#data annotation in machine learning
Explore tagged Tumblr posts
Text
Betting Big on Data Annotation Companies: Building the Future of AI Models
Explore the pivotal role of data annotation companies in shaping the future of AI models. Delve into the strategies, innovations, and market dynamics driving these companies to the forefront of the AI revolution. Gain insights into the profound impact of meticulous data labeling on the efficacy of Machine Learning algorithms. Read the blog:…
View On WordPress
#data annotation#data annotation companies#data annotation company#data annotation for AI/ML#Data Annotation in Machine Learning
0 notes
Text
🛎 Ensure Accuracy in Labeling With AI Data Annotation Services
🚦 The demand for speed in data labeling annotation has reached unprecedented levels. Damco integrates predictive and automated AI data annotation with the expertise of world-class annotators and subject matter specialists to provide the training sets required for rapid production. All annotation services work is turned around rapidly by a highly qualified team of subject matter experts.
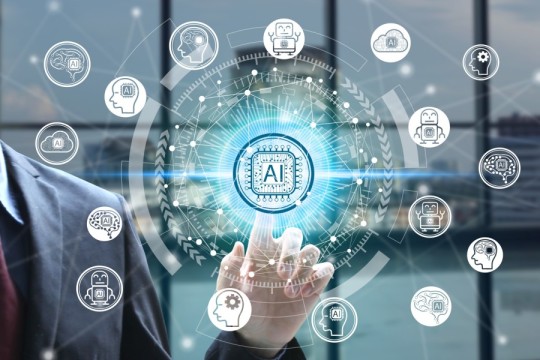
#AI data annotation#data annotation in machine learning#data annotation for ml#data annotation company#data annotation#data annotation services
0 notes
Text
What is a Data pipeline for Machine Learning?
As machine learning technologies continue to advance, the need for high-quality data has become increasingly important. Data is the lifeblood of computer vision applications, as it provides the foundation for machine learning algorithms to learn and recognize patterns within images or video. Without high-quality data, computer vision models will not be able to effectively identify objects, recognize faces, or accurately track movements.
Machine learning algorithms require large amounts of data to learn and identify patterns, and this is especially true for computer vision, which deals with visual data. By providing annotated data that identifies objects within images and provides context around them, machine learning algorithms can more accurately detect and identify similar objects within new images.
Moreover, data is also essential in validating computer vision models. Once a model has been trained, it is important to test its accuracy and performance on new data. This requires additional labeled data to evaluate the model's performance. Without this validation data, it is impossible to accurately determine the effectiveness of the model.
Data Requirement at multiple ML stage
Data is required at various stages in the development of computer vision systems.
Here are some key stages where data is required:
Training: In the training phase, a large amount of labeled data is required to teach the machine learning algorithm to recognize patterns and make accurate predictions. The labeled data is used to train the algorithm to identify objects, faces, gestures, and other features in images or videos.
Validation: Once the algorithm has been trained, it is essential to validate its performance on a separate set of labeled data. This helps to ensure that the algorithm has learned the appropriate features and can generalize well to new data.
Testing: Testing is typically done on real-world data to assess the performance of the model in the field. This helps to identify any limitations or areas for improvement in the model and the data it was trained on.
Re-training: After testing, the model may need to be re-trained with additional data or re-labeled data to address any issues or limitations discovered in the testing phase.
In addition to these key stages, data is also required for ongoing model maintenance and improvement. As new data becomes available, it can be used to refine and improve the performance of the model over time.
Types of Data used in ML model preparation
The team has to work on various types of data at each stage of model development.
Streamline, structured, and unstructured data are all important when creating computer vision models, as they can each provide valuable insights and information that can be used to train the model.
Streamline data refers to data that is captured in real-time or near real-time from a single source. This can include data from sensors, cameras, or other monitoring devices that capture information about a particular environment or process.
Structured data, on the other hand, refers to data that is organized in a specific format, such as a database or spreadsheet. This type of data can be easier to work with and analyze, as it is already formatted in a way that can be easily understood by the computer.
Unstructured data includes any type of data that is not organized in a specific way, such as text, images, or video. This type of data can be more difficult to work with, but it can also provide valuable insights that may not be captured by structured data alone.
When creating a computer vision model, it is important to consider all three types of data in order to get a complete picture of the environment or process being analyzed. This can involve using a combination of sensors and cameras to capture streamline data, organizing structured data in a database or spreadsheet, and using machine learning algorithms to analyze and make sense of unstructured data such as images or text. By leveraging all three types of data, it is possible to create a more robust and accurate computer vision model.
Data Pipeline for machine learning
The data pipeline for machine learning involves a series of steps, starting from collecting raw data to deploying the final model. Each step is critical in ensuring the model is trained on high-quality data and performs well on new inputs in the real world.
Below is the description of the steps involved in a typical data pipeline for machine learning and computer vision:
Data Collection: The first step is to collect raw data in the form of images or videos. This can be done through various sources such as publicly available datasets, web scraping, or data acquisition from hardware devices.
Data Cleaning: The collected data often contains noise, missing values, or inconsistencies that can negatively affect the performance of the model. Hence, data cleaning is performed to remove any such issues and ensure the data is ready for annotation.
Data Annotation: In this step, experts annotate the images with labels to make it easier for the model to learn from the data. Data annotation can be in the form of bounding boxes, polygons, or pixel-level segmentation masks.
Data Augmentation: To increase the diversity of the data and prevent overfitting, data augmentation techniques are applied to the annotated data. These techniques include random cropping, flipping, rotation, and color jittering.
Data Splitting: The annotated data is split into training, validation, and testing sets. The training set is used to train the model, the validation set is used to tune the hyperparameters and prevent overfitting, and the testing set is used to evaluate the final performance of the model.
Model Training: The next step is to train the computer vision model using the annotated and augmented data. This involves selecting an appropriate architecture, loss function, and optimization algorithm, and tuning the hyperparameters to achieve the best performance.
Model Evaluation: Once the model is trained, it is evaluated on the testing set to measure its performance. Metrics such as accuracy, precision, recall, and score are computed to assess the model's performance.
Model Deployment: The final step is to deploy the model in the production environment, where it can be used to solve real-world computer vision problems. This involves integrating the model into the target system and ensuring it can handle new inputs and operate in real time.
TagX Data as a Service
Data as a service (DaaS) refers to the provision of data by a company to other companies. TagX provides DaaS to AI companies by collecting, preparing, and annotating data that can be used to train and test AI models.
Here’s a more detailed explanation of how TagX provides DaaS to AI companies:
Data Collection: TagX collects a wide range of data from various sources such as public data sets, proprietary data, and third-party providers. This data includes image, video, text, and audio data that can be used to train AI models for various use cases.
Data Preparation: Once the data is collected, TagX prepares the data for use in AI models by cleaning, normalizing, and formatting the data. This ensures that the data is in a format that can be easily used by AI models.
Data Annotation: TagX uses a team of annotators to label and tag the data, identifying specific attributes and features that will be used by the AI models. This includes image annotation, video annotation, text annotation, and audio annotation. This step is crucial for the training of AI models, as the models learn from the labeled data.
Data Governance: TagX ensures that the data is properly managed and governed, including data privacy and security. We follow data governance best practices and regulations to ensure that the data provided is trustworthy and compliant with regulations.
Data Monitoring: TagX continuously monitors the data and updates it as needed to ensure that it is relevant and up-to-date. This helps to ensure that the AI models trained using our data are accurate and reliable.
By providing data as a service, TagX makes it easy for AI companies to access high-quality, relevant data that can be used to train and test AI models. This helps AI companies to improve the speed, quality, and reliability of their models, and reduce the time and cost of developing AI systems. Additionally, by providing data that is properly annotated and managed, the AI models developed can be exp
2 notes
·
View notes
Text
The Power of AI and Human Collaboration in Media Content Analysis
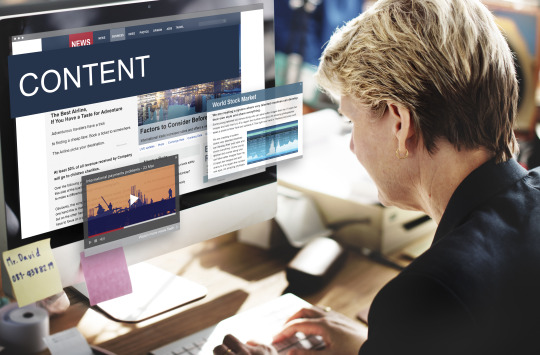
In today’s world binge watching has become a way of life not just for Gen-Z but also for many baby boomers. Viewers are watching more content than ever. In particular, Over-The-Top (OTT) and Video-On-Demand (VOD) platforms provide a rich selection of content choices anytime, anywhere, and on any screen. With proliferating content volumes, media companies are facing challenges in preparing and managing their content. This is crucial to provide a high-quality viewing experience and better monetizing content.
Some of the use cases involved are,
Finding opening of credits, Intro start, Intro end, recap start, recap end and other video segments
Choosing the right spots to insert advertisements to ensure logical pause for users
Creating automated personalized trailers by getting interesting themes from videos
Identify audio and video synchronization issues
While these approaches were traditionally handled by large teams of trained human workforces, many AI based approaches have evolved such as Amazon Rekognition’s video segmentation API. AI models are getting better at addressing above mentioned use cases, but they are typically pre-trained on a different type of content and may not be accurate for your content library. So, what if we use AI enabled human in the loop approach to reduce cost and improve accuracy of video segmentation tasks.
In our approach, the AI based APIs can provide weaker labels to detect video segments and send for review to be trained human reviewers for creating picture perfect segments. The approach tremendously improves your media content understanding and helps generate ground truth to fine-tune AI models. Below is workflow of end-2-end solution,
Raw media content is uploaded to Amazon S3 cloud storage. The content may need to be preprocessed or transcoded to make it suitable for streaming platform (e.g convert to .mp4, upsample or downsample)
AWS Elemental MediaConvert transcodes file-based content into live stream assets quickly and reliably. Convert content libraries of any size for broadcast and streaming. Media files are transcoded to .mp4 format
Amazon Rekognition Video provides an API that identifies useful segments of video, such as black frames and end credits.
Objectways has developed a Video segmentation annotator custom workflow with SageMaker Ground Truth labeling service that can ingest labels from Amazon Rekognition. Optionally, you can skip step#3 if you want to create your own labels for training custom ML model or applying directly to your content.
The content may have privacy and digitial rights management requirements and protection. The Objectway’s Video Segmentaton tool also supports Digital Rights Management provider integration to ensure only authorized analyst can look at the content. Moreover, the content analysts operate out of SOC2 TYPE2 compliant facilities where no downloads or screen capture are allowed.
The media analysts at Objectways’ are experts in content understanding and video segmentation labeling for a variety of use cases. Depending on your accuracy requirements, each video can be reviewed or annotated by two independent analysts and segment time codes difference thresholds are used for weeding out human bias (e.g., out of consensus if time code differs by 5 milliseconds). The out of consensus labels can be adjudicated by senior quality analyst to provide higher quality guarantees.
The Objectways Media analyst team provides throughput and quality gurantees and continues to deliver daily throughtput depending on your business needs. The segmented content labels are then saved to Amazon S3 as JSON manifest format and can be directly ingested into your Media streaming platform.
Conclusion
Artificial intelligence (AI) has become ubiquitous in Media and Entertainment to improve content understanding to increase user engagement and also drive ad revenue. The AI enabled Human in the loop approach outlined is best of breed solution to reduce the human cost and provide highest quality. The approach can be also extended to other use cases such as content moderation, ad placement and personalized trailer generation.
Contact [email protected] for more information.
2 notes
·
View notes
Text
Data labeling and annotation
Boost your AI and machine learning models with professional data labeling and annotation services. Accurate and high-quality annotations enhance model performance by providing reliable training data. Whether for image, text, or video, our data labeling ensures precise categorization and tagging, accelerating AI development. Outsource your annotation tasks to save time, reduce costs, and scale efficiently. Choose expert data labeling and annotation solutions to drive smarter automation and better decision-making. Ideal for startups, enterprises, and research institutions alike.
#artificial intelligence#ai prompts#data analytics#datascience#data annotation#ai agency#ai & machine learning#aws
0 notes
Text
Data Annotation: The Overlooked Pillar of AI and Machine Learning
Introduction
In the ever-evolving landscape of artificial intelligence (AI) and machine learning (ML), remarkable innovations frequently capture public attention. Yet, a vital and often neglected component underpins every effective AI model: data annotation. The absence of precise and thoroughly annotated data renders even the most advanced algorithms incapable of producing dependable outcomes. This article delves into the significance of data annotation as the overlooked pillar of AI and machine learning, highlighting its role in fostering innovation.
Defining Data Annotation
Data Annotation refers to the systematic process of labeling or tagging data to enhance its interpretability for machine learning algorithms. It serves as a conduit between unprocessed data and actionable insights, empowering models to identify patterns, generate predictions, and execute tasks with precision. Annotation encompasses a variety of data types, such as:
Text: Including sentiment tagging, entity recognition, and keyword labeling.
Images: Encompassing object detection, segmentation, and classification.
Audio: Covering transcription, speaker identification, and sound labeling.
Video: Involving frame-by-frame labeling and activity recognition.
The Significance of Data Annotation
Machine learning models derive their learning from examples. Data annotation supplies these examples by emphasizing the features and patterns that the model should prioritize. The quality of the annotation has a direct correlation with the efficacy of AI systems, affecting:
Accuracy: High-quality annotations enhance the precision of model predictions.
Scalability: Well-annotated datasets allow models to adapt to new situations effectively.
Efficiency: Properly executed annotation minimizes the time needed for training and refining models.
Categories of Data Annotation
Text Annotation
Text annotation consists of tagging words, phrases, or sentences to identify:
Named entities (such as individuals, organizations, and locations)
Sentiments (positive, negative, or neutral)
Intent (including commands, inquiries, and statements)
Image Annotation
Image annotation involves labeling various components within an image for purposes such as:
Object detection (utilizing bounding boxes)
Semantic segmentation (performing pixel-level annotations)
Keypoint detection (identifying facial landmarks and estimating poses)
Audio Annotation
Audio annotation encompasses:
Transcribing verbal communication
Recognizing different speakers
Classifying sound types (for instance, music or background noise)
Video Annotation
In the context of video data, annotations may include:
Action recognition
Frame classification
Temporal segmentation
Challenges in Data Annotation
Scalability
Annotating large datasets demands considerable time and resources. Effectively managing this scale while maintaining quality presents a significant challenge.
Consistency
It is essential to maintain uniformity in annotations across various annotators and datasets to ensure optimal model performance.
Domain Expertise
Certain datasets, such as those involving medical imagery or legal documentation, necessitate specialized knowledge for precise annotation.
Cost and Time
Producing high-quality annotations can be resource-intensive, representing a substantial investment in the development of artificial intelligence.
Best Practices for Data Annotation
Establish Clear Guidelines
Create comprehensive annotation standards and provide explicit instructions to annotators to maintain uniformity.
Utilize Appropriate Tools
Employ data annotation platforms and software such as Labelbox, Amazon SageMaker Ground Truth, or GTS.AI’s tailored solutions to enhance workflow efficiency.
Implement Quality Assurance
Introduce validation procedures to regularly assess and improve annotations.
Automate Where Feasible
Integrate manual annotation with automated solutions to expedite the process while maintaining high quality.
Prioritize Training
Offer training programs for annotators, particularly for intricate tasks that necessitate specialized knowledge.
How GTS.AI Can Assist
At GTS.AI, we recognize the essential role of data annotation in the success of AI initiatives. Our skilled team delivers comprehensive annotation services customized to meet the specific requirements of your project. From scalable solutions to specialized domain knowledge, we ensure your datasets are optimized for excellence. Utilizing advanced tools and stringent quality assurance measures, GTS.AI enables your models to achieve exceptional accuracy.
Conclusion
Although algorithms and technologies often dominate discussions, data annotation is the fundamental element that drives advancements in AI and machine learning. By committing to high-quality annotation, organizations can fully harness the potential of their AI systems and provide transformative solutions. Whether you are embarking on your AI journey or seeking to enhance your current models, GTS.AI stands ready to be your reliable partner in data annotation.
1 note
·
View note
Text

How Video Transcription Services Improve AI Training Through Annotated Datasets
Video transcription services play a crucial role in AI training by converting raw video data into structured, annotated datasets, enhancing the accuracy and performance of machine learning models.
#video transcription services#aitraining#Annotated Datasets#machine learning#ultimate sex machine#Data Collection for AI#AI Data Solutions#Video Data Annotation#Improving AI Accuracy
0 notes
Text

0 notes
Text
Generative AI | High-Quality Human Expert Labeling | Apex Data Sciences
Apex Data Sciences combines cutting-edge generative AI with RLHF for superior data labeling solutions. Get high-quality labeled data for your AI projects.
#GenerativeAI#AIDataLabeling#HumanExpertLabeling#High-Quality Data Labeling#Apex Data Sciences#Machine Learning Data Annotation#AI Training Data#Data Labeling Services#Expert Data Annotation#Quality AI Data#Generative AI Data Labeling Services#High-Quality Human Expert Data Labeling#Best AI Data Annotation Companies#Reliable Data Labeling for Machine Learning#AI Training Data Labeling Experts#Accurate Data Labeling for AI#Professional Data Annotation Services#Custom Data Labeling Solutions#Data Labeling for AI and ML#Apex Data Sciences Labeling Services
1 note
·
View note
Text
Top 7 Data Labeling Challenges and Their Solution
Addressing data labeling challenges is crucial for Machine Learning success. This content explores the top seven issues faced in data labeling and provides effective solutions. From ambiguous labels to scalability concerns, discover insights to enhance the accuracy and efficiency of your labeled datasets, fostering better AI model training. Read the article:…
View On WordPress
#data annotation#data annotation companies#data annotation company#data annotation for AI/ML#Data Annotation in Machine Learning#data labeling
0 notes
Text
Empowering Machine Learning Algorithms with Data Annotation Services
Given the costly and time-intensive aspects of data annotation, collaborating with professional providers like Damco is the best way out. By availing of data annotation services for Machine Learning and Artificial Intelligence. you can relieve yourself from the burden associated with this high-risk decision and simultaneously economize both time and financial resources.

#Data Annotation Services#data annotation company#data annotation#data annotation for ml#data annotation in machine learning
0 notes
Text
Unlock the potential of your AI projects with high-quality data. Our comprehensive data solutions provide the accuracy, relevance, and depth needed to propel your AI forward. Access meticulously curated datasets, real-time data streams, and advanced analytics to enhance machine learning models and drive innovation. Whether you’re developing cutting-edge applications or refining existing systems, our data empowers you to achieve superior results. Stay ahead of the competition with the insights and precision that only top-tier data can offer. Transform your AI initiatives and unlock new possibilities with our premium data annotation services. Propel your AI to new heights today.
0 notes
Text
#machine learning#Data Labeling#cogito ai#ai data annotation#V7 Darwin#Labelbox#Scale AI#Dataloop#SuperAnnotate
0 notes
Text
#across the spiderverse#https://24x7offshoring.com/machine-learning/data-collection/#https://24x7offshoring.com/localization/multimedia-localization/transcription/#https://24x7offshoring.com/localization/translation/media-translation/#https://24x7offshoring.com/localization/translation/#https://24x7offshoring.com/transliteration-english/#https://24x7offshoring.com/translator-english-to-kannada/#https://24x7offshoring.com/localization/#https://24x7offshoring.com/english-to-gujarati-translation/#http://24x7offshoring.com/bengali-to-english-translators/#https://24x7offshoring.com/machine-learning/annotation/#https://24x7offshoring.com/localization/interpretation/#https://24x7offshoring.com/localization/multimedia-localization/
1 note
·
View note
Text
ML Datasets Demystified: Types, Challenges, and Best Practices
Introduction:
Machine Learning (ML) has transformed various sectors, fostering advancements in healthcare, finance, entertainment, and more. Central to the success of any ML model is a vital element: datasets. A comprehensive understanding of the different types, challenges, and best practices related to ML Datasets is crucial for developing robust and effective models. Let us delve into the intricacies of ML datasets and examine how to optimize their potential.
Classification of ML Datasets
Datasets can be classified according to the nature of the data they encompass and their function within the ML workflow. The main categories are as follows:
Structured vs. Unstructured Datasets
Structured Data: This category consists of data that is well-organized and easily searchable, typically arranged in rows and columns within relational databases. Examples include spreadsheets containing customer information, sales data, and sensor outputs.
Unstructured Data: In contrast, unstructured data does not adhere to a specific format and encompasses images, videos, audio recordings, and text. Examples include photographs shared on social media platforms or customer feedback.
2. Labeled vs. Unlabeled Datasets
Labeled Data: This type of dataset includes data points that are accompanied by specific labels or outputs. Labeled data is crucial for supervised learning tasks, including classification and regression. An example would be an image dataset where each image is tagged with the corresponding object it depicts.
Unlabeled Data: In contrast, unlabeled datasets consist of raw data that lacks predefined labels. These datasets are typically utilized in unsupervised learning or semi-supervised learning tasks, such as clustering or detecting anomalies.
3. Domain-Specific Datasets
Datasets can also be classified according to their specific domain or application. Examples include:
Medical Datasets: These are utilized in healthcare settings, encompassing items such as CT scans or patient medical records.
Financial Datasets: This category includes stock prices, transaction logs, and various economic indicators.
Text Datasets: These consist of collections of documents, chat logs, or social media interactions, which are employed in natural language processing (NLP).
4. Static vs. Streaming Datasets
Static Datasets: These datasets are fixed and collected at a particular moment in time, remaining unchanged thereafter. Examples include historical weather data or previous sales records.
Streaming Datasets: This type of data is generated continuously in real-time, such as live sensor outputs, social media updates, or network activity logs.
Challenges Associated with Machine Learning Datasets
Data Quality Concerns
Inadequate data quality, characterized by missing entries, duplicate records, or inconsistent formatting, can result in erroneous predictions from models. It is essential to undertake data cleaning as a critical measure to rectify these problems.
2. Data Bias
Data bias occurs when certain demographics or patterns are either underrepresented or overrepresented within a dataset. This imbalance can lead to biased or discriminatory results in machine learning models. For example, a facial recognition system trained on a non-diverse dataset may struggle to accurately recognize individuals from various demographic groups.
3. Imbalanced Datasets
An imbalanced dataset features an unequal distribution of classes. For instance, in a fraud detection scenario, a dataset may consist of 95% legitimate transactions and only 5% fraudulent ones. Such disparities can distort the predictions made by the model.
4. Data Volume and Scalability
Extensive datasets can create challenges related to storage and processing capabilities. High-dimensional data, frequently encountered in domains such as genomics or image analysis, requires substantial computational power and effective algorithms to manage.
5. Privacy and Ethical Considerations
Datasets frequently include sensitive information, including personal and financial data. It is imperative to maintain data privacy and adhere to regulations such as GDPR or CCPA. Additionally, ethical implications must be considered, particularly in contexts like facial recognition and surveillance.
Best Practices for Working with Machine Learning Datasets
Define the Problem Statement
It is essential to articulate the specific problem that your machine learning model intends to address. This clarity will guide you in selecting or gathering appropriate datasets. For example, if the objective is to perform sentiment analysis, it is crucial to utilize text datasets that contain labeled sentiments.
2. Data Preprocessing
Address Missing Data: Implement strategies such as imputation or removal to fill in gaps within the dataset.
Normalize and Scale Data: Ensure that numerical features are standardized to a similar range, which can enhance the performance of the model.
Feature Engineering: Identify and extract significant features that improve the model's capacity to recognize patterns.
3. Promote Data Diversity
Incorporate a wide range of representative samples to mitigate bias. When gathering data, take into account variations in demographics, geography, and time.
4. Implement Effective Data Splitting
Segment datasets into training, validation, and test sets. A typical distribution is 70-20-10, which allows the model to be trained, fine-tuned, and evaluated on separate subsets, thereby reducing the risk of overfitting.
5. Enhance Data through Augmentation
Utilize data augmentation methods, such as flipping, rotating, or scaling images, to expand the size and diversity of the dataset without the need for additional data collection.
6. Utilize Open Datasets Judiciously
Make use of publicly accessible datasets such as ImageNet, UCI Machine Learning Repository, or Kaggle datasets. These resources offer extensive data for various machine learning applications, but it is important to ensure they are relevant to your specific problem statement.
7. Maintain Documentation and Version Control
Keep thorough documentation regarding the sources of datasets, preprocessing procedures, and any updates made. Implementing version control is vital for tracking changes and ensuring reproducibility.
8. Conduct Comprehensive Validation and Testing of Models
It is essential to validate your model using a variety of test sets to confirm its reliability. Employing cross-validation methods can offer valuable insights into the model's ability to generalize.
Conclusion
Machine learning datasets serve as the cornerstone for effective machine learning models. By comprehending the various types of datasets, tackling associated challenges, and implementing best practices, practitioners can develop models that are precise, equitable, and scalable. As the field of machine learning progresses, so too will the methodologies for managing and enhancing datasets. Remaining informed and proactive is crucial for realizing the full potential of data within the realms of artificial intelligence and machine learning.
Machine learning datasets are the foundation of successful AI systems, and understanding their types, challenges, and best practices is crucial. Experts from Globose Technology Solutions highlight that selecting the right dataset, ensuring data quality, and addressing biases are vital steps for robust model performance. Leveraging diverse datasets while adhering to ethical considerations ensures fairness and generalizability. By adopting systematic data preparation, validation techniques, and domain-specific expertise, practitioners can unlock the true potential of ML applications.
0 notes