity, while NLG handles the grunt work.
Table: Key Benefits of NLG Across Industries
Industry
NLG Use Case
Benefit
Finance
Automated investment summaries
Saves time, reduces analyst workload
Retail
Product descriptions and recommendations
Boosts conversions, supports SEO
Healthcare
Patient data summaries
Enhances accuracy, saves doctor time
Media
Automated sports/news reporting
Real-time reporting at large scale
Marketing
Personalized email and ad copy
Higher engagement and CTR
E-commerce
Review generation and FAQs
Enriches content, improves UX
Real-World Example: How NLG Helps Businesses
Financial Services
A global investment firm implemented NLG to automate weekly portfolio performance reports for over 3,000 clients. Previously, this required a team of 10 analysts. With NLG, reports are now generated in seconds, allowing analysts to focus on deeper insights and client strategy.
E-commerce
An online marketplace used NLG to write product descriptions for over 100,000 SKUs. Instead of manually writing each one, NLG generated SEO-friendly, accurate, and tailored descriptions in minutes—resulting in a 25% increase in organic search traffic.
NLG vs Traditional Writing: What’s the Difference?
Feature
Traditional Writing
NLG-Powered Writing
Speed
Hours or days
Seconds or minutes
Scalability
Limited by workforce
Infinite scale
Consistency
Varies by writer
Uniform tone and format
Personalization
Manual and limited
Automated, data-driven
Multilingual Support
Requires human translators
Built-in, real-time translation
Addressing Common Myths About NLG
Myth: “NLG will replace human writers.” Reality: NLG augments human writers by taking over repetitive tasks, freeing up time for strategic and creative work.
Myth: “NLG outputs are robotic and stiff.” Reality: Modern NLG models (especially those using deep learning) can produce content that is fluent, contextual, and even witty.
Myth: “Only big companies can afford NLG.” Reality: Thanks to cloud-based APIs and tools, even startups can use NLG affordably.
Future of NLG: Where It’s Headed
The future of NLG lies in real-time, contextual generation that blends seamlessly into user experiences. Expect more:
Voice-enabled NLG (for assistants like Siri, Alexa)
Emotional tone detection and variation
Domain-specific generators (e.g. legal, medical, scientific)
Better integrations with business tools (CRMs, ERPs)
As LLMs (Large Language Models) like GPT and Claude evolve, NLG will continue to become more intuitive, accurate, and human-like.
Final Thoughts
Natural Language Generation is not just about automation—it’s about amplifying human intelligence. It enables businesses to turn their data into dialogue, their dashboards into narratives, and their insights into impact.
Whether you're in marketing, finance, healthcare, or tech, now is the time to explore how NLG can drive growth, boost productivity, and transform communication.
NOTE: Start small—automate one task, measure the results, and scale from there.
0 notes
Text
#Natural Language Generation (NLG) Market size#Natural Language Generation (NLG) Market scope#Natural Language Generation (NLG) Market report
0 notes
Text
Everything You Need to Know About Natural Language Generation | USAII®
Natural Language Generation an essential component of Natural Language Processing has been transforming industries. Enhance your understanding of NLG here.
Read more: https://shorturl.at/mQ2Rf
Natural language generation (NLG), artificial intelligence models, AI models, NLG technology, NLP technology, NLG algorithm, NLP models, Machine learning Certifications, ML Engineer, Best AI ML Certifications

0 notes
Text
youtube
STOP Using Fake Human Faces in AI
#GenerativeAI#GANs (Generative Adversarial Networks)#VAEs (Variational Autoencoders)#Artificial Intelligence#Machine Learning#Deep Learning#Neural Networks#AI Applications#CreativeAI#Natural Language Generation (NLG)#Image Synthesis#Text Generation#Computer Vision#Deepfake Technology#AI Art#Generative Design#Autonomous Systems#ContentCreation#Transfer Learning#Reinforcement Learning#Creative Coding#AI Innovation#TDM#health#healthcare#bootcamp#llm#youtube#branding#animation
1 note
·
View note
Text
Getting the Best Out of ChatGPT
Getting the Best Out of ChatGPT
ChatGPT is a powerful natural language generation (NLG) tool that can help lawyers with various tasks, such as drafting documents, summarising cases, answering questions, and more. ChatGPT uses a deep neural network model that has been trained on a large corpus of text from various domains, including legal texts. ChatGPT can generate fluent and coherent texts based on a given input, such as a…
View On WordPress
0 notes
Text
GPTWhisper Review: How to Automate Your Digital Marketing with AI
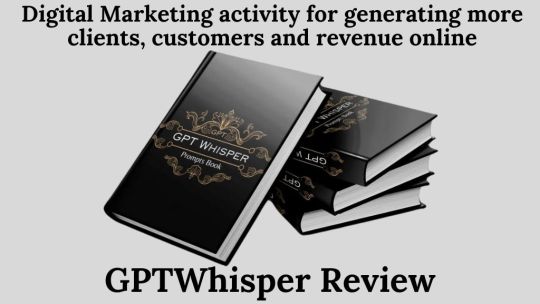
You can use GPTWhisper to save time, money and effort to grow your digital business, as it is the ultimate AI shortcut perfect for online marketers.
GPTWhisper is a web-based platform that harnesses the power of GPT-4, the most advanced Natural Language Generation (NLG) technology for your business, helping you generate high-quality content, copy, headlines, slogans, emails, ads and more.
GPTWhisper works by taking your input, such as a keyword, a topic, a product name, or a brief description, and generating relevant and engaging text for you in seconds. You can also choose from different tones, styles, and formats to suit your needs and preferences.
It isn't just a substance generator yet additionally a substance enhancer. It can assist you with working on your current substance by revising, summing up, rewording, or adding watchwords, invitations to take action, or snares. It can assist you with examining your substance for coherence, opinion, Search engine optimization and literary theft.
It is intended to be not difficult to utilize, instinctive and adaptable. You can get to it from any gadget, program or application and incorporate it with your #1 instruments, like WordPress, Shopify, Mailchimp, Facebook, Google Promotions and that's only the tip of the iceberg. You can likewise trade your substance in various arrangements like PDF, DOCX, HTML or TXT
GPTWhisper isn't just a device, yet additionally a local area. You can join the GPTWhisper Club, where you can interface with different clients, share your input, demand new highlights, get tips and deceives, and access select assets and rewards.
GPTWhisper is a unique advantage for computerized advertisers who need to use the force of man-made intelligence to robotize and scale their web based showcasing endeavors. Whether you are a fledgling or a specialist, a consultant or an office, a blogger or an internet business proprietor, GPTWhisper can help you make and enhance content that draws in, connects with, and changes over your crowd.
To attempt GPTWhisper for yourself, you can pursue a free preliminary and get 10 credits to use on any of the elements. You can likewise move up to a superior arrangement and get limitless admittance to every one of the highlights, in addition to additional credits, backing, and advantages.
GPTWhisper is a definitive simulated intelligence easy route for computerized showcasing. Try not to botch this chance to take your internet based business to a higher level with GPTWhisper. Join today and see the distinction for yourself!
GPTWhisper Review: Overview
Product: GPTWhisper
Launch Date: 2024-Jan-31
Launch Time: 10:00 EST
Front-End Price: 17
Niche: Software
Get More Info
#seo and traffic#GPTWhisper Review#GPTWhisper#GPTWhisper Software#Digital Marketing#Affiliate Marketing
2 notes
·
View notes
Text
Quiz Time with Digital Brolly! Are you ready to flex your digital marketing brain? 👉 What does Natural Language Generation (NLG) actually do in AI marketing tools?
💬 Got the answer? Drop it in the replies and tag a fellow AI geek! Let’s see who’s really keeping up with the future of marketing.
🎯 Master AI-Powered Digital Marketing at Digital Brolly!
Join Hyderabad’s most advanced AI-based Digital Marketing Training in Telugu & English – perfect for job seekers, professionals, students, and business owners.
💡 Why Choose Us? ✅ First-ever AI Marketing Course in Telugu ✅ 35+ Modules | 600+ Topics | 3 Trainers | 7 Mentors ✅ Real-time Projects & Live Paid Campaigns using AI ✅ 100% Placement Support + Internship Until You’re Placed ✅ Soft Skills + Resume Building + Profile Marketing ✅ Daily Lab Practice & One-on-One Mentoring
🎓 Get real work experience in just 3–4 months with full offline support + video access!
✨ Digital Marketing Antey Digital Brolly ✨
📞 Call: 81869 44555 📞 Call: 96 96 96 3446
🌐 Visit: digitalbrolly.com 📧 Email: [email protected]
📍 Institute Address:
3rd Floor, Dr Atmaram Estates, beside Sri Bhramaramba Theatre, Metro Pillar No: A689, Near JNTU Metro Station, Nizampet X Roads, Hyderabad, Telangana 500072
#digitalbrolly#quiztime#operationsindoor#operationsindoorin2025#aiinmarketing#naturallanguagegeneration#aipoweredtools#digitalmarketingquiz#learnwithbrolly#marketingwithai#contentcreation#trendingnow#instaquiz#facebookquiz#edutainment#aicontentcreation#socialmediachallenge#digitallearning#reeltrend#aitools2025#humanlikecontent
0 notes
Text
Storytelling with Data in 2025: Emerging Trends Every Analyst Should Know
In an age where data is the new oil, storytelling with data has never been more critical. The year 2025 marks a tipping point for how businesses, governments, and individuals harness insights through visual narratives. As data becomes increasingly complex, the ability to distill meaningful stories from vast datasets is a crucial skill. Whether you are a seasoned data visualization specialist, an aspiring analyst, or a business executive seeking clearer insights, the trends shaping storytelling with data in 2025 are essential to grasp.

1. From Dashboards to Dynamic Narratives
Traditional dashboards are transforming. No longer are they static displays of KPIs. In 2025, they have evolved into dynamic storytelling platforms. Today’s tools leverage natural language generation (NLG), AI-driven annotations, and automated pattern detection to craft contextual narratives around data.
Modern data visualization tools integrate real-time feeds, predictive analytics, and smart visual cues that change based on the viewer's role or inquiry. For example, a marketing executive and a financial analyst accessing the same dashboard will receive different visual stories tailored to their needs.
This shift reflects a broader cultural change: people crave context, not just numbers. Dynamic dashboards serve as interactive stories, guiding users through cause-effect relationships and strategic decision points.
2. Rise of Augmented Analytics
One of the defining trends of 2025 is the convergence of AI and human cognition in analytics. Augmented analytics use machine learning algorithms to surface the most relevant insights automatically.
A data visualization specialist today isn't just a chart designer; they are a curator of augmented stories. These professionals now work closely with AI to guide data exploration, suggest optimal visual formats, and highlight anomalies.
The impact on data visualization applications is profound. Platforms now include smart recommendations, automated anomaly detection, and even sentiment analysis integration. This helps analysts quickly identify and communicate the story within the data without manual sifting.
3. Multimodal Storytelling
Data stories in 2025 are no longer confined to graphs and charts. With the rise of multimodal interfaces, data can now be presented through voice, AR/VR, and immersive experiences.
Picture a logistics manager walking through a warehouse using AR glasses that overlay real-time data about inventory, delivery schedules, and efficiency metrics. These are not science fiction but actual data visualization applications being piloted across industries.
Voice interfaces allow analysts to query data verbally: "Show me last quarter's sales dip in the Midwest region," followed by a narrated explanation with accompanying visuals. These multimodal experiences increase accessibility and engagement across diverse audiences.
4. Democratization of Data Storytelling
2025 heralds a new era of self-service analytics. With the proliferation of no-code and low-code data visualization tools, even non-technical users can now create compelling data stories.
The role of a data visualization specialist is shifting from creator to enabler. Their new mission: empower others to tell their own stories. This involves developing templates, training materials, and governance structures to ensure data consistency and clarity.
Education platforms are now incorporating data storytelling as a core competency. Expect MBA graduates, public policy students, and healthcare professionals to possess a baseline proficiency in data visualization applications.
5. Ethical and Inclusive Visualization
As data-driven decisions grow in scale and impact, ethical storytelling becomes essential. Analysts must now consider the societal implications of their visualizations.
In 2025, leading data visualization tools include bias detection algorithms, color-blind friendly palettes, and accessibility features like screen reader compatibility. These tools guide analysts in making visuals that are not only effective but also inclusive.
A responsible data visualization specialist asks: Who might misinterpret this graphic? Who is excluded from understanding this data? Inclusive visualization requires empathy, cultural awareness, and a commitment to equity.
6. Hyper-Personalization
Every user views the world through a unique lens. In response, data stories are becoming hyper-personalized. AI-driven personalization engines tailor dashboards and visuals based on user behavior, preferences, and past decisions.
Imagine a data visualization application that learns an executive's typical filters and prioritizes similar metrics in future reports. Or a tool that adapts its visualizations for different cognitive styles—presenting summaries visually for some, and text-heavy narratives for others.
Hyper-personalization doesn't mean pandering—it means relevance. By aligning data storytelling with individual needs, organizations enhance comprehension and decision-making.
7. Real-Time, Action-Oriented Insights
Gone are the days of weekly reports. In 2025, organizations demand real-time, action-oriented insights that can drive instant decisions.
Modern data visualization tools are deeply integrated with live data sources, predictive engines, and alert systems. When a key metric deviates unexpectedly, the system not only alerts the user but provides a story: why it happened, what it affects, and what action to take.
A data visualization specialist must now think like a UX designer—optimizing the experience for rapid comprehension and response. This involves focusing on clarity, hierarchy, and visual cues that direct attention to the most critical elements.
8. Emotional Storytelling through Data
One of the most profound shifts is the rise of emotional resonance in data storytelling. Human decisions are often driven by emotion, not logic. Storytellers who blend data with human narratives evoke stronger engagement and understanding.
For example, a nonprofit using a data visualization application to show refugee displacement trends can pair it with firsthand stories, imagery, and dynamic maps. These experiences generate empathy and drive action.
The future belongs to data visualization specialists who can blend the rational with the emotional—crafting stories that move people, not just inform them.
9. Cross-Functional Collaboration
Storytelling with data is no longer a siloed activity. In 2025, it is a cross-functional process involving data scientists, designers, domain experts, and communicators.
Data visualization tools now offer collaborative features: shared dashboards, comment threads, version control, and real-time co-editing. These features mirror tools like Google Docs or Figma but are tailored for data work.
A modern data visualization specialist acts as a bridge—facilitating communication between technical and non-technical stakeholders. They help teams co-create narratives that drive aligned decisions.
10. Future-Proofing Your Skillset
To remain relevant in 2025 and beyond, analysts must continuously evolve. This means:
Mastering advanced data visualization tools like Tableau, Power BI, and emerging platforms with AI integration.
Learning scripting languages (e.g., Python, R) to augment visual storytelling with automated workflows.
Understanding behavioral psychology to craft persuasive, human-centered visuals.
Developing ethical literacy around data privacy, bias, and inclusion.
Whether you are a novice or a senior data visualization specialist, your journey must include both technical and narrative growth. The most successful professionals are those who combine sharp analytical thinking with compelling storytelling prowess.
Conclusion
In 2025, storytelling with data is no longer a niche skill—it is the currency of decision-making. The convergence of AI, interactivity, inclusivity, and emotional intelligence has redefined what it means to be a data visualization specialist. With the right data visualization tools and thoughtful data visualization applications, anyone can transform raw information into actionable wisdom.
As we move further into the decade, one thing is certain: the future belongs to those who can tell stories that people understand, remember, and act upon.
#data migration services#data visualization specialist#data visualization consultant#data visualization in finance
0 notes
Text
How AI Is Revolutionizing Contact Centers in 2025
As contact centers evolve from reactive customer service hubs to proactive experience engines, artificial intelligence (AI) has emerged as the cornerstone of this transformation. In 2025, modern contact center architectures are being redefined through AI-based technologies that streamline operations, enhance customer satisfaction, and drive measurable business outcomes.
This article takes a technical deep dive into the AI-powered components transforming contact centers—from natural language models and intelligent routing to real-time analytics and automation frameworks.
1. AI Architecture in Modern Contact Centers
At the core of today’s AI-based contact centers is a modular, cloud-native architecture. This typically consists of:
NLP and ASR engines (e.g., Google Dialogflow, AWS Lex, OpenAI Whisper)
Real-time data pipelines for event streaming (e.g., Apache Kafka, Amazon Kinesis)
Machine Learning Models for intent classification, sentiment analysis, and next-best-action
RPA (Robotic Process Automation) for back-office task automation
CDP/CRM Integration to access customer profiles and journey data
Omnichannel orchestration layer that ensures consistent CX across chat, voice, email, and social
These components are containerized (via Kubernetes) and deployed via CI/CD pipelines, enabling rapid iteration and scalability.
2. Conversational AI and Natural Language Understanding
The most visible face of AI in contact centers is the conversational interface—delivered via AI-powered voice bots and chatbots.
Key Technologies:
Automatic Speech Recognition (ASR): Converts spoken input to text in real time. Example: OpenAI Whisper, Deepgram, Google Cloud Speech-to-Text.
Natural Language Understanding (NLU): Determines intent and entities from user input. Typically fine-tuned BERT or LLaMA models power these layers.
Dialog Management: Manages context-aware conversations using finite state machines or transformer-based dialog engines.
Natural Language Generation (NLG): Generates dynamic responses based on context. GPT-based models (e.g., GPT-4) are increasingly embedded for open-ended interactions.
Architecture Snapshot:
plaintext
CopyEdit
Customer Input (Voice/Text)
↓
ASR Engine (if voice)
↓
NLU Engine → Intent Classification + Entity Recognition
↓
Dialog Manager → Context State
↓
NLG Engine → Response Generation
↓
Omnichannel Delivery Layer
These AI systems are often deployed on low-latency, edge-compute infrastructure to minimize delay and improve UX.
3. AI-Augmented Agent Assist
AI doesn’t only serve customers—it empowers human agents as well.
Features:
Real-Time Transcription: Streaming STT pipelines provide transcripts as the customer speaks.
Sentiment Analysis: Transformers and CNNs trained on customer service data flag negative sentiment or stress cues.
Contextual Suggestions: Based on historical data, ML models suggest actions or FAQ snippets.
Auto-Summarization: Post-call summaries are generated using abstractive summarization models (e.g., PEGASUS, BART).
Technical Workflow:
Voice input transcribed → parsed by NLP engine
Real-time context is compared with knowledge base (vector similarity via FAISS or Pinecone)
Agent UI receives predictive suggestions via API push
4. Intelligent Call Routing and Queuing
AI-based routing uses predictive analytics and reinforcement learning (RL) to dynamically assign incoming interactions.
Routing Criteria:
Customer intent + sentiment
Agent skill level and availability
Predicted handle time (via regression models)
Customer lifetime value (CLV)
Model Stack:
Intent Detection: Multi-label classifiers (e.g., fine-tuned RoBERTa)
Queue Prediction: Time-series forecasting (e.g., Prophet, LSTM)
RL-based Routing: Models trained via Q-learning or Proximal Policy Optimization (PPO) to optimize wait time vs. resolution rate
5. Knowledge Mining and Retrieval-Augmented Generation (RAG)
Large contact centers manage thousands of documents, SOPs, and product manuals. AI facilitates rapid knowledge access through:
Vector Embedding of documents (e.g., using OpenAI, Cohere, or Hugging Face models)
Retrieval-Augmented Generation (RAG): Combines dense retrieval with LLMs for grounded responses
Semantic Search: Replaces keyword-based search with intent-aware queries
This enables agents and bots to answer complex questions with dynamic, accurate information.
6. Customer Journey Analytics and Predictive Modeling
AI enables real-time customer journey mapping and predictive support.
Key ML Models:
Churn Prediction: Gradient Boosted Trees (XGBoost, LightGBM)
Propensity Modeling: Logistic regression and deep neural networks to predict upsell potential
Anomaly Detection: Autoencoders flag unusual user behavior or possible fraud
Streaming Frameworks:
Apache Kafka / Flink / Spark Streaming for ingesting and processing customer signals (page views, clicks, call events) in real time
These insights are visualized through BI dashboards or fed back into orchestration engines to trigger proactive interventions.
7. Automation & RPA Integration
Routine post-call processes like updating CRMs, issuing refunds, or sending emails are handled via AI + RPA integration.
Tools:
UiPath, Automation Anywhere, Microsoft Power Automate
Workflows triggered via APIs or event listeners (e.g., on call disposition)
AI models can determine intent, then trigger the appropriate bot to complete the action in backend systems (ERP, CRM, databases)
8. Security, Compliance, and Ethical AI
As AI handles more sensitive data, contact centers embed security at multiple levels:
Voice biometrics for authentication (e.g., Nuance, Pindrop)
PII Redaction via entity recognition models
Audit Trails of AI decisions for compliance (especially in finance/healthcare)
Bias Monitoring Pipelines to detect model drift or demographic skew
Data governance frameworks like ISO 27001, GDPR, and SOC 2 compliance are standard in enterprise AI deployments.
Final Thoughts
AI in 2025 has moved far beyond simple automation. It now orchestrates entire contact center ecosystems—powering conversational agents, augmenting human reps, automating back-office workflows, and delivering predictive intelligence in real time.
The technical stack is increasingly cloud-native, model-driven, and infused with real-time analytics. For engineering teams, the focus is now on building scalable, secure, and ethical AI infrastructures that deliver measurable impact across customer satisfaction, cost savings, and employee productivity.
As AI models continue to advance, contact centers will evolve into fully adaptive systems, capable of learning, optimizing, and personalizing in real time. The revolution is already here—and it's deeply technical.
#AI-based contact center#conversational AI in contact centers#natural language processing (NLP)#virtual agents for customer service#real-time sentiment analysis#AI agent assist tools#speech-to-text AI#AI-powered chatbots#contact center automation#AI in customer support#omnichannel AI solutions#AI for customer experience#predictive analytics contact center#retrieval-augmented generation (RAG)#voice biometrics security#AI-powered knowledge base#machine learning contact center#robotic process automation (RPA)#AI customer journey analytics
0 notes
Text
AI-Powered Cyber Attacks: How Hackers Are Using Generative AI
Introduction
Artificial Intelligence (AI) has revolutionized industries, from healthcare to finance, but it has also opened new doors for cybercriminals. With the rise of generative AI tools like ChatGPT, Deepfake generators, and AI-driven malware, hackers are finding sophisticated ways to automate and enhance cyber attacks. This article explores how cybercriminals are leveraging AI to conduct more effective and evasive attacks—and what organizations can do to defend against them.

How Hackers Are Using Generative AI
1. AI-Generated Phishing & Social Engineering Attacks
Phishing attacks have become far more convincing with generative AI. Attackers can now:
Craft highly personalized phishing emails using AI to mimic writing styles of colleagues or executives (CEO fraud).
Automate large-scale spear-phishing campaigns by scraping social media profiles to generate believable messages.
Bypass traditional spam filters by using AI to refine language and avoid detection.
Example: An AI-powered phishing email might impersonate a company’s IT department, using natural language generation (NLG) to sound authentic and urgent.
2. Deepfake Audio & Video for Fraud
Generative AI can create deepfake voice clones and videos to deceive victims. Cybercriminals use this for:
CEO fraud: Fake audio calls instructing employees to transfer funds.
Disinformation campaigns: Fabricated videos of public figures spreading false information.
Identity theft: Mimicking voices to bypass voice authentication systems.
Example: In 2023, a Hong Kong finance worker was tricked into transferring $25 million after a deepfake video call with a "colleague."
3. AI-Powered Malware & Evasion Techniques
Hackers are using AI to develop polymorphic malware that constantly changes its code to evade detection. AI helps:
Automate vulnerability scanning to find weaknesses in networks faster.
Adapt malware behavior based on the target’s defenses.
Generate zero-day exploits by analyzing code for undiscovered flaws.
Example: AI-driven ransomware can now decide which files to encrypt based on perceived value, maximizing extortion payouts.
4. Automated Password Cracking & Credential Stuffing
AI accelerates brute-force attacks by:
Predicting password patterns based on leaked databases.
Generating likely password combinations using machine learning.
Bypassing CAPTCHAs with AI-powered solving tools.
Example: Tools like PassGAN use generative adversarial networks (GANs) to guess passwords more efficiently than traditional methods.
5. AI-Assisted Social Media Manipulation
Cybercriminals use AI bots to:
Spread disinformation at scale by generating fake posts and comments.
Impersonate real users to conduct scams or influence public opinion.
Automate fake customer support accounts to steal credentials.
Example:AI-generated Twitter (X) bots have been used to spread cryptocurrency scams, impersonating Elon Musk and other influencers.
How to Defend Against AI-Powered Cyber Attacks
As AI threats evolve, organizations must adopt AI-driven cybersecurity to fight back. Key strategies include:
AI-Powered Threat Detection – Use machine learning to detect anomalies in network behavior.
Multi-Factor Authentication (MFA) – Prevent AI-assisted credential stuffing with biometrics or hardware keys.
Employee Training – Teach staff to recognize AI-generated phishing and deepfakes.
Zero Trust Security Model – Verify every access request, even from "trusted" sources.
Deepfake Detection Tools – Deploy AI-based solutions to spot manipulated media.
Conclusion Generative AI is a double-edged sword—while it brings innovation, it also empowers cybercriminals with unprecedented attack capabilities. Organizations must stay ahead by integrating AI-driven defenses, improving employee awareness, and adopting advanced authentication methods. The future of cybersecurity will be a constant AI vs. AI battle, where only the most adaptive defenses will prevail.
Source Link:https://medium.com/@wafinews/title-ai-powered-cyber-attacks-how-hackers-are-using-generative-ai-516d97d4455e
0 notes
Text
Revolutionizing SEO with Advanced GEO Techniques by ThatWare LLP
In the evolving landscape of digital marketing, traditional SEO is giving way to smarter, AI-powered methods — and GEO techniques are leading this shift. Designed to work in harmony with modern search engine algorithms, GEO techniques combine the power of machine learning, natural language generation (NLG), and semantic understanding to create content that ranks better, engages deeper, and adapts faster. ThatWare LLP implementation of GEO techniques enables businesses to generate contextually rich, intent-driven content that aligns perfectly with user search patterns. Whether it's dynamic keyword clustering, automated meta tag creation, or predictive content modeling, every part of the strategy is powered by cutting-edge AI.
0 notes
Text
AI Agent Development: A Comprehensive Guide for Building Intelligent Virtual Assistants
In an era where automation and personalization are at the heart of business innovation, AI agents—also known as intelligent virtual assistants (IVAs)—are transforming how we work, communicate, and engage with customers. Whether it’s answering support queries, scheduling meetings, or helping users shop online, these AI-powered assistants are becoming indispensable.
If you're considering building an AI agent for your business or app, this comprehensive guide will walk you through the what, why, and how of AI agent development.

What Is an AI Agent?
An AI agent is a software program that can autonomously perform tasks on behalf of a user or system. These agents use technologies like natural language processing (NLP), machine learning (ML), and contextual understanding to carry out actions based on user inputs or environmental triggers.
They can:
Understand and respond to text or voice inputs.
Learn user preferences over time.
Integrate with external systems like CRMs, calendars, and databases.
Automate repetitive or complex workflows.
Common examples include Siri, Alexa, Google Assistant, ChatGPT, and enterprise-level AI assistants used in banking, healthcare, and IT support.
Why Build an Intelligent Virtual Assistant?
1. 24/7 Availability
AI agents never sleep. They can offer continuous service, enhancing customer satisfaction.
2. Scalability
Unlike human teams, AI agents can handle hundreds or thousands of conversations simultaneously.
3. Cost-Effectiveness
Once deployed, AI assistants reduce labor costs and improve operational efficiency.
4. Personalization
They learn from interactions to tailor responses and recommendations based on user behavior.
5. Process Automation
They can take over repetitive, rule-based tasks like answering FAQs, booking appointments, or generating reports.
Key Components of an AI Agent
To build a high-performing AI agent, several components must come together seamlessly:
🔹 Natural Language Understanding (NLU)
This enables the assistant to understand user intent and extract relevant entities from a query.
🔹 Dialogue Management
The brain of the assistant. It controls conversation flow, keeps context, and decides how to respond.
🔹 Natural Language Generation (NLG)
It converts machine-level data into human-like responses.
🔹 Machine Learning Models
They help the AI agent improve over time through supervised or reinforcement learning.
🔹 Integrations
APIs and back-end connections that allow the assistant to perform tasks like sending emails, checking calendars, or querying databases.
Step-by-Step Guide to AI Agent Development
Here’s a breakdown of the typical development process:
1. Define the Use Case
Start with a clear objective: Do you want a customer service bot? A virtual shopping assistant? Or a healthcare guide? Define the user journey and scope.
2. Choose a Development Framework
Some popular platforms include:
Rasa – Open-source and customizable
Dialogflow (by Google) – Cloud-based with ML support
Microsoft Bot Framework – Integrates with Azure
IBM Watson Assistant
OpenAI API – For GPT-based assistants
3. Design Conversation Flows
Create dialogue maps for various intents. Include fallback responses, error handling, and multi-turn conversation capabilities.
4. Train the NLU Model
Feed the model with training data:
Intents (e.g., “check order status”)
Entities (e.g., order number, date)
Sample utterances for each intent
5. Integrate with External Services
Link the AI assistant to required data sources like CRMs, ticketing systems, payment gateways, or scheduling tools.
6. Test and Iterate
Use beta testing with real users to refine language models, conversation flows, and performance.
7. Deploy and Monitor
Launch the assistant on your desired platform (web, mobile app, WhatsApp, Slack, etc.). Continuously monitor user interactions and improve the agent’s capabilities based on feedback.
Challenges in AI Agent Development
Despite the potential, some challenges need to be tackled:
Data Privacy: Sensitive user information must be securely stored and processed.
Context Retention: Managing long, multi-turn conversations can be complex.
Bias & Fairness: Training data must be representative to avoid bias.
User Trust: Responses should be transparent, especially in critical industries like healthcare or finance.
The Future of AI Agents
As multi-agent systems, emotion recognition, and generative AI become more mainstream, the next generation of virtual assistants will be:
Proactive instead of reactive
Emotionally intelligent
Autonomous in making business decisions
Capable of deep personal and contextual understanding
Enterprises are already integrating AI agents with LLMs (Large Language Models) and enterprise search systems to act as personalized copilots across tools like Microsoft Teams, Slack, and CRMs.
Final Thoughts
Developing a capable AI agent goes beyond chatbot basics. It's about creating a responsive, intelligent, and adaptive assistant that fits naturally into your users' digital experiences. Whether you’re a startup or an enterprise, investing in AI agent development today can give you a serious edge in automation, personalization, and user satisfaction.
1 note
·
View note
Text
#Natural Language Generation (NLG) Market share#Natural Language Generation (NLG) Market trends#Natural Language Generation (NLG) Market growth
0 notes
Text
key elements of conversational AI
Conversational AI hinges on several critical elements to simulate natural human interaction. At its core lies Natural Language Understanding (NLU), enabling the AI to interpret user intent and extract relevant entities. Natural Language Generation (NLG) crafts human-like responses, while dialogue management orchestrates the conversation flow. Contextual awareness ensures the AI remembers past interactions, and sentiment analysis gauges user emotions. Machine learning algorithms refine the AI's performance, allowing for personalized and adaptive conversations. Voice recognition and text-to-speech capabilities facilitate multimodal interactions. Ethical considerations and robust error handling are essential for responsible and effective deployment. These elements collectively empower conversational AI to engage users in meaningful and intuitive ways. To Know more: https://gyatagpt.ai/
0 notes
Text
youtube
MIND-BLOWING Semantic Data Secrets Revealed in AI and Machine Learning
#GenerativeAI#GANs (Generative Adversarial Networks)#VAEs (Variational Autoencoders)#Artificial Intelligence#Machine Learning#Deep Learning#Neural Networks#AI Applications#CreativeAI#Natural Language Generation (NLG)#Image Synthesis#Text Generation#Computer Vision#Deepfake Technology#AI Art#Generative Design#Autonomous Systems#ContentCreation#Transfer Learning#Reinforcement Learning#Creative Coding#AI Innovation#TDM#health#healthcare#bootcamp#llm#branding#youtube#animation
1 note
·
View note
Text
0 notes