#gpt-3 prompt engineering
Explore tagged Tumblr posts
Text
Pegasus 1.2: High-Performance Video Language Model

Pegasus 1.2 revolutionises long-form video AI with high accuracy and low latency. Scalable video querying is supported by this commercial tool.
TwelveLabs and Amazon Web Services (AWS) announced that Amazon Bedrock will soon provide Marengo and Pegasus, TwelveLabs' cutting-edge multimodal foundation models. Amazon Bedrock, a managed service, lets developers access top AI models from leading organisations via a single API. With seamless access to TwelveLabs' comprehensive video comprehension capabilities, developers and companies can revolutionise how they search for, assess, and derive insights from video content using AWS's security, privacy, and performance. TwelveLabs models were initially offered by AWS.
Introducing Pegasus 1.2
Unlike many academic contexts, real-world video applications face two challenges:
Real-world videos might be seconds or hours lengthy.
Proper temporal understanding is needed.
TwelveLabs is announcing Pegasus 1.2, a substantial industry-grade video language model upgrade, to meet commercial demands. Pegasus 1.2 interprets long films at cutting-edge levels. With low latency, low cost, and best-in-class accuracy, model can handle hour-long videos. Their embedded storage ingeniously caches movies, making it faster and cheaper to query the same film repeatedly.
Pegasus 1.2 is a cutting-edge technology that delivers corporate value through its intelligent, focused system architecture and excels in production-grade video processing pipelines.
Superior video language model for extended videos
Business requires handling long films, yet processing time and time-to-value are important concerns. As input films increase longer, a standard video processing/inference system cannot handle orders of magnitude more frames, making it unsuitable for general adoption and commercial use. A commercial system must also answer input prompts and enquiries accurately across larger time periods.
Latency
To evaluate Pegasus 1.2's speed, it compares time-to-first-token (TTFT) for 3–60-minute videos utilising frontier model APIs GPT-4o and Gemini 1.5 Pro. Pegasus 1.2 consistently displays time-to-first-token latency for films up to 15 minutes and responds faster to lengthier material because to its video-focused model design and optimised inference engine.
Performance
Pegasus 1.2 is compared to frontier model APIs using VideoMME-Long, a subset of Video-MME that contains films longer than 30 minutes. Pegasus 1.2 excels above all flagship APIs, displaying cutting-edge performance.
Pricing
Cost Pegasus 1.2 provides best-in-class commercial video processing at low cost. TwelveLabs focusses on long videos and accurate temporal information rather than everything. Its highly optimised system performs well at a competitive price with a focused approach.
Better still, system can generate many video-to-text without costing much. Pegasus 1.2 produces rich video embeddings from indexed movies and saves them in the database for future API queries, allowing clients to build continually at little cost. Google Gemini 1.5 Pro's cache cost is $4.5 per hour of storage, or 1 million tokens, which is around the token count for an hour of video. However, integrated storage costs $0.09 per video hour per month, x36,000 less. Concept benefits customers with large video archives that need to understand everything cheaply.
Model Overview & Limitations
Architecture
Pegasus 1.2's encoder-decoder architecture for video understanding includes a video encoder, tokeniser, and big language model. Though efficient, its design allows for full textual and visual data analysis.
These pieces provide a cohesive system that can understand long-term contextual information and fine-grained specifics. It architecture illustrates that tiny models may interpret video by making careful design decisions and solving fundamental multimodal processing difficulties creatively.
Restrictions
Safety and bias
Pegasus 1.2 contains safety protections, but like any AI model, it might produce objectionable or hazardous material without enough oversight and control. Video foundation model safety and ethics are being studied. It will provide a complete assessment and ethics report after more testing and input.
Hallucinations
Occasionally, Pegasus 1.2 may produce incorrect findings. Despite advances since Pegasus 1.1 to reduce hallucinations, users should be aware of this constraint, especially for precise and factual tasks.
#technology#technews#govindhtech#news#technologynews#AI#artificial intelligence#Pegasus 1.2#TwelveLabs#Amazon Bedrock#Gemini 1.5 Pro#multimodal#API
2 notes
·
View notes
Text
I could write so many tumblr posts about ChatGPT.
Zero: Already written. GPT-3/Midjourney is not a good tool for procedural level/content generation.
One: Remember when Siri was the future, or when Siri was the beginning of intelligent machines, or when Siri meant humans would just stop thinking for themselves and outsource things to computers, or at least when Siri, Alexa, Cortana, and "OK, Google" were spelling doom for the touchscreen/mouse and keyboard because in The Future, we will all talk to our computers like Captain Picard? Do these people have egg on their face or are they boldly ignoring their past mistakes?
Two: Remember when we called it "Machine Learning" instead of AI, because remember what happened the last time we hyped up things as AI? Why are people doing this again?
Three: Back to Siri. People were prognosticating that Siri would only get smarter. In many ways, it did, but that didn't result in a "general intelligence". And yet, Siri (and "OK Google") knows so many things for sure. Unlike GPT-3, which essentially suffers from fluent aphasia or Korsakoff Syndrome, Siri had a knowledge base and could reason. It wasn't intelligent, I grant you that. But do you understand why Siri, or IBM's Watson, or even Wolfram Alpha did not scale up to become ChatGPT? I mean I do, it's software engineering and marketing and economics of scale. But do those people who make grand predictions about GPT-4 understand this?
Four: Here in Germany, I hear politicians call for a more "competitive" AI policy, which mostly means less data protection. We are already in the absurd situation where a doctor can't publish the success rates of different surgical techniques in retrospect, because that would be a study and subjects have to consent in advance and a study on human subjects needs a good reason and also a control group - while at the same time the government wants to give health data to medical app start-ups in bulk. You think this isn't really about ChatGPT, but it is about machine learning. It looks like the government doesn't want doctors to analyse data, but start-ups, and it doesn't want studies, but products.
Five: AI is a marketing gimmick anyway. Many products just use AI to use AI. Blog posts about using AI to do a task exist to create FOMO in people who don't use AI. Products use "AI" in order to court controversy.
Six: Prompt injection and prompt leaking should be easily solved in principle, and I am sure by this time next year they will be "solved", and have been in some proof of concept projects, but in practice economic incentives apply that make this difficult or we would have solved it already.
Seven: Prompt engineering is difficult. It requires some insight into the behaviour of a language model, or at least its inner workings. Will prompt engineering stay relevant? On a related note, Google-Fu still as relevant as it used to be in 2004?
Eight: Did Siri get worse?
18 notes
·
View notes
Text
Just in case there are any students following me who might actually try this:
CHAT GPT AIN'T WORTH IT. Just write the damned essay.
I am a writing tutor. I specifically tutor essay writing and as of right now have reviewed 2292 student essays over the pat three years. 500 of those within the past 3 months. I can spot a ChatGPT essay a mile away.
They are very bad.
They sound pretty. The language is exquisite. The content, nnnnnotsomuch. It's bland. Just based on how the model was trained, and what it was designed to do, it is trained to produce very pretty mediocrity.
Real essays need analysis. You need evidence, specific claims, a clear structure, a thesis. ChatGPT does not produce essays with any of these. It writes articles with sophisticated words and statements that sound true (but which may or may not be true).
It may be possible to get ChatGPT, or some other language-learning model, to produce an essay I couldn't clock as AI-written, but that would require some very sophisticated prompt engineering, and you would need to do the research and analysis yourself. You may as well write the essay at that point.
Yes, it can make your language sound prettier. But don't let it. That's a skill you want to develop so that you can express your ideas clearly. I promise you. It's not worth it.
7 notes
·
View notes
Text
Until the dramatic departure of OpenAI’s cofounder and CEO Sam Altman on Friday, Mira Murati was its chief technology officer—but you could also call her its minister of truth. In addition to heading the teams that develop tools such as ChatGPT and Dall-E, it’s been her job to make sure those products don’t mislead people, show bias, or snuff out humanity altogether.
This interview was conducted in July 2023 for WIRED’s cover story on OpenAI. It is being published today after Sam Altman’s sudden departure to provide a glimpse at the thinking of the powerful AI company’s new boss.
Steven Levy: How did you come to join OpenAI?
Mira Murati: My background is in engineering, and I worked in aerospace, automotive, VR, and AR. Both in my time at Tesla [where she shepherded the Model X], and at a VR company [Leap Motion] I was doing applications of AI in the real world. I very quickly believed that AGI would be the last and most important major technology that we built, and I wanted to be at the heart of it. Open AI was the only organization at the time that was incentivized to work on the capabilities of AI technology and also make sure that it goes well. When I joined in 2018, I began working on our supercomputing strategy and managing a couple of research teams.
What moments stand out to you as key milestones during your tenure here?
There are so many big-deal moments, it’s hard to remember. We live in the future, and we see crazy things every day. But I do remember GPT-3 being able to translate. I speak Italian, Albanian, and English. I remember just creating pair prompts of English and Italian. And all of a sudden, even though we never trained it to translate in Italian, it could do it fairly well.
You were at OpenAI early enough to be there when it changed from a pure nonprofit to reorganizing so that a for-profit entity lived inside the structure. How did you feel about that?
It was not something that was done lightly. To really understand how to make our models better and safer, you need to deploy them at scale. That costs a lot of money. It requires you to have a business plan, because your generous nonprofit donors aren't going to give billions like investors would. As far as I know, there's no other structure like this. The key thing was protecting the mission of the nonprofit.
That might be tricky since you partner so deeply with a big tech company. Do you feel your mission is aligned with Microsoft’s?
In the sense that they believe that this is our mission.
But that's not their mission.
No, that's not their mission. But it was important for the investor to actually believe that it’s our mission.
When you joined in 2018, OpenAI was mainly a research lab. While you still do research, you’re now very much a product company. Has that changed the culture?
It has definitely changed the company a lot. I feel like almost every year, there's some sort of paradigm shift where we have to reconsider how we're doing things. It is kind of like an evolution. What's more obvious now to everyone is this need for continuous adaptation in society, helping bring this technology to the world in a responsible way, and helping society adapt to this change. That wasn't necessarily obvious five years ago, when we were just doing stuff in our lab. But putting GPT-3 in an API, in working with customers and developers, helped us build this muscle of understanding the potential that the technology has to change things in the real world, often in ways that are different than what we predict.
You were involved in Dall-E. Because it outputs imagery, you had to consider different things than a text model, including who owns the images that the model draws upon. What were your fears and how successful you think you were?
Obviously, we did a ton of red-teaming. I remember it being a source of joy, levity, and fun. People came up with all these like creative, crazy prompts. We decided to make it available in labs, as an easy way for people to interact with the technology and learn about it. And also to think about policy implications and about how Dall-E can affect products and social media or other things out there. We also worked a lot with creatives, to get their input along the way, because we see it internally as a tool that really enhances creativity, as opposed to replacing it. Initially there was speculation that AI would first automate a bunch of jobs, and creativity was the area where we humans had a monopoly. But we've seen that these AI models actually have a potential to really be creative. When you see artists play with Dall-E, the outputs are really magnificent.
Since OpenAI has released its products, there have been questions about their immediate impact in things like copyright, plagiarism, and jobs. By putting things like GPT-4 in the wild, it’s almost like you’re forcing the public to deal with those issues. Was that intentional?
Definitely. It's actually very important to figure out how to bring it out there in a way that's safe and responsible, and helps people integrate it into their workflow. It’s going to change entire industries; people have compared it to electricity or the printing press. And so it's very important to start actually integrating it in every layer of society and think about things like copyright laws, privacy, governance and regulation. We have to make sure that people really experience for themselves what this technology is capable of versus reading about it in some press release, especially as the technological progress continues to be so rapid. It's futile to resist it. I think it's important to embrace it and figure out how it's going to go well.
Are you convinced that that's the optimal way to move us toward AGI?
I haven't come up with a better way than iterative deployments to figure out how you get this continuous adaptation and feedback from the real end feeding back into the technology to make it more robust to these use cases. It’s very important to do this now, while the stakes are still low. As we get closer to AGI, it's probably going to evolve again, and our deployment strategy will change as we get closer to it.
5 notes
·
View notes
Text
Generate corporate profiles rich with data with CorporateBots from @Lemonbarski on POE.
It’s free to use with a free POE AI account. Powered by GPT3 from OpenAI, the CorporateBots are ready to compile comprehensive corporate data files in CSV format - so you can read it and so can your computer.
Use cases: Prospecting, SWOT analysis, Business Plans, Market Assessment, Competitive Threat Analysis, Job Search.
Each of the CorporateBots series by Lemonbarski Labs by Steven Lewandowski (@Lemonbarski) provides a piece of a comprehensive corporate profile for leaders in an industry, product category, market, or sector.
Combine the datasets for a full picture of a corporate organization and begin your project with a strong, data-focused foundation and a complete picture of a corporate entity’s business, organization, finances, and market position.
Lemonbarski Labs by Steven Lewandowski is the Generative AI Prompt Engineer of CorporateBots on POE | Created on the POE platform by Quora | Utilizes GPT-3 Large Language Model Courtesy of OpenAI | https://lemonbarski.com | https://Stevenlewandowski.us | Where applicable, copyright 2023 Lemonbarski Labs by Steven Lewandowski
Steven Lewandowski is a creative, curious, & collaborative marketer, researcher, developer, activist, & entrepreneur based in Chicago, IL, USA
Find Steven Lewandowski on social media by visiting https://Stevenlewandowski.us/connect | Learn more at https://Steven.Lemonbarski.com or https://stevenlewandowski.us
#poe ai#lemonbarski#generative ai#llm#chatbot#chatgpt#open ai#gpt3#data collection services#chicago#swotanalysis#job search#competitive intelligence#companies#csv
2 notes
·
View notes
Text
Prompt Examples for Learning Web Development
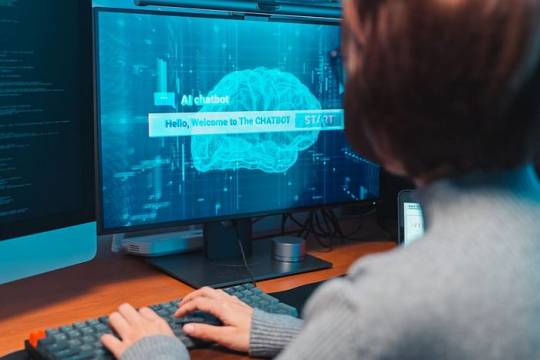
Coding is both an art and a science. It���s about creatively solving problems, bringing ideas to life, and constantly learning and adapting.
Because technology advances at such a rapid pace, it is essential to be fluent in a variety of languages, tools, and domains.
Sometimes it’s difficult to pick up the right resources from the ocean of tutorials, demos, and resources.
And on top of that, sometimes we have to learn and apply so fast due to tight deadlines of the projects. In this case, we need a friend who can help us learn and work faster and better. And thanks to AI by this, our learning becomes faster and more fun.
Today, we’ll look at how learning prompts that AI drives can change the way you learn web development.
How you can craft prompt engineering for web development, the difference between a generic prompt and a bit tweaked prompt can eventually change your desired results and make your learning journey more smooth and more enjoyable.
You can also use this knowledge to learn other fields more quickly and interactively.
Table of Contents
Learning Prompts
HTML Prompt Examples
CSS Prompt Examples
Debugging Prompts
Testing Prompts
Crafting Better Prompts
Further Reading and Resources
🎯Learning Prompts
Prompts are at the heart of AI-powered learning. Prompts are questions or commands that guide AI models like GPT-3 or GPT-4 to generate the desired responses. They act as a springboard for the AI to dive into the knowledge it’s been trained on and come up with relevant outputs.
You can use AI’s capabilities in a variety of scenarios in web development, including debugging, code generation, and even learning new web development concepts.
Now, we’ll go through some basic prompts and their outputs, as well as a little tweaking of the prompt commands to see how the output is becoming more result oriented, giving you a sense of how you may build your prompt commands for better results.
Prompt Commands for Learning HTML Basics
Learning the basics of web development involves understanding the structure and syntax of HTML, CSS, and JavaScript. Here are some prompt examples you can use:
Create a simple HTML structure with a header, main content section, and footer.
This prompt returns a simple HTML skeleton. But if you want a more detailed structure, you could modify the prompt to include specific HTML elements. For example:
Create a simple HTML structure with a header containing a navigation bar, a main content section with a paragraph and an image, and a footer with copyright information.
Curious to know more? Visit our blog for the complete post and dive deeper into Learning Web Development with AI Prompts.
3 notes
·
View notes
Text
Global Apprenticeship in Generative AI: Upskill with Clevered for the Future of Work
In a world rapidly transforming under the influence of artificial intelligence, staying ahead means more than just understanding the basics—it means mastering the tools of tomorrow. That’s where the Global Apprenticeship in Generative AI, launched in partnership with Clevered, comes in. Designed as a future-ready upskilling program, this apprenticeship empowers students, professionals, and career-switchers with the cutting-edge skills needed to thrive in the AI-driven world.
Whether you're an aspiring data scientist, a creative technologist, or a working professional eager to pivot into AI, this immersive, hands-on program is your launchpad into the dynamic field of Generative AI.
Why Generative AI?
Generative AI has become one of the most transformative technologies of the 21st century. From AI-generated art and synthetic media to large language models like GPT, DALL·E, and Stable Diffusion, generative AI is not just shaping the future—it is the future.
With applications across healthcare, finance, education, design, and software engineering, the demand for skilled professionals in this domain has skyrocketed. However, the talent gap remains wide.
This is why Clevered, a leader in global learning and development, has created a globally accessible, industry-aligned apprenticeship program focused exclusively on Generative AI upskilling—bridging the skills gap and unlocking opportunities for talent everywhere.
Program Overview
The Global Apprenticeship in Generative AI is a structured, project-based learning experience that blends theory with real-world application. Spanning across 12–16 weeks, the program is designed to be flexible yet intensive, with live mentorship, global networking opportunities, and access to enterprise-grade AI tools.
Key Features:
Global Access: Open to learners from all regions—no matter where you're based, you can join and learn.
Live Sessions & Mentorship: Industry experts and AI engineers guide you through hands-on labs, model building, and career development.
Capstone Projects: Work on real-world generative AI applications in areas like content generation, synthetic data, and automation.
AI Ethics & Responsible Innovation: Explore bias mitigation, responsible AI design, and the importance of explainability.
Career Acceleration: Personalized career coaching, LinkedIn profile development, and mock interviews included.
Certification: Earn a globally recognized certificate co-branded with Clevered and top AI companies.
What You'll Learn
This apprenticeship is more than just a bootcamp—it's an ecosystem for deep AI mastery, structured around four major learning tracks:
1. Foundations of Generative AI
What is generative AI?
Differences between discriminative and generative models
Introduction to neural networks, deep learning, and transformers
2. Core Technical Skills
Building and fine-tuning models like GPT, BERT, and diffusion models
Prompt engineering and LLM application development
Text-to-image, audio synthesis, and code generation with AI
3. Responsible AI
Fairness, transparency, and explainability
Risks and mitigation strategies in deploying generative models
Introduction to AI governance and policy trends
4. Innovation & Impact
How generative AI is transforming industries (health, finance, media)
Real-world case studies
Ideating and prototyping your own generative AI application
Who Should Apply?
This program is designed to be inclusive, accessible, and globally relevant. Whether you're a student, early-career professional, or someone switching fields, this apprenticeship gives you both the theoretical foundation and practical tools needed to lead in AI.
Ideal for:
Computer science or engineering students
Creative professionals (designers, writers, marketers)
Educators and researchers
Entrepreneurs and startup founders
Mid-career professionals in tech or adjacent industries
No advanced coding or math background? No problem. The program starts with foundational concepts and builds up progressively, with optional deep-dives for advanced learners.
Why Choose Clevered?
Clevered is redefining how upskilling works at scale. Known for its commitment to accessibility, equity, and global learning innovation, Clevered partners with leading universities, companies, and technology platforms to create future-ready talent.
With Clevered, you get:
A trusted, mission-driven learning partner
Global peer community & alumni network
Opportunities for internships and job placements
Ongoing support even after the program ends
Success Stories
Application Process
Joining the Global Apprenticeship in Generative AI is simple:
Apply Online: Fill out a short application form outlining your goals and background.
Screening: Participate in a brief aptitude assessment or portfolio review (no coding test for non-technical tracks).
Interview: A short conversation with our admissions team to align expectations and learning goals.
Enroll & Begin: Get access to your learning dashboard, community channels, and upcoming sessions.
Rolling admissions now open for the next cohort. Spaces are limited to ensure personalized mentorship.
Invest in Your Future
In an era where AI is reshaping every aspect of work and creativity, the best investment you can make is in your ability to learn, adapt, and lead.
This apprenticeship with Clevered isn’t just about upskilling—it’s about future-proofing your career and becoming part of a global movement shaping the ethical, innovative use of generative AI.
Ready to step into the future?
FAQs
Q: Do I need to have a technical background?
A: No. We offer differentiated tracks for technical and non-technical learners. Everyone can contribute to the AI future.
Q: Is the program online?
A: Yes, it’s 100% online with live sessions, self-paced modules, and asynchronous collaboration.
Q: Will I get a certificate?
A: Yes, a verified certificate issued by Clevered and participating partner organizations.
#Generative AI apprenticeship#AI apprenticeship program#Generative AI education#AI skill development
0 notes
Text
AI and the Future of Work - The New Era of Productivity

Generative AI has evolved rapidly from novelty to necessity. But despite its explosive adoption, most professionals are still underutilizing its real potential. Why?
Because they treat AI like a search engine, not a strategy partner.
Tools like ChatGPT, DeepSeek, Claude, and Gemini can do far more than answer questions—they can elevate thinking, accelerate decision-making, and automate complex processes. In this new era of Productivity 4.0, the key differentiator isn’t access to AI—it’s how well you use it.
The Productivity Paradox: Everyone Has AI, But Few Get Results

McKinsey’s 2023 report on the economic potential of generative AI estimated that it could add up to $4.4 trillion annually to the global economy. But here’s the paradox: while adoption is widespread, impact is uneven.
Most professionals report using AI for surface-level tasks—like writing emails or summarizing documents. These are helpful, but not transformative. The real gains come when AI is integrated into core workflows, especially in areas like:
Strategic planning
Data interpretation
Team management and training
Problem-solving and innovation cycles
The question is: How do you get there?
AI Is Only as Good as the User: Why Prompting Is the New Power Skill

We’ve entered a new skill economy where prompt engineering—the art of giving AI the right instructions—defines how useful the tool becomes. According to MIT Sloan, professionals who use iterative prompting (refining AI responses step-by-step) can generate outcomes 40% more accurate and relevant than those who rely on single queries.
Example Use Case: Strategic Brainstorming
Instead of asking:
“Give me marketing ideas for a new product.”
A skilled professional might guide AI through a layered prompt path:
“Identify customer segments based on this product feature set.”
“Based on Segment A, generate emotional messaging that resonates with early adopters.”
“What platforms are most aligned with their behavior?”
The output becomes customized strategy, not generic advice.
From Information Overload to Insight Extraction

Professionals are drowning in data. According to IDC, data creation will reach 180 zettabytes by 2025, and yet, less than 3% of it is analyzed. That’s a staggering waste of potential insight.
Generative AI can help bridge this gap—but only if guided properly. When used intentionally, AI can:
Cluster and summarize large reports
Identify trends from unstructured datasets
Extract key actions or red flags from customer feedback or performance logs
Case Study:
A Fortune 500 HR department used GPT-based tools to analyze over 10,000 open-ended exit interview comments. Through advanced prompting and sentiment analysis, they uncovered a pattern of dissatisfaction tied to a specific managerial policy—insight that had been missed for years using traditional analysis.
Result? Policy change led to a 12% reduction in employee churn within 6 months.
Training, Upskilling, and Knowledge Transfer at Scale
AI can also reshape learning and development. By turning internal documents and expert knowledge into adaptive AI tutors, companies are accelerating onboarding and reskilling.
Imagine giving new hires access to an AI agent that understands your company’s SOPs, tone of voice, and workflows—and can answer questions 24/7 with consistency. According to Deloitte, firms using AI-enhanced learning solutions are seeing 30–50% reductions in training time while improving retention.
This is no longer the future. It’s happening now.
The Real Opportunity: AI as a Co-Creator of Value

To lead in the Productivity 4.0 era, professionals must move beyond passive use. They need to actively partner with AI to:
Design better strategies
Refine customer experiences
Accelerate innovation pipelines
Improve cross-functional collaboration
This shift isn’t about replacing humans—it’s about amplifying them.
Action Framework: From Tool to Transformation
Adopt: Experiment with different tools and models.
Adapt: Integrate AI into day-to-day workflows (not just occasional tasks).
Advance: Develop internal use cases that align with team goals and KPIs.
Augment: Combine AI outputs with human judgment to make decisions faster and better.
Conclusion: The Leaders of Tomorrow Are Learning AI Today
Those who unlock the deeper use cases of AI will not only outperform—they’ll redefine what performance looks like.
Whether you're in operations, marketing, HR, or executive leadership, now is the time to explore how AI can integrate into your strategic stack, not just your task list.
The difference between professionals who keep up—and those who leap forward—comes down to this: Are you learning how to use AI effectively, or just using it occasionally?
Your Blueprint for Success: Transform Your Productivity and Master Stress-Free Leadership
The good news is that these principles don’t have to be learned through trial and error. Productivity 4.0 offers a proven blueprint to help executives master these strategies in a clear and structured way.
By applying the tools and techniques from the Productivity 4.0 Premium Course, you’ll learn how to streamline your email workflow, optimize your task management, and organize your information—all in a way that reduces stress and boosts your decision-making capacity.
Are you ready to take control of your time, reduce stress, and become a more effective executive? The tools and strategies are at your fingertips. It’s time to transform your productivity and unlock your full leadership potential.
Scheduling more—it’s in managing your energy smarter. 🚀
📺 Watch this video to learn how top performers structure their day around peak energy levels, maximize deep work, and avoid burnout. Discover science-backed strategies to work smarter, not harder! 💡
How to setup a new office for your startup with high productivity
Besides, these are useful tips if you want to cultivate your skills as a leader:
Leadership Development Guide: Watch Strategy (Yes, it's Free)
Process Improvement Toolkit: Download PDF (Yes, it's Free)
Workforce Flywheel Framework Training: Watch here (Yes, it's Free)
Tools for HR Leaders Access Here (Yes, it's Free)
References:
David Allen. Getting Things Done: The Art of Stress-Free Productivity. Penguin Books, 2001.
Tony Schwartz. The Power of Full Engagement: Managing Energy, Not Time, Is the Key to High Performance and Personal Renewal. Free Press, 2003.
Cal Newport. Deep Work: Rules for Focused Success in a Distracted World. Grand Central Publishing, 2016.
Author information: My HoaPassionate Learning & Program Officer VSHR Pro Academy
0 notes
Text
What Can Only an AI Expert Do That a Beginner Cannot?
On a platform like AI Experts Review, which positions itself as a hub for the Best AI Tools Review in 2025, the difference between an AI expert and a beginner is both strategic and deeply technical. Here’s how:
🔍 1. In-Depth AI Tools Review (Beyond the Basics)
AI Expert: Can critically analyze how an AI tool’s algorithm works, its training data, bias risks, model limitations, and scalability.
Beginner: May only rely on the tool’s surface features or marketing claims.
✅ For example, on AI Experts Review, an expert won’t just say ChatGPT is “good for writing.” They’ll explain how its transformer architecture contributes to contextual accuracy, and where it outperforms or underperforms compared to other models.
📈 2. Evaluate AI Tools for SEO Like a Pro
AI Expert: Understands how AI integrates with search engine algorithms (like Google's BERT or RankBrain) and can test tools for keyword clustering, intent detection, and SERP forecasting.
Beginner: Might only use AI tools for content generation or basic keyword suggestions without strategy.
✅ A detailed post on "AI Tools for SEO" by an expert would include tools like Surfer SEO, Clearscope, or Frase and compare how their AI models adapt to Google’s core updates.
🧠 3. Model Customization and Prompt Engineering
AI Expert: Can fine-tune models (like OpenAI’s GPT or Meta’s LLaMA), write advanced prompts for specific tasks, and integrate APIs for custom workflows.
Beginner: Might copy-paste prompts without understanding their effect or limitations.
✅ A top-level AI Tools Review can only come from someone who knows how these tools are built and tested — not just used.
💡 4. Future-Proofing Tool Selection
AI Expert: Can predict which tools are built on sustainable architecture (like transformer models vs. heuristic ones) and assess long-term viability.
Beginner: May go with whatever’s trending, risking investments in short-lived tools.
✅ Your website gains authority when reviews don’t just rank tools — but forecast which will thrive based on technical depth, especially in the Best AI Tools Review in 2025.
Final Thought:
AI Experts Review isn’t just a place for tool listings — it's where AI expertise meets practical application. If you want to be known for the Best AI Tools Review in 2025, make sure your content reflects what only an expert can uncover.
0 notes
Text
Is Hiring a Prompt Engineer Worth It for Your AI Startup?
As artificial intelligence (AI) continues to evolve, startups are rapidly adopting AI technologies to improve customer experience, streamline operations, and drive innovation. One crucial role emerging within the AI ecosystem is that of the prompt engineer. But is hiring a prompt engineer really worth it for your AI startup? Let’s explore the growing importance of this specialized role and how it can take your AI-driven solutions to the next level.
🔍 What Is a Prompt Engineer?
Before diving into the value a prompt engineer can bring to your AI startup, it’s important to understand what this role entails. A prompt engineer is responsible for crafting and optimizing input queries or prompts to achieve the desired output from AI models, particularly natural language processing (NLP) models like GPT (Generative Pre-trained Transformer). The role goes beyond simply providing commands to AI systems; prompt engineers need to understand the intricacies of language, context, and AI model behavior to fine-tune prompts for maximum efficiency and accuracy.
Prompt engineers are also responsible for:
Optimizing AI responses to be more accurate and contextually appropriate.
Refining prompts to ensure AI models understand user intent correctly.
Improving user interactions with AI tools, ensuring smoother and more human-like conversations.
🤖 Why Hire a Prompt Engineer for Your AI Startup?
1. Enhanced AI Model Performance
In AI development, the quality of output is only as good as the input. This is especially true for language models and conversational AI systems. By hiring a prompt engineer, you gain a professional who understands how to formulate complex queries that drive better, more accurate results.
For AI startups working with chatbots, virtual assistants, or content generation tools, a prompt engineer can fine-tune the model to ensure that AI responses are precise, relevant, and align with your startup’s brand voice.
2. Increased Efficiency and Productivity
A well-optimized prompt can drastically reduce the time it takes for AI to produce relevant results. Startups often work with limited resources and tight deadlines. By hiring a prompt engineer, you ensure that your AI models are operating at peak efficiency, which ultimately saves time, resources, and the frustration of dealing with AI responses that miss the mark.
Whether you're developing a chatbot to handle customer queries or a content generation tool to automate marketing copy, a prompt engineer will streamline these processes, improving overall productivity.
3. Cost Savings in the Long Run
AI development can be expensive, particularly for startups with tight budgets. One of the biggest costs of implementing AI is the trial and error involved in training and optimizing models. With a prompt engineer on your team, you’re more likely to avoid the costly mistakes that can arise from poorly designed prompts or ineffective training.
Instead of spending weeks or months retraining AI models, prompt engineers can quickly adjust input queries to ensure that your AI delivers consistent and high-quality output from the start. This reduces development time, lowers costs, and accelerates your AI product's time-to-market.
4. Customization and Personalization
In a highly competitive market, offering personalized experiences is essential for the success of any AI-powered product. A prompt engineer can tailor AI models to understand specific nuances, customer needs, and industry-specific language.
For example, if your AI startup operates in healthcare, a prompt engineer can refine your prompts to ensure that AI understands complex medical terminology, thus providing more accurate and relevant responses. This level of customization would be difficult to achieve without the expertise of a prompt engineer.
5. Scalability and Future-Proofing
As your AI startup grows, so will the complexity of your AI models. A prompt engineer ensures that your AI system remains scalable and adaptable to new challenges. Whether you’re expanding into new markets, developing more advanced AI models, or adding more features, a prompt engineer can help optimize prompts for different use cases and environments.
This adaptability is crucial as AI technologies continue to evolve, and businesses need to remain flexible to stay competitive.
🏆 Is It Worth the Investment?
While hiring a prompt engineer may seem like an additional cost for a startup, the long-term benefits far outweigh the initial investment. By hiring a prompt engineer, you're ensuring that your AI-powered solutions are optimized for efficiency, accuracy, and scalability—critical factors that can significantly impact your startup's success.
The right prompt engineer can streamline your AI development process, enhance the performance of your AI models, and ensure that your AI solutions meet the specific needs of your customers. This ultimately gives your AI startup a competitive edge in a rapidly evolving market.
📝 How to Hire the Right Prompt Engineer
Hiring the right prompt engineer for your AI startup is key to achieving the best results. Look for candidates with:
Experience in AI and NLP models, particularly with models like GPT, BERT, or other transformer-based architectures.
Strong problem-solving skills to handle the nuances of AI behavior and improve outputs.
Excellent communication skills, as they must understand both the technical and user-facing aspects of AI interactions.
Knowledge of machine learning frameworks like TensorFlow, PyTorch, or similar platforms.
By focusing on these skills and qualifications, you can ensure that you hire the right talent to optimize your AI solutions.
💡 Conclusion
In conclusion, hiring a prompt engineer can significantly enhance the performance and efficiency of your AI startup's solutions. From improving AI output accuracy to reducing development costs and ensuring scalability, the expertise of a prompt engineer is an investment that will pay off in the long run.
As AI technologies continue to shape the future of business, startups that embrace specialized roles like prompt engineers will have a distinct advantage in delivering high-quality, effective AI products that meet customer needs and stay ahead of the competition.
Ready to optimize your AI models with a prompt engineer? If you’re an AI startup looking to enhance the performance of your AI systems, hiring a prompt engineer could be the game-changer you need. Reach out to us today to discuss how we can help you scale your AI solutions efficiently and effectively.
0 notes
Text
Generative AI Platform Development Explained: Architecture, Frameworks, and Use Cases That Matter in 2025
The rise of generative AI is no longer confined to experimental labs or tech demos—it’s transforming how businesses automate tasks, create content, and serve customers at scale. In 2025, companies are not just adopting generative AI tools—they’re building custom generative AI platforms that are tailored to their workflows, data, and industry needs.
This blog dives into the architecture, leading frameworks, and powerful use cases of generative AI platform development in 2025. Whether you're a CTO, AI engineer, or digital transformation strategist, this is your comprehensive guide to making sense of this booming space.
Why Generative AI Platform Development Matters Today
Generative AI has matured from narrow use cases (like text or image generation) to enterprise-grade platforms capable of handling complex workflows. Here’s why organizations are investing in custom platform development:
Data ownership and compliance: Public APIs like ChatGPT don’t offer the privacy guarantees many businesses need.
Domain-specific intelligence: Off-the-shelf models often lack nuance for healthcare, finance, law, etc.
Workflow integration: Businesses want AI to plug into their existing tools—CRMs, ERPs, ticketing systems—not operate in isolation.
Customization and control: A platform allows fine-tuning, governance, and feature expansion over time.
Core Architecture of a Generative AI Platform
A generative AI platform is more than just a language model with a UI. It’s a modular system with several architectural layers working in sync. Here’s a breakdown of the typical architecture:
1. Foundation Model Layer
This is the brain of the system, typically built on:
LLMs (e.g., GPT-4, Claude, Mistral, LLaMA 3)
Multimodal models (for image, text, audio, or code generation)
You can:
Use open-source models
Fine-tune foundation models
Integrate multiple models via a routing system
2. Retrieval-Augmented Generation (RAG) Layer
This layer allows dynamic grounding of the model in your enterprise data using:
Vector databases (e.g., Pinecone, Weaviate, FAISS)
Embeddings for semantic search
Document pipelines (PDFs, SQL, APIs)
RAG ensures that generative outputs are factual, current, and contextual.
3. Orchestration & Agent Layer
In 2025, most platforms include AI agents to perform tasks:
Execute multi-step logic
Query APIs
Take user actions (e.g., book, update, generate report)
Frameworks like LangChain, LlamaIndex, and CrewAI are widely used.
4. Data & Prompt Engineering Layer
The control center for:
Prompt templates
Tool calling
Memory persistence
Feedback loops for fine-tuning
5. Security & Governance Layer
Enterprise-grade platforms include:
Role-based access
Prompt logging
Data redaction and PII masking
Human-in-the-loop moderation
6. UI/UX & API Layer
This exposes the platform to users via:
Chat interfaces (Slack, Teams, Web apps)
APIs for integration with internal tools
Dashboards for admin controls
Popular Frameworks Used in 2025
Here's a quick overview of frameworks dominating generative AI platform development today: FrameworkPurposeWhy It MattersLangChainAgent orchestration & tool useDominant for building AI workflowsLlamaIndexIndexing + RAGPowerful for knowledge-based appsRay + HuggingFaceScalable model servingProduction-ready deploymentsFastAPIAPI backend for GenAI appsLightweight and easy to scalePinecone / WeaviateVector DBsCore for context-aware outputsOpenAI Function Calling / ToolsTool use & plugin-like behaviorPlug-in capabilities without agentsGuardrails.ai / Rebuff.aiOutput validationFor safe and filtered responses
Most Impactful Use Cases of Generative AI Platforms in 2025
Custom generative AI platforms are now being deployed across virtually every sector. Below are some of the most impactful applications:
1. AI Customer Support Assistants
Auto-resolve 70% of tickets with contextual data from CRM, knowledge base
Integrate with Zendesk, Freshdesk, Intercom
Use RAG to pull product info dynamically
2. AI Content Engines for Marketing Teams
Generate email campaigns, ad copy, and product descriptions
Align with tone, brand voice, and regional nuances
Automate A/B testing and SEO optimization
3. AI Coding Assistants for Developer Teams
Context-aware suggestions from internal codebase
Documentation generation, test script creation
Debugging assistant with natural language inputs
4. AI Financial Analysts for Enterprise
Generate earnings summaries, budget predictions
Parse and summarize internal spreadsheets
Draft financial reports with integrated charts
5. Legal Document Intelligence
Draft NDAs, contracts based on templates
Highlight risk clauses
Translate legal jargon to plain language
6. Enterprise Knowledge Assistants
Index all internal documents, chat logs, SOPs
Let employees query processes instantly
Enforce role-based visibility
Challenges in Generative AI Platform Development
Despite the promise, building a generative AI platform isn’t plug-and-play. Key challenges include:
Data quality and labeling: Garbage in, garbage out.
Latency in RAG systems: Slow response times affect UX.
Model hallucination: Even with context, LLMs can fabricate.
Scalability issues: From GPU costs to query limits.
Privacy & compliance: Especially in finance, healthcare, legal sectors.
What’s New in 2025?
Private LLMs: Enterprises increasingly train or fine-tune their own models (via platforms like MosaicML, Databricks).
Multi-Agent Systems: Agent networks are collaborating to perform tasks in parallel.
Guardrails and AI Policy Layers: Compliance-ready platforms with audit logs, content filters, and human approvals.
Auto-RAG Pipelines: Tools now auto-index and update knowledge bases without manual effort.
Conclusion
Generative AI platform development in 2025 is not just about building chatbots—it's about creating intelligent ecosystems that plug into your business, speak your data, and drive real ROI. With the right architecture, frameworks, and enterprise-grade controls, these platforms are becoming the new digital workforce.
0 notes
Text
Top 5 Tools Every Digital Marketer Should Use in 2025
The digital marketing landscape is evolving rapidly, and staying ahead requires more than just strategy—you need the right tools in your tech stack. Whether you’re a freelancer, agency owner, or marketing enthusiast, using effective tools can streamline your workflow, improve results, and give you a competitive edge.
In this blog, we’ll cover the Top 5 digital marketing tools every marketer should be using in 2025 to stay productive, creative, and data-driven.
1. SEMrush – For SEO & Competitive Analysis
Search Engine Optimization remains a cornerstone of digital marketing success, and SEMrush continues to be a powerhouse in 2025.
Why SEMrush?
Conduct keyword research & track rankings
Spy on competitors’ websites & ad campaigns
Audit your site for SEO issues
Plan content based on data-driven insights
Pro Tip: Use SEMrush's Content Marketing Toolkit to discover trending topics, optimize blog posts, and generate SEO-friendly outlines.
🔗 Visit SEMrush
2. Canva Pro – For Graphic Design & Branding
Visual content is king—and Canva Pro makes it easy for non-designers to create professional-quality graphics.
Why Canva Pro?
Drag-and-drop interface for social media posts, presentations, ads, etc.
Access to thousands of premium templates, fonts, and images
Brand Kit feature to maintain visual consistency
Video editing tools included
Pro Tip: Batch-create content using Canva’s Magic Resize and Content Planner for consistent posting across platforms.
🔗 Visit Canva
3. MailerLite – For Email Marketing Automation
Email marketing continues to deliver one of the highest ROIs—and MailerLite is the go-to choice for modern marketers in 2025.
Why MailerLite?
Clean, user-friendly interface
Advanced automation workflows
Drag-and-drop email builder
Landing page and form builder included
Pro Tip: Set up welcome sequences and lead nurturing workflows to convert more subscribers into customers.
🔗 Visit MailerLite
4. ClickUp – For Project & Task Management
If you’re juggling multiple campaigns, clients, or platforms, ClickUp is a lifesaver. It’s one of the best project management tools in the digital space.
Why ClickUp?
Organize work with tasks, boards, and timelines
Set goals, priorities, and deadlines
Collaborate with team members in real-time
Track progress with built-in analytics
Pro Tip: Use the "Docs" and "Content Calendar" features to streamline blog and social media planning.
🔗 Visit ClickUp
5. ChatGPT (by OpenAI) – For Content Creation & Strategy
AI is no longer the future—it’s now. ChatGPT helps marketers brainstorm ideas, write content, generate captions, and even plan campaigns faster.
Why ChatGPT?
Write blogs, email drafts, ad copy, and captions in seconds
Create SEO-friendly outlines and CTAs
Get data insights or industry trends explained in simple terms
Available as browser tools, mobile apps, and Chrome extensions
Pro Tip: Use custom GPTs or prompt templates to streamline repetitive tasks like weekly newsletter generation or social caption writing.
🔗 Try ChatGPT
Final Thoughts
Digital marketing success in 2025 isn’t about doing more—it’s about doing smarter. With these tools in your toolbox, you can automate repetitive tasks, deliver higher-quality content, and drive better results across all channels.
🎯 Want to learn how to implement these tools for your business growth? 👉 Check out our digital services at Digital With Sayed Afjal and let us help you get started the right way.
1 note
·
View note
Text
Mastering the Future: Why an Online Generative AI Course is Your Gateway to the Next Tech Revolution
In the blink of an eye, artificial intelligence has evolved from a futuristic concept to an everyday reality. From personalized recommendations on Netflix to AI-generated artwork and code, we are witnessing a transformation across industries driven by one particular branch of AI—Generative AI.
This groundbreaking technology has opened up opportunities not just for researchers and tech giants, but for everyday learners, professionals, and entrepreneurs. And as demand for skilled professionals surges, enrolling in an online generative AI course has become one of the smartest career moves you can make today.
What is Generative AI, and Why Is It a Big Deal?
Generative AI refers to AI systems that can generate text, images, audio, code, video, and even synthetic data. Unlike traditional AI that analyzes or predicts based on existing data, generative AI creates. Models like OpenAI’s GPT-4, DALL·E, Google’s Gemini, and Adobe Firefly have already made waves, and we’re only scratching the surface.
These tools are being rapidly integrated into content creation, marketing, customer service, healthcare, education, gaming, and design—bringing automation, creativity, and efficiency together in unprecedented ways.
The implications are massive:
Writers can co-create with AI.
Developers can generate code snippets.
Designers can produce prototypes in seconds.
Businesses can automate entire processes.
In short, generative AI is not just a trend; it’s a revolution.
Why an Online Generative AI Course Is the Perfect Launchpad
You may be wondering—why go for an online course instead of a traditional classroom format? The answer lies in accessibility, flexibility, and industry relevance.
Here’s why an online generative AI course is the smartest move in 2025:
1. Learn From Anywhere, Anytime
With remote work and digital learning on the rise, online education has become not just acceptable but preferred. Whether you're a student, a working professional, or a startup founder, an online course allows you to upskill without disrupting your routine.
2. Hands-On, Project-Based Learning
Top-tier online courses in generative AI go beyond theory. They offer:
Real-world projects using GPT, Stable Diffusion, or MidJourney
Python-based implementations of transformer models
Prompt engineering techniques
Ethics and safety modules for responsible AI usage
You’re not just learning—you’re building.
3. Learn from Industry Experts
The best online courses bring in AI practitioners from top tech companies who’ve built or deployed real-world generative AI systems. You gain insights from those who’ve been there, done that.
4. Fast-Track to High-Paying Jobs
According to a 2024 report by PwC, jobs involving AI skills are expected to grow by 37% over the next three years. Roles like:
AI Prompt Engineer
Generative AI Specialist
AI Product Manager
Machine Learning Engineer
…are in high demand across sectors.
Why Bangalore is Becoming the Generative AI Capital of India
While generative AI is a global trend, Bangalore is quickly becoming its Indian hub. Known as India’s Silicon Valley, the city houses top AI startups, innovation labs, and tech giants like Google, Amazon, Microsoft, and Infosys.
There’s a massive push in Bangalore toward adopting and innovating in AI, with generative models taking center stage in R&D and application development.
Enrolling in a generative AI course in Bangalore can offer unique benefits:
Networking with professionals in the AI ecosystem
Workshops and meetups in cutting-edge AI applications
Internship and placement support from top AI startups
Whether you’re based in Bangalore or plan to relocate, taking a course that’s plugged into this ecosystem can give you a serious edge.
Who Should Take a Generative AI Course?
The beauty of generative AI lies in its cross-domain applicability. It’s not just for data scientists. You should consider an online course if you’re:
A Developer: Want to integrate GPT APIs or build AI-driven apps
A Marketer: Looking to leverage AI for content, SEO, and campaign generation
A Designer: Interested in AI-based image or video generation
A Writer or Content Creator: Want to co-write with AI or automate content
A Business Owner: Looking to implement AI in customer service or product recommendations
A Student or Career Switcher: Interested in entering the world of AI with hands-on experience
What to Look For in an Online Generative AI Course
Not all courses are created equal. To truly master this field, make sure your chosen course offers:
Foundational theory (NLP, transformers, diffusion models)
Hands-on projects with ChatGPT, DALL·E, or Google Gemini
Prompt engineering strategies
Real-world use cases in healthcare, marketing, education, etc.
Live mentorship and community support
Certification recognized by the industry
Many reputed institutes are now offering structured courses in both online and offline formats—especially in cities like Bangalore.
The Future is Generative—Are You Ready?
We’re at a pivotal point in tech history where creativity and computation have merged like never before. Generative AI is already changing the way we work, create, and think.
Whether you're building a career, launching a startup, or looking to future-proof your job, now is the time to invest in a generative AI course. And with the flexibility of an online generative AI course, you can start your journey right from the comfort of your home.
If you're in India—or aiming to be in the heart of its AI revolution—consider enrolling in a generative AI course in Bangalore to tap into a thriving community of innovators, developers, and dreamers.
Final Thoughts
The next tech revolution won’t be televised—it will be generated.
Generative AI is not just a skill; it’s a mindset shift. It enables you to go from consumer to creator, from idea to innovation. And the best way to get started? Take that first step with a course that empowers you to build, experiment, and lead in this bold new era.
0 notes
Text
The Pen is Now the Processor
In the ever-evolving landscape of technology, artificial intelligence (AI) has emerged as a formidable force, reshaping industries and redefining the boundaries of creativity. One of the most intriguing developments is the advent of AI-generated content—where machines craft everything from articles and advertisements to art and music. This phenomenon, often referred to as "AI-Generation," is not just a fleeting trend but a transformative shift that's here to stay.
The Emergence of AI-Generated Content
AI-generated content refers to material produced by algorithms and machine learning models without direct human authorship. These systems analyze vast datasets, learn patterns, and generate content that mimics human creativity. The applications are vast:
Marketing: AI tools like ChatGPT and Jasper are used to draft emails, social media posts, and product descriptions.
Journalism: News agencies employ AI to generate reports on financial earnings, sports summaries, and weather updates.
Art and Design: Platforms like DALL·E and Midjourney create visual art based on textual prompts.
Music: AI composers generate original music tracks for various genres and moods.
The Mechanics Behind AI-Generation
At the heart of AI-Generation are advanced machine learning models, particularly those based on deep learning and natural language processing (NLP). These models are trained on extensive datasets comprising text, images, audio, and more. By recognizing patterns and structures within this data, AI can generate new content that aligns with the learned styles and formats.
For instance, GPT-4, developed by OpenAI, is a language model trained on diverse internet text. It can generate coherent and contextually relevant text, making it a powerful tool for content creation.
The Pros of AI-Generated Content
1. Efficiency and Speed
AI can produce content at an unprecedented pace. While a human writer might take hours to draft an article, AI can generate similar content in minutes. This efficiency is invaluable for businesses needing rapid content turnover.
"AI provides almost immediate results for speedy content creation, no matter if the subject is simple or complicated." — HubSpot
2. Cost-Effectiveness
Employing human writers can be expensive, especially for large-scale content needs. AI offers a cost-effective alternative, reducing the need for extensive human labor.
"AI can make content at a fraction of the cost of a full-time writer or freelancer." — Analytics Insight
3. Consistency and Scalability
AI ensures a consistent tone and style across all content, which is crucial for brand identity. Moreover, it can scale content production to meet growing demands without compromising quality.
4. SEO Optimization
AI tools can analyze trending keywords and optimize content for search engines, enhancing visibility and reach.
"AI-generated content's SEO-related capabilities are one of the software's key selling points." — HubSpot
5. Overcoming Writer's Block
AI can assist writers by generating ideas, outlines, or even entire drafts, serving as a valuable tool to overcome creative hurdles.
"AI tools can create detailed outlines and key points to help the writer determine what should be included in the article." — TechTarget
The Cons of AI-Generated Content
1. Lack of Creativity and Originality
While AI can mimic human writing, it often lacks genuine creativity and the ability to produce truly original ideas.
"AI lacks that human touch, that spark of creativity that turns a good piece of content into a great one." — Toxigon
2. Quality and Accuracy Concerns
AI-generated content may contain inaccuracies or lack depth, especially on complex or nuanced topics.
"AI tools may misinterpret data or produce content with grammatical errors and awkward phrasing." — Zoho Social
3. Ethical and Legal Issues
The use of AI in content creation raises concerns about plagiarism, copyright infringement, and the authenticity of the content.
"AI algorithms may inadvertently replicate existing content without proper attribution, leading to intellectual property disputes." — Zoho Social
4. Emotional Intelligence Limitations
AI struggles to infuse content with emotion, empathy, or cultural nuances, which are often essential for engaging storytelling.
"AI-generated content may miss the subtlety and empathy that human writers can infuse into their work." — WebAllWays
5. Dependence on Data Quality
AI's output is only as good as the data it's trained on. Biased or low-quality data can lead to flawed content.
"Poor-quality or biased data can result in flawed content that perpetuates misinformation or reinforces stereotypes." — Zoho Social
Real-World Applications and Impacts
Fashion Industry
The fashion world is embracing AI-generated models for marketing campaigns. Brands like H&M are using AI-created "digital twins" to showcase their products, offering speed and customization. However, this shift has sparked debates about consent, job displacement, and the authenticity of creative work.
"The fashion industry is increasingly embracing AI-generated digital models, exemplified by H&M’s announcement to use AI-created 'digital twins' for marketing." — Vogue Business
Journalism
AI's role in journalism is growing, with tools generating reports and summaries. While this enhances efficiency, it also raises concerns about the exploitation of journalistic content and the spread of misinformation.
"Media organisations across Europe are urgently calling for stronger protections against the exploitation of journalism by artificial intelligence (AI) systems." — The Sun
Art and Creativity
Artists are exploring AI as a tool for creativity, blending traditional techniques with AI-generated elements. This fusion opens new avenues for artistic expression but also challenges notions of originality and authorship.
"The article explores the complex and evolving relationship between artificial intelligence (AI) and art, debating whether there is a 'right' way to use AI in creative work." — The Verge
The Future of AI-Generated Content
As AI continues to evolve, its role in content creation will expand. However, the future will likely involve a symbiotic relationship between humans and AI, where AI handles repetitive tasks, and humans focus on creativity and critical thinking.
"We need to be thinking about things like regulation, and transparency. Should AI have to disclose that it's AI? Who's responsible when AI makes a mistake?" — Toxigon
In conclusion AI-Generation is transforming the content creation landscape, offering efficiency, scalability, and new creative possibilities. However, it also presents challenges that require careful consideration. By understanding the strengths and limitations of AI-generated content, we can harness its potential while preserving the human elements that make content truly resonate.
0 notes
Text
The Ultimate Guide to AI Agent Development for Enterprise Automation in 2025
In the fast-evolving landscape of enterprise technology, AI agents have emerged as powerful tools driving automation, efficiency, and innovation. As we step into 2025, organizations are no longer asking if they should adopt AI agents—but how fast they can build and scale them across workflows.
This comprehensive guide unpacks everything you need to know about AI agent development for enterprise automation—from definitions and benefits to architecture, tools, and best practices.

🚀 What Are AI Agents?
AI agents are intelligent software entities that can autonomously perceive their environment, make decisions, and act on behalf of users or systems to achieve specific goals. Unlike traditional bots, AI agents can reason, learn, and interact contextually, enabling them to handle complex, dynamic enterprise tasks.
Think of them as your enterprise’s digital co-workers—automating tasks, communicating across systems, and continuously improving through feedback.
🧠 Why AI Agents Are Key to Enterprise Automation in 2025
1. Hyperautomation Demands Intelligence
Gartner predicts that by 2025, 70% of organizations will implement structured automation frameworks, where intelligent agents play a central role in managing workflows across HR, finance, customer service, IT, and supply chain.
2. Cost Reduction & Productivity Gains
Enterprises using AI agents report up to 40% reduction in operational costs and 50% faster task completion rates, especially in repetitive and decision-heavy processes.
3. 24/7 Autonomy and Scalability
Unlike human teams, AI agents work round-the-clock, handle large volumes of data, and scale effortlessly across cloud-based environments.
🏗️ Core Components of an Enterprise AI Agent
To develop powerful AI agents, understanding their architecture is key. The modern enterprise AI agent typically includes:
Perception Layer: Integrates with sensors, databases, APIs, or user input to observe its environment.
Reasoning Engine: Uses logic, rules, and LLMs (Large Language Models) to make decisions.
Planning Module: Generates action steps to achieve goals.
Action Layer: Executes commands via APIs, RPA bots, or enterprise applications.
Learning Module: Continuously improves via feedback loops and historical data.
🧰 Tools and Technologies for AI Agent Development in 2025
Developers and enterprises now have access to an expansive toolkit. Key technologies include:
🤖 LLMs (Large Language Models)
OpenAI GPT-4+, Anthropic Claude, Meta Llama 3
Used for task understanding, conversational interaction, summarization
🛠️ Agent Frameworks
LangChain, AutoGen, CrewAI, MetaGPT
Enable multi-agent systems, memory handling, tool integration
🧩 Integration Platforms
Zapier, Make, Microsoft Power Automate
Used for task automation and API-level integrations
🧠 RAG (Retrieval-Augmented Generation)
Enables agents to access external knowledge sources, ensuring context-aware and up-to-date responses
🔄 Vector Databases & Memory
Pinecone, Weaviate, Chroma
Let agents retain long-term memory and user-specific knowledge
🛠️ Steps to Build an Enterprise AI Agent in 2025
Here’s a streamlined process to develop robust AI agents tailored to your enterprise needs:
1. Define the Use Case
Start with a clear objective. Popular enterprise use cases include:
IT support automation
HR onboarding and management
Sales enablement
Invoice processing
Customer service response
2. Choose Your Agent Architecture
Decide between:
Single-agent systems (for simple tasks)
Multi-agent orchestration (for collaborative, goal-driven tasks)
3. Select the Right Tools
LLM provider (OpenAI, Anthropic)
Agent framework (LangChain, AutoGen)
Vector database for memory
APIs or RPA tools for action execution
4. Develop & Train
Build prompts or workflows
Integrate APIs and data sources
Train agents to adapt and improve from user feedback
5. Test and Deploy
Run real-world scenarios
Monitor behavior and adjust reasoning logic
Ensure enterprise-grade security, compliance, and scalability
🛡️ Security, Privacy, and Governance
As agents operate across enterprise systems, security and compliance must be integral to your development process:
Enforce role-based access control (RBAC)
Use private LLMs or secure APIs for sensitive data
Implement audit trails and logging for transparency
Regularly update models to prevent hallucinations or bias
📊 KPIs to Measure AI Agent Performance
To ensure ongoing improvement and ROI, track:
Task Completion Rate
Average Handling Time
Agent Escalation Rate
User Satisfaction (CSAT)
Cost Savings Per Workflow
🧩 Agentic AI: The Future of Enterprise Workflows
2025 marks the beginning of agentic enterprises, where AI agents become core building blocks of decision-making and operations. From autonomous procurement to dynamic scheduling, businesses are building systems where humans collaborate with agents, not just deploy them.
In the near future, we’ll see:
Goal-based agents with autonomy
Multi-agent systems negotiating outcomes
Cross-department agents driving insights
🏁 Final Thoughts: Start Building Now
AI agents are not just another automation trend—they are the new operating layer of enterprises. If you're looking to stay competitive in 2025 and beyond, investing in AI agent development is not optional. It’s strategic.
Start small, scale fast, and always design with your users and business outcomes in mind.
📣 Ready to Develop Your AI Agent?
Whether you're automating workflows, enhancing productivity, or creating next-gen customer experiences, building an AI agent tailored to your enterprise is within reach.
Partner with experienced AI agent developers to move from concept to implementation with speed, security, and scale.
0 notes
Text
Why a Generative AI Proof of Concept Matters for Your Business
Generative AI is reshaping how companies ideate, design, and engage with data. But before pouring resources into a full-scale deployment, a targeted generative ai proof of concept is invaluable. By launching a small yet strategic pilot, organizations test assumptions, gather actionable feedback, and gain stakeholders’ confidence in generative AI’s potential. This blog explores why an AI proof of concept is essential, what key benefits it brings, and how to secure the best outcomes.
1. Understanding the Value of Generative AI
Generative AI employs models—like GPT or other transformer-based architectures—that produce new content, whether text, designs, or data expansions. Beyond chatbots, it can automatically generate marketing copy, design prototypes, or even synthetic data for training. By harnessing generative AI, businesses can accelerate innovation without manual overhead, spurring new solutions in a fraction of conventional timelines.
Shortened Development Cycles: Need new product concepts? Generative models can produce design drafts or architecture outlines.
Creative Enhancements: Marketing teams use AI to craft ad variations or unique brand visuals quickly.
Data Synthesis: In regulated settings, synthetic data helps train or test models while protecting real data privacy.
2. Why a Proof of Concept is Essential
2.1 Risk Reduction
Despite its promise, generative AI is no silver bullet. A small-scale generative ai proof of concept confirms the technology fits your domain and data constraints. Early detection of issues—like data biases or performance stalls—lets you pivot with minimal loss rather than making major revisions post-implementation.
2.2 Visible Results for Stakeholders
Senior management often wants evidence of ROI. A well-defined PoC can show how generative AI shortens content creation cycles or spurs new product prototypes, offering direct numbers—like time saved or conversions boosted—to justify further investment.
2.3 Incremental Learning
Users might need training to handle generative outputs responsibly. A pilot fosters this learning environment, teaching teams about reviewing AI suggestions, verifying outputs for correctness, and refining the model if it veers off-topic or context.
3. Key Steps in a Generative AI Proof of Concept
Define Narrow Objectives: Pick one scenario—like auto-generating marketing slogans or summarizing lengthy documents. A narrower scope ensures the PoC remains manageable and yields fast insights.
Assess Data Requirements: Determine if textual data, domain-specific knowledge, or design assets need curating. Generative AI thrives on large, relevant datasets; incomplete or mismatched data undermines results.
Model Selection: While large language models (LLMs) are popular, consider specialized frameworks if your use case demands image generation or technical domain understanding.
Build & Test: Train the model, define prompts or input structures, then test with real data. Measure generation quality, user acceptance, and system performance.
Refine & Validate: Gather feedback from end-users or domain experts. Adjust model parameters, augment training data, or create structured prompts for better output reliability.
4. Advantages of a Successful Pilot
4.1 Operational Efficiency
If the PoC focuses on content generation, employees gain freed hours from mundane tasks—like drafting email campaigns or product descriptions. Reinvest these hours in higher-value strategy or creativity.
4.2 Rapid Innovation
Demonstrating that generative AI can produce workable prototypes or content quickly encourages broader experimentation. For instance, engineering teams may soon apply the same AI to internal code documentation or product FAQ generation.
4.3 Enhanced Data Utilization
Generative models feed on domain data, turning it into new, context-rich outputs. This not only maximizes data usage but can reveal hidden patterns or opportunities that static analytics might miss.
5. Addressing Common Concerns
Content Accuracy: Generative outputs risk “hallucination,” or producing factually incorrect statements. Thorough review cycles or layered editorial checks mitigate misinformation.
Ethical & Compliance: If AI accesses proprietary or user data, enforce privacy regulations—like anonymizing inputs or restricting sensitive content generation.
Sustained Maintenance: Generative models need re-training as brand styles, user preferences, or product lines evolve. Plan ongoing updates or integrate real-time user feedback loops.
6. Moving from PoC to Full Rollout
A positive pilot often raises the question: “What’s next?” Key considerations for scaling generative AI:
Integration: Connect the AI solution to existing content management systems or design platforms, enabling seamless generation.
Governance: Adhere to brand tone guidelines or regulatory frameworks, especially in sensitive or public-facing content.
Continuous Training: Set up data pipelines that feed new samples or user corrections back into the training cycle, continually refining model quality.
Conclusion
Adopting generative AI can leapfrog conventional methods in design, content creation, or even data synthesis. Yet ensuring alignment with real-world needs is crucial. A focused generative ai proof of concept validates feasibility, builds organizational buy-in, and charts the best path forward. By clarifying project goals, refining data, and structuring user feedback, businesses can confidently scale generative AI—transforming how they innovate, communicate, and compete in an increasingly data-driven marketplace.
0 notes