#graph datascience
Explore tagged Tumblr posts
Text
How to Become a Data Scientist as a CSE Student?
If you’re a CSE (Computer Science and Engineering) student, chances are you’ve already heard the term data science quite a few times. It’s one of the fastest-growing career fields in tech—and for good reason. Companies across the world rely on data to make decisions, and they need skilled people who can make sense of all that information.
So, how do you become a data scientist when you’re still a student? Let’s break it down step by step.
Understand What a Data Scientist Really Does
A data scientist is someone who collects, cleans, and analyzes large amounts of data to help businesses or organizations make smarter decisions. This can involve writing code, using math, working with databases, and communicating findings clearly.
It’s not just about writing programs—it’s also about thinking critically and solving real-world problems with the help of data.
Step 1: Get Your Basics Right
As a CSE student, you already have a head start. Your curriculum likely includes core subjects like programming, algorithms, databases, and computer networks. Pay close attention to:
Python or R (Python is more widely used in data science)
SQL for handling data in databases
Math and Statistics, especially probability, linear algebra, and basic statistics
Data Structures and Algorithms for handling data efficiently
These skills form the foundation of data science work.
Step 2: Learn Data Science Tools and Libraries
Once you’re comfortable with basic programming and math, it’s time to explore tools used by data scientists. You don’t need to master everything at once—start small.
NumPy and Pandas (for handling and analyzing data)
Matplotlib and Seaborn (for creating charts and graphs)
Scikit-learn (for machine learning models)
Jupyter Notebooks (for writing and sharing your code easily)
Plenty of free tutorials and YouTube videos can help you practice these.
Step 3: Take Online Courses and Certifications
While your college of engineering and technology in Bhubaneswar may offer some electives or workshops, adding extra courses can boost your learning. Platforms like Coursera, edX, Udemy, and Kaggle offer beginner-friendly courses in data science and machine learning.
Look for beginner tracks with practical projects. Try courses like:
"Introduction to Data Science in Python"
"Machine Learning" by Andrew Ng
"Python for Data Science and AI" by IBM
These certifications can also strengthen your resume later on.
Step 4: Work on Real Projects
Theory is important, but nothing beats hands-on experience. Try simple projects first:
Analyze sales or weather data
Build a movie recommendation system
Predict house prices using public datasets
Visualize COVID-19 data trends
You can find datasets on sites like Kaggle, UCI Machine Learning Repository, or Google Dataset Search. Try to write a short blog post or upload your project to GitHub so others can see your work.
Step 5: Join Internships and Communities
Real-world experience can help you build both skills and confidence. Look for internships related to data analysis, data science, or even software roles that deal with data.
Also, join online communities like:
Kaggle (for competitions and discussions)
Reddit’s r/datascience
LinkedIn groups focused on analytics
Discord or Telegram groups for peer learning
You’ll learn a lot from seeing what others are doing and sharing your progress too.
Step 6: Build a Portfolio
Before applying for full-time jobs, put together a portfolio. This can include:
2–3 well-documented projects
A GitHub profile with clean code
A short resume highlighting your tools and skills
A LinkedIn profile that reflects your interests in data science
If you're studying at a college of engineering and technology in Bhubaneswar, consider joining tech fests, project expos, or coding clubs that give you space to present your work.
Conclusions
Becoming a data scientist as a CSE student doesn’t require fancy degrees or expensive tools. What you do need is consistency, curiosity, and a clear plan. Start with your basics, explore tools, build small projects, and grow step by step.
The field of data science is full of opportunities—and your classroom is just the beginning.
#top 5 engineering colleges in bhubaneswar#top engineering colleges in odisha#bhubaneswar b tech colleges#college of engineering and technology bhubaneswar#best colleges in bhubaneswar#college of engineering bhubaneswar
0 notes
Text
🚀 Unlock the Power of Metadata Extraction with Ease!
Tired of manually scraping metadata from websites? Say hello to the Metadata Extractor by Dainty Screw!
✨ What It Does:
This powerful tool extracts essential metadata (like titles, descriptions, keywords, Open Graph tags, and more) from any webpage in just seconds. Whether you’re a marketer, developer, or SEO expert, it’s your go-to for efficient web data extraction.
💡 Why You’ll Love It:
• Accurate: Captures key metadata reliably.
• Fast: Processes pages in lightning speed.
• Customizable: Supports various input options to tailor your scraping needs.
• SEO-Friendly: Perfect for content audits, competitive analysis, or building smarter apps!
🔗 Ready to Try?
Head over to Apify and start extracting data like a pro: Metadata Extractor
🙌 Join the thousands already transforming how they work with metadata.
Your next big idea starts with smarter data extraction. Don’t wait—try it now and let us know your thoughts!
Tags: #WebScraping #MetadataExtractor #SEO #Automation #WebDevelopment #DataScience #OpenGraph
0 notes
Video
youtube
Microsoft GraphRAG Meets Neo4j: A Game-Changer!#TechRevolution #Scientif...
Microsoft GraphRAG Meets Neo4j: A Game-Changer!" highlights the revolutionary integration of Microsoft GraphRAG with Neo4j, transforming data analytics and relationship mapping. Microsoft GraphRAG, known for its robust graph-based AI capabilities, now seamlessly collaborates with Neo4j, the leading graph database technology. This powerful synergy enhances data connectivity, enabling organizations to uncover complex relationships and insights with unprecedented efficiency. By combining Microsoft’s advanced AI with Neo4j's scalable graph database, users can achieve superior data modeling, real-time analytics, and predictive intelligence. This integration paves the way for innovative applications across various industries, setting a new standard in data-driven decision-making and knowledge discovery
#MicrosoftGraphRAG #Neo4j #GraphDatabase #DataAnalytics #AI #BigData #DataScience #MachineLearning #PredictiveAnalytics #DataVisualization #TechInnovation #KnowledgeDiscovery #ScienceFather #Innovation #Research #STEM #ScienceConference #TechConference #Researcher #ScientificResearch #AcademicResearch #Science #STEM #Innovation #ResearchCommunity #PhDLife #LabLife #ResearchAndDevelopment #R&D #ScienceMatters #ResearchImpact #DataScience #TechResearch #ScientificDiscovery #ResearchLab #ResearcherLife #FieldResearch #ScienceCommunication #Scholar #PostDoc #ResearchProjects #ScienceInnovation #FutureOfResearch #AcademicLife #ResearchFunding #ResearchCollaboration #ScienceIsAwesome #ScientificInnovation
International Young Scientist Awards
Website link: youngscientistawards.com
Nomination Link : https://x-i.me/suwyou5
Contact Us: [email protected]
Social Media:
Twitter : https://twitter.com/youngsc06963908
Linkedin- : https://www.linkedin.com/in/shravya-r...
Pinterest : https://in.pinterest.com/youngscienti...
Blog : https://youngscientistaward.blogspot....
Tumblr : https://www.tumblr.com/blog/shravya96
0 notes
Text
Deconstructing Data Roles: Scientist, Analyst, and Researcher
Professionals with the ability to infer inferences from data are in great demand in the big data era. However, technical terms employed in data-related fields might lead to miscommunications.Using knowledge from Pune's Best DataScience Course, let's investigate the primary reasons behind people's fascination with data science.
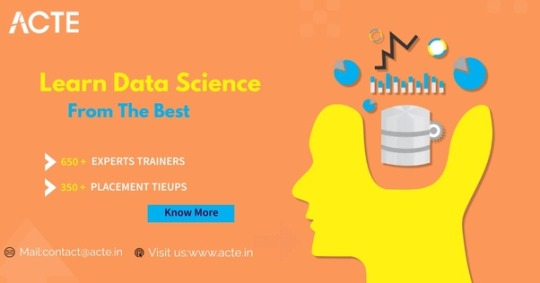
Data Researcher An explorer in the vast realm of data is what a researcher is like. Their primary duty is to collect and organize data from various sources. Think of them as information gatherers who look through datasets, databases, and other sources to find relevant content.
Primary Responsibility
1. Data Collection: They look through a range of sources, such as websites, surveys, research papers, and databases, to obtain raw data. 2. Data Cleaning: Raw data, which is often chaotic, must be cleaned and structured before analysis can begin. Data researchers are in charge of this initial cleanup. 3. Data exploration: Prior to diving into in-depth analysis, they conduct preliminary research to understand the structure, patterns, and potential insights within the data. 4. Documentation: Keeping thorough records of the procedures and data sources
Data Analyst:
Once the data has been gathered and cleaned up, the data analyst ought to take over. Data analysts go through data to identify important trends, patterns, and insights, much like detectives do. Because they provide certificates and job placement options, I heartily recommend the Datascience Online Training if you're interested in learning more about data science. These services are available both offline and online.
Primary Responsibility
1. Data Interpretation: Data analysts use statistical techniques and visualization tools to identify trends and glean insights from data. 2. Report Generation: Often with the aid of tables, graphs, and charts, they compile their findings into concise and understandable reports and dashboards. 3. Visualization: When complicated information is presented visually, stakeholders can understand it more easily. Data analysts are adept at visualizing data using tools like Tableau, Power BI, and Python libraries like Matplotlib and Seaborn. 4. Data-oriented Suggestions: Based on their research, data analysts make recommendations.
Data Scientist:
The wizards of the data realm are data scientists. They can tackle complex problems because they have a special combination of subject knowledge, statistical experience, and programming skills.
Primary Responsibility
1. Advanced Analytics: Data scientists use state-of-the-art statistical techniques and machine learning algorithms to extract patterns and forecasts from data.
2. Model Development: They build and enhance predictive models to solve specific business problems such as demand forecasting or customer churn prediction. 3. Algorithm Implementation: Data scientists write code to implement models and algorithms using Python or R, among other computer languages. 4. Cross-functional Collaboration: Data scientists work closely with stakeholders from all departments to understand business objectives and align data-driven solutions with organizational goals.
0 notes
Text
Starting Your Data Science Adventure: Beginner-Friendly Tips and Resources
In today's data-driven world, Python programming has emerged as a powerhouse for data science. If you're curious about diving into this exciting field, you're in the right place! Whether you're a complete beginner or have some programming experience, this guide will equip you with the essential tips and resources to start your data science adventure with Python.
1. Why Python for Data Science?
Python has become a go-to language for data science due to its simplicity and versatility. It offers a wide range of libraries and frameworks tailored for data analysis, visualization, and machine learning. Plus, its readability makes it a favorite among beginners and seasoned professionals alike.
2. Getting Started with Python
If you're new to Python, don't worry! Getting started is easier than you might think. Begin by installing Python on your computer. You can download it for free from the official Python website (python.org). Choose the latest stable version, and follow the installation instructions for your operating system.
3. Understanding Python Basics
Before delving into data science, familiarize yourself with Python's basic syntax and principles. Online platforms like Codecademy and Coursera offer free introductory Python courses. These courses cover fundamental topics such as variables, data types, loops, and functions. Remember, a solid foundation in Python will serve as a strong pillar for your data science journey.
4. Exploring Data Science Libraries
One of Python's strengths lies in its vast array of libraries designed for data science. Three essential libraries you'll encounter frequently are:
NumPy: For numerical computing and handling arrays.
Pandas: Ideal for data manipulation and analysis, especially with tabular data.
Matplotlib & Seaborn: These libraries excel in data visualization, helping you create insightful plots and graphs.
5. Hands-On Projects
The best way to learn is by doing! Start with small data science projects to apply your Python skills. For instance, you could:
Analyze a dataset of your favorite movies, exploring trends and ratings.
Predict housing prices using a dataset from platforms like Kaggle.
Classify images of handwritten digits using machine learning algorithms.
6. Online Courses and Tutorials
Numerous online resources offer comprehensive data science courses with a focus on Python. Websites like Udemy, DataCamp, and edX host courses ranging from beginner to advanced levels. These courses often include video lectures, coding exercises, and real-world projects to reinforce your learning.
7. Joining Data Science Communities
Immerse yourself in the vibrant data science community! Platforms like Stack Overflow and Reddit's r/datascience are excellent places to ask questions, seek advice, and connect with fellow learners and professionals. Participating in discussions and reading others' experiences can provide valuable insights and motivation.
8. Books for Data Science with Python
Books are timeless resources for learning. Consider adding these titles to your reading list:
"Python for Data Analysis" by Wes McKinney: A comprehensive guide to using Python for data manipulation and analysis with Pandas.
"Hands-On Machine Learning with Scikit-Learn, Keras, and TensorFlow" by Aurélien Géron: Dive into practical machine learning with Python's popular libraries.
9. Practice, Patience, and Perseverance
Data science, like any skill, requires practice and patience. Don't be discouraged by challenges; instead, view them as opportunities to learn and grow. Dedicate time each day to coding and exploring new concepts. Celebrate your progress, no matter how small, and stay curious about the vast possibilities of Python in data science.
Conclusion
Embarking on a data science journey with Python is an exciting and rewarding endeavor. By following these beginner-friendly tips and leveraging the abundant resources available, you'll build a solid foundation for your exploration of data analysis, machine learning, and beyond. Remember, the key ingredients for success are curiosity, persistence, and a willingness to learn. Happy coding!
0 notes
Text
Navigating the Data Science Learning Curve: A Beginner's Unveiling of Secrets
Embarking on the data science learning journey as a beginner might seem daunting, but fret not! With the right approach and resources, you can delve into this exciting field and unlock a world of insights. This blog will outline the optimal way to learn data science in straightforward terms, guiding you through essential steps. Enhancing your career at the Data Science Course in Coimbatore entails taking a systematic strategy and looking into enrolling in a suitable course that will greatly expand your learning journey while harmonizing with your preferences.

BEST WAYS TO LEARN DATA SCIENCE
Step 1: Establish a Strong Foundation in Mathematics and Statistics:
Before venturing into data science, it's crucial to have a robust foundation in basic mathematics and statistics. Concepts like algebra, probability, and descriptive statistics will accompany you throughout this journey.
Step 2: Master the Fundamentals of Programming:
Data science heavily relies on programming, with Python being the preferred language for many. Understanding coding syntax and logic is a key skill that will empower you in handling data efficiently. For individuals eager to excel in Data Science, exploring Data Science Online Training is highly recommended. Look for classes that align with your preferred programming language and learning approach.
Step 3: Engage in Hands-On Data Exploration:
The essence of data science lies in working with data. Initiate your hands-on experience by exploring datasets on platforms like Kaggle. Kaggle provides a collaborative environment where you can access datasets, participate in competitions, and learn from others. This practical exposure will help you grasp the intricacies of manipulating, cleaning, and analyzing data.
Step 4: Learn the Art of Data Visualization:
Data visualization is crucial for presenting data in a visually appealing and understandable way. Tools like Matplotlib and Seaborn in Python can assist in creating insightful visualizations. Understanding how to communicate your findings through graphs and charts is essential for conveying the story behind the data.
Step 5: Dive into the World of Machine Learning:
Machine learning is a core component of data science, enabling you to build predictive models and uncover patterns within data. Begin with introductory courses on platforms like Coursera (Andrew Ng's Machine Learning course is highly recommended) or explore resources like the "Introduction to Machine Learning with Python" book by Andreas C. Müller and Sarah Guido.
Step 6: Immerse Yourself in Data Science Communities:
Connect with the vibrant data science community online. Platforms such as Stack Overflow, Reddit (visit the r/datascience subreddit), and LinkedIn groups serve as excellent places to ask questions, share insights, and learn from experienced professionals.
Step 7: Pursue Specializations:
As you progress, consider specializing in areas aligned with your interests and career goals. Whether it's natural language processing, computer vision, or deep learning, numerous avenues within data science await exploration.

Embarking on the data science learning journey as a beginner is both exciting and rewarding. By establishing a solid foundation in mathematics, mastering programming, engaging in hands-on data exploration, and continuously expanding your knowledge, you'll be well on your way to becoming a proficient data scientist. Remember, persistence and curiosity are your best companions in this ever-evolving field. Happy learning!
If you want to gain knowledge in data science, then you should contact Data Science training in Coimbatore. Experienced teachers can help you learn better. To live life to the fullest and improve your learning process, they provide certification programs and opportunities for job placement overseen by knowledgeable teachers. You can get these resources in person or online. Taking a step-by-step approach and thinking about enrolling in a course could be helpful, if it fits with your interests.
#data science#data science certification#data science course#data science training#data scientist#data science online course
0 notes
Text
The first neural language model, that's Yoshua Bengio, one of the "Godfathers of Deep Learning"! He is widely regarded as one of the most impactful people in natural language processing and unsupervised learning. Here are his main contributions:
- 1994 - Identified the problem of vanishing and exploding gradients in RNN: https://lnkd.in/gNNWDnGG
- 1995 - He applied with Lecun Convolutional Neural Network to the speech and time series learning task: https://lnkd.in/gSJT7rd4
- 1998 - He suggested with LeCun a new CNN architecture (LeNet - Graph Transformer Networks) for the document recognition learning task: https://lnkd.in/gFyijKin
- 2003 - Proposed the first neural language model: https://lnkd.in/gJgngk_K
- 2006 - Proposed a new greedy training method for Deep Networks: https://lnkd.in/g4TfHwKc
- 2008 - Invented the Denoising Autoencoder model: https://lnkd.in/gUvwXGNN
- 2009 Developed a new method called curriculum learning, which is a form of structured learning that gradually exposes models to more difficult examples: https://lnkd.in/gyBirMxN
- 2010 - Proposed a new unsupervised learning technique, Stacked Denoising Autoencoder architecture: https://lnkd.in/gyH5-JTs
- 2010 - Proposed with Glorot the weight initialization technique used in most modern neural nets: https://lnkd.in/g4nqvxzh
- 2011 - Invented the ReLU activation function: https://lnkd.in/gwJsxHYQ
- 2011 - Proposed Bayesian optimization for hyperparameter optimization: https://lnkd.in/geSTQZWU
- 2012 - Proposed the random search technique for hyperparameter optimization: https://lnkd.in/gtx_ABwi
- 2013 - Proposed a unifying perspective on representation learning: https://lnkd.in/gVFU7iUh
- 2013 - Invented the gradient clipping strategy to prevent the vanishing and exploding gradient problem in RNN: https://lnkd.in/gQWkKMYq
- 2013 - Proposed a new activation function for Maxout networks: https://lnkd.in/gWdB72dH
- 2014 - He proposed the RNN encoder-decoder architecture: https://lnkd.in/gnGFsdJe
- 2014 - He proposed with Ian Goodfellow, the Generative Adversarial Networks, a novel class of deep learning models designed to generate synthetic data that resembles real data: https://arxiv.org/pdf/1406.2661.pdf
- 2014 - He proposed the gated recursive convolutional method for machine translation: https://lnkd.in/g-rRQ6km
- 2014 - He invented the Attention mechanism with Bahdanau: https://lnkd.in/g2C3sHjf
- 2014 - Proposed FitNets, a new distillation method to compress Deep NN: https://lnkd.in/gsQy8f8m
- 2014 - Showed the efficacy of transfer learning: https://lnkd.in/g4vfNNUx
- 2015 - Proposed BinaryConnect, a new method for efficient training with binary weights: https://lnkd.in/g-Pg63RT
- 2015 - Proposed a visual Attention mechanism for image caption generation: https://lnkd.in/gbmGDwA2
- 2017 - Proposed a novel architecture with self-attention layers for graph-structured data: https://lnkd.in/gg8tQBtP
- 2017 - Proposed a new convolutional architecture for brain tumor segmentation: https://lnkd.in/gxFuqr53
#machinelearning #datascience #artificialintelligence

0 notes
Text
Meet Concept2Box: Bridging the Gap Between High-Level Concepts and Fine-Grained Entities in Knowledge Graphs A Dual Geometric Approach
📢 Exciting News! Introducing Concept2Box, a Dual Geometric Approach that bridges the gap between high-level concepts and fine-grained entities in knowledge graphs. 🌐🔀 Learn how Concept2Box employs dual geometric representations, using box embeddings for concepts and vector embeddings for entities, enabling the learning of hierarchical structures and complex relationships within knowledge graphs. 📚📊 Discover how this approach addresses the limitations of traditional methods, capturing structural distinctions and hierarchical relationships more effectively. Experimental evaluations on DBpedia and an industrial knowledge graph have shown the remarkable effectiveness of Concept2Box. 💡📈 Grab a coffee and dive deeper into the details here: [Link to Blog Post](https://ift.tt/5uSB73Q) Remember to stay informed about the latest developments and insights from AI Lab Itinai.com by following them on Twitter (@itinaicom). 📣🔑 #knowledgegraphs #datascience #AI #Concept2Box #geometricapproach List of Useful Links: AI Scrum Bot - ask about AI scrum and agile Our Telegram @itinai Twitter - @itinaicom
#itinai.com#AI#News#Meet Concept2Box: Bridging the Gap Between High-Level Concepts and Fine-Grained Entities in Knowledge Graphs – A Dual Geometric Approach#AI News#AI tools#Innovation#itinai#Janhavi Lande#LLM#MarkTechPost#Productivity Meet Concept2Box: Bridging the Gap Between High-Level Concepts and Fine-Grained Entities in Knowledge Graphs – A Dual Geometri
0 notes
Text

The mortgage market has experienced a significant upheaval as interest rates have surged to a 21-year high. A striking comparison reveals the extent of this climb: only last year, the 30-year fixed-rate mortgage stood at 5.45%, while in 2021, it lingered in the more favorable 3% range. This stark contrast underscores the substantial escalation in costs that has unfolded since the Federal Reserve's initiation of rate hikes. This tightening financial landscape has led to a notable decline in applications for home loan refinancing, registering a 2% drop in a week and a substantial 35% decrease compared to the same week in the previous year. A snapshot of the recent market conditions reveals that on August 15, the average rate for the 30-year fixed mortgage reached a staggering 7.26%. This figure stands as the loftiest observed since the preceding November, signifying the ongoing volatility and challenges confronting both prospective homebuyers and those seeking to manage their existing mortgages.
#mortgage #mortgagerates #mortgages #mortgagerate #interestrates #federalreserve #freddiemac #realestate #housingmarket #inflation #housing #finance #dataviz #datavisualization #data #infographic #visualization #graph #chart #datascience #dataanalytics #housingmarket #loans #30yearfixed #dataart #dataisbeautiful #vizalytiq
0 notes
Video
youtube
أهلاً بكم متابعينا الأعزاء، نود أن نشكركم على مشاهدة فيديونا ونأمل أن يكون قد نال إعجابكم. يرجى النقر على زر الإعجاب والاشتراك في القناة ليصلكم كل جديد. https://ift.tt/W1hcD4B لموقع ده بيجاوب على اكتر من سؤال أنا هبدأ بايه ومحتاج اخلص ايه وايه الخطوة اللي بعد دي لانها بيعمل Graph هايل عشان يحدد العلاقات بين الموضوعات وأنت بتتعلم Data science , machine learning #machinelearning #datascience https://ift.tt/swbXkpS نشرك للمقطع هو اكبر دعم ممكن تقدمه لي ... أتمنى أن ينال الشرح أعجابكم ...................................................................... https://ift.tt/v0wWfoQ https://ift.tt/hwEgQ5x شير مشاركة #BIMarabia اشترك في القناة لمتابعة الشروحات الجديدة videos https://www.youtube.com/channel/UCZYaOLTtPmOQX1fgtDFW52Q?sub_confirmation=1 بيم ارابيا http://bimarabia.com/ ❤️ رابط الاشتراك في القناة https://www.youtube.com/channel/UCZYaOLTtPmOQX1fgtDFW52Q?sub_confirmation=1 ❤️ روابط التواصل ✅ انستجرام https://ift.tt/FrWRQ5B وإذا كنت ترغب في دعم القناة، يمكنك شراء لنا فنجان قهوة عبر الرابط التالي: https://ift.tt/Ms0VYSW ✅ الفيسبوك https://ift.tt/owUmV8W ✅ تويتر https://twitter.com/bimarabia ✅ لينكد ان https://ift.tt/w7tbXOu ✅ قناة التلجرام https://t.me/bimarabia1 ✅ الموقع الالكتروني الشخصي https://ift.tt/v0wWfoQ https://ift.tt/iRBPGCe https://ift.tt/Ah0ZSr6 augmented reality https://www.youtube.com/watch?v=-9c5h0X-Kqw&list=PLNMim060_nUKpt2st91YUPa7BqWd0U2eb open source مفتوحة المصدر https://www.youtube.com/watch?v=WNYyejjLa-s&list=PLNMim060_nUK6qoVzrpjLucG_aBNaz_Ny revit workshop ورشة عمل ريفيت https://www.youtube.com/watch?v=-FIZBNN7CLk&list=PLNMim060_nULOxkcpmsGdaRXRnEj7rmGC OPEN STREET MAP https://www.youtube.com/watch?v=QKHnpu5birw&list=PLNMim060_nUJBibv97w-SfqGMjQCDc6xT المدن الذكية https://www.youtube.com/watch?v=1nGsbGafZ6c&list=PLNMim060_nUKxO8GJj5c-9POTgiCfOdmE الاستدامة https://www.youtube.com/watch?v=m_dma-4wOJU&list=PLNMim060_nUKIQ9OEPA5xGjQ471AQyp3F الذكاء الاصطناعي https://www.youtube.com/watch?v=UWmW84ZBrbg&list=PLNMim060_nUJs5lSTwbFK8Pe1BCUPT_EB by عمر سليم - بيم ارابيا
0 notes
Text
DESTINATION : Scatter Plot
ROUTE : Scatter plots (also called scatter graphs, scatter charts, scatter diagrams and scattergrams ) are similar to line graphs. A line graph uses a line on an X-Y axis to plot a continuous function, while a scatter plot uses dots to represent individual pieces of data. In statistics, these plots are useful to see if two variables are related to each other. For example, a scatter chart can suggest a linear relationship (i.e. a straight line).Scatter plot suggesting a linear relationship.
Scatter Plot suggesting a linear relationship
Correlation in Scatter Plots - The relationship between variables is called correlation. Correlation is just another word for “relationship.” For example, how much you weigh is related (correlated) to how much you eat. There are two type of correlation: positive correlation and negative correlation. If data points make a line from the origin from low x and y values to high x and y values the data points are positively correlated, like in the above graph. If the graph starts off with high y-values and continues to low y-values then the graph is negatively correlated. You can think of positive correlation as something that produces a positive result. For example, the more you exercise, the better your cardiovascular health. “Positive” doesn’t necessarily mean “good”! More smoking leads to more chance of cancer and the more you drive, the more likely you are to be in a car accident.
0 notes
Text
💾 Types of Databases you should know! 📊
From RDBMS to Graph & Time-series DBs, choosing the right one is key! Stay tuned for more insights. 🚀
📞 +91 9948861888 | 🌐 www.datascienceschool.in
#Database #DataScience #BigData #SQL #NoSQL
#datascience#machinelearning#datascienceschool#ai#python#data scientist#learndatascience#bigdata#data#database
0 notes
Text
Everyone is going to play - a statistical analysis of FIFA World Cup formats and participation.
Throughout the history of the FIFA World Cup, the number of teams participating has gradually increased. In the first edition of the tournament, held in 1930, only 13 teams participated with no qualifiers held before the tounrament. Each country that played has been invited by FIFA. This number increased to 16 in the 1934 tournament, and then to 24 teams in the 1982 edition. Noticable outlier in the chart below is the 1950 tournament in Switzerland - 15 countries met the requirements to play with Japan unable to participate due to failing to fulfill their payment duty.
If you have watched the World Cup lately, there is a good chance that it has been played by 32 teams in eight groups of four teams each. This format has been in place since 1998, and the tournament has been held in different countries every four years. In total, 64 matches are played during the tournament, with the top two teams of each group advancing to the knockout stage.
In 2026, the Football World Cup is set to be expanded to 48 teams, with the tournament being hosted by Canada, Mexico, and the United States. This will be the first time in the tournament's history that three countries will co-host the event. The tournament is said to be played in three team groups, with a total of 80 matches being played. This format however is not formally approved as of now and FIFA are considering alternative approaches, one of them is dividing the teams into 12 groups of 4, and having the 8 best third-placed teams andvance alongside the top two teams from each group.
The "best third places advancing" wouldn't be a new approach in a World Cup. The 1982-1994 editions were played similarly to how UEFA Euros are played today - 24 teams in 6 groups with 12 teams advancing by being in the top 2 of their group and 4 best third placed team advancing alongside them.
Earlier competitions did not have a set-in-stone approach to deciding how group when two or more teams were tied on points. In the 1958 tournament hosted by Sweden two teams could play against one another in the group stage more than once (!). Three play-offs were played between teams that were tied on points.
Overall, the argument for expansion of the Football World Cup to 48 teams is that it will provide more opportunities for countries to participate in the tournament which may result in a larger and more exciting competition for fans around the world. One of the main criticisms of the expansioln is that it will result in a less competitive and less exciting tournament. With an additional 16 teams participating, there will be more matches and more teams advancing to the knockout stage. This could potentially lead to having to sit through 16 groups of 3 with weaker squads before finally getting to watch the latter stages of the tournament. Sceptics also mention that 3-team would group stage would require one team in each group to watch the other two teams play, instead of participating in a game themselves. In a 4-team group stage, all teams would have the opportunity to play and compete in every matchday, with the last two games being played simultaneously.
Charts made with: Python Seaborn & Matplotlib Modules.
Sources
https://www.espn.com/soccer/fifa-world-cup/story/4825387/fifa-could-abandon-three-team-groups-for-2026-world-cup
https://www.kaggle.com/datasets/piterfm/fifa-football-world-cup?resource=download
https://en.wikipedia.org/wiki/FIFA_World_Cup
https://www.fifa.com/tournaments/mens/worldcup/1958sweden
#worldcup#fifa matchday#fifa wc 2022#fifa world cup#football#soccer#world cup 2022#world cup#data#datascience#datavisualization#dataanalysis#sports#football data#soccer data#python#coding#graph#chart
2 notes
·
View notes
Text
can I physically make a graph in R? obviously. its easy. im not a child i know how to ggplot
emotionally however i cannot handle the toll. its too much. for one graph? importing a whole ass csv and then having to do another thing to plot it? thats two things! forget it.
#niche post niche post niche post#datasci#you gotta have three charts worth for me to consider importing into r otherwise im makin shitty excel graphs
5 notes
·
View notes
Text
Ooh noo

0 notes
Text
Build Knowledge Graph Using Python
Build Knowledge Graph Using Python
What is a Knowledge Graph A Knowledge Graph is a set of data points connected by relations that describe a domain, for instance, a business, an organization, or a field of study. It is a powerful way of representing data because Knowledge Graphs can be built automatically and can then be explored to reveal new insights about the domain. The concept of Knowledge Graphs borrows from the Graph…

View On WordPress
#Data Collection#Dataframe#DataScience#Datasets#Knowledge Graphs#Machine learning#Programming#Python#Wiki Sentence
0 notes