#data annotation for AI/ML
Explore tagged Tumblr posts
Text
Betting Big on Data Annotation Companies: Building the Future of AI Models
Explore the pivotal role of data annotation companies in shaping the future of AI models. Delve into the strategies, innovations, and market dynamics driving these companies to the forefront of the AI revolution. Gain insights into the profound impact of meticulous data labeling on the efficacy of Machine Learning algorithms. Read the blog:âŚ
View On WordPress
#data annotation#data annotation companies#data annotation company#data annotation for AI/ML#Data Annotation in Machine Learning
0 notes
Text
Generative AI | High-Quality Human Expert Labeling | Apex Data Sciences
Apex Data Sciences combines cutting-edge generative AI with RLHF for superior data labeling solutions. Get high-quality labeled data for your AI projects.
#GenerativeAI#AIDataLabeling#HumanExpertLabeling#High-Quality Data Labeling#Apex Data Sciences#Machine Learning Data Annotation#AI Training Data#Data Labeling Services#Expert Data Annotation#Quality AI Data#Generative AI Data Labeling Services#High-Quality Human Expert Data Labeling#Best AI Data Annotation Companies#Reliable Data Labeling for Machine Learning#AI Training Data Labeling Experts#Accurate Data Labeling for AI#Professional Data Annotation Services#Custom Data Labeling Solutions#Data Labeling for AI and ML#Apex Data Sciences Labeling Services
1 note
¡
View note
Text
Medical Datasets represent the cornerstone of healthcare innovation in the AI era. Through careful analysis and interpretation, these datasets empower healthcare professionals to deliver more accurate diagnoses, personalized treatments, and proactive interventions. At Globose Technology Solutions, we are committed to harnessing the transformative power of medical datasets, pushing the boundaries of healthcare excellence, and ushering in a future where every patient will receive the care they deserve.
#Medical Datasets#Healthcare datasets#Healthcare AI Data Collection#Data Collection in Machine Learning#data collection company#datasets#data collection#globose technology solutions#ai#technology#data annotation for ml
0 notes
Text
Decoding the Power of Speech: A Deep Dive into Speech Data Annotation
Introduction
In the realm of artificial intelligence (AI) and machine learning (ML), the importance of high-quality labeled data cannot be overstated. Speech data, in particular, plays a pivotal role in advancing various applications such as speech recognition, natural language processing, and virtual assistants. The process of enriching raw audio with annotations, known as speech data annotation, is a critical step in training robust and accurate models. In this in-depth blog, we'll delve into the intricacies of speech data annotation, exploring its significance, methods, challenges, and emerging trends.
The Significance of Speech Data Annotation
1. Training Ground for Speech Recognition: Speech data annotation serves as the foundation for training speech recognition models. Accurate annotations help algorithms understand and transcribe spoken language effectively.
2. Natural Language Processing (NLP) Advancements: Annotated speech data contributes to the development of sophisticated NLP models, enabling machines to comprehend and respond to human language nuances.
3. Virtual Assistants and Voice-Activated Systems: Applications like virtual assistants heavily rely on annotated speech data to provide seamless interactions, and understanding user commands and queries accurately.
Methods of Speech Data Annotation
1. Phonetic Annotation: Phonetic annotation involves marking the phonemes or smallest units of sound in a given language. This method is fundamental for training speech recognition systems.
2. Transcription: Transcription involves converting spoken words into written text. Transcribed data is commonly used for training models in natural language understanding and processing.
3. Emotion and Sentiment Annotation: Beyond words, annotating speech for emotions and sentiments is crucial for applications like sentiment analysis and emotionally aware virtual assistants.
4. Speaker Diarization: Speaker diarization involves labeling different speakers in an audio recording. This is essential for applications where distinguishing between multiple speakers is crucial, such as meeting transcription.
Challenges in Speech Data Annotation
1. Accurate Annotation: Ensuring accuracy in annotations is a major challenge. Human annotators must be well-trained and consistent to avoid introducing errors into the dataset.
2. Diverse Accents and Dialects: Speech data can vary significantly in terms of accents and dialects. Annotating diverse linguistic nuances poses challenges in creating a comprehensive and representative dataset.
3. Subjectivity in Emotion Annotation: Emotion annotation is subjective and can vary between annotators. Developing standardized guidelines and training annotators for emotional context becomes imperative.
Emerging Trends in Speech Data Annotation
1. Transfer Learning for Speech Annotation: Transfer learning techniques are increasingly being applied to speech data annotation, leveraging pre-trained models to improve efficiency and reduce the need for extensive labeled data.
2. Multimodal Annotation: Integrating speech data annotation with other modalities such as video and text is becoming more common, allowing for a richer understanding of context and meaning.
3. Crowdsourcing and Collaborative Annotation Platforms: Crowdsourcing platforms and collaborative annotation tools are gaining popularity, enabling the collective efforts of annotators worldwide to annotate large datasets efficiently.
Wrapping it up!
In conclusion, speech data annotation is a cornerstone in the development of advanced AI and ML models, particularly in the domain of speech recognition and natural language understanding. The ongoing challenges in accuracy, diversity, and subjectivity necessitate continuous research and innovation in annotation methodologies. As technology evolves, so too will the methods and tools used in speech data annotation, paving the way for more accurate, efficient, and context-aware AI applications.
At ProtoTech Solutions, we offer cutting-edge Data Annotation Services, leveraging expertise to annotate diverse datasets for AI/ML training. Their precise annotations enhance model accuracy, enabling businesses to unlock the full potential of machine-learning applications. Trust ProtoTech for meticulous data labeling and accelerated AI innovation.
#speech data annotation#Speech data#artificial intelligence (AI)#machine learning (ML)#speech#Data Annotation Services#labeling services for ml#ai/ml annotation#annotation solution for ml#data annotation machine learning services#data annotation services for ml#data annotation and labeling services#data annotation services for machine learning#ai data labeling solution provider#ai annotation and data labelling services#data labelling#ai data labeling#ai data annotation
0 notes
Text
đ Ensure Accuracy in Labeling With AI Data Annotation Services
đŚ The demand for speed in data labeling annotation has reached unprecedented levels. Damco integrates predictive and automated AI data annotation with the expertise of world-class annotators and subject matter specialists to provide the training sets required for rapid production. All annotation services work is turned around rapidly by a highly qualified team of subject matter experts.
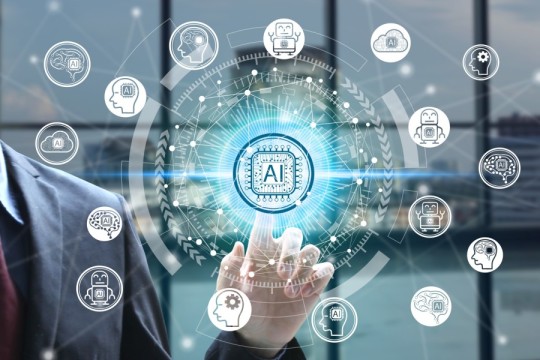
#AI data annotation#data annotation in machine learning#data annotation for ml#data annotation company#data annotation#data annotation services
0 notes
Text
Best data extraction services in USA
In today's fiercely competitive business landscape, the strategic selection of a web data extraction services provider becomes crucial. Outsource Bigdata stands out by offering access to high-quality data through a meticulously crafted automated, AI-augmented process designed to extract valuable insights from websites. Our team ensures data precision and reliability, facilitating decision-making processes.
For more details, visit: https://outsourcebigdata.com/data-automation/web-scraping-services/web-data-extraction-services/.
About AIMLEAP
Outsource Bigdata is a division of Aimleap. AIMLEAP is an ISO 9001:2015 and ISO/IEC 27001:2013 certified global technology consulting and service provider offering AI-augmented Data Solutions, Data Engineering, Automation, IT Services, and Digital Marketing Services. AIMLEAP has been recognized as a âGreat Place to WorkÂŽâ.
With a special focus on AI and automation, we built quite a few AI & ML solutions, AI-driven web scraping solutions, AI-data Labeling, AI-Data-Hub, and Self-serving BI solutions. We started in 2012 and successfully delivered IT & digital transformation projects, automation-driven data solutions, on-demand data, and digital marketing for more than 750 fast-growing companies in the USA, Europe, New Zealand, Australia, Canada; and more.âŻ
-An ISO 9001:2015 and ISO/IEC 27001:2013 certified⯠-Served 750+ customers⯠-11+ Years of industry experience⯠-98% client retention⯠-Great Place to WorkÂŽ certified⯠-Global delivery centers in the USA, Canada, India & AustraliaâŻ
Our Data Solutions
APISCRAPY: AI driven web scraping & workflow automation platform APISCRAPY is an AI driven web scraping and automation platform that converts any web data into ready-to-use data. The platform is capable to extract data from websites, process data, automate workflows, classify data and integrate ready to consume data into database or deliver data in any desired format.âŻ
AI-Labeler:âŻAI augmented annotation & labeling solution AI-Labeler is an AI augmented data annotation platform that combines the power of artificial intelligence with in-person involvement to label, annotate and classify data, and allowing faster development of robust and accurate models.
AI-Data-Hub: On-demand data for building AI products & services On-demand AI data hub for curated data, pre-annotated data, pre-classified data, and allowing enterprises to obtain easily and efficiently, and exploit high-quality data for training and developing AI models.
PRICESCRAPY: AI enabled real-time pricing solution An AI and automation driven price solution that provides real timeâŻprice monitoring, pricing analytics, and dynamic pricing for companies across theâŻworld.âŻ
APIKART: AI driven data API solution hub⯠APIKART is a data API hub that allows businesses and developers to access and integrate large volume of data from various sources through APIs. It is a data solution hub for accessing data through APIs, allowing companies to leverage data, and integrate APIs into their systems and applications.âŻ
Locations: USA: 1-30235 14656⯠Canada: +1 4378 370 063⯠India: +91 810 527 1615⯠Australia: +61 402 576 615 Email: [email protected]
2 notes
¡
View notes
Text
AI Research Methods: Designing and Evaluating Intelligent Systems

The field of artificial intelligence (AI) is evolving rapidly, and with it, the importance of understanding its core methodologies. Whether you're a beginner in tech or a researcher delving into machine learning, itâs essential to be familiar with the foundational artificial intelligence course subjects that shape the study and application of intelligent systems. These subjects provide the tools, frameworks, and scientific rigor needed to design, develop, and evaluate AI-driven technologies effectively.
What Are AI Research Methods?
AI research methods are the systematic approaches used to investigate and create intelligent systems. These methods allow researchers and developers to model intelligent behavior, simulate reasoning processes, and validate the performance of AI models.
Broadly, AI research spans across several domains, including natural language processing (NLP), computer vision, robotics, expert systems, and neural networks. The aim is not only to make systems smarter but also to ensure they are safe, ethical, and efficient in solving real-world problems.
Core Approaches in AI Research
1. Symbolic (Knowledge-Based) AI
This approach focuses on logic, rules, and knowledge representation. Researchers design systems that mimic human reasoning through formal logic. Expert systems like MYCIN, for example, use a rule-based framework to make medical diagnoses.
Symbolic AI is particularly useful in domains where rules are well-defined. However, it struggles in areas involving uncertainty or massive data inputsâchallenges addressed more effectively by modern statistical methods.
2. Machine Learning
Machine learning (ML) is one of the most active research areas in AI. It involves algorithms that learn from data to make predictions or decisions without being explicitly programmed. Supervised learning, unsupervised learning, and reinforcement learning are key types of ML.
This approach thrives in pattern recognition tasks such as facial recognition, recommendation engines, and speech-to-text applications. It heavily relies on data availability and quality, making dataset design and preprocessing crucial research activities.
3. Neural Networks and Deep Learning
Deep learning uses multi-layered neural networks to model complex patterns and behaviors. Itâs particularly effective for tasks like image recognition, voice synthesis, and language translation.
Research in this area explores architecture design (e.g., convolutional neural networks, transformers), optimization techniques, and scalability for real-world applications. Evaluation often involves benchmarking models on standard datasets and fine-tuning for specific tasks.
4. Evolutionary Algorithms
These methods take inspiration from biological evolution. Algorithms such as genetic programming or swarm intelligence evolve solutions to problems by selecting the best-performing candidates from a population.
AI researchers apply these techniques in optimization problems, game design, and robotics, where traditional programming struggles to adapt to dynamic environments.
5. Probabilistic Models
When systems must reason under uncertainty, probabilistic methods like Bayesian networks and Markov decision processes offer powerful frameworks. Researchers use these to create models that can weigh risks and make decisions in uncertain conditions, such as medical diagnostics or autonomous driving.
Designing Intelligent Systems
Designing an AI system requires careful consideration of the task, data, and objectives. The process typically includes:
Defining the Problem: What is the task? Classification, regression, decision-making, or language translation?
Choosing the Right Model: Depending on the problem type, researchers select symbolic models, neural networks, or hybrid systems.
Data Collection and Preparation: Good data is essential. Researchers clean, preprocess, and annotate data before feeding it into the model.
Training and Testing: The system learns from training data and is evaluated on unseen test data.
Evaluation Metrics: Accuracy, precision, recall, F1 score, or area under the curve (AUC) are commonly used to assess performance.
Iteration and Optimization: Models are tuned, retrained, and improved over time.
Evaluating AI Systems
Evaluating an AI system goes beyond just checking accuracy. Researchers must also consider:
Robustness: Does the system perform well under changing conditions?
Fairness: Are there biases in the predictions?
Explainability: Can humans understand how the system made a decision?
Efficiency: Does it meet performance standards in real-time settings?
Scalability: Can the system be applied to large-scale environments?
These factors are increasingly important as AI systems are integrated into critical industries like healthcare, finance, and security.
The Ethical Dimension
Modern AI research doesnât operate in a vacuum. With powerful tools comes the responsibility to ensure ethical standards are met. Questions around data privacy, surveillance, algorithmic bias, and AI misuse have become central to contemporary research discussions.
Ethics are now embedded in many artificial intelligence course subjects, prompting students and professionals to consider societal impact alongside technical performance.
Conclusion
AI research methods offer a structured path to innovation, enabling us to build intelligent systems that can perceive, reason, and act. Whether you're designing a chatbot, developing a recommendation engine, or improving healthcare diagnostics, understanding these methods is crucial for success.
By exploring the artificial intelligence course subjects in depth, students and professionals alike gain the knowledge and tools necessary to contribute meaningfully to the future of AI. With a solid foundation, the possibilities are endlessâlimited only by imagination and ethical responsibility.
#ArtificialIntelligence#AI#MachineLearning#DeepLearning#AIResearch#IntelligentSystems#AIEducation#FutureOfAI#AIInnovation#DataScienc
0 notes
Text

Expert Object Detection Annotation Services
Want your computer vision models to truly understand the world around them?
Wisepl deliver high-quality, pixel-perfect object detection annotations to fuel your AI/ML systems with accuracy and consistency.
Bounding Box Annotation
Polygon & Polyline Annotation
Smart Labeling for Custom Objects
Scalable Workforce + Quick Turnarounds
NDAs & Full Data Confidentiality
From autonomous vehicles to retail analytics and agriculture tech - we power the AI behind it all.
Contact us today for a free trial or sample annotation! www.wisepl.com | [email protected]
#DataAnnotation#ObjectDetection#AITrainingData#ComputerVision#MachineLearning#AIData#AnnotationServices#Wisepl#BoundingBox#TechForAI#AIDevelopment#LabelingExperts#SmartData
0 notes
Text
How Data Annotation Tools Are Paving the Way for Advanced AI and Autonomous Systems
The global data annotation tools market size was estimated at USD 1.02 billion in 2023 and is anticipated to grow at a CAGR of 26.3% from 2024 to 2030. The growth is majorly driven by the increasing adoption of image data annotation tools in the automotive, retail, and healthcare sectors. The data annotation tools enable users to enhance the value of data by adding attribute tags to it or labeling it. The key benefit of using annotation tools is that the combination of data attributes enables users to manage the data definition at a single location and eliminates the need to rewrite similar rules in multiple places.
The rise of big data and a surge in the number of large datasets are likely to necessitate the use of artificial intelligence technologies in the field of data annotations. The data annotation industry is also expected to have benefited from the rising demands for improvements in machine learning as well as in the rising investment in advanced autonomous driving technology.
Technologies such as the Internet of Things (IoT), Machine Learning (ML), robotics, advanced predictive analytics, and Artificial Intelligence (AI) generate massive data. With changing technologies, data efficiency proves to be essential for creating new business innovations, infrastructure, and new economics. These factors have significantly contributed to the growth of the industry. Owing to the rising potential of growth in data annotation, companies developing AI-enabled healthcare applications are collaborating with data annotation companies to provide the required data sets that can assist them in enhancing their machine learning and deep learning capabilities.
For instance, in November 2022, Medcase, a developer of healthcare AI solutions, and NTT DATA, formalized a legally binding agreement. Under this partnership, the two companies announced their collaboration to offer data discovery and enrichment solutions for medical imaging. Through this partnership, customers of Medcase will gain access to NTT DATA's Advocate AI services. This access enables innovators to obtain patient studies, including medical imaging, for their projects.
However, the inaccuracy of data annotation tools acts as a restraint to the growth of the market. For instance, a given image may have low resolution and include multiple objects, making it difficult to label. The primary challenge faced by the market is issues related to inaccuracy in the quality of data labeled. In some cases, the data labeled manually may contain erroneous labeling and the time to detect such erroneous labels may vary, which further adds to the cost of the entire annotation process. However, with the development of sophisticated algorithms, the accuracy of automated data annotation tools is improving thus reducing the dependency on manual annotation and the cost of the tools.
Global Data Annotation Tools Market Report Segmentation
Grand View Research has segmented the global data annotation tools market report based on type, annotation type, vertical, and region:
Type Outlook (Revenue, USD Million, 2017 - 2030)
Text
Image/Video
Audio
Annotation Type Outlook (Revenue, USD Million, 2017 - 2030)
Manual
Semi-supervised
Automatic
Vertical Outlook (Revenue, USD Million, 2017 - 2030)
IT
Automotive
Government
Healthcare
Financial Services
Retail
Others
Regional Outlook (Revenue, USD Million, 2017 - 2030)
North America
US
Canada
Mexico
Europe
Germany
UK
France
Asia Pacific
China
Japan
India
South America
Brazil
Middle East and Africa (MEA)
Key Data Annotation Tools Companies:
The following are the leading companies in the data annotation tools market. These companies collectively hold the largest market share and dictate industry trends.
Annotate.com
Appen Limited
CloudApp
Cogito Tech LLC
Deep Systems
Labelbox, Inc
LightTag
Lotus Quality Assurance
Playment Inc
Tagtog Sp. z o.o
CloudFactory Limited
ClickWorker GmbH
Alegion
Figure Eight Inc.
Amazon Mechanical Turk, Inc
Explosion AI GMbH
Mighty AI, Inc.
Trilldata Technologies Pvt Ltd
Scale AI, Inc.
Google LLC
Lionbridge Technologies, Inc
SuperAnnotate LLC
Recent Developments
In November 2023, Appen Limited, a high-quality data provider for the AI lifecycle, chose Amazon Web Services (AWS) as its primary cloud for AI solutions and innovation. As Appen utilizes additional enterprise solutions for AI data source, annotation, and model validation, the firms are expanding their collaboration with a multi-year deal. Appen is strengthening its AI data platform, which serves as the bridge between people and AI, by integrating cutting-edge AWS services.
In September 2023, Labelbox launched Large Language Model (LLM) solution to assist organizations in innovating with generative AI and deepen the partnership with Google Cloud. With the introduction of large language models (LLMs), enterprises now have a plethora of chances to generate new competitive advantages and commercial value. LLM systems have the ability to revolutionize a wide range of intelligent applications; nevertheless, in many cases, organizations will need to adjust or finetune LLMs in order to align with human preferences. Labelbox, as part of an expanded cooperation, is leveraging Google Cloud's generative AI capabilities to assist organizations in developing LLM solutions with Vertex AI. Labelbox's AI platform will be integrated with Google Cloud's leading AI and Data Cloud tools, including Vertex AI and Google Cloud's Model Garden repository, allowing ML teams to access cutting-edge machine learning (ML) models for vision and natural language processing (NLP) and automate key workflows.
In March 2023, has released the most recent version of Enlitic Curie, a platform aimed at improving radiology department workflow. This platform includes Curie|ENDEX, which uses natural language processing and computer vision to analyze and process medical images, and Curie|ENCOG, which uses artificial intelligence to detect and protect medical images in Health Information Security.
In November 2022, Appen Limited, a global leader in data for the AI Lifecycle, announced its partnership with CLEAR Global, a nonprofit organization dedicated to ensuring access to essential information and amplifying voices across languages. This collaboration aims to develop a speech-based healthcare FAQ bot tailored for Sheng, a Nairobi slang language.
 Order a free sample PDF of the Market Intelligence Study, published by Grand View Research.
0 notes
Text
The Data Collection And Labeling Market was valued at USD 3.0 Billion in 2023 and is expected to reach USD 29.2 Billion by 2032, growing at a CAGR of 28.54% from 2024-2032.
The data collection and labeling market is witnessing transformative growth as artificial intelligence (AI), machine learning (ML), and deep learning applications continue to expand across industries. As organizations strive to unlock the value of big data, the demand for accurately labeled datasets has surged, making data annotation a critical component in developing intelligent systems. Companies in sectors such as healthcare, automotive, retail, and finance are investing heavily in curated data pipelines that drive smarter algorithms, more efficient automation, and personalized customer experiences.
Data Collection and Labeling Market Fueled by innovation and technological advancement, the data collection and labeling market is evolving to meet the growing complexities of AI models. Enterprises increasingly seek comprehensive data solutionsâranging from image, text, audio, and video annotation to real-time sensor and geospatial data labelingâto power mission-critical applications. Human-in-the-loop systems, crowdsourcing platforms, and AI-assisted labeling tools are at the forefront of this evolution, ensuring the creation of high-quality training datasets that minimize bias and improve predictive performance.
Get Sample Copy of This Report:Â https://www.snsinsider.com/sample-request/5925Â
Market Keyplayers:
Scale AIÂ â Scale Data Engine
Appen â Appen Data Annotation Platform
Labelbox â Labelbox AI Annotation Platform
Amazon Web Services (AWS)Â â Amazon SageMaker Ground Truth
Google â Google Cloud AutoML Data Labeling Service
IBMÂ â IBM Watson Data Annotation
Microsoft â Azure Machine Learning Data Labeling
Playment (by TELUS International AI)Â â Playment Annotation Platform
Hive AIÂ â Hive Data Labeling Platform
Samasource â Sama AI Data Annotation
CloudFactory â CloudFactory Data Labeling Services
SuperAnnotate â SuperAnnotate AI Annotation Tool
iMerit â iMerit Data Enrichment Services
Figure Eight (by Appen)Â â Figure Eight Data Labeling
Cogito Tech â Cogito Data Annotation Services
Market Analysis The market's growth is driven by the convergence of AI deployment and the increasing demand for labeled data to support supervised learning models. Startups and tech giants alike are intensifying their focus on data preparation workflows. Strategic partnerships and outsourcing to data labeling service providers have become common approaches to manage scalability and reduce costs. The competitive landscape features a mix of established players and emerging platforms offering specialized labeling services and tools, creating a highly dynamic ecosystem.
Market Trends
Increasing adoption of AI and ML across diverse sectors
Rising preference for cloud-based data annotation tools
Surge in demand for multilingual and cross-domain data labeling
Expansion of video and 3D image annotation for autonomous systems
Growing emphasis on ethical AI and reduction of labeling bias
Integration of AI-assisted labeling to accelerate workflows
Outsourcing of labeling processes to specialized firms for scalability
Enhanced use of synthetic data for model training and validation
Market Scope The data collection and labeling market serves as the foundation for AI applications across verticals. From autonomous vehicles requiring high-accuracy image labeling to chatbots trained on annotated customer interactions, the scope encompasses every industry where intelligent automation is pursued. As AI maturity increases, the need for diverse, structured, and domain-specific datasets will further elevate the relevance of comprehensive labeling solutions.
Market Forecast The market is expected to maintain strong momentum, driven by increasing digital transformation initiatives and investment in smart technologies. Continuous innovation in labeling techniques, enhanced platform capabilities, and regulatory compliance for data privacy are expected to shape the future landscape. Organizations will prioritize scalable, accurate, and cost-efficient data annotation solutions to stay competitive in an AI-driven economy. The role of data labeling is poised to shift from a support function to a strategic imperative.
Access Complete Report:Â https://www.snsinsider.com/reports/data-collection-and-labeling-market-5925Â
Conclusion The data collection and labeling market is not just a stepping stone in the AI journeyâit is becoming a strategic cornerstone that determines the success of intelligent systems. As enterprises aim to harness the full potential of AI, the quality, variety, and scalability of labeled data will define the competitive edge. Those who invest early in refined data pipelines and ethical labeling practices will lead in innovation, relevance, and customer trust in the evolving digital world.
About Us:
SNS Insider is one of the leading market research and consulting agencies that dominates the market research industry globally. Our company's aim is to give clients the knowledge they require in order to function in changing circumstances. In order to give you current, accurate market data, consumer insights, and opinions so that you can make decisions with confidence, we employ a variety of techniques, including surveys, video talks, and focus groups around the world.
Contact Us:
Jagney Dave - Vice President of Client Engagement
Phone: +1-315 636 4242 (US) | +44- 20 3290 5010 (UK)
0 notes
Text
Boost AI ML Accuracy and Efficiency: Utilize Data Labeling Solutions by EnFuse

Improve AI ML outcomes with EnFuse Solutionsâ comprehensive data labeling services. From images and text to audio and video, they deliver accurate, consistent annotations for reliable model training. EnFuseâs scalable services streamline your workflow and empower faster, smarter decisions powered by clean, structured data.
Visit here to see how EnFuse Solutions empowers AI and ML models with expert data labeling services: https://www.enfuse-solutions.com/services/ai-ml-enablement/labeling-curation/
#DataLabeling#DataLabelingServices#DataCurationServices#ImageLabeling#AudioLabeling#VideoLabeling#TextLabeling#DataLabelingCompaniesIndia#DataLabelingAndAnnotation#AnnotationServices#EnFuseSolutions#EnFuseSolutionsIndia
0 notes
Text
Top 7 Data Labeling Challenges and Their Solution
Addressing data labeling challenges is crucial for Machine Learning success. This content explores the top seven issues faced in data labeling and provides effective solutions. From ambiguous labels to scalability concerns, discover insights to enhance the accuracy and efficiency of your labeled datasets, fostering better AI model training. Read the article:âŚ
View On WordPress
#data annotation#data annotation companies#data annotation company#data annotation for AI/ML#Data Annotation in Machine Learning#data labeling
0 notes
Text
Top Video Data Collection Services for AI and Machine Learning
Introduction
In the contemporary landscape dominated by artificial intelligence, video data is essential for the training and enhancement of machine learning models, particularly in fields such as computer vision, autonomous systems, surveillance, and retail analytics. However, obtaining high-quality video data is not a spontaneous occurrence; it necessitates meticulous planning, collection, and annotation. This is where specialized Video Data Collection Services become crucial. In this article, we will examine the characteristics that define an effective video data collection service and showcase how companies like GTS.AI are establishing new benchmarks in this industry.
Why Video Data Is Crucial for AI Models
Video data provides comprehensive and dynamic insights that surpass the capabilities of static images or text. It aids machine learning models in recognizing movement and patterns in real time, understanding object behavior and interactions, and enhancing temporal decision-making in complex environments. Video datasets are essential for various real-world AI applications, including the training of self-driving vehicles, the advancement of smart surveillance systems, and the improvement of gesture recognition in augmented and virtual reality.
What to Look for in a Video Data Collection Service
When assessing a service provider for the collection of video datasets, it is essential to take into account the following critical factors:
Varied Environmental Capture
Your models must be able to generalize across different lighting conditions, geographical locations, weather variations, and more. The most reputable providers offer global crowd-sourced collection or customized video capture designed for specific environments.
2. High-Quality, Real-Time Capture
Quality is paramount. Seek services that provide 4K or HD capture, high frame rates, and various camera angles to replicate real-world situations.
3. Privacy Compliance
In light of the growing number of regulations such as GDPR and HIPAA, it is imperative to implement measures for face and license plate blurring, consent tracking, and secure data management.
Annotation and Metadata
Raw footage alone is insufficient. The most reputable providers offer annotated datasets that include bounding boxes, object tracking, activity tagging, and additional features necessary for training supervised learning models.
Scalability
Regardless of whether your requirement is for 100 or 100,000 videos, the provider must possess the capability to scale efficiently without sacrificing quality.
GTS.AI: A Leader in Video Data Collection Services
At GTS.AI, we focus on delivering tailored, scalable, and premium video dataset solutions for AI and ML teams across various sectors.
What Sets GTS.AI Apart?
Our unique advantages include a global reach through a crowdsource network in over 100 countries, enabling diverse data collection.
We offer flexible video types, accommodating indoor retail and outdoor traffic scenarios with scripted, semi-scripted, and natural video capture tailored to client needs.
Our compliance-first approach ensures data privacy through anonymization techniques and adherence to regulations.
Additionally, we provide an end-to-end workflow that includes comprehensive video annotation services such as frame-by-frame labeling, object tracking, and scene segmentation.
For those requiring quick access to data, our systems are designed for rapid deployment and delivery while maintaining high quality.
Use Cases We Support
Autonomous Driving and Advanced Driver Assistance Systems,
Smart Surveillance and Security Analytics,
Retail Behavior Analysis,
Healthcare Monitoring such as Patient Movement Tracking,
Robotics and Human Interaction,
Gesture and Action Recognition.
Ready to Power Your AI Model with High-Quality Video Data?
Regardless of whether you are developing next-generation autonomous systems or creating advanced security solutions, Globose Technology Solution .AI's video data collection services can provide precisely what you requireâefficiently, rapidly, and with accuracy.
0 notes
Text
AI is playing a crucial role in healthcare innovation by leveraging medical datasets. At Globose Technology Solutions, we're committed to addressing challenges, embracing ethics, and collaborating with healthcare stakeholders to reshape the future of healthcare. Our AI solutions aim to improve patient outcomes and create a sustainable healthcare ecosystem. Visit GTS Healthcare for more insights.
#Medical Dataset#medical practices#healthcare#Medical Datasets in AI#Data collection Services#data collection company#technology#dataset#data collection#globose technology solutions#ai#data annotation for ml#video annotation#datasets
0 notes
Text
Using AI to Predict a Blockbuster Movie
New Post has been published on https://thedigitalinsider.com/using-ai-to-predict-a-blockbuster-movie/
Using AI to Predict a Blockbuster Movie
Although film and television are often seen as creative and open-ended industries, they have long been risk-averse. High production costs (which may soon lose the offsetting advantage of cheaper overseas locations, at least for US projects) and a fragmented production landscape make it difficult for independent companies to absorb a significant loss.
Therefore, over the past decade, the industry has taken a growing interest in whether machine learning can detect trends or patterns in how audiences respond to proposed film and television projects.
The main data sources remain the Nielsen system (which offers scale, though its roots lie in TV and advertising) and sample-based methods such as focus groups, which trade scale for curated demographics. This latter category also includes scorecard feedback from free movie previews â however, by that point, most of a productionâs budget is already spent.
The âBig Hitâ Theory/Theories
Initially, ML systems leveraged traditional analysis methods such as linear regression, K-Nearest Neighbors, Stochastic Gradient Descent, Decision Tree and Forests, and Neural Networks, usually in various combinations nearer in style to pre-AI statistical analysis, such as a 2019 University of Central Florida initiative to forecast successful TV shows based on combinations of actors and writers (among other factors):
A 2018 study rated the performance of episodes based on combinations of characters and/or writer (most episodes were written by more than one person). Source: https://arxiv.org/pdf/1910.12589
The most relevant related work, at least that which is deployed in the wild (though often criticized) is in the field of recommender systems:
A typical video recommendation pipeline. Videos in the catalog are indexed using features that may be manually annotated or automatically extracted. Recommendations are generated in two stages by first selecting candidate videos and then ranking them according to a user profile inferred from viewing preferences. Source: https://www.frontiersin.org/journals/big-data/articles/10.3389/fdata.2023.1281614/full
However, these kinds of approaches analyze projects that are already successful. In the case of prospective new shows or movies, it is not clear what kind of ground truth would be most applicable â not least because changes in public taste, combined with improvements and augmentations of data sources, mean that decades of consistent data is usually not available.
This is an instance of the cold start problem, where recommendation systems must evaluate candidates without any prior interaction data. In such cases, traditional collaborative filtering breaks down, because it relies on patterns in user behavior (such as viewing, rating, or sharing) to generate predictions. The problem is that in the case of most new movies or shows, there is not yet enough audience feedback to support these methods.
Comcast Predicts
A new paper from Comcast Technology AI, in association with George Washington University, proposes a solution to this problem by prompting a language model with structured metadata about unreleased movies.
The inputs include cast, genre, synopsis, content rating, mood, and awards, with the model returning a ranked list of likely future hits.
The authors use the modelâs output as a stand-in for audience interest when no engagement data is available, hoping to avoid early bias toward titles that are already well known.
The very short (three-page) paper, titled Predicting Movie Hits Before They Happen with LLMs, comes from six researchers at Comcast Technology AI, and one from GWU, and states:
âOur results show that LLMs, when using movie metadata, can significantly outperform the baselines. This approach could serve as an assisted system for multiple use cases, enabling the automatic scoring of large volumes of new content released daily and weekly.
âBy providing early insights before editorial teams or algorithms have accumulated sufficient interaction data, LLMs can streamline the content review process.
âWith continuous improvements in LLM efficiency and the rise of recommendation agents, the insights from this work are valuable and adaptable to a wide range of domains.â
If the approach proves robust, it could reduce the industryâs reliance on retrospective metrics and heavily-promoted titles by introducing a scalable way to flag promising content prior to release. Thus, rather than waiting for user behavior to signal demand, editorial teams could receive early, metadata-driven forecasts of audience interest, potentially redistributing exposure across a wider range of new releases.
Method and Data
The authors outline a four-stage workflow: construction of a dedicated dataset from unreleased movie metadata; the establishment of a baseline model for comparison; the evaluation of apposite LLMs using both natural language reasoning and embedding-based prediction; and the optimization of outputs through prompt engineering in generative mode, using Metaâs Llama 3.1 and 3.3 language models.
Since, the authors state, no publicly available dataset offered a direct way to test their hypothesis (because most existing collections predate LLMs, and lack detailed metadata), they built a benchmark dataset from the Comcast entertainment platform, which serves tens of millions of users across direct and third-party interfaces.
The dataset tracks newly-released movies, and whether they later became popular, with popularity defined through user interactions.
The collection focuses on movies rather than series, and the authors state:
âWe focused on movies because they are less influenced by external knowledge than TV series, improving the reliability of experiments.â
Labels were assigned by analyzing the time it took for a title to become popular across different time windows and list sizes. The LLM was prompted with metadata fields such as genre, synopsis, rating, era, cast, crew, mood, awards, and character types.
For comparison, the authors used two baselines: a random ordering; and a Popular Embedding (PE) model (which we will come to shortly).
The project used large language models as the primary ranking method, generating ordered lists of movies with predicted popularity scores and accompanying justifications â and these outputs were shaped by prompt engineering strategies designed to guide the modelâs predictions using structured metadata.
The prompting strategy framed the model as an âeditorial assistantâ assigned with identifying which upcoming movies were most likely to become popular, based solely on structured metadata, and then tasked with reordering a fixed list of titles without introducing new items, and to return the output in JSON format.
Each response consisted of a ranked list, assigned popularity scores, justifications for the rankings, and references to any prior examples that influenced the outcome. These multiple levels of metadata were intended to improve the modelâs contextual grasp, and its ability to anticipate future audience trends.
Tests
The experiment followed two main stages: initially, the authors tested several model variants to establish a baseline, involving the identification of the version which performed better than a random-ordering approach.
Second, they tested large language models in generative mode, by comparing their output to a stronger baseline, rather than a random ranking, raising the difficulty of the task.
This meant the models had to do better than a system that already showed some ability to predict which movies would become popular. As a result, the authors assert, the evaluation better reflected real-world conditions, where editorial teams and recommender systems are rarely choosing between a model and chance, but between competing systems with varying levels of predictive ability.
The Advantage of Ignorance
A key constraint in this setup was the time gap between the modelsâ knowledge cutoff and the actual release dates of the movies. Because the language models were trained on data that ended six to twelve months before the movies became available, they had no access to post-release information, ensuring that the predictions were based entirely on metadata, and not on any learned audience response.
Baseline Evaluation
To construct a baseline, the authors generated semantic representations of movie metadata using three embedding models: BERT V4; Linq-Embed-Mistral 7B; and Llama 3.3 70B, quantized to 8-bit precision to meet the constraints of the experimental environment.
Linq-Embed-Mistral was selected for inclusion due to its top position on the MTEB (Massive Text Embedding Benchmark) leaderboard.
Each model produced vector embeddings of candidate movies, which were then compared to the average embedding of the top one hundred most popular titles from the weeks preceding each movieâs release.
Popularity was inferred using cosine similarity between these embeddings, with higher similarity scores indicating higher predicted appeal. The ranking accuracy of each model was evaluated by measuring performance against a random ordering baseline.
Performance improvement of Popular Embedding models compared to a random baseline. Each model was tested using four metadata configurations: V1 includes only genre; V2 includes only synopsis; V3 combines genre, synopsis, content rating, character types, mood, and release era; V4 adds cast, crew, and awards to the V3 configuration. Results show how richer metadata inputs affect ranking accuracy. Source: https://arxiv.org/pdf/2505.02693
The results (shown above), demonstrate that BERT V4 and Linq-Embed-Mistral 7B delivered the strongest improvements in identifying the top three most popular titles, although both fell slightly short in predicting the single most popular item.
BERT was ultimately selected as the baseline model for comparison with the LLMs, as its efficiency and overall gains outweighed its limitations.
LLM Evaluation
The researchers assessed performance using two ranking approaches: pairwise and listwise. Pairwise ranking evaluates whether the model correctly orders one item relative to another; and listwise ranking considers the accuracy of the entire ordered list of candidates.
This combination made it possible to evaluate not only whether individual movie pairs were ranked correctly (local accuracy), but also how well the full list of candidates reflected the true popularity order (global accuracy).
Full, non-quantized models were employed to prevent performance loss, ensuring a consistent and reproducible comparison between LLM-based predictions and embedding-based baselines.
Metrics
To assess how effectively the language models predicted movie popularity, both ranking-based and classification-based metrics were used, with particular attention to identifying the top three most popular titles.
Four metrics were applied: Accuracy@1 measured how often the most popular item appeared in the first position; Reciprocal Rank captured how high the top actual item ranked in the predicted list by taking the inverse of its position; Normalized Discounted Cumulative Gain (NDCG@k) evaluated how well the entire ranking matched actual popularity, with higher scores indicating better alignment; and Recall@3 measured the proportion of truly popular titles that appeared in the modelâs top three predictions.
Since most user engagement happens near the top of ranked menus, the evaluation focused on lower values of k, to reflect practical use cases.
Performance improvement of large language models over BERT V4, measured as percentage gains across ranking metrics. Results were averaged over ten runs per model-prompt combination, with the top two values highlighted. Reported figures reflect the average percentage improvement across all metrics.
The performance of Llama model 3.1 (8B), 3.1 (405B), and 3.3 (70B) was evaluated by measuring metric improvements relative to the earlier-established BERT V4 baseline. Each model was tested using a series of prompts, ranging from minimal to information-rich, to examine the effect of input detail on prediction quality.
The authors state:
âThe best performance is achieved when using Llama 3.1 (405B) with the most informative prompt, followed by Llama 3.3 (70B). Based on the observed trend, when using a complex and lengthy prompt (MD V4), a more complex language model generally leads to improved performance across various metrics. However, it is sensitive to the type of information added.â
Performance improved when cast awards were included as part of the prompt â in this case, the number of major awards received by the top five billed actors in each film. This richer metadata was part of the most detailed prompt configuration, outperforming a simpler version that excluded cast recognition. The benefit was most evident in the larger models, Llama 3.1 (405B) and 3.3 (70B), both of which showed stronger predictive accuracy when given this additional signal of prestige and audience familiarity.
By contrast, the smallest model, Llama 3.1 (8B), showed improved performance as prompts became slightly more detailed, progressing from genre to synopsis, but declined when more fields were added, suggesting that the model lacked the capacity to integrate complex prompts effectively, leading to weaker generalization.
When prompts were restricted to genre alone, all models under-performed against the baseline, demonstrating that limited metadata was insufficient to support meaningful predictions.
Conclusion
LLMs have become the poster child for generative AI, which might explain why theyâre being put to work in areas where other methods could be a better fit. Even so, thereâs still a lot we donât know about what they can do across different industries, so it makes sense to give them a shot.
In this particular case, as with stock markets and weather forecasting, there is only a limited extent to which historical data can serve as the foundation of future predictions. In the case of movies and TV shows, the very delivery method is now a moving target, in contrast to the period between 1978-2011, when cable, satellite and portable media (VHS, DVD, et al.) represented a series of transitory or evolving historical disruptions.
Neither can any prediction method account for the extent to which the success or failure of other productions may influence the viability of a proposed property â and yet this is frequently the case in the movie and TV industry, which loves to ride a trend.
Nonetheless, when used thoughtfully, LLMs could help strengthen recommendation systems during the cold-start phase, offering useful support across a range of predictive methods.
First published Tuesday, May 6, 2025
#2023#2025#Advanced LLMs#advertising#agents#ai#Algorithms#Analysis#Anderson's Angle#approach#Articles#Artificial Intelligence#attention#Behavior#benchmark#BERT#Bias#collaborative#Collections#comcast#Companies#comparison#construction#content#continuous#data#data sources#dates#Decision Tree#domains
0 notes
Text
Video Annotation: Transforming Visual Data into AI-Ready Insights
As AI technology develops, so does its ability to receive and interpret visual data. The emergence of autonomous vehicles maneuvering around congested roads and security systems recognizing potential threats in real-time, AI understanding video data is transforming businesses across the globe. For AI to interpret visual data efficiently, it needs one essential piece of information: annotated video data.
Video annotation is a key aspect in converting unstructured visual data into structured information, which AI systems can understand, learn and utilize. Annotating video data is an important part of training machine learning (ML) models to perform tasks such as object detection, facial recognition, and activity recognition.
What is Video Annotation?
Video annotation is simply the act of adding labels or metadata to the frames or specific objects in a video file. These annotations are important to help AI models identify certain features, actions, or patterns in the video and by tagging important subjects or objects within the video such as people, objects, cars, and specific actions which could include gestures and other event, annotated video files become powerful training materials for AI systems.
Video annotation, in contrast to image annotation, incorporates the unique challenges inherent in moving imagery, including tracking objects over time, among other considerations requiring specific methodologies to confirm the validity of this analysis.
Why is Video Annotation Important for AI?
The significance of video annotation for AI should not be underestimated, for this may serve enhanced purposes as follows:
Augmenting Visual Comprehension for AI Models: In order to analyze video material, AI needs to clearly "understand" the significance of what it is seeing in each frame and over time.
Enhancing the Referencing of Objects and Activities: Whether you are observing videos of pedestrians using Autonomous Vehicles or a video recording of suspected fraudulent activity, annotated video data can facilitate the distinction of object activities or referring to certain objects or activities.
Training for Complex Situations: Certain applications like medical imaging or sports analytics have data that involves complex scenes that require understanding context, sequences of events, and behaviors. Annotated video datasets provide a structure for building AI systems that not only learn from a single frame but also learn from the relationships of frames.
Powering Action Recognition and Event Detection: Video annotation in AI gives the AI the means to learn to detect actions in video clips like recognizing waving from a person or a car making a turn. By labeling actions and events from the video clips, video annotation builds AI models to learn how to recognize and understand the same events in real-life situations.
How is Video Annotation Done?
Video annotation is a very painstaking task that incorporates human intervention and advanced technologies. Below are some basic methods used in the annotation of video data.
Object Detection and Tracking: In video annotation, object detection is the term used to represent the process of recognizing and tagging objects in each frame. Once the objects are tagged, tracking using algorithms will be done from frame to frame.
Activity Recognition: Annotators tag actions within the video, e.g. a person walking, running, or using an object. This helps the AI model recognize and classify several actions when it exhibits new video data. Activity recognition in video is valuable in several fields such as security, sports analysis and medicine.
Semantic Segmentation: If the level of detail is more granular, video annotation can also use semantic segmentation to classify pixels in a frame by their role in the scene (e.g. roads, pedestrians, cars). Semantic segmentation is commonplace in autonomous driving, where the AI model must separate the different objects and surfaces.
Event tagging: Event tagging applies annotations to a specific occurrence or pattern occurring in video clips, such as a crime, medical emergency or other unexplained occurrence. Annotated videos that highlight such events can be a huge asset in training AI systems in real-time event detection.
Applications of video annotation in AI
Video annotation is not constrained to one or two industries, there are wide possibilities of applications of video annotation across many industries, all of which can explore advantages of AI-enabled systems interpreting and analyzing video content. Here a few examples of video annotation applications.
Autonomous Vehicles: Self-driving cars use video annotation to identify and observe objects such as pedestrians, road signs, and other vehicles. Annotated video footage can be used to train AI models to successfully navigate complex experiences.
Surveillance and Security: Video annotation can help identify and recognize suspicious activities in security by identifying and recognizing unauthorized access or criminal activity. Video annotation can improve the ability of security systems to react to new threats in real-time.
Healthcare and Medical Imaging: Medical video and video from surgeries, can be annotated and be helpful for an AI system to identify and study symptoms, anomalies, or conditions. The use of video annotation in healthcare can help with early diagnosis and treatment planning.
Sports Analytics: Sports organizations use video annotation in sports analytics, to identify and analyze movements of players and players' metrics and performance. The use of video annotation can help coaches and analysts gain insights that can facilitate working on the team's strategies and the player's performance.
Retail and Consumer Behavior: Retailers can harness the use of video annotation for analyzing consumer behavior by documenting where consumers are looking and tracking foot movements in a store. This information provides data for retailers to make informed decisions on store layout, product placement, and marketing activities.
Issues with Video Annotation
Video annotation is important, but it offers challenges:
High Volume and Complexity: Annotating video data is hard work, especially with large amounts of footage requiring labels; annotating complex scenes that have multiple actions or objects adds to the challenge.
Cost and Resources: Depending how much work is needed, if the need for human annotators requires manual labeling large video datasets, it can be resource heavy and costly. Good news is, many companies help to alleviate readability costs and readability time with AI assisted annotation tools.
Accuracy and consistency: Consistent, accurate video annotation can be important for training reliable AI Models, as the annotators must perform the task correctly and remain consistent, shaping expectations for annotation guidelines from one video, to the next, and from scenario to scenario.
The Future of Video Annotation in AI
As AI Technologies will always continue to advance, the demand for video data that has being annotated will remain growing. Although AI tools can help promote and quicken the label process, human annotation is still required to name high-quality, higher-dimensional data representations.
Ultimately, video annotation has a promising future where improvements to accuracy, scalability, and efficiency can be achieved while promoting video annotation across fields such as autonomous driving, stimulation training, healthcare, entertainment, and many, many more.
Conclusion
Video annotation fuses the gap between video content to actionable data and is a fundamental process of AI-based visual recognition. Through video annotation, AI systems can understand and comprehend video and cinematic data.
Therefore, video annotation is fulfilling and fulfilling opportunities for industries around the world. Video annotation is a fundamental process, enabling a world with artificially intelligent systems that can intelligently recognize, react, and predict what happens in the world.
Visit Globose Technology Solutions to see how the team can speed up your face image datasets.
0 notes