#anomaly detection for data quality
Explore tagged Tumblr posts
Text
What is Anomaly Detection? Anomaly detection – also known as outlier analysis – is an approach to data quality control that identifies those data points that lie outside the norms for that dataset.
0 notes
Text
Why You Should Be Analyzing Your Company's Data Usage
Companies use and generate troves of data every year. More data is flowing in and out of a business than most realize. While every bit is important, some datasets hold greater importance than others.
With a data usage analytics platform, organizations can get a clear picture of the data environment and understand how their teams use data. Here are a few reasons to analyze your company's data usage.
Identifying Opportunities to Improve
One of the best reasons to analyze data usage in your organization is to discover new ways to improve. Maximizing productivity is always a top priority, but few companies fully consider how data plays a role in efficiency.
Understanding how the company uses data will enable you to fine-tune operations. Discover which operations are the most effective and use them as a framework to make improvements across the board. Conversely, analyzing data usage enables you to see what operations underperform. That information lets you know what needs work, giving you the tools to make changes that count.
Protect Your Most Vital Assets
Another benefit of using a data usage analytics platform is learning more about your company's most important data assets. Why does that matter? It empowers you to take steps to protect it.
Data quality and resiliency are critical. But it's even more important for the data assets your company relies on most for its success and efficiency. Use what you learn to allocate resources for monitoring and testing. You can spend more time on the data that requires your company's attention.
Save Resources
Data debt and compute costs can lead to inflated budgets and uncontrollable spending. Analyzing data usage is a fantastic way to identify areas where you can save. Discover slow queries that need optimization, perfect latency dependencies for smoother accessibility and more.
Knowing more about how your company uses data enables you to reduce costs as much as possible. You can direct your attention to bloated datasets, clean house and cut back on unnecessary resources used to manage them. It's about optimization throughout your data environment.
Gain insights with the best data observability platform - Explore this website for unparalleled visibility!
#best data observability platform#data anomaly detection software#data usage analytics platform#data quality management software
0 notes
Text
How does AI contribute to the automation of software testing?
AI-Based Testing Services
In today’s modern rapid growing software development competitive market, ensuring and assuming quality while keeping up with fast release cycles is challenging and a vital part. That’s where AI-Based Testing comes into play and role. Artificial Intelligence - Ai is changing the software testing and checking process by making it a faster, smarter, and more accurate option to go for.
Smart Test Case Generation:
AI can automatically & on its own analyze past test results, user behavior, and application logic to generate relevant test cases with its implementation. This reduces the burden on QA teams, saves time, and assures that the key user and scenarios are always covered—something manual processes might overlook and forget.
Faster Bug Detection and Resolution:
AI-Based Testing leverages the machine learning algorithms to detect the defects more efficiently by identifying the code patterns and anomalies in the code behavior and structure. This proactive approach helps and assists the testers to catch the bugs as early as possible in the development cycle, improving product quality and reducing the cost of fixes.
Improved Test Maintenance:
Even a small or minor UI change can break or last the multiple test scripts in traditional automation with its adaptation. The AI models can adapt to these changes, self-heal broken scripts, and update them automatically. This makes test maintenance less time-consuming and more reliable.
Enhanced Test Coverage:
AI assures that broader test coverage and areas are covered by simulating the realtime-user interactions and analyzing vast present datasets into the scenario. It aids to identify the edge cases and potential issues that might not be obvious to human testers. As a result, AI-based testing significantly reduces the risk of bugs in production.
Predictive Analytics for Risk Management:
AI tools and its features can analyze the historical testing data to predict areas of the application or product crafted that are more likely to fail. This insight helps the teams to prioritize their testing efforts, optimize resources, and make better decisions throughout the development lifecycle.
Seamless Integration with Agile and DevOps:
AI-powered testing tools are built to support continuous testing environments. They integrate seamlessly with CI/CD pipelines, enabling faster feedback, quick deployment, and improved collaboration between development and QA teams.
Top technology providers like Suma Soft, IBM, Cyntexa, and Cignex lead the way in AI-Based Testing solutions. They offer and assist with customized services that help the businesses to automate down the Testing process, improve the software quality, and accelerate time to market with advanced AI-driven tools.
#it services#technology#software#saas#saas development company#saas technology#digital transformation#software testing
2 notes
·
View notes
Text
What are the Latest Trends in Building Construction in Dubai?

The construction industry in Dubai has long been a symbol of innovation, luxury, and futuristic design. With its rapid development and architectural marvels, the emirate has consistently set the bar for what is possible in the construction sector. Over the years, the building construction industry in Dubai has evolved significantly, and new trends continue to emerge, reshaping the landscape of urban development. From sustainability to technology integration, Dubai is at the forefront of the latest building construction trends. In this article, we will explore the key trends influencing the building construction sector in Dubai.
1. Sustainable and Green Building Practices
As the global emphasis on sustainability continues to grow, Dubai is also embracing green building practices. This trend is driven by the increasing need for energy-efficient buildings and eco-friendly solutions that minimize the environmental footprint of construction projects. Developers and building construction company in Dubai are now focusing on incorporating sustainable materials, energy-saving technologies, and eco-friendly designs in their projects.
One of the key features of sustainable buildings in Dubai is the use of solar panels. Solar energy is becoming a popular choice for powering buildings, helping to reduce energy consumption and carbon emissions. In addition to this, there is an increased use of recycled materials such as reclaimed wood, steel, and glass, which not only helps to preserve natural resources but also contributes to the reduction of construction waste.
Moreover, Dubai's commitment to sustainability is evident in the numerous green certifications, such as the Dubai Green Building Regulations, which encourage developers to integrate environmentally friendly technologies into their buildings. The use of smart building systems that optimize energy consumption and reduce water usage is also a growing trend. The shift towards green building practices is expected to continue as Dubai aims to reduce its carbon footprint and enhance the quality of life for its residents.
2. Smart and Connected Buildings
The concept of smart buildings has gained significant traction in Dubai over the past few years. With advancements in technology, there is a growing demand for buildings that are equipped with intelligent systems capable of enhancing comfort, convenience, and security. Smart buildings in Dubai are being designed with integrated technologies that allow for real-time monitoring and management of building systems such as lighting, HVAC (heating, ventilation, and air conditioning), security, and energy consumption.
The integration of Internet of Things (IoT) devices into building infrastructure is another trend that is revolutionizing the construction industry in Dubai. These devices can collect data on building performance, detect anomalies, and provide insights into how the building can be optimized for energy efficiency and occupant comfort. For instance, smart thermostats can automatically adjust the temperature based on occupancy, while intelligent lighting systems can dim or brighten according to the time of day or the presence of people.
Building automation systems (BAS) are becoming a crucial part of construction projects, allowing building managers to remotely control and monitor systems, reducing operational costs and improving efficiency. These smart technologies not only enhance the living and working experience but also provide a competitive edge for property developers and investors who are looking to attract tech-savvy tenants.
3. Modular and Prefabricated Construction
Modular and prefabricated construction is gaining popularity in Dubai as a way to speed up the construction process and reduce costs. Prefabricated construction involves assembling parts of a building off-site in a controlled environment before transporting and assembling them at the construction site. This method allows for greater precision and quality control, as the components are built in a factory setting rather than at the construction site.
One of the main benefits of modular and prefabricated construction is the significant reduction in construction time. In Dubai, where there is a constant demand for rapid development, this approach helps to meet deadlines and reduces the overall time required to complete projects. Additionally, it minimizes the disruption to the surrounding environment, as fewer materials are stored and handled on-site.
Another advantage of this construction method is the potential for cost savings. Since much of the work is completed off-site, labor costs are lower, and there is less waste generated. Furthermore, modular and prefabricated construction allows for the creation of more standardized and repeatable designs, which is ideal for large-scale developments such as residential communities or commercial buildings.
4. Use of Advanced Building Materials
Dubai’s construction industry is also witnessing a shift toward the use of advanced building materials that offer improved durability, sustainability, and aesthetic appeal. These materials include high-performance concrete, glass, and steel, as well as newer innovations such as self-healing concrete, transparent aluminum, and carbon fiber.
Self-healing concrete, for example, is a breakthrough material that has the ability to repair its cracks over time. This material can significantly extend the lifespan of buildings and reduce maintenance costs, making it an attractive option for developers in Dubai. Transparent aluminum, on the other hand, offers a unique combination of strength and transparency, making it an ideal material for modern facades and windows.
The use of carbon fiber in construction is also on the rise, particularly in the reinforcement of concrete structures. Carbon fiber is lightweight yet incredibly strong, making it an ideal material for improving the structural integrity of buildings without adding significant weight. As the demand for innovative, high-performance materials continues to grow, building construction companies in Dubai are exploring new ways to incorporate these materials into their projects.
5. Vertical Construction and Skyscrapers
As land space becomes increasingly limited in Dubai, vertical construction continues to be a key trend. Skyscrapers and high-rise buildings are a hallmark of Dubai’s skyline, and this trend is not slowing down. The demand for tall buildings in Dubai is driven by the need to accommodate a growing population and provide commercial and residential spaces in the limited available land area.
Dubai has already set several records in the construction of skyscrapers, including the iconic Burj Khalifa, the tallest building in the world. Vertical construction allows for the development of more compact, space-efficient buildings that maximize the use of available land. In addition, skyscrapers are designed to be multi-purpose, combining residential, commercial, and leisure spaces within the same structure.
In recent years, there has been a push towards even taller and more innovative designs. Architects and developers are constantly pushing the boundaries of what is possible in vertical construction, incorporating advanced technologies and engineering solutions to create more sustainable and energy-efficient high-rise buildings.
6. Luxury and High-End Residential Developments
Dubai has long been known for its luxury real estate market, and this trend shows no signs of slowing down. In recent years, there has been an increasing demand for high-end villas and residential properties that offer exceptional levels of comfort, style, and exclusivity. Developers are focusing on creating luxurious living spaces that cater to the needs and desires of affluent residents.
Villa construction companies in Abu Dhabi and Dubai are capitalizing on this demand by designing and building opulent villas with state-of-the-art amenities, such as private pools, gyms, and expansive gardens. These properties are often located in prime locations, such as beachfront areas or exclusive gated communities, providing residents with the ultimate in privacy and luxury.
The rise of luxury residential developments is also influencing the broader construction market in Dubai. Developers are incorporating cutting-edge designs, high-end finishes, and the latest technologies to ensure that their properties stand out in a competitive market. With a growing number of wealthy investors and residents, the demand for top-tier residential developments is expected to continue.
7. Focus on Mixed-Use Developments
Mixed-use developments are another major trend shaping the construction industry in Dubai. These projects combine residential, commercial, retail, and recreational spaces into a single, integrated development. The goal of mixed-use developments is to create self-sufficient communities where residents can live, work, and play without having to leave the development.
The rise of mixed-use developments in Dubai is driven by the need for more efficient land use and the desire to create vibrant, walkable communities. These developments often feature high-rise buildings, modern amenities, and extensive green spaces, providing a balanced lifestyle for residents and workers alike. Mixed-use developments also offer a sustainable solution to urban growth by reducing the need for long commutes and promoting a more connected, community-oriented way of life.
Conclusion
The construction industry in Dubai is undergoing significant transformations, with new trends and innovations reshaping the way buildings are designed and constructed. From sustainable building practices to the use of cutting-edge technologies, the emirate continues to set the standard for modern construction. As the demand for luxury, smart, and sustainable buildings continues to grow, building construction companies in Dubai will need to adapt and embrace these trends to stay competitive and meet the evolving needs of the market.
With the increasing popularity of modular and prefabricated construction, advanced materials, and vertical construction, Dubai’s skyline will continue to evolve, providing residents and visitors with an ever-changing and dynamic urban landscape. The future of construction in Dubai looks bright, and the trends we are witnessing today are only the beginning of what promises to be an exciting era for the industry.
#Building Contracting Company In Dubai#Building Construction Company In Dubai#General Contracting Company In Abu Dhabi#Best Construction Companies In Abu Dhabi#Contracting Companies In Al Ain#Construction Companies In Al Ain#Interior Design Fit Out Company Dubai#Interior Fit Out Contracting Company Dubai#Fit Out Contracting Company In Abu Dhabi#Villa Construction Companies In Abu Dhabi
2 notes
·
View notes
Text
MedAI by Tech4Biz Solutions: Pioneering Next-Gen Medical Technologies
The healthcare industry is undergoing a seismic shift as advanced technologies continue to transform the way care is delivered. MedAI by Tech4Biz Solutions is at the forefront of this revolution, leveraging artificial intelligence and cutting-edge tools to develop next-generation medical solutions. By enhancing diagnostics, personalizing patient care, and streamlining operations, MedAI is empowering healthcare providers to deliver better outcomes.
1. AI-Driven Medical Insights
MedAI harnesses the power of artificial intelligence to analyze complex medical data and generate actionable insights. Its advanced algorithms can detect anomalies, predict disease progression, and recommend treatment pathways with unprecedented accuracy.
Case Study: A large medical center integrated MedAI’s diagnostic platform, leading to:
Faster identification of rare conditions.
A 30% reduction in misdiagnoses.
Enhanced clinician confidence in treatment decisions.
These capabilities underscore MedAI’s role in advancing clinical decision-making.
2. Personalized Patient Care
Personalization is key to modern healthcare, and MedAI’s data-driven approach ensures treatment plans are tailored to individual needs. By analyzing patient histories, lifestyle factors, and genetic data, MedAI offers more targeted and effective interventions.
Example: A chronic disease management clinic used MedAI to create personalized care plans, resulting in:
Improved medication adherence.
Decreased hospital readmission rates.
Greater patient satisfaction and engagement.
MedAI’s solutions allow providers to offer more precise, patient-centered care.
3. Enhanced Operational Efficiency
MedAI goes beyond clinical improvements by optimizing healthcare operations. Its automation tools reduce administrative burdens, freeing healthcare professionals to focus on patient care.
Insight: A regional hospital implemented MedAI’s workflow automation system, achieving:
A 40% reduction in administrative errors.
Faster patient registration and billing processes.
Streamlined appointment scheduling.
These improvements enhance overall operational efficiency and patient experiences.
4. Advanced Predictive Analytics
Predictive analytics play a vital role in preventive care. MedAI’s algorithms identify patients at high risk of developing chronic conditions, enabling early interventions.
Case Study: A primary care network used MedAI’s predictive models to monitor high-risk patients, leading to:
Early lifestyle adjustments and medical interventions.
A 25% drop in emergency room visits.
Higher enrollment in wellness programs.
By shifting to proactive care, MedAI helps reduce healthcare costs and improve long-term outcomes.
5. Revolutionizing Telemedicine
The rise of telemedicine has been accelerated by MedAI’s AI-powered virtual care solutions. These tools enhance remote consultations by providing real-time patient insights and symptom analysis.
Example: A telehealth provider adopted MedAI’s platform and reported:
Improved diagnostic accuracy during virtual visits.
Reduced wait times for consultations.
Increased access to care for rural and underserved populations.
MedAI’s telemedicine tools ensure equitable, high-quality virtual care for all.
6. Streamlining Drug Development
MedAI accelerates the drug discovery process by analyzing clinical trial data and simulating drug interactions. Its AI models help identify promising compounds faster and improve trial success rates.
Case Study: A pharmaceutical company partnered with MedAI to enhance its drug development process, achieving:
Faster identification of viable drug candidates.
Shorter trial durations.
Reduced costs associated with trial phases.
These innovations are driving faster development of life-saving medications.
7. Natural Language Processing for Clinical Data
MedAI’s natural language processing (NLP) capabilities extract insights from unstructured medical data, such as physician notes and discharge summaries. This allows for faster retrieval of vital patient information.
Insight: A healthcare system implemented MedAI’s NLP engine and experienced:
Improved documentation accuracy.
Quicker clinical decision-making.
Enhanced risk assessment for high-priority cases.
By automating data extraction, MedAI reduces clinician workloads and improves care quality.
8. Robust Data Security and Compliance
Data security is paramount in healthcare. MedAI employs advanced encryption, threat monitoring, and regulatory compliance measures to safeguard patient information.
Example: A hospital using MedAI’s security solutions reported:
Early detection of potential data breaches.
Full compliance with healthcare privacy regulations.
Increased patient trust and confidence in data protection.
MedAI ensures that sensitive medical data remains secure in an evolving digital landscape.
Conclusion
MedAI by Tech4Biz Solutions is redefining healthcare through its pioneering medical technologies. By delivering AI-driven insights, personalized care, operational efficiency, and robust security, MedAI empowers healthcare providers to navigate the future of medicine with confidence.
As healthcare continues to evolve, MedAI remains a trailblazer, driving innovation that transforms patient care and outcomes. Explore MedAI’s comprehensive solutions today and discover the next frontier of medical excellence.
For More Reachout :https://medai.tech4bizsolutions.com/
3 notes
·
View notes
Text
In the subject of data analytics, this is the most important concept that everyone needs to understand. The capacity to draw insightful conclusions from data is a highly sought-after talent in today's data-driven environment. In this process, data analytics is essential because it gives businesses the competitive edge by enabling them to find hidden patterns, make informed decisions, and acquire insight. This thorough guide will take you step-by-step through the fundamentals of data analytics, whether you're a business professional trying to improve your decision-making or a data enthusiast eager to explore the world of analytics.
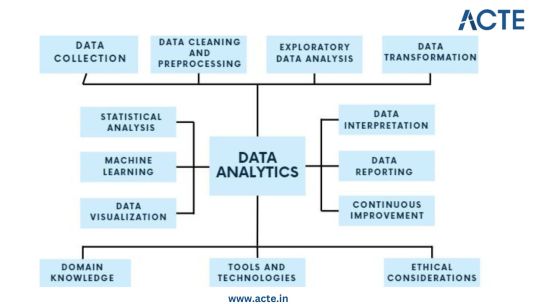
Step 1: Data Collection - Building the Foundation
Identify Data Sources: Begin by pinpointing the relevant sources of data, which could include databases, surveys, web scraping, or IoT devices, aligning them with your analysis objectives. Define Clear Objectives: Clearly articulate the goals and objectives of your analysis to ensure that the collected data serves a specific purpose. Include Structured and Unstructured Data: Collect both structured data, such as databases and spreadsheets, and unstructured data like text documents or images to gain a comprehensive view. Establish Data Collection Protocols: Develop protocols and procedures for data collection to maintain consistency and reliability. Ensure Data Quality and Integrity: Implement measures to ensure the quality and integrity of your data throughout the collection process.
Step 2: Data Cleaning and Preprocessing - Purifying the Raw Material
Handle Missing Values: Address missing data through techniques like imputation to ensure your dataset is complete. Remove Duplicates: Identify and eliminate duplicate entries to maintain data accuracy. Address Outliers: Detect and manage outliers using statistical methods to prevent them from skewing your analysis. Standardize and Normalize Data: Bring data to a common scale, making it easier to compare and analyze. Ensure Data Integrity: Ensure that data remains accurate and consistent during the cleaning and preprocessing phase.
Step 3: Exploratory Data Analysis (EDA) - Understanding the Data
Visualize Data with Histograms, Scatter Plots, etc.: Use visualization tools like histograms, scatter plots, and box plots to gain insights into data distributions and patterns. Calculate Summary Statistics: Compute summary statistics such as means, medians, and standard deviations to understand central tendencies. Identify Patterns and Trends: Uncover underlying patterns, trends, or anomalies that can inform subsequent analysis. Explore Relationships Between Variables: Investigate correlations and dependencies between variables to inform hypothesis testing. Guide Subsequent Analysis Steps: The insights gained from EDA serve as a foundation for guiding the remainder of your analytical journey.
Step 4: Data Transformation - Shaping the Data for Analysis
Aggregate Data (e.g., Averages, Sums): Aggregate data points to create higher-level summaries, such as calculating averages or sums. Create New Features: Generate new features or variables that provide additional context or insights. Encode Categorical Variables: Convert categorical variables into numerical representations to make them compatible with analytical techniques. Maintain Data Relevance: Ensure that data transformations align with your analysis objectives and domain knowledge.
Step 5: Statistical Analysis - Quantifying Relationships
Hypothesis Testing: Conduct hypothesis tests to determine the significance of relationships or differences within the data. Correlation Analysis: Measure correlations between variables to identify how they are related. Regression Analysis: Apply regression techniques to model and predict relationships between variables. Descriptive Statistics: Employ descriptive statistics to summarize data and provide context for your analysis. Inferential Statistics: Make inferences about populations based on sample data to draw meaningful conclusions.
Step 6: Machine Learning - Predictive Analytics
Algorithm Selection: Choose suitable machine learning algorithms based on your analysis goals and data characteristics. Model Training: Train machine learning models using historical data to learn patterns. Validation and Testing: Evaluate model performance using validation and testing datasets to ensure reliability. Prediction and Classification: Apply trained models to make predictions or classify new data. Model Interpretation: Understand and interpret machine learning model outputs to extract insights.
Step 7: Data Visualization - Communicating Insights
Chart and Graph Creation: Create various types of charts, graphs, and visualizations to represent data effectively. Dashboard Development: Build interactive dashboards to provide stakeholders with dynamic views of insights. Visual Storytelling: Use data visualization to tell a compelling and coherent story that communicates findings clearly. Audience Consideration: Tailor visualizations to suit the needs of both technical and non-technical stakeholders. Enhance Decision-Making: Visualization aids decision-makers in understanding complex data and making informed choices.
Step 8: Data Interpretation - Drawing Conclusions and Recommendations
Recommendations: Provide actionable recommendations based on your conclusions and their implications. Stakeholder Communication: Communicate analysis results effectively to decision-makers and stakeholders. Domain Expertise: Apply domain knowledge to ensure that conclusions align with the context of the problem.
Step 9: Continuous Improvement - The Iterative Process
Monitoring Outcomes: Continuously monitor the real-world outcomes of your decisions and predictions. Model Refinement: Adapt and refine models based on new data and changing circumstances. Iterative Analysis: Embrace an iterative approach to data analysis to maintain relevance and effectiveness. Feedback Loop: Incorporate feedback from stakeholders and users to improve analytical processes and models.
Step 10: Ethical Considerations - Data Integrity and Responsibility
Data Privacy: Ensure that data handling respects individuals' privacy rights and complies with data protection regulations. Bias Detection and Mitigation: Identify and mitigate bias in data and algorithms to ensure fairness. Fairness: Strive for fairness and equitable outcomes in decision-making processes influenced by data. Ethical Guidelines: Adhere to ethical and legal guidelines in all aspects of data analytics to maintain trust and credibility.
Data analytics is an exciting and profitable field that enables people and companies to use data to make wise decisions. You'll be prepared to start your data analytics journey by understanding the fundamentals described in this guide. To become a skilled data analyst, keep in mind that practice and ongoing learning are essential. If you need help implementing data analytics in your organization or if you want to learn more, you should consult professionals or sign up for specialized courses. The ACTE Institute offers comprehensive data analytics training courses that can provide you the knowledge and skills necessary to excel in this field, along with job placement and certification. So put on your work boots, investigate the resources, and begin transforming.
24 notes
·
View notes
Text
UAVISUALS: Leading Australia’s Drone Inspection and Data Solutions with Precision and Innovation
Industries across Australia are transforming through the power of drone technology, and UAVISUALS stands at the forefront of this revolution. UAVISUALS offers advanced drone inspections and data solutions to enhance safety, efficiency, and data accuracy across multiple sectors. From construction and energy to environmental monitoring, UAVISUALS empowers industries to leverage aerial data insights that drive better decision-making and operational excellence.
Rethinking Inspections with Drone Technology
Traditional inspection methods are often labor-intensive, costly, and risky, requiring personnel to access difficult or dangerous locations. UAVISUALS redefines this process with drone technology, providing safe, efficient, and detailed inspections without the need for scaffolding, cranes, or extensive downtime. Licensed by the Civil Aviation Safety Authority (CASA), UAVISUALS brings a high standard of safety and regulatory compliance to every mission.
With drones equipped with high-resolution cameras, UAVISUALS captures precise visuals of assets such as power lines, towers, bridges, and rooftops, identifying potential issues before they escalate into costly repairs or operational interruptions. This proactive approach enables companies to conduct timely maintenance, reduce costs, and extend the life of their assets.
Comprehensive Data Solutions Across Industries
UAVISUALS is not just an inspection provider; it’s a full-spectrum data solutions partner. The company offers specialized services tailored to meet the diverse needs of Australia’s industries, including:
Asset and Infrastructure Inspections: UAVISUALS conducts thorough inspections on a wide range of industrial assets, capturing data from hard-to-reach places with exceptional clarity. Their drones can identify issues like corrosion, structural defects, and equipment wear, helping clients address maintenance needs efficiently.
3D Mapping and Topographic Surveys: Using photogrammetry and LiDAR, UAVISUALS generates accurate 3D models and maps, ideal for construction planning, urban development, and land management. These models provide essential measurements and an overall view that enhances precision for project managers, architects, and engineers.
Thermal Imaging and Fault Detection: UAVISUALS’ thermal drones detect temperature anomalies that signal equipment malfunctions or energy inefficiencies, making them invaluable for industries such as renewable energy, utilities, and manufacturing. By spotting issues early, clients can implement corrective actions, reducing risks and avoiding potential failures.
Environmental Monitoring: For agricultural and environmental applications, UAVISUALS offers drone solutions that monitor vegetation health, water quality, and land use. These insights are crucial for sustainable farming practices, conservation efforts, and ecological studies.
Empowering Australian Businesses with Actionable Insights
At UAVISUALS, the goal is not just to collect data but to provide clients with insights they can act on. The UAVISUALS team consists of experienced drone operators, data analysts, and industry experts who turn raw data into meaningful reports and recommendations. Whether it’s helping clients plan maintenance, optimize resource usage, or monitor environmental impacts, UAVISUALS delivers actionable insights that drive smarter, more informed decisions.
Their services streamline processes, save time, and enhance safety, allowing clients to focus on core operations while UAVISUALS handles data capture and analysis. From routine inspections to complex surveys, UAVISUALS’ solutions make data accessible, understandable, and highly relevant to each client’s objectives.
Pioneering the Future of Drone Technology in Australia
As demand for drone technology grows, UAVISUALS remains committed to staying at the cutting edge. The company continually invests in the latest drone models, advanced imaging technology, and data processing tools to ensure clients receive the best solutions available. This dedication to innovation positions UAVISUALS as a leader in the evolving drone services industry, ready to meet the changing needs of Australian businesses.
For companies looking to integrate advanced data solutions, UAVISUALS offers a partnership that combines technological excellence with practical expertise. By transforming how businesses approach inspections, mapping, and monitoring, UAVISUALS enables a safer, smarter, and more sustainable future across Australia.
2 notes
·
View notes
Text
How Large Language Models (LLMs) are Transforming Data Cleaning in 2024
Data is the new oil, and just like crude oil, it needs refining before it can be utilized effectively. Data cleaning, a crucial part of data preprocessing, is one of the most time-consuming and tedious tasks in data analytics. With the advent of Artificial Intelligence, particularly Large Language Models (LLMs), the landscape of data cleaning has started to shift dramatically. This blog delves into how LLMs are revolutionizing data cleaning in 2024 and what this means for businesses and data scientists.
The Growing Importance of Data Cleaning
Data cleaning involves identifying and rectifying errors, missing values, outliers, duplicates, and inconsistencies within datasets to ensure that data is accurate and usable. This step can take up to 80% of a data scientist's time. Inaccurate data can lead to flawed analysis, costing businesses both time and money. Hence, automating the data cleaning process without compromising data quality is essential. This is where LLMs come into play.
What are Large Language Models (LLMs)?
LLMs, like OpenAI's GPT-4 and Google's BERT, are deep learning models that have been trained on vast amounts of text data. These models are capable of understanding and generating human-like text, answering complex queries, and even writing code. With millions (sometimes billions) of parameters, LLMs can capture context, semantics, and nuances from data, making them ideal candidates for tasks beyond text generation—such as data cleaning.
To see how LLMs are also transforming other domains, like Business Intelligence (BI) and Analytics, check out our blog How LLMs are Transforming Business Intelligence (BI) and Analytics.

Traditional Data Cleaning Methods vs. LLM-Driven Approaches
Traditionally, data cleaning has relied heavily on rule-based systems and manual intervention. Common methods include:
Handling missing values: Methods like mean imputation or simply removing rows with missing data are used.
Detecting outliers: Outliers are identified using statistical methods, such as standard deviation or the Interquartile Range (IQR).
Deduplication: Exact or fuzzy matching algorithms identify and remove duplicates in datasets.
However, these traditional approaches come with significant limitations. For instance, rule-based systems often fail when dealing with unstructured data or context-specific errors. They also require constant updates to account for new data patterns.
LLM-driven approaches offer a more dynamic, context-aware solution to these problems.

How LLMs are Transforming Data Cleaning
1. Understanding Contextual Data Anomalies
LLMs excel in natural language understanding, which allows them to detect context-specific anomalies that rule-based systems might overlook. For example, an LLM can be trained to recognize that “N/A” in a field might mean "Not Available" in some contexts and "Not Applicable" in others. This contextual awareness ensures that data anomalies are corrected more accurately.
2. Data Imputation Using Natural Language Understanding
Missing data is one of the most common issues in data cleaning. LLMs, thanks to their vast training on text data, can fill in missing data points intelligently. For example, if a dataset contains customer reviews with missing ratings, an LLM could predict the likely rating based on the review's sentiment and content.
A recent study conducted by researchers at MIT (2023) demonstrated that LLMs could improve imputation accuracy by up to 30% compared to traditional statistical methods. These models were trained to understand patterns in missing data and generate contextually accurate predictions, which proved to be especially useful in cases where human oversight was traditionally required.
3. Automating Deduplication and Data Normalization
LLMs can handle text-based duplication much more effectively than traditional fuzzy matching algorithms. Since these models understand the nuances of language, they can identify duplicate entries even when the text is not an exact match. For example, consider two entries: "Apple Inc." and "Apple Incorporated." Traditional algorithms might not catch this as a duplicate, but an LLM can easily detect that both refer to the same entity.
Similarly, data normalization—ensuring that data is formatted uniformly across a dataset—can be automated with LLMs. These models can normalize everything from addresses to company names based on their understanding of common patterns and formats.
4. Handling Unstructured Data
One of the greatest strengths of LLMs is their ability to work with unstructured data, which is often neglected in traditional data cleaning processes. While rule-based systems struggle to clean unstructured text, such as customer feedback or social media comments, LLMs excel in this domain. For instance, they can classify, summarize, and extract insights from large volumes of unstructured text, converting it into a more analyzable format.
For businesses dealing with social media data, LLMs can be used to clean and organize comments by detecting sentiment, identifying spam or irrelevant information, and removing outliers from the dataset. This is an area where LLMs offer significant advantages over traditional data cleaning methods.
For those interested in leveraging both LLMs and DevOps for data cleaning, see our blog Leveraging LLMs and DevOps for Effective Data Cleaning: A Modern Approach.

Real-World Applications
1. Healthcare Sector
Data quality in healthcare is critical for effective treatment, patient safety, and research. LLMs have proven useful in cleaning messy medical data such as patient records, diagnostic reports, and treatment plans. For example, the use of LLMs has enabled hospitals to automate the cleaning of Electronic Health Records (EHRs) by understanding the medical context of missing or inconsistent information.
2. Financial Services
Financial institutions deal with massive datasets, ranging from customer transactions to market data. In the past, cleaning this data required extensive manual work and rule-based algorithms that often missed nuances. LLMs can assist in identifying fraudulent transactions, cleaning duplicate financial records, and even predicting market movements by analyzing unstructured market reports or news articles.
3. E-commerce
In e-commerce, product listings often contain inconsistent data due to manual entry or differing data formats across platforms. LLMs are helping e-commerce giants like Amazon clean and standardize product data more efficiently by detecting duplicates and filling in missing information based on customer reviews or product descriptions.

Challenges and Limitations
While LLMs have shown significant potential in data cleaning, they are not without challenges.
Training Data Quality: The effectiveness of an LLM depends on the quality of the data it was trained on. Poorly trained models might perpetuate errors in data cleaning.
Resource-Intensive: LLMs require substantial computational resources to function, which can be a limitation for small to medium-sized enterprises.
Data Privacy: Since LLMs are often cloud-based, using them to clean sensitive datasets, such as financial or healthcare data, raises concerns about data privacy and security.

The Future of Data Cleaning with LLMs
The advancements in LLMs represent a paradigm shift in how data cleaning will be conducted moving forward. As these models become more efficient and accessible, businesses will increasingly rely on them to automate data preprocessing tasks. We can expect further improvements in imputation techniques, anomaly detection, and the handling of unstructured data, all driven by the power of LLMs.
By integrating LLMs into data pipelines, organizations can not only save time but also improve the accuracy and reliability of their data, resulting in more informed decision-making and enhanced business outcomes. As we move further into 2024, the role of LLMs in data cleaning is set to expand, making this an exciting space to watch.
Large Language Models are poised to revolutionize the field of data cleaning by automating and enhancing key processes. Their ability to understand context, handle unstructured data, and perform intelligent imputation offers a glimpse into the future of data preprocessing. While challenges remain, the potential benefits of LLMs in transforming data cleaning processes are undeniable, and businesses that harness this technology are likely to gain a competitive edge in the era of big data.
#Artificial Intelligence#Machine Learning#Data Preprocessing#Data Quality#Natural Language Processing#Business Intelligence#Data Analytics#automation#datascience#datacleaning#large language model#ai
2 notes
·
View notes
Text
honestly what's most frustrating about ai is that it has so much potential to be such a powerful helping tool
like. heres technology that could be used to detect and fix bugs in data protection software. or aid doctors and scientists with elaborate sequences, finding miniscule anomalies that could help detect otherwise fatal diseases early.
it could be used to translate a phone conversation in real time for people who live in a place where they dont speak the language. Heres something that could really help in the pursuit of knowledge by directing you to new sources and providing data you may not have considered, given you cross-reference to make sure what you have is real and relevant.
but no we insist on using it to make shitty memes and replace hard working writers and artists with extremely garbage quality souless image or script generation. uhg.
#i loooooooove being an illustrator in todays climate. :)#ai#fuck i hate it here#half of the comedy of a super deranged meme used to be that someone likely spent an absurd amount of time making it#now its just like. idk the charm isnt there. the shitty ugly deeprfried textures are all replaced with smooth “perfection”#everywhere i see more and more posters using generative ai#it kills me
2 notes
·
View notes
Text
Biometric Attendance Machine
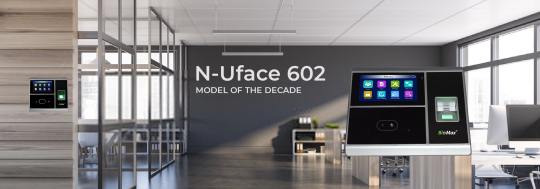
A biometric attendance machine is a technology used to track and manage employee attendance based on biometric data, such as fingerprints, facial recognition, or iris scans. These systems are often employed in workplaces, educational institutions, and other organizations to ensure accurate and secure tracking of time and attendance. Here’s a comprehensive overview of biometric attendance machines:
Types of Biometric Attendance Machines
Fingerprint Scanners
Description: Use fingerprint recognition to verify identity. Employees place their finger on a sensor, and the system matches the fingerprint against a stored template.
Pros: Quick and reliable; well-suited for high-traffic areas.
Cons: May be less effective with dirty or damaged fingers; requires regular cleaning.
Facial Recognition Systems
Description: Use facial recognition technology to identify individuals based on their facial features. Employees look into a camera, and the system matches their face against a database.
Pros: Contactless and convenient; can be integrated with other security measures.
Cons: May be affected by changes in lighting or facial features; requires good camera quality.
Iris Scanners
Description: Scan the unique patterns in the iris of the eye to identify individuals. Employees look into a device that captures the iris pattern.
Pros: Highly accurate; difficult to spoof.
Cons: Typically more expensive; requires careful alignment.
Voice Recognition Systems
Description: Use voice patterns for identification. Employees speak into a microphone, and the system analyzes their voice.
Pros: Contactless; can be used in various environments.
Cons: Can be affected by background noise or voice changes.
Hand Geometry Systems
Description: Measure the shape and size of the hand and fingers. Employees place their hand on a scanner, which records its dimensions.
Pros: Effective and reliable; less invasive.
Cons: Requires specific hand placement; less common than fingerprint or facial recognition systems.
Key Features
Data Storage and Management
Centralized Database: Stores biometric data and attendance records securely.
Integration: Often integrates with HR and payroll systems to streamline data management.
Accuracy and Speed
High Accuracy: Minimizes errors and false positives/negatives in identification.
Fast Processing: Ensures quick check-in and check-out times for employees.
Security
Data Encryption: Protects biometric data with encryption to prevent unauthorized access.
Anti-Spoofing: Includes features to detect and prevent fraudulent attempts, such as using fake fingerprints or photos.
User Interface
Ease of Use: Features a simple interface for both employees and administrators.
Reporting: Generates detailed reports on attendance, overtime, and absences.
Customization
Settings: Allows customization of attendance policies, work schedules, and shift timings.
Alerts and Notifications: Sends alerts for exceptions or anomalies, such as missed clock-ins or outs.
Benefits
Improved Accuracy: Reduces errors and fraud associated with manual or card-based systems.
Enhanced Security: Ensures that only authorized personnel can access facilities and clock in/out.
Time Efficiency: Speeds up the check-in and check-out process, reducing queues and wait times.
Automated Tracking: Automates attendance management, reducing administrative workload.
Detailed Reporting: Provides comprehensive data on attendance patterns, helping with workforce management and planning.
Considerations
Privacy Concerns: Ensure compliance with privacy laws and regulations regarding biometric data collection and storage.
Cost: Evaluate the initial investment and ongoing maintenance costs. High-end biometric systems may be more expensive.
Integration: Consider how well the system integrates with existing HR and payroll software.
User Acceptance: Provide training to employees and address any concerns about the use of biometric technology.
Popular Brands and Models
ZKTeco: Known for a wide range of biometric solutions, including fingerprint and facial recognition systems.
Hikvision: Offers advanced facial recognition systems with integrated attendance management.
Suprema: Provides high-quality fingerprint and facial recognition devices.
BioTime: Specializes in biometric attendance systems with robust reporting and integration features.
Anviz: Offers various biometric solutions, including fingerprint and facial recognition devices.
By choosing the right biometric attendance machine and properly implementing it, organizations can improve attendance tracking, enhance security, and streamline HR processes.
2 notes
·
View notes
Text
What are the uses of a Weighbridge?
A weighbridge is a large industrial scale used primarily for weighing trucks, trailers, and their contents. Here are some common uses of a weighbridge:
Weight Verification: Weighbridges are used to accurately measure the weight of trucks and their cargo to ensure compliance with legal weight limits. This is crucial for safety reasons and to prevent damage to roads and infrastructure.
Trade Transactions: Weighbridges are often used in industries where goods are bought and sold by weight, such as agriculture, mining, waste management, and logistics. They provide an official and accurate measurement of the goods being traded.
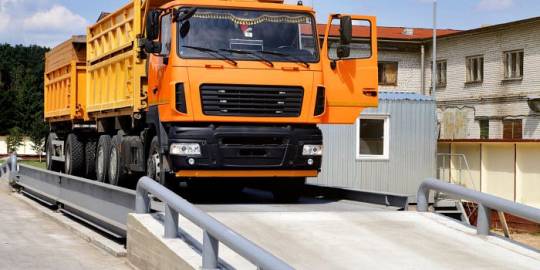
3. Inventory Management: Weighbridges help businesses manage their inventory by accurately measuring the weight of incoming and outgoing goods. This information is essential for stock control, logistics planning, and financial reporting.
4. Quality Control: In industries where product quality is closely tied to weight, such as food processing or manufacturing, weighbridges ensure consistency and adherence to quality standards.
5. Taxation and Fees: Governments may use weighbridge data to levy taxes or fees based on the weight of goods transported, especially for heavy vehicles that put more strain on infrastructure.
6. Safety and Compliance: Weighbridges help enforce safety regulations by ensuring vehicles are not overloaded, which can lead to accidents, road damage, and environmental hazards.
7. Data Collection and Analysis: Modern weighbridges are often equipped with software that collects and analyzes weight data. This data can be used for trend analysis, forecasting, and optimizing operational efficiencies.
8. Security Checks: Weighbridges are sometimes used at security checkpoints to verify the weight of vehicles and detect any anomalies that may indicate unauthorized cargo or activities.
Overall, weighbridges play a crucial role in various industries by providing accurate weight measurements that are essential for compliance, financial transactions, operational efficiency, and safety.
For more details, please contact us!
Website :- https://www.essaarweigh.com/
Contact No. :- 09310648864, 09810648864, 09313051477
Email :- [email protected]
#Weighbridge#fully electronic weighbridge#Weighbridge manufacturer in India#Weighbridge manufacturer in Delhi#Weighbridge manufacturer in Ghaziabad#Weighbridge manufacturer in Noida#Weighbridge manufacturer in Gurugram#Weighbridge manufacturer in Faridabad#Weighbridge supplier in India#Essaar weigh
2 notes
·
View notes
Text
How AI is Reshaping the Future of Fintech Technology
In the rapidly evolving landscape of financial technology (fintech), the integration of artificial intelligence (AI) is reshaping the future in profound ways. From revolutionizing customer experiences to optimizing operational efficiency, AI is unlocking new opportunities for innovation and growth across the fintech ecosystem. As a pioneer in fintech software development, Xettle Technologies is at the forefront of leveraging AI to drive transformative change and shape the future of finance.
Fintech technology encompasses a wide range of solutions, including digital banking, payment processing, wealth management, and insurance. In each of these areas, AI is playing a pivotal role in driving innovation, enhancing competitiveness, and delivering value to businesses and consumers alike.
One of the key areas where AI is reshaping the future of fintech technology is in customer experiences. Through techniques such as natural language processing (NLP) and machine learning, AI-powered chatbots and virtual assistants are revolutionizing the way customers interact with financial institutions.
Xettle Technologies has pioneered the integration of AI-powered chatbots into its digital banking platforms, providing customers with personalized assistance and support around the clock. These chatbots can understand and respond to natural language queries, provide account information, offer product recommendations, and even execute transactions, all in real-time. By delivering seamless and intuitive experiences, AI-driven chatbots enhance customer satisfaction, increase engagement, and drive loyalty.
Moreover, AI is enabling financial institutions to gain deeper insights into customer behavior, preferences, and needs. Through advanced analytics and predictive modeling, AI algorithms can analyze vast amounts of data to identify patterns, trends, and correlations that were previously invisible to human analysts.
Xettle Technologies' AI-powered analytics platforms leverage machine learning to extract actionable insights from transaction data, social media activity, and other sources. By understanding customer preferences and market dynamics more accurately, businesses can tailor their offerings, refine their marketing strategies, and drive growth in targeted segments.
AI is also transforming the way financial institutions manage risk and detect fraud. Through the use of advanced algorithms and data analytics, AI can analyze transaction patterns, detect anomalies, and identify potential threats in real-time.
Xettle Technologies has developed sophisticated fraud detection systems that leverage AI to monitor transactions, identify suspicious activity, and prevent fraudulent transactions before they occur. By continuously learning from new data and adapting to emerging threats, these AI-powered systems provide businesses with robust security measures and peace of mind.
In addition to enhancing customer experiences and mitigating risks, AI is driving operational efficiency and innovation in fintech software development. Through techniques such as robotic process automation (RPA) and intelligent workflow management, AI-powered systems can automate routine tasks, streamline processes, and accelerate time-to-market for new products and services.
Xettle Technologies has embraced AI-driven automation across its software development lifecycle, from code generation and testing to deployment and maintenance. By automating repetitive tasks and optimizing workflows, Xettle's development teams can focus on innovation and value-added activities, delivering high-quality fintech solutions more efficiently and effectively.
Looking ahead, the integration of AI into fintech technology is expected to accelerate, driven by advancements in machine learning, natural language processing, and computational power. As AI algorithms become more sophisticated and data sources become more diverse, the potential for innovation in fintech software is virtually limitless.
For Xettle Technologies, this presents a unique opportunity to continue pushing the boundaries of what is possible in fintech innovation. By investing in research and development, forging strategic partnerships, and staying ahead of emerging trends, Xettle is committed to delivering cutting-edge solutions that empower businesses, drive growth, and shape the future of finance.
In conclusion, AI is reshaping the future of fintech technology in profound and exciting ways. From enhancing customer experiences and mitigating risks to driving operational efficiency and innovation, AI-powered solutions hold immense potential for businesses and consumers alike. As a leader in fintech software development, Xettle Technologies is at the forefront of this transformation, leveraging AI to drive meaningful change and shape the future of finance.
#Fintech Technologies#Fintech Software#Artificial Intelligence#Finance#Fintech Startups#technology#ecommerce#fintech#xettle technologies#writers on tumblr
6 notes
·
View notes
Text
How To Prevent Fraud In Custom Online Panel Research?

Custom online panel research has emerged as a powerful method for gathering targeted insights from specific demographics or groups. However, amidst its efficacy, ensuring the integrity and authenticity of data remains a critical concern. Fraudulent activities such as bot responses, duplicate entries, and dishonest participation can compromise the reliability and validity of research findings. Implementing robust measures to prevent fraud is imperative for maintaining the credibility and value of custom online panel research.
Strategic Participant Screening: Implementing stringent screening processes for panel participants is the first line of defense against fraudulent entries. Thoroughly vetting and verifying participants through detailed registration forms, including qualifying questions, and verifying identities can significantly reduce the likelihood of fraudulent or dishonest responses.
Utilize CAPTCHA and Human Verification: Integrating CAPTCHA challenges or other human verification tools in the survey process can help differentiate between human respondents and automated bots. These mechanisms ensure that real individuals are participating in the research, minimizing the risk of bot-generated responses.
IP Address Monitoring and Restrictions: Tracking IP addresses and restricting multiple responses from the same IP or device helps prevent duplicate entries. Implementing limitations on the number of responses per IP address or device ensures that each participant contributes only once, maintaining data accuracy.
Implement Data Validation Checks: Employing data validation checks within the survey ensures that responses align with expected patterns or logic. Flagging inconsistent or improbable responses for further review can help identify potential fraudulent entries.
Educate Participants on Expectations: Clearly communicate survey guidelines, expectations, and the importance of honest and accurate responses to participants. Emphasize the significance of truthful participation in maintaining research integrity, encouraging ethical behavior among panel members.
Anonymous Responses with Confidentiality Assurances: Assure participants of the confidentiality of their responses and provide options for anonymous participation when feasible. Creating a secure and non-judgmental environment encourages truthful responses and discourages fraudulent behaviors.
Regular Audits and Monitoring: Conduct regular audits and data checks to identify anomalies or suspicious patterns in responses. Proactive monitoring allows for timely intervention and corrective actions to maintain the quality and authenticity of collected data.
Utilize Technology and Advanced Algorithms: Leverage advanced technologies such as AI-based fraud detection algorithms to identify and flag potentially fraudulent responses. These algorithms can detect anomalies in response patterns or behavior, alerting administrators for further investigation.
Compliance with Ethical Standards and Regulations: Adhere to ethical research standards and comply with relevant regulations like GDPR, CCPA, or industry-specific guidelines. Ensuring transparency, consent, and compliance with data protection regulations builds trust and credibility in panel research practices.
Continuous Improvement and Adaptation: Stay vigilant and continuously adapt strategies to evolving fraud tactics. Regularly update security measures, improve screening processes, and incorporate new technologies to stay ahead of potential fraudulent activities.
By implementing a combination of these preventive measures and maintaining a proactive stance against fraudulent activities, researchers and organizations can enhance the credibility and reliability of custom online panel research. Upholding research integrity not only ensures the quality of insights but also fosters trust among participants and stakeholders, reinforcing the value of data collected through online panel research.
To know more: fraud detection and reporting tool
survey fraud detection tool
3 notes
·
View notes
Text
Daniel Reitberg: Harnessing AI for Enhanced Production Cycle Optimization and Forecasting Accuracy in the Chemical Industry

Introduction
The chemical industry is constantly seeking ways to optimize production cycles and improve forecasting accuracy to meet the ever-changing market demands. In this article, we delve into the groundbreaking applications of artificial intelligence (AI) in achieving these goals. Daniel Reitberg, an esteemed AI expert, is at the forefront of revolutionizing the chemical industry with his innovative approaches to production optimization and forecasting accuracy.
Enhancing Production Cycle Efficiency through AI
AI algorithms developed by Daniel Reitberg analyze vast amounts of data, including production records, equipment performance, and environmental factors, to extract valuable insights. By leveraging machine learning and predictive analytics, AI systems can identify patterns, detect anomalies, and optimize various aspects of the production cycle. This results in enhanced efficiency, reduced waste, and improved overall productivity.
Improving Forecasting Accuracy with AI
Accurate forecasting is crucial in the chemical industry to align production capacity with market demand. Daniel Reitberg's expertise in AI enables him to develop advanced forecasting models that take into account historical data, market trends, and external factors. By leveraging AI algorithms, these models can make accurate predictions, enabling chemical companies to optimize their inventory management, reduce costs, and improve customer satisfaction.
The Benefits of AI in the Chemical Industry
The application of AI in optimizing production cycles and improving forecasting accuracy brings numerous benefits to the chemical industry. AI-driven production optimization reduces operational costs, minimizes downtime, and ensures consistent product quality. By leveraging AI for forecasting accuracy, chemical companies can make informed decisions regarding resource allocation, production planning, and market strategies. This leads to improved profitability, reduced waste, and enhanced competitiveness.
Conclusion
Daniel Reitberg's pioneering work in AI-driven production optimization and forecasting accuracy is reshaping the chemical industry. By leveraging the power of AI, chemical companies can achieve unprecedented levels of efficiency, accuracy, and profitability. As AI technology continues to advance, experts like Daniel Reitberg will continue to drive innovation and transform the way the chemical industry operates. With AI, the future of production optimization and forecasting accuracy in the chemical industry is brighter than ever before.
3 notes
·
View notes
Text
Monitoring health care safety using SEnergy IoT
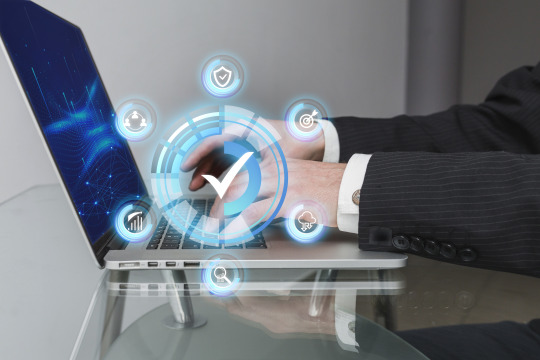
Monitoring healthcare safety using IoT (Internet of Things) technology, including SEnergy IoT, can greatly enhance patient care, streamline operations, and improve overall safety in healthcare facilities. SEnergy IoT, if specialized for healthcare applications, can offer several advantages in this context. Here's how monitoring healthcare safety using SEnergy IoT can be beneficial:
Patient Monitoring: SEnergy IoT can be used to monitor patient vital signs in real-time. Wearable devices equipped with sensors can track heart rate, blood pressure, temperature, and other critical parameters. Any deviations from normal values can trigger alerts to healthcare providers, allowing for timely intervention.
Fall Detection: IoT sensors, including accelerometers and motion detectors, can be used to detect falls in patients, especially the elderly or those with mobility issues. Alerts can be sent to healthcare staff, reducing response times and minimizing the risk of injuries.
Medication Management: IoT can be used to ensure medication adherence. Smart pill dispensers can remind patients to take their medications, dispense the correct dosage, and send notifications to caregivers or healthcare providers in case of missed doses.
Infection Control: SEnergy IoT can help monitor and control infections within healthcare facilities. Smart sensors can track hand hygiene compliance, air quality, and the movement of personnel and patients, helping to identify and mitigate potential sources of infection.
Asset Tracking: IoT can be used to track and manage medical equipment and supplies, ensuring that critical resources are always available when needed. This can reduce the risk of equipment shortages or misplacement.
Environmental Monitoring: SEnergy IoT can monitor environmental factors such as temperature, humidity, and air quality in healthcare facilities. This is crucial for maintaining the integrity of medications, medical devices, and the comfort of patients and staff.
Security and Access Control: IoT can enhance security within healthcare facilities by providing access control systems that use biometrics or smart cards. It can also monitor unauthorized access to sensitive areas and send alerts in real-time.
Patient Privacy: SEnergy IoT can help ensure patient privacy and data security by implementing robust encryption and access control measures for healthcare data transmitted over the network.
Predictive Maintenance: IoT sensors can be used to monitor the condition of critical equipment and predict when maintenance is needed. This proactive approach can reduce downtime and improve the safety of medical devices.
Emergency Response: In case of emergencies, SEnergy IoT can automatically trigger alerts and initiate emergency response protocols. For example, in the event of a fire, IoT sensors can detect smoke or elevated temperatures and activate alarms and evacuation procedures.
Data Analytics: The data collected through SEnergy IoT devices can be analyzed to identify trends, patterns, and anomalies. This can help healthcare providers make informed decisions, improve patient outcomes, and enhance safety protocols.
Remote Monitoring: IoT enables remote monitoring of patients, allowing healthcare providers to keep an eye on patients' health and well-being even when they are not in a healthcare facility.
Compliance and Reporting: SEnergy IoT can facilitate compliance with regulatory requirements by automating data collection and reporting processes, reducing the risk of errors and non-compliance.
To effectively implement SEnergy IoT for healthcare safety, it's crucial to address privacy and security concerns, ensure interoperability among various devices and systems, and establish clear protocols for responding to alerts and data analysis. Additionally, healthcare professionals should be trained in using IoT solutions to maximize their benefits and ensure patient safety.
2 notes
·
View notes
Text
"Unveiling the Future: How Data Science is Revolutionizing Upcoming Industries"
Data science continues to have a substantial impact on various industries, and its scope is expected to expand as new technologies emerge and businesses realize the potential of data-driven insights. Here are some upcoming industries where data science is likely to play a significant role:
Healthcare and Life Sciences: Data science can aid in personalized medicine, drug discovery, predictive analytics for patient outcomes, and healthcare operations optimization.
Financial Services: Financial institutions use data science for fraud detection, risk assessment, algorithmic trading, customer behavior analysis, and credit scoring.
Retail and E-Commerce: Data science helps optimize inventory management, pricing strategies, recommendation systems, and customer segmentation for targeted marketing.
Energy and Utilities: The energy sector benefits from data analytics for smart grid management, predictive maintenance of equipment, and energy consumption optimization.
Manufacturing: Data science improves manufacturing processes through predictive maintenance, quality control, supply chain optimization, and demand forecasting.
Agriculture: Precision agriculture utilizes data science to optimize crop yield, resource allocation, pest control, and environmental monitoring.
Transportation and Logistics: Data science plays a role in route optimization, fleet management, demand forecasting, and autonomous vehicles.
Telecommunications: Data science assists in customer churn prediction, network optimization, and personalized service offerings.
Media and Entertainment: Content recommendation, audience segmentation, and analyzing viewer engagement are areas where data science is making an impact.
Real Estate: Data science helps in property price prediction, market trend analysis, and investment decision-making.
Environmental Conservation: Data science aids in monitoring and analyzing environmental data, including climate patterns, pollution levels, and habitat preservation.
Education: Data science can personalize learning experiences, assess student performance, and optimize educational resources.
Government and Public Services: Data-driven decision-making is becoming increasingly important for optimizing public services, policy formulation, and resource allocation.
Insurance: Insurers use data science for risk assessment, claims processing, fraud detection, and customized pricing.
Travel and Tourism: Data science enhances traveler experiences through personalized recommendations, pricing optimization, and destination insights.
Pharmaceuticals: Data science plays a role in drug discovery, clinical trials optimization, and pharmacovigilance.
Smart Cities: The concept of smart cities involves integrating data science for efficient urban planning, traffic management, energy consumption, and public services.
Cybersecurity: Data science helps in identifying and responding to cyber threats by analyzing patterns and anomalies in network data.
As technology continues to advance and businesses recognize the value of data-driven insights, certybox is creating a difference in providing the top professional courses along with job assistance. It's essential for professionals in the field to stay updated with the latest developments and tools to make the most of these opportunities.
5 notes
·
View notes