#NaturalLanguageProcessing(NLP)
Explore tagged Tumblr posts
Text
Learn how Qwen2.5, a large language model developed by Alibaba Cloud, revolutionizes AI with its ability to process long contexts up to 128K tokens and support over 29 languages. Pretrained on a large-scale dataset of 18 trillion tokens, it enhances high-quality code, mathematics, and multilingual data. Discover how it matches Llama-3-405B’s accuracy with only one-fifth of the parameters.
#Qwen2.5#AI#AlibabaCloud#LargeLanguageModels#MachineLearning#ArtificialIntelligence#AIModel#DataScience#NLP#NaturalLanguageProcessing#artificial intelligence#open source#machine learning#opensource#software engineering#programming#ai technology#technology#ai tech
2 notes
·
View notes
Text
#DataScience#DataScientist#StatisticalAnalysis#MachineLearning#DataVisualization#LargeDatasets#Insights#Predictions#ProblemSolving#BusinessDecisions#ToolsandTechnologies#NaturalLanguageProcessing(NLP)#TextData#LanguageTranslation#SentimentAnalysis#Chatbots#DataAnalysis#InformationExtraction#UnstructuredText#ValuableInsights
2 notes
·
View notes
Text
Demystifying NLP: The Bridge Between Human Language and Artificial Intelligence
As AI continues to evolve, it’s becoming more human — not in form, but in understanding. One of the most fascinating fields behind this transformation is Natural Language Processing (NLP), a powerful branch of AI that enables machines to understand, interpret, and even generate human language.
Recently, Intellitron Genesis published a comprehensive guide to NLP in AI, detailing how businesses can harness this technology across web development, mobile applications, e-commerce, and more. It’s a must-read for tech leaders, digital marketers, and entrepreneurs.
We also followed up with a Blogger post that expands on how NLP is not just a tech trend but a digital necessity for modern brands.
💡 Why NLP Matters
Imagine your website automatically understanding your customer’s intent — whether they type, speak, or search. That’s NLP at work. It powers:
Chatbots that speak your language
Product search filters that think like shoppers
Email responses that sound natural
Sentiment analysis that adapts your marketing in real time
At Intellitron Genesis, NLP is more than theory — it’s built into the very fabric of their development services. Whether you're launching an AI-driven app, optimizing an e-commerce platform, or building a smart website, they integrate NLP to make every interaction feel intuitive and human.
🚀 Digital Solutions Reinvented with NLP
Here’s how Intellitron Genesis combines NLP with smart digital services:
🌍 Website Development: Smart search, multilingual content optimization, voice-friendly navigation
📱 Mobile Application Development: Voice assistants, speech-to-text features, chatbot integrations
🛒 E-commerce Development: NLP for personalized shopping, review analysis, customer engagement
📈 Digital Marketing: Better keyword research, content analysis, audience sentiment tracking
🎨 Graphic & 3D Product Design: Scripted design flows, AI content generation for creatives
🎞️ Video Editing: NLP-driven storyboard creation, smart subtitles, automated editing prompts
🔗 Read, Share, Build
Want to dive deeper into how NLP can give your brand a digital edge?
📖 Read the full blog on Intellitron Genesis's official website 📰 Check out our supporting post on Blogger
And don’t forget to follow Intellitron Genesis for future-ready updates in AI, web development, app innovation, marketing, and more!
💬 Let’s Talk
What NLP-powered tools have you seen (or used) recently? Drop a comment below or message us — we're always curious about the intersection of tech and language.
#NLP#ArtificialIntelligence#WebDevelopment#MobileApps#EcommerceSolutions#DigitalMarketing#AIinDesign#VideoEditingAI#IntellitronGenesis#FutureTech#BusinessInnovation#NaturalLanguageProcessing
0 notes
Text
The Rise of Small Language Models: Are They the Future of NLP?
In recent years, large language models like GPT-4 and PaLM have dominated the field of NLP (Natural Language Processing). However, in 2025, we are witnessing a major shift: the rise of small language models (SLMs). Models like LLaMA 3, Mistral, and Gemma are proving that bigger isn't always better for NLP tasks.
Unlike their massive counterparts, small models are designed to be lightweight, faster, and cost-effective, making them ideal for a variety of NLP applications such as real-time translation, chatbots, and voice assistants. They require significantly less computing power, making them perfect for edge computing, mobile devices, and private deployments where traditional NLP systems were too heavy to operate.
Moreover, small language models offer better customization, privacy, and control over NLP systems, allowing businesses to fine-tune models for specific needs without relying on external cloud services.
While large models still dominate in highly complex tasks, small language models are shaping the future of NLP — bringing powerful language capabilities to every device and business, big or small.
#NLP#SmallLanguageModels#AI#MachineLearning#EdgeComputing#NaturalLanguageProcessing#AIFuture#TechInnovation#AIEfficiency#NLPTrends#FutureOfAI#ArtificialIntelligence#LanguageModels#AIin2025#TechForBusiness#AIandPrivacy#SustainableAI#ModelOptimization
0 notes
Text

"NLP Techniques: The Building Blocks of Conversational AI"
Natural Language Processing (NLP) is a branch of AI that enables machines to understand, interpret, and generate human language. Key NLP techniques include:1. Text Processing & Cleaning2. Text Representation3. Sentiment Analysis4. Named Entity Recognition (NER)5. Part-of-Speech (POS) Tagging6. Machine Translation7. Speech Recognition8. Text Summarization9. Chatbots & Conversational AI
1 note
·
View note
Text
NLP in Business: Techniques and Applications You Need to Know
Natural Language Processing (NLP) is revolutionizing businesses by enabling machines to understand and process human language. It powers chatbots, sentiment analysis, and automated support, enhancing efficiency and user experience. NLP also helps extract insights from unstructured data, aiding in smarter decision-making. Additionally, tools like speech recognition and language translation break communication barriers, driving global business growth Read More..

0 notes
Text

NOSTALGIA UK
Dr. Ahmad and 6 words are too many (1994)
Dr. Ahmad was another lecturer I have never forgotten—the person who first introduced me to the world of Artificial Intelligence (AI), particularly natural language processing (NLP). At the time, he was a well-regarded researcher in the field, with numerous PhD students under his guidance, all deeply immersed in the challenge of trying to make machines understand, interpret, and generate human language. This was about four years before the birth of Google, and ELIZA was still widely recognised in AI (for those unfamiliar with "her," feel free to Google it now!).
Dr. Ahmad was Pakistani, and from his accent, it was clear he had come to the UK later in life. I assumed he had come to the UK for his studies before eventually becoming a lecturer at the university. He always seemed incredibly busy—never walking at a leisurely pace, always rushing from one room to the next. It was difficult to catch even five minutes of conversation with him. Whenever he stepped into the classroom, he carried an air of urgency, as if eager to get through the lecture and move on to his next responsibility.
Yet, there was no doubt about his passion for AI, especially natural language processing. One thing from him has stuck with me over the years—his constant reminder that a well-constructed sentence should contain no more than six words (or ... maybe eight). Any longer, he warned, ambiguity would start creeping in. At the time, striking a balance between clarity and naturalness in language was a critical challenge in NLP—after all, this was still the early days of the field.
For my final-year project, upon returning from my industrial placement, I chose Dr. Ahmad as my supervisor—a sketch and story that will come later.
I learned so much from him about AI, and the guidance he provided during that final year was invaluable. I also fondly remember the wonderful dinner that Faeez, Leni, and I shared with him, his wife, and his son Waqas at their home near campus. That invitation meant a lot—thank you again for the invitation.
#guildford#drahmed#artificialintelligence#AI#universityofsurrey#UniS#petronasscholar#nostalgiauk#naturallanguageprocessing#NLP
0 notes
Text





#TechKnowledge Ever wondered how machines understand human language?
Let’s explore Natural Language Processing
👉 Stay tuned for more simple and insightful tech tips by following us.
💻 Explore the latest in #technology on our Blog Page: https://simplelogic-it.com/blogs/
✨ Looking for your next career opportunity? Check out our #Careers page for exciting roles: https://simplelogic-it.com/careers/
#techterms#technologyterms#techcommunity#simplelogicit#makingitsimple#techinsight#techtalk#naturallanguageprocessing#nlp#ai#humanlanguage#chatgpt#alexa#siri#communication#technology#knowledgeIispower#makeitsimple#simplelogic#didyouknow
0 notes
Text
NLP Tools for Text Analysis
NLP tools automate the analysis of text for patterns and sentiments.
They include features for text mining, sentiment analysis, and language interpretation.
Key tools offer scalability, accuracy, and integration with various platforms.
Used widely in marketing, finance, healthcare, and research. read more
#NLP#TextAnalysis#NaturalLanguageProcessing#MachineLearning#AI#DataScience#TextMining#AItools#DeepLearning#SentimentAnalysis#NLPTools#LanguageProcessing#DataAnalysis
0 notes
Text
Revolutionizing SEO with NLP and Hyper Intelligence

In today’s fast-paced digital landscape, traditional SEO strategies need to evolve to keep up with the complexities of user intent and search algorithms. By combining Natural Language Processing (NLP) entity recognition, and advanced tools like the Hyper Intelligence SEO framework, businesses can unlock unprecedented opportunities to enhance their digital presence.
Understanding NLP-Driven Entity Recognition
Entity recognition powered by NLP enables precise identification and categorization of key elements within content. These elements, such as people, locations, brands, and topics, help search engines and users alike understand the context of your content better. Leveraging NLP tools to automate the discovery of such entities ensures:
Enhanced content relevance.
Precise semantic indexing.
Improved user engagement through targeted information.
For example, identifying entities like "SEO analyzer" or "content optimization" ensures that your web pages are contextually rich and aligned with search queries.
The Role of Hyper Intelligence SEO
Hyper Intelligence SEO bridges the gap between analytics, optimization, and predictive insights. This cutting-edge framework provides real-time recommendations to:
Pinpoint content gaps.
Optimize for search intent.
Strengthen linking structures, both internal and external.
It leverages AI-driven algorithms and real-time analytics to predict search trends and guide your strategy towards high-ranking opportunities.
Synergizing Entity Recognition with Hyper Intelligence
Integrating NLP-driven techniques and Hyper Intelligence creates a symbiotic relationship where entity recognition feeds into intelligent SEO workflows. This synergy ensures:
Automated content linking: Connects related topics seamlessly, reducing manual efforts.
Enhanced keyword targeting: Aligns your strategy with trending and contextually relevant search terms.
Scalable optimization: Allows for continual refinement of large-scale SEO campaigns.
For instance, NLP can recognize entities such as "SEO analyzer tools" or "keyword research insights," which Hyper Intelligence uses to suggest optimized linking pathways and metadata strategies.
Impact on SEO Outcomes
By adopting these technologies, businesses can achieve measurable benefits, such as:
Higher organic visibility: Appearing for specific, long-tail queries.
Improved engagement rates: Serving users content that matches their search behavior.
Scalable strategy execution: Automating repetitive tasks like keyword mapping and link insertion.
The Future of Intelligent SEO
As search algorithms continue to evolve, Hyper Intelligence SEO combined with NLP-driven processes will become indispensable for businesses aiming to stay ahead. By aligning technology with user intent, businesses can secure a competitive edge in the ever-dynamic SEO ecosystem.
Embrace this transformation today and explore tools like KBT SEO Analyzer to experience the future of intelligent SEO optimization.
0 notes
Text
AI News Brief 🧞♀️: The Ultimate Guide to LangChain and LangGraph: Which One is Right for You?
#AgenticAI#LangChain#LangGraph#AIFrameworks#MachineLearning#DeepLearning#NaturalLanguageProcessing#NLP#AIApplications#ArtificialIntelligence#AIDevelopment#AIResearch#AIEngineering#AIInnovation#AIIndustry#AICommunity#AIExperts#AIEnthusiasts#AIStartups#AIBusiness#AIFuture#AIRevolution#dozers#FraggleRock#Spideysense#Spiderman#MarvelComics#JimHenson#SpiderSense#FieldsOfTheNephilim
0 notes
Text
Introduction to the LangChain Framework

LangChain is an open-source framework designed to simplify and enhance the development of applications powered by large language models (LLMs). By combining prompt engineering, chaining processes, and integrations with external systems, LangChain enables developers to build applications with powerful reasoning and contextual capabilities. This tutorial introduces the core components of LangChain, highlights its strengths, and provides practical steps to build your first LangChain-powered application.
What is LangChain?
LangChain is a framework that lets you connect LLMs like OpenAI's GPT models with external tools, data sources, and complex workflows. It focuses on enabling three key capabilities: - Chaining: Create sequences of operations or prompts for more complex interactions. - Memory: Maintain contextual memory for multi-turn conversations or iterative tasks. - Tool Integration: Connect LLMs with APIs, databases, or custom functions. LangChain is modular, meaning you can use specific components as needed or combine them into a cohesive application.
Getting Started
Installation First, install the LangChain package using pip: pip install langchain Additionally, you'll need to install an LLM provider (e.g., OpenAI or Hugging Face) and any tools you plan to integrate: pip install openai
Core Concepts in LangChain
1. Chains Chains are sequences of steps that process inputs and outputs through the LLM or other components. Examples include: - Sequential chains: A linear series of tasks. - Conditional chains: Tasks that branch based on conditions. 2. Memory LangChain offers memory modules for maintaining context across multiple interactions. This is particularly useful for chatbots and conversational agents. 3. Tools and Plugins LangChain supports integrations with APIs, databases, and custom Python functions, enabling LLMs to interact with external systems. 4. Agents Agents dynamically decide which tool or chain to use based on the user’s input. They are ideal for multi-tool workflows or flexible decision-making.
Building Your First LangChain Application
In this section, we’ll build a LangChain app that integrates OpenAI’s GPT API, processes user queries, and retrieves data from an external source. Step 1: Setup and Configuration Before diving in, configure your OpenAI API key: import os from langchain.llms import OpenAI # Set API Key os.environ = "your-openai-api-key" # Initialize LLM llm = OpenAI(model_name="text-davinci-003") Step 2: Simple Chain Create a simple chain that takes user input, processes it through the LLM, and returns a result. from langchain.prompts import PromptTemplate from langchain.chains import LLMChain # Define a prompt template = PromptTemplate( input_variables=, template="Explain {topic} in simple terms." ) # Create a chain simple_chain = LLMChain(llm=llm, prompt=template) # Run the chain response = simple_chain.run("Quantum computing") print(response) Step 3: Adding Memory To make the application context-aware, we add memory. LangChain supports several memory types, such as conversational memory and buffer memory. from langchain.chains import ConversationChain from langchain.memory import ConversationBufferMemory # Add memory to the chain memory = ConversationBufferMemory() conversation = ConversationChain(llm=llm, memory=memory) # Simulate a conversation print(conversation.run("What is LangChain?")) print(conversation.run("Can it remember what we talked about?")) Step 4: Integrating Tools LangChain can integrate with APIs or custom tools. Here’s an example of creating a tool for retrieving Wikipedia summaries. from langchain.tools import Tool # Define a custom tool def wikipedia_summary(query: str): import wikipedia return wikipedia.summary(query, sentences=2) # Register the tool wiki_tool = Tool(name="Wikipedia", func=wikipedia_summary, description="Retrieve summaries from Wikipedia.") # Test the tool print(wiki_tool.run("LangChain")) Step 5: Using Agents Agents allow dynamic decision-making in workflows. Let’s create an agent that decides whether to fetch information or explain a topic. from langchain.agents import initialize_agent, Tool from langchain.agents import AgentType # Define tools tools = # Initialize agent agent = initialize_agent(tools, llm, agent=AgentType.ZERO_SHOT_REACT_DESCRIPTION, verbose=True) # Query the agent response = agent.run("Tell me about LangChain using Wikipedia.") print(response) Advanced Topics 1. Connecting with Databases LangChain can integrate with databases like PostgreSQL or MongoDB to fetch data dynamically during interactions. 2. Extending Functionality Use LangChain to create custom logic, such as summarizing large documents, generating reports, or automating tasks. 3. Deployment LangChain applications can be deployed as web apps using frameworks like Flask or FastAPI. Use Cases - Conversational Agents: Develop context-aware chatbots for customer support or virtual assistance. - Knowledge Retrieval: Combine LLMs with external data sources for research and learning tools. - Process Automation: Automate repetitive tasks by chaining workflows. Conclusion LangChain provides a robust and modular framework for building applications with large language models. Its focus on chaining, memory, and integrations makes it ideal for creating sophisticated, interactive applications. This tutorial covered the basics, but LangChain’s potential is vast. Explore the official LangChain documentation for deeper insights and advanced capabilities. Happy coding! Read the full article
#AIFramework#AI-poweredapplications#automation#context-aware#dataintegration#dynamicapplications#LangChain#largelanguagemodels#LLMs#MachineLearning#ML#NaturalLanguageProcessing#NLP#workflowautomation
0 notes
Text
Vee Technologies' Natural Language Processing Services

Using token classification, entity and relation extraction, named entity recognition (NER), entity classification, and contextual analysis, our NLP services can accomplish a wide variety of tasks for your organization.
Explore More: https://www.veetechnologies.com/services/it-services/artificial-intelligence/natural-language-processing.htm
#NaturalLanguageProcessing#NLP#AI#MachineLearning#ArtificialIntelligence#TextAnalysis#DeepLearning#SentimentAnalysis#DataScience
1 note
·
View note
Text
Vee Technologies' Natural Language Processing Services
Using token classification, entity and relation extraction, named entity recognition (NER), entity classification, and contextual analysis, our NLP services can accomplish a wide variety of tasks for your organization.
Explore More: https://www.veetechnologies.com/services/it-services/artificial-intelligence/natural-language-processing.htm
#NaturalLanguageProcessing#NLP#AI#MachineLearning#ArtificialIntelligence#TextAnalysis#DeepLearning#SentimentAnalysis#DataScience#NLPApplications#LanguageTech#SpeechToText#ComputationalLinguistics#AIinLanguage#NLPResearch#VoiceAI#VeeTechnologies
0 notes
Text
Mastering Language Models: AI Conversation Building Block
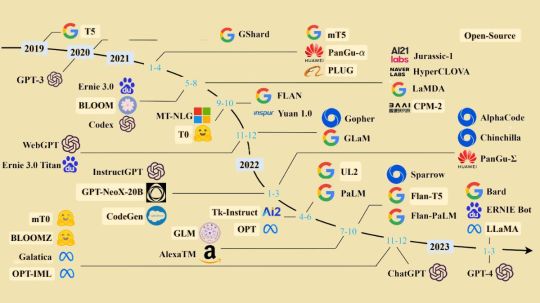
What are language Models?
One kind of machine learning model that has been taught to perform a probability distribution over words is called a language models. In short, a model uses the context of the provided text to forecast the next best word to fill in a blank space in a sentence or phrase.
Since language models enable computers to comprehend, produce, and analyze human language, they are an essential part of natural language processing (NLP). A large text dataset, such a library of books or articles, is mostly used to train them. The next word in a phrase or the creation of fresh, grammatically and semantically coherent material are then predicted by models using the patterns they have learned from this training data.
The Capabilities of language models
Have you ever noticed how the Microsoft SwiftKey and Google Gboard keyboards have clever capabilities that automatically suggest whole phrases while you’re composing text messages? Among the many applications of language models is this one.
Many NLP activities, including text summarization, machine translation, and voice recognition, require it.
Creation of content: Content creation is one of the domains where language models excel. This involves using the information and terms supplied by people to generate whole texts or portions of them. Press releases, blog entries, product descriptions for online stores, poetry, and guitar tabs are just a few examples of the kind of content that may be found there.
POS (part-of-speech) labeling: World-class POS tagging performance is achieved by extensive use of this model. POS tagging assigns a noun, verb, adjective, or other part of speech to each document word. The models can estimate a word’s POS based on its context and the words that surround it in a phrase since they have been trained on vast volumes of annotated text data.
Addressing questions: It is possible to train language models to comprehend and respond to queries both with and without the provided context. They may respond in a variety of ways, such by selecting from a list of possibilities, paraphrasing the response, or extracting certain words.
Summary of a text: Documents, articles, podcasts, movies, and more may all be automatically condensed into their most essential chunks using language models. Models may be used to either summarize the material without using the original language or to extract the most significant information from the original text.
Examination of sentiment: Because it can capture the tone of voice and semantic orientation of texts, the language modeling technique is a strong choice for sentiment analysis applications.
AI that can converse: Voice-enabled apps that need to translate voice to text and voice versa inevitably include language models. This may respond to inputs with relevant text as part of conversational AI systems.
Translation by machine: Machine translation has been improved by ML-powered language models’ capacity to generalize well to lengthy contexts. It may learn the representations of input and output sequences and provide reliable results rather than translating text word for word.
Finishing the code: The capacity of recent large-scale language models to produce, modify, and explain code has been outstanding. They can only, however, translate instructions into code and verify it for mistakes to finish basic programming jobs.
Key aspects of language models
1. Natural Language Processing (NLP)
Language models use NLP approaches to analyze human language and extract meaningful components from words, phrases, and paragraphs.
2. Training
The models are trained on large datasets of text sources such as books, webpages, papers, and more. Training helps them anticipate the next word in a phrase and write like humans by teaching grammar, context, and word connections.
3. Deep Learning
Most recent language models, such as GPT and BERT, rely on deep learning, particularly transformer topologies, to effectively interpret language patterns. Transformers are effective at addressing long-term text dependencies.
4. Applications
It may be used for different activities, such as:
Text creation: Writing tales, poetry, and essays.
Translation: Language translation.
Natural discussion with chatbots.
Summarization: Shortening lengthy articles.
Answering questions with knowledge.
5. Examples
Famous language models include:
GPT-3 by OpenAI generates human-like writing and powers AI apps.
BERT by Google: Useful for search and sentiment analysis, optimized for linguistic context.
The Text-to-Text Transfer Transformer (T5) treats all NLP issues as text production problems and is used for many purposes.
It can allow robots to read, write, and speak like humans.
The Future of language models
Historically, AI business applications concentrated on predictive activities including forecasting, fraud detection, click-through rates, conversions, and low-skill job automation. These restricted uses took great effort to execute and interpret, and were only practical at large scale. However, massive language models altered this.
Large language models like GPT-3 and generative models like Midjouney and DALL-E are transforming the sector, and AI will likely touch practically every part of business in the next years.
Top language model trends are listed below.
Scale and intricacy: The quantity of data and parameters learned on language models will certainly scale.
Multimodality: Integration of language models with visuals, video, and music is intended to enhance their worldview and allow new applications.
Explaining and showing: With more AI in decision-making, ML models must be explainable and transparent. Researchers are trying to make language models more understandable and explain their predictions.
Conversation: It will be utilized increasingly in chatbots, virtual assistants, and customer service to interpret and react to user inputs more naturally.
Language models are projected to improve and be utilized in more applications across fields.
Read more on govindhtech.com
#MasteringLanguageModels#AIConversation#BuildingBlock#machinelearning#voicetext#DALE#naturallanguageprocessing#NLP#OpenAI#ai#Keyaspectslanguagemodels#deeplearning#languagemodels#Applications#technology#technews#news#govindhtech
0 notes
Text
Equipping Businesses with AI: Strategies and Best Practices

AI initiatives will be successful in the long term only if they are led by human expertise. Read More. https://www.sify.com/ai-analytics/equipping-businesses-with-ai-strategies-and-best-practices/
0 notes