#query engine
Explore tagged Tumblr posts
Text
How AlloyDB AI Query Engine Empower Smart Apps Developers

AlloyDB AI query engine helps developers build smarter apps with quick, intelligent data management and rich insights.
Artificial intelligence and intelligent agents, which can understand commands and queries in natural language and act on their own, are causing major changes. The “AI-ready” enterprise database, a dynamic, intelligent engine that understands the semantics of both structured and unstructured data and leverages foundation models to build a platform that opens up new enterprise data possibilities, is at the heart of this transformation.
Google Cloud Next introduced many new AlloyDB AI technologies this week to speed up intelligent agent and application development. These include autonomous vector index management, high-performance filtered vector search with enhanced semantic search, and improved search quality using the recently announced Vertex AI Ranking API and AlloyDB AI query engine. Also, the AI query engine filters SQL queries with AI-powered operators.
They are adding natural language capabilities to provide people and bots deep insights from natural language searches. These advancements make AlloyDB the foundation of agentic AI, converting it from a database for storing data and conducting SQL queries to one where intelligent agents may interact with the data and conduct autonomous research.
Effective, high-quality, and simple semantic search
Modern apps need smart data retrieval that combines structured and unstructured text and graphics. AlloyDB AI enabled semantic searches over unstructured data and extensively integrated vector search with PostgreSQL to keep search results updated. Google cloud next AlloyDB AI features address customer needs for better search results, faster performance, and cheap autonomous maintenance.
Adaptive filtering, a new mechanism in preview, ensures filters, joins, and vector indexes function effectively together. After learning the genuine filter selectivity as it accesses data, adaptive filtering optimises the query strategy and switches amongst filtered vector search methods.
Vector index auto-maintenance: reduces vector index rebuilds and ensures correctness and performance even when data changes. Vector index auto-maintenance can be enabled while building or editing an index.
The recently unveiled AlloyDB AI query engine may enhance semantic search by combining vector search with high-accuracy AI reranking utilising the new Vertex AI cross-attention Ranking API. After vector search generates initial candidates (like Top N), reranking capability uses the high-quality cross-attention Ranking API to dependably identify the best results (like Top 10). AlloyDB AI can integrate with any third-party ranking API, including bespoke ones, for maximum versatility.
Remember evaluator. This widely available feature provides transparency to manage and improve vector search results. A simple stored procedure may evaluate end-to-end recall for any query, even complex ones like filters, joins, and reranking.
Previously many times that amount, index build parallelisation is now commonly accessible and allows developers to produce 1 billion-row indexes in hours. AlloyDB AI launches parallel processes to distribute the load and build indexes faster.
The deep integration of AlloyDB AI's Scalable Nearest Neighbours (ScaNN) vector index with PostgreSQL's query planner speeds up performance:
10 times quicker filtered vector search than PostgreSQL's HNSW index.
Index building takes ten times less time than PostgreSQL's HNSW index.
Vector search is four times quicker than PostgreSQL's HNSW index.
AI AlloyDB natural language
AI technologies helped natural language interfaces on databases improve in 2024 by converting agent and user requests into SQL queries that give results.
Additional precision requires a quantum leap. Its new capabilities allow you to design interactive natural language user interfaces that effectively comprehend user intent and produce exceptionally accurate mappings from user questions to SQL queries that offer replies, improving on last year's natural language support.
Disambiguation: Natural language is ambiguous. AlloyDB AI natural language interface will ask follow-up questions to gather further user intent data. The database is excellent at resolving ambiguity since it's often embedded in the data.
If a query mentions “John Smith,” the database may include two John Smiths or a “Jon Smith” with a misspelt initial. AlloyDB concept categories and the values index help find relevant entities and ideas when the inquiry is unclear.
High accuracy and intent explanation: AlloyDB AI natural language uses faceted and plain templates that match parameterised SQL queries to answer important and predictable enquiries with almost verified correctness.
The millions of product attributes on a retailer's product search page might overwhelm a screen-based faceted search experience. But even with one simple search field, a faceted search template can handle any query that directly or indirectly raises any combination of property criteria. Additional templates can be provided to expand query coverage beyond those AlloyDB generates from query logs. AlloyDB clearly explains how it understands user queries to ensure results reliability.
In unpredictable questions that require flexible responses, AlloyDB automatically adds rich data from the schema, data (including sample data), and query logs to the context used to map the question to SQL.
Parameterised secure views: AlloyDB's new parameterised secure views restrict database-level access to end-user data to prevent quick injection attacks.
In addition to AlloyDB, Google Agentspace offers AlloyDB AI natural language for creating agents that can reply to questions by combining AlloyDB data with other databases or the internet.
AlloyDB AI query engine
The AlloyDB AI query engine can extract deep semantic insights from corporate data using AI-powered SQL operators, allowing user-friendly and powerful AI applications. AI query engines employ Model Endpoint Management to call any AI model on any platform.
The AlloyDB AI query engine and new AI model-enabled capabilities will be examined:
Artificial intelligence query engine AlloyDB SQL now supports simple but useful AI operators like AI.IF() for filters and joins and AI.RANK() for ordering. These operators use plain language to communicate SQL query ranking and filtering criteria. Cross-attention models, which are strong because of foundation models and real-world information, may bring logic and practical knowledge to SQL queries. For the most relevant results, AI.RANK() can use the Vertex AI Ranking API.
Previous versions of AlloyDB AI made multimodal embeddings from SQL statement text easy for SQL developers. It has expanded this functionality to integrate text, photographs, and videos to provide multimodal search.
Updated text embedding generation: AlloyDB AI query engine integrates Google DeepMind text-embedding creation out of the box.
Beginning
The AlloyDB AI query engine, next-generation natural language support, and better filtered vector search unveiled today provide the framework for future databases, according to Google cloud. AI-ready data gives agents proactive insights to anticipate and act. AlloyDB AI's database revolution will let you boldly join this intelligent future and unlock your data's boundless potential.
#technology#technews#govindhtech#news#technologynews#AI#artificial intelligence#AlloyDB AI query engine#AlloyDB AI#query engine#AlloyDB AI natural language#PostgreSQL#AI query engine#Vertex AI
0 notes
Text
You can add: “filetype:pdf” to a search query and get related pdfs.
Examples:




#pdf#filetype:pdf#Internet tricks#tips#search engin#search queries#advice#skibidi#if this screenshot gets preserved ppl will get mentally flashbanged with “skibidi”
23 notes
·
View notes
Text
TypeScript on the backend is a cardinal sin
How do you see this and go "oh yeah, I want that shit on prod ASAP".
#codeblr#programming#software engineering#progblr#casually spending 5 hours trying to figure out why the delete query builder says it's affecting rows but doesn't do jack#javascript#typescript
85 notes
·
View notes
Text
I see there's a new post on AO3 on AI and data scraping, the contents of which I would describe as a real mixed bag, and the sheer number of comments on it is activating my self-preservation instincts too much for me to subject myself to reading through them. Instead I'm thinking about how much daylight there is between does or doesn't constitute a TOS violation and what does or doesn't violate community norms, and how AO3 finally rolled out that blocking and muting feature recently, and how I think it would be good, actually, if most people's immediate reaction to seeing a work that announces itself as being the product of generative AI was to mute the user who posted it.
That's my reaction, anyway!
#ao3#christ i'm annoyed by so many people lately#i feel the same way about people posting “fic” they generated from a chat-gpt prompt as i would if they posted their google search results#is crafting a good prompt or query a skill? yeah#is it creative work? no#is there a difference between a human being creating based on existing art and a fancy predictive engine spitting out rearrangements?#absolutely#and that's not even getting into the problems with the ml datasets behind the fancy predictive engines#and the way this tech is already being used to decimate creative industries because capitalism privileges the shitty and cheap#or the way companies already generate shitty machine translations and try to pay translators next to nothing to “improve” them#which is typically much more work than starting from scratch#or just run with the shitty machine translation as is because they don't give a fuck if it's useless and confusing to users#anyway i'm real fucking grumpy#please stop trying to automate art and start automating the shit that gets in the way of humans having the time and resources to make art
77 notes
·
View notes
Note
NO! are you trying to reinvent the kotlc food wars in the year of our lord and savior sophie elizabeth foster 2023. wait hang on what does elizabeth mean. okay there's a few different iterations as always with translation, but its got to do with god and oaths. fascinating. please don't drink motor oil, just eat peanut butter if you want the flavor
#quil's queries#dizzeners#need to live up to my dream self and magically appear to revoke your right to motor oil#what are you an automobile?#your engine does not need lubrication#trust me I know about doctors
8 notes
·
View notes
Text
Empowering Digital Explorers: LinkLinkGo.com Journey to Online Safety and Information Access
What is LinkLinkGo.com
Empowering Digital Explorers: LinkLinkGo.com Journey to Online Safety and Information Access.

LinkLinkGo.com is a website dedicated to #helping users search for information on the internet more efficiently. If you're facing difficulties while searching online, you can turn to us for search assistance. Our primary goal is to save your time on the internet while also raising #awareness about cybercrime, cyber security, and cyber fraud. We provide guidance on how to protect yourself from these threats online.
If you encounter complex search queries or need #comprehensive information on a topic, our website is designed for you, and our services are available for free. We offer personalized #assistance to help you find the #information you need.
Our Mission:
Our mission at LinkLinkGo.com is to streamline the process of searching for information on the internet and offer assistance when you need it. We also aim to create awareness about cybercrime, cyber security, and cyber fraud, and we provide guidance on how to safeguard yourself while using the internet.
We are a group of #volunteers who are committed to helping people and sharing our knowledge for the betterment of humanity. Our mission revolves around ensuring people's online safety and providing them with the best possible information.
Story:
Our journey is rooted in the mission of empowering individuals to navigate the digital world safely. We are dedicated to helping users find the information they need while ensuring their online protection.
As a group of volunteers, we recognized the potential of the internet as a powerful tool for connecting people and sharing information. However, we were also aware of the risks posed by cybercrime, cyber fraud, and other forms of cyber threats.
Driven by our passion and expertise, we set out to create LinkLinkGo.com as a resource for internet users. Our website serves as a platform for finding trustworthy information on a wide range of topics while also educating users about cyber security.
Our tireless efforts to curate reliable information and offer guidance on cyber threats paid off as we received positive feedback from users. We were delighted to know that we were making a positive impact on people's lives by helping them navigate the digital world safely.
Through our #website, we can reach people from all backgrounds and assist them in feeling more secure and informed online. Looking ahead, we remain dedicated to our mission of ensuring online safety and #knowledge in the digital era.
Website link = https://www.linklinkgo.com
#linklinkgo.com#Search.linklinkgo.com#Search Engine#Advanced Search#Advanced Search Operators#Google Dork operators#Search Queries#Security Researchers#Web Developers#Sensitive Information#Digital World#Online Protection#Navigating Safely#Trusted Guide
2 notes
·
View notes
Text
Data Engineering Concepts, Tools, and Projects
All the associations in the world have large amounts of data. If not worked upon and anatomized, this data does not amount to anything. Data masterminds are the ones. who make this data pure for consideration. Data Engineering can nominate the process of developing, operating, and maintaining software systems that collect, dissect, and store the association’s data. In modern data analytics, data masterminds produce data channels, which are the structure armature.
How to become a data engineer:
While there is no specific degree requirement for data engineering, a bachelor's or master's degree in computer science, software engineering, information systems, or a related field can provide a solid foundation. Courses in databases, programming, data structures, algorithms, and statistics are particularly beneficial. Data engineers should have strong programming skills. Focus on languages commonly used in data engineering, such as Python, SQL, and Scala. Learn the basics of data manipulation, scripting, and querying databases.
Familiarize yourself with various database systems like MySQL, PostgreSQL, and NoSQL databases such as MongoDB or Apache Cassandra.Knowledge of data warehousing concepts, including schema design, indexing, and optimization techniques.
Data engineering tools recommendations:
Data Engineering makes sure to use a variety of languages and tools to negotiate its objects. These tools allow data masterminds to apply tasks like creating channels and algorithms in a much easier as well as effective manner.
1. Amazon Redshift: A widely used cloud data warehouse built by Amazon, Redshift is the go-to choice for many teams and businesses. It is a comprehensive tool that enables the setup and scaling of data warehouses, making it incredibly easy to use.
One of the most popular tools used for businesses purpose is Amazon Redshift, which provides a powerful platform for managing large amounts of data. It allows users to quickly analyze complex datasets, build models that can be used for predictive analytics, and create visualizations that make it easier to interpret results. With its scalability and flexibility, Amazon Redshift has become one of the go-to solutions when it comes to data engineering tasks.
2. Big Query: Just like Redshift, Big Query is a cloud data warehouse fully managed by Google. It's especially favored by companies that have experience with the Google Cloud Platform. BigQuery not only can scale but also has robust machine learning features that make data analysis much easier. 3. Tableau: A powerful BI tool, Tableau is the second most popular one from our survey. It helps extract and gather data stored in multiple locations and comes with an intuitive drag-and-drop interface. Tableau makes data across departments readily available for data engineers and managers to create useful dashboards. 4. Looker: An essential BI software, Looker helps visualize data more effectively. Unlike traditional BI tools, Looker has developed a LookML layer, which is a language for explaining data, aggregates, calculations, and relationships in a SQL database. A spectacle is a newly-released tool that assists in deploying the LookML layer, ensuring non-technical personnel have a much simpler time when utilizing company data.
5. Apache Spark: An open-source unified analytics engine, Apache Spark is excellent for processing large data sets. It also offers great distribution and runs easily alongside other distributed computing programs, making it essential for data mining and machine learning. 6. Airflow: With Airflow, programming, and scheduling can be done quickly and accurately, and users can keep an eye on it through the built-in UI. It is the most used workflow solution, as 25% of data teams reported using it. 7. Apache Hive: Another data warehouse project on Apache Hadoop, Hive simplifies data queries and analysis with its SQL-like interface. This language enables MapReduce tasks to be executed on Hadoop and is mainly used for data summarization, analysis, and query. 8. Segment: An efficient and comprehensive tool, Segment assists in collecting and using data from digital properties. It transforms, sends, and archives customer data, and also makes the entire process much more manageable. 9. Snowflake: This cloud data warehouse has become very popular lately due to its capabilities in storing and computing data. Snowflake’s unique shared data architecture allows for a wide range of applications, making it an ideal choice for large-scale data storage, data engineering, and data science. 10. DBT: A command-line tool that uses SQL to transform data, DBT is the perfect choice for data engineers and analysts. DBT streamlines the entire transformation process and is highly praised by many data engineers.
Data Engineering Projects:
Data engineering is an important process for businesses to understand and utilize to gain insights from their data. It involves designing, constructing, maintaining, and troubleshooting databases to ensure they are running optimally. There are many tools available for data engineers to use in their work such as My SQL, SQL server, oracle RDBMS, Open Refine, TRIFACTA, Data Ladder, Keras, Watson, TensorFlow, etc. Each tool has its strengths and weaknesses so it’s important to research each one thoroughly before making recommendations about which ones should be used for specific tasks or projects.
Smart IoT Infrastructure:
As the IoT continues to develop, the measure of data consumed with high haste is growing at an intimidating rate. It creates challenges for companies regarding storehouses, analysis, and visualization.
Data Ingestion:
Data ingestion is moving data from one or further sources to a target point for further preparation and analysis. This target point is generally a data storehouse, a unique database designed for effective reporting.
Data Quality and Testing:
Understand the importance of data quality and testing in data engineering projects. Learn about techniques and tools to ensure data accuracy and consistency.
Streaming Data:
Familiarize yourself with real-time data processing and streaming frameworks like Apache Kafka and Apache Flink. Develop your problem-solving skills through practical exercises and challenges.
Conclusion:
Data engineers are using these tools for building data systems. My SQL, SQL server and Oracle RDBMS involve collecting, storing, managing, transforming, and analyzing large amounts of data to gain insights. Data engineers are responsible for designing efficient solutions that can handle high volumes of data while ensuring accuracy and reliability. They use a variety of technologies including databases, programming languages, machine learning algorithms, and more to create powerful applications that help businesses make better decisions based on their collected data.
2 notes
·
View notes
Text
The manipulation of the semantic matching on search queries intrigued me; however, wired has since removed the linked article with the stated reason. Editor’s Note 10/6/2023: After careful review of the op-ed, "How Google Alters Search Queries to Get at Your Wallet," and relevant material provided to us following its publication, WIRED editorial leadership has determined that the story does not meet our editorial standards. It has been removed. You can read it here: https://web.archive.org/web/20231002123158/https://www.wired.com/story/google-antitrust-lawsuit-search-results/ Here's an excerpt: Say you search for “children’s clothing.” Google converts it, without your knowledge, to a search for “NIKOLAI-brand kidswear,” making a behind-the-scenes substitution of your actual query with a different query that just happens to generate more money for the company, and will generate results you weren’t searching for at all. It’s not possible for you to opt out of the substitution. If you don’t get the results you want, and you try to refine your query, you are wasting your time. This is a twisted shopping mall you can’t escape.
Why would Google want to do this? First, the generated results to the latter query are more likely to be shopping-oriented, triggering your subsequent behavior much like the candy display at a grocery store’s checkout. Second, that latter query will automatically generate the keyword ads placed on the search engine results page by stores like TJ Maxx, which pay Google every time you click on them. In short, it's a guaranteed way to line Google’s pockets.
It’s also a guaranteed way to harm everyone except Google. This system reduces search engine quality for users and drives up advertiser expenses. Google can get away with it because these manipulations are imperceptible to the user and advertiser, and the company has effectively captured more than 90 percent market share.
Google’s enshittification memos
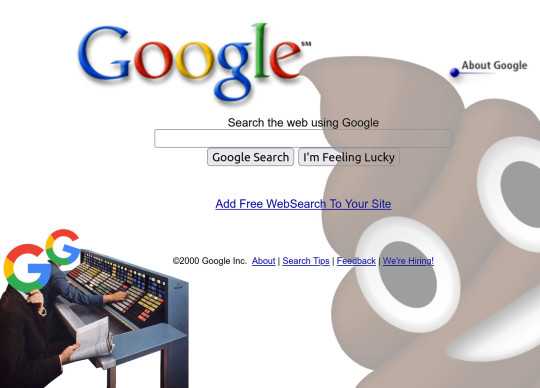
On October 7–8, I'm in Milan to keynote Wired Nextfest.
When I think about how the old, good internet turned into the enshitternet, I imagine a series of small compromises, each seemingly reasonable at the time, each contributing to a cultural norm of making good things worse, and worse, and worse.
Think about Unity President Marc Whitten's nonpology for his company's disastrous rug-pull, in which they declared that everyone who had paid good money to use their tool to make a game would have to keep paying, every time someone downloaded that game:
The most fundamental thing that we’re trying to do is we’re building a sustainable business for Unity. And for us, that means that we do need to have a model that includes some sort of balancing change, including shared success.
https://www.wired.com/story/unity-walks-back-policies-lost-trust/
"Shared success" is code for, "If you use our tool to make money, we should make money too." This is bullshit. It's like saying, "We just want to find a way to share the success of the painters who use our brushes, so every time you sell a painting, we want to tax that sale." Or "Every time you sell a house, the company that made the hammer gets to wet its beak."
And note that they're not talking about shared risk here – no one at Unity is saying, "If you try to make a game with our tools and you lose a million bucks, we're on the hook for ten percent of your losses." This isn't partnership, it's extortion.
How did a company like Unity – which became a market leader by making a tool that understood the needs of game developers and filled them – turn into a protection racket? One bad decision at a time. One rationalization and then another. Slowly, and then all at once.
When I think about this enshittification curve, I often think of Google, a company that had its users' backs for years, which created a genuinely innovative search engine that worked so well it seemed like *magic, a company whose employees often had their pick of jobs, but chose the "don't be evil" gig because that mattered to them.
People make fun of that "don't be evil" motto, but if your key employees took the gig because they didn't want to be evil, and then you ask them to be evil, they might just quit. Hell, they might make a stink on the way out the door, too:
https://theintercept.com/2018/09/13/google-china-search-engine-employee-resigns/
Google is a company whose founders started out by publishing a scientific paper describing their search methodology, in which they said, "Oh, and by the way, ads will inevitably turn your search engine into a pile of shit, so we're gonna stay the fuck away from them":
http://infolab.stanford.edu/pub/papers/google.pdf
Those same founders retained a controlling interest in the company after it went IPO, explaining to investors that they were going to run the business without having their elbows jostled by shortsighted Wall Street assholes, so they could keep it from turning into a pile of shit:
https://abc.xyz/investor/founders-letters/ipo-letter/
And yet, it's turned into a pile of shit. Google search is so bad you might as well ask Jeeves. The company's big plan to fix it? Replace links to webpages with florid paragraphs of chatbot nonsense filled with a supremely confident lies:
https://pluralistic.net/2023/05/14/googles-ai-hype-circle/
How did the company get this bad? In part, this is the "curse of bigness." The company can't grow by attracting new users. When you have 90%+ of the market, there are no new customers to sign up. Hypothetically, they could grow by going into new lines of business, but Google is incapable of making a successful product in-house and also kills most of the products it buys from other, more innovative companies:
https://killedbygoogle.com/
Theoretically, the company could pursue new lines of business in-house, and indeed, the current leaders of companies like Amazon, Microsoft and Apple are all execs who figured out how to get the whole company to do something new, and were elevated to the CEO's office, making each one a billionaire and sealing their place in history.
It is for this very reason that any exec at a large firm who tries to make a business-wide improvement gets immediately and repeatedly knifed by all their colleagues, who correctly reason that if someone else becomes CEO, then they won't become CEO. Machiavelli was an optimist:
https://pluralistic.net/2023/07/28/microincentives-and-enshittification/
With no growth from new customers, and no growth from new businesses, "growth" has to come from squeezing workers (say, laying off 12,000 engineers after a stock buyback that would have paid their salaries for the next 27 years), or business customers (say, by colluding with Facebook to rig the ad market with the Jedi Blue conspiracy), or end-users.
Now, in theory, we might never know exactly what led to the enshittification of Google. In theory, all of compromises, debates and plots could be lost to history. But tech is not an oral culture, it's a written one, and techies write everything down and nothing is ever truly deleted.
Time and again, Big Tech tells on itself. Think of FTX's main conspirators all hanging out in a group chat called "Wirefraud." Amazon naming its program targeting weak, small publishers the "Gazelle Project" ("approach these small publishers the way a cheetah would pursue a sickly gazelle”). Amazon documenting the fact that users were unknowingly signing up for Prime and getting pissed; then figuring out how to reduce accidental signups, then deciding not to do it because it liked the money too much. Think of Zuck emailing his CFO in the middle of the night to defend his outsized offer to buy Instagram on the basis that users like Insta better and Facebook couldn't compete with them on quality.
It's like every Big Tech schemer has a folder on their desktop called "Mens Rea" filled with files like "Copy_of_Premeditated_Murder.docx":
https://doctorow.medium.com/big-tech-cant-stop-telling-on-itself-f7f0eb6d215a?sk=351f8a54ab8e02d7340620e5eec5024d
Right now, Google's on trial for its sins against antitrust law. It's a hard case to make. To secure a win, the prosecutors at the DoJ Antitrust Division are going to have to prove what was going on in Google execs' minds when the took the actions that led to the company's dominance. They're going to have to show that the company deliberately undertook to harm its users and customers.
Of course, it helps that Google put it all in writing.
Last week, there was a huge kerfuffile over the DoJ's practice of posting its exhibits from the trial to a website each night. This is a totally normal thing to do – a practice that dates back to the Microsoft antitrust trial. But Google pitched a tantrum over this and said that the docs the DoJ were posting would be turned into "clickbait." Which is another way of saying, "the public would find these documents very interesting, and they would be damning to us and our case":
https://www.bigtechontrial.com/p/secrecy-is-systemic
After initially deferring to Google, Judge Amit Mehta finally gave the Justice Department the greenlight to post the document. It's up. It's wild:
https://www.justice.gov/d9/2023-09/416692.pdf
The document is described as "notes for a course on communication" that Google VP for Finance Michael Roszak prepared. Roszak says he can't remember whether he ever gave the presentation, but insists that the remit for the course required him to tell students "things I didn't believe," and that's why the document is "full of hyperbole and exaggeration."
OK.
But here's what the document says: "search advertising is one of the world's greatest business models ever created…illicit businesses (cigarettes or drugs) could rival these economics…[W]e can mostly ignore the demand side…(users and queries) and only focus on the supply side of advertisers, ad formats and sales."
It goes on to say that this might be changing, and proposes a way to balance the interests of the search and ads teams, which are at odds, with search worrying that ads are pushing them to produce "unnatural search experiences to chase revenue."
"Unnatural search experiences to chase revenue" is a thinly veiled euphemism for the prophetic warnings in that 1998 Pagerank paper: "The goals of the advertising business model do not always correspond to providing quality search to users." Or, more plainly, "ads will turn our search engine into a pile of shit."
And, as Roszak writes, Google is "able to ignore one of the fundamental laws of economics…supply and demand." That is, the company has become so dominant and cemented its position so thoroughly as the default search engine across every platforms and system that even if it makes its search terrible to goose revenues, users won't leave. As Lily Tomlin put it on SNL: "We don't have to care, we're the phone company."
In the enshittification cycle, companies first lure in users with surpluses – like providing the best search results rather than the most profitable ones – with an eye to locking them in. In Google's case, that lock-in has multiple facets, but the big one is spending billions of dollars – enough to buy a whole Twitter, every single year – to be the default search everywhere.
Google doesn't buy its way to dominance because it has the very best search results and it wants to shield you from inferior competitors. The economically rational case for buying default position is that preventing competition is more profitable than succeeding by outperforming competitors. The best reason to buy the default everywhere is that it lets you lower quality without losing business. You can "ignore the demand side, and only focus on advertisers."
For a lot of people, the analysis stops here. "If you're not paying for the product, you're the product." Google locks in users and sells them to advertisers, who are their co-conspirators in a scheme to screw the rest of us.
But that's not right. For one thing, paying for a product doesn't mean you won't be the product. Apple charges a thousand bucks for an iPhone and then nonconsensually spies on every iOS user in order to target ads to them (and lies about it):
https://pluralistic.net/2022/11/14/luxury-surveillance/#liar-liar
John Deere charges six figures for its tractors, then runs a grift that blocks farmers from fixing their own machines, and then uses their control over repair to silence farmers who complain about it:
https://pluralistic.net/2022/05/31/dealers-choice/#be-a-shame-if-something-were-to-happen-to-it
Fair treatment from a corporation isn't a loyalty program that you earn by through sufficient spending. Companies that can sell you out, will sell you out, and then cry victim, insisting that they were only doing their fiduciary duty for their sacred shareholders. Companies are disciplined by fear of competition, regulation or – in the case of tech platforms – customers seizing the means of computation and installing ad-blockers, alternative clients, multiprotocol readers, etc:
https://doctorow.medium.com/an-audacious-plan-to-halt-the-internets-enshittification-and-throw-it-into-reverse-3cc01e7e4604?sk=85b3f5f7d051804521c3411711f0b554
Which is where the next stage of enshittification comes in: when the platform withdraws the surplus it had allocated to lure in – and then lock in – business customers (like advertisers) and reallocate it to the platform's shareholders.
For Google, there are several rackets that let it screw over advertisers as well as searchers (the advertisers are paying for the product, and they're also the product). Some of those rackets are well-known, like Jedi Blue, the market-rigging conspiracy that Google and Facebook colluded on:
https://en.wikipedia.org/wiki/Jedi_Blue
But thanks to the antitrust trial, we're learning about more of these. Megan Gray – ex-FTC, ex-DuckDuckGo – was in the courtroom last week when evidence was presented on Google execs' panic over a decline in "ad generating searches" and the sleazy gimmick they came up with to address it: manipulating the "semantic matching" on user queries:
https://www.wired.com/story/google-antitrust-lawsuit-search-results/
When you send a query to Google, it expands that query with terms that are similar – for example, if you search on "Weds" it might also search for "Wednesday." In the slides shown in the Google trial, we learned about another kind of semantic matching that Google performed, this one intended to turn your search results into "a twisted shopping mall you can’t escape."
Here's how that worked: when you ran a query like "children's clothing," Google secretly appended the brand name of a kids' clothing manufacturer to the query. This, in turn, triggered a ton of ads – because rival brands will have bought ads against their competitors' name (like Pepsi buying ads that are shown over queries for Coke).
Here we see surpluses being taken away from both end-users and business customers – that is, searchers and advertisers. For searchers, it doesn't matter how much you refine your query, you're still going to get crummy search results because there's an unkillable, hidden search term stuck to your query, like a piece of shit that Google keeps sticking to the sole of your shoe.
But for advertisers, this is also a scam. They're paying to be matched to users who search on a brand name, and you didn't search on that brand name. It's especially bad for the company whose name has been appended to your search, because Google has a protection racket where the company that matches your search has to pay extra in order to show up overtop of rivals who are worse matches. Both the matching company and those rivals have given Google a credit-card that Google gets to bill every time a user searches on the company's name, and Google is just running fraudulent charges through those cards.
And, of course, Google put this in writing. I mean, of course they did. As we learned from the documentary The Incredibles, supervillains can't stop themselves from monologuing, and in big, sprawling monopolists, these monologues have to transmitted electronically – and often indelibly – to far-flung co-cabalists.
As Gray points out, this is an incredibly blunt enshittification technique: "it hadn’t even occurred to me that Google just flat out deletes queries and replaces them with ones that monetize better." We don't know how long Google did this for or how frequently this bait-and-switch was deployed.
But if this is a blunt way of Google smashing its fist down on the scales that balance search quality against ad revenues, there's plenty of subtler ways the company could sneak a thumb on there. A Google exec at the trial rhapsodized about his company's "contract with the user" to deliver an "honest results policy," but given how bad Google search is these days, we're left to either believe he's lying or that Google sucks at search.
The paper trail offers a tantalizing look at how a company went from doing something that was so good it felt like a magic trick to being "able to ignore one of the fundamental laws of economics…supply and demand," able to "ignore the demand side…(users and queries) and only focus on the supply side of advertisers."
What's more, this is a system where everyone loses (except for Google): this isn't a grift run by Google and advertisers on users – it's a grift Google runs on everyone.
If you'd like an essay-formatted version of this post to read or share, here's a link to it on pluralistic.net, my surveillance-free, ad-free, tracker-free blog:
https://pluralistic.net/2023/10/03/not-feeling-lucky/#fundamental-laws-of-economics

My next novel is The Lost Cause, a hopeful novel of the climate emergency. Amazon won't sell the audiobook, so I made my own and I'm pre-selling it on Kickstarter!
#google#google manipulating search queries to the detriment of users and advertisers#this is a good time to move to metasearch engines like searx
6K notes
·
View notes
Text
I'd like to complain about something.
When I do a Google search and I'm looking to buy something and I select "Nearby"
I will never
NEVER
want to see results that include shipping.
EVER.
When I select "Nearby", I want to physically go to a store TODAY and leave with the product in my hands.
TODAY.
I don't feel that this is an unreasonable want.
#The internet has become a place to get results that don't answer your query#enshittification#Google hasn't been a good search engine in a long time - I know
0 notes
Text
Google Cloud’s BigQuery Autonomous Data To AI Platform

BigQuery automates data analysis, transformation, and insight generation using AI. AI and natural language interaction simplify difficult operations.
The fast-paced world needs data access and a real-time data activation flywheel. Artificial intelligence that integrates directly into the data environment and works with intelligent agents is emerging. These catalysts open doors and enable self-directed, rapid action, which is vital for success. This flywheel uses Google's Data & AI Cloud to activate data in real time. BigQuery has five times more organisations than the two leading cloud providers that just offer data science and data warehousing solutions due to this emphasis.
Examples of top companies:
With BigQuery, Radisson Hotel Group enhanced campaign productivity by 50% and revenue by over 20% by fine-tuning the Gemini model.
By connecting over 170 data sources with BigQuery, Gordon Food Service established a scalable, modern, AI-ready data architecture. This improved real-time response to critical business demands, enabled complete analytics, boosted client usage of their ordering systems, and offered staff rapid insights while cutting costs and boosting market share.
J.B. Hunt is revolutionising logistics for shippers and carriers by integrating Databricks into BigQuery.
General Mills saves over $100 million using BigQuery and Vertex AI to give workers secure access to LLMs for structured and unstructured data searches.
Google Cloud is unveiling many new features with its autonomous data to AI platform powered by BigQuery and Looker, a unified, trustworthy, and conversational BI platform:
New assistive and agentic experiences based on your trusted data and available through BigQuery and Looker will make data scientists, data engineers, analysts, and business users' jobs simpler and faster.
Advanced analytics and data science acceleration: Along with seamless integration with real-time and open-source technologies, BigQuery AI-assisted notebooks improve data science workflows and BigQuery AI Query Engine provides fresh insights.
Autonomous data foundation: BigQuery can collect, manage, and orchestrate any data with its new autonomous features, which include native support for unstructured data processing and open data formats like Iceberg.
Look at each change in detail.
User-specific agents
It believes everyone should have AI. BigQuery and Looker made AI-powered helpful experiences generally available, but Google Cloud now offers specialised agents for all data chores, such as:
Data engineering agents integrated with BigQuery pipelines help create data pipelines, convert and enhance data, discover anomalies, and automate metadata development. These agents provide trustworthy data and replace time-consuming and repetitive tasks, enhancing data team productivity. Data engineers traditionally spend hours cleaning, processing, and confirming data.
The data science agent in Google's Colab notebook enables model development at every step. Scalable training, intelligent model selection, automated feature engineering, and faster iteration are possible. This agent lets data science teams focus on complex methods rather than data and infrastructure.
Looker conversational analytics lets everyone utilise natural language with data. Expanded capabilities provided with DeepMind let all users understand the agent's actions and easily resolve misconceptions by undertaking advanced analysis and explaining its logic. Looker's semantic layer boosts accuracy by two-thirds. The agent understands business language like “revenue” and “segments” and can compute metrics in real time, ensuring trustworthy, accurate, and relevant results. An API for conversational analytics is also being introduced to help developers integrate it into processes and apps.
In the BigQuery autonomous data to AI platform, Google Cloud introduced the BigQuery knowledge engine to power assistive and agentic experiences. It models data associations, suggests business vocabulary words, and creates metadata instantaneously using Gemini's table descriptions, query histories, and schema connections. This knowledge engine grounds AI and agents in business context, enabling semantic search across BigQuery and AI-powered data insights.
All customers may access Gemini-powered agentic and assistive experiences in BigQuery and Looker without add-ons in the existing price model tiers!
Accelerating data science and advanced analytics
BigQuery autonomous data to AI platform is revolutionising data science and analytics by enabling new AI-driven data science experiences and engines to manage complex data and provide real-time analytics.
First, AI improves BigQuery notebooks. It adds intelligent SQL cells to your notebook that can merge data sources, comprehend data context, and make code-writing suggestions. It also uses native exploratory analysis and visualisation capabilities for data exploration and peer collaboration. Data scientists can also schedule analyses and update insights. Google Cloud also lets you construct laptop-driven, dynamic, user-friendly, interactive data apps to share insights across the organisation.
This enhanced notebook experience is complemented by the BigQuery AI query engine for AI-driven analytics. This engine lets data scientists easily manage organised and unstructured data and add real-world context—not simply retrieve it. BigQuery AI co-processes SQL and Gemini, adding runtime verbal comprehension, reasoning skills, and real-world knowledge. Their new engine processes unstructured photographs and matches them to your product catalogue. This engine supports several use cases, including model enhancement, sophisticated segmentation, and new insights.
Additionally, it provides users with the most cloud-optimized open-source environment. Google Cloud for Apache Kafka enables real-time data pipelines for event sourcing, model scoring, communications, and analytics in BigQuery for serverless Apache Spark execution. Customers have almost doubled their serverless Spark use in the last year, and Google Cloud has upgraded this engine to handle data 2.7 times faster.
BigQuery lets data scientists utilise SQL, Spark, or foundation models on Google's serverless and scalable architecture to innovate faster without the challenges of traditional infrastructure.
An independent data foundation throughout data lifetime
An independent data foundation created for modern data complexity supports its advanced analytics engines and specialised agents. BigQuery is transforming the environment by making unstructured data first-class citizens. New platform features, such as orchestration for a variety of data workloads, autonomous and invisible governance, and open formats for flexibility, ensure that your data is always ready for data science or artificial intelligence issues. It does this while giving the best cost and decreasing operational overhead.
For many companies, unstructured data is their biggest untapped potential. Even while structured data provides analytical avenues, unique ideas in text, audio, video, and photographs are often underutilised and discovered in siloed systems. BigQuery instantly tackles this issue by making unstructured data a first-class citizen using multimodal tables (preview), which integrate structured data with rich, complex data types for unified querying and storage.
Google Cloud's expanded BigQuery governance enables data stewards and professionals a single perspective to manage discovery, classification, curation, quality, usage, and sharing, including automatic cataloguing and metadata production, to efficiently manage this large data estate. BigQuery continuous queries use SQL to analyse and act on streaming data regardless of format, ensuring timely insights from all your data streams.
Customers utilise Google's AI models in BigQuery for multimodal analysis 16 times more than last year, driven by advanced support for structured and unstructured multimodal data. BigQuery with Vertex AI are 8–16 times cheaper than independent data warehouse and AI solutions.
Google Cloud maintains open ecology. BigQuery tables for Apache Iceberg combine BigQuery's performance and integrated capabilities with the flexibility of an open data lakehouse to link Iceberg data to SQL, Spark, AI, and third-party engines in an open and interoperable fashion. This service provides adaptive and autonomous table management, high-performance streaming, auto-AI-generated insights, practically infinite serverless scalability, and improved governance. Cloud storage enables fail-safe features and centralised fine-grained access control management in their managed solution.
Finaly, AI platform autonomous data optimises. Scaling resources, managing workloads, and ensuring cost-effectiveness are its competencies. The new BigQuery spend commit unifies spending throughout BigQuery platform and allows flexibility in shifting spend across streaming, governance, data processing engines, and more, making purchase easier.
Start your data and AI adventure with BigQuery data migration. Google Cloud wants to know how you innovate with data.
#technology#technews#govindhtech#news#technologynews#BigQuery autonomous data to AI platform#BigQuery#autonomous data to AI platform#BigQuery platform#autonomous data#BigQuery AI Query Engine
2 notes
·
View notes
Text
📈 Dive into the world of Google AI Overviews! Discover how the search engine trends towards authoritative sites and its impact on key industries. Is your content ready for the future? #GoogleAI #SEO #DigitalMarketing #AuthoritativeContent
#AI in search#authoritative sites#B2B technology#content authority#digital marketing#eCommerce SEO#entertainment industry trends#Google AI Overviews#healthcare SEO#insurance queries#long-tail keywords#online visibility#search engine rankings#SEO trends#travel industry search#user expectations.
0 notes
Text
currently experiencing a gut problem that is gross but not painful at all, which makes all my attempts to self-diagnose using dr. google totally useless bc every digestion problem that i think to investigate has the symptom "abdominal pain or cramps"
1 note
·
View note
Text
logic provided to search engine nodes by artificial intelligence search queries
0 notes
Text
Flask and Pydantic
Introduction In this tutorial we are going to learn how to use Pydantic together with Flask to perform validation of query parameters and request bodies. Continue reading Flask and Pydantic
View On WordPress
#body parameters#Flask#HTTP server#pydantic#Python#Query Parameters#software engineering#tutorial#validation
1 note
·
View note
Text
Mastering Query Deserves Freshness (QDF): Your Key to SEO Success
In the ever-evolving world of SEO, staying ahead means understanding the strategies that make search engines tick. One such crucial concept is Query Deserves Freshness (QDF) — a game-changer for ranking in search results. Whether you’re an SEO beginner or a seasoned marketer, grasping QDF can significantly impact your strategy. So, what is QDF, and how can it shape your approach to search engine optimization? Let’s break it down.

What is Query Deserves Freshness (QDF)?
Query Deserves Freshness (QDF) is a concept introduced by search engines to prioritize fresh, relevant content for specific queries. It recognizes that some searches — like those related to current events, trends, or rapidly changing topics — demand up-to-date information. By leveraging QDF, businesses can improve their chances of appearing in top search results, delivering timely value to their audiences.
For instance, when users search for “new iPhone launch,” Google’s algorithm ensures that the latest, most relevant content takes center stage. This dynamic approach helps users access accurate information while offering businesses a unique opportunity to capture attention through fresh content.
Why is Query Deserves Freshness Important?
Search engines aim to satisfy user intent, and QDF is a key tool for delivering real-time relevance. Whether your niche is technology, finance, or healthcare, QDF ensures your content aligns with what’s trending in your industry.
By prioritizing fresh and timely content, search engines reward businesses that stay on top of emerging topics. This is especially critical for businesses targeting trending keywords or localized searches where up-to-date information is essential to engage users.
How Does Query Deserves Freshness Work?
The QDF mechanism evaluates various freshness signals to rank content. Here’s how it works:
Freshness Signals: Google considers factors like publish date, content updates, and user engagement to assess how current the content is.
Timely Content Ranking: Newly created or updated content that aligns with a trending topic gets preference in search results.
User Intent Alignment: QDF prioritizes content that directly satisfies search intent, ensuring users receive valuable insights promptly.
Why QDF is a Must-Have for Your SEO Strategy
For businesses in dynamic industries, QDF can be a goldmine. Regularly publishing fresh content or updating existing posts boosts your visibility for queries requiring the latest information. This not only drives traffic but positions your website as an authoritative source.
Whether you’re focused on national SEO or local SEO, leveraging QDF enables you to connect with users searching for timely, relevant insights. It’s not just about ranking higher — it’s about being a trusted resource for fresh, actionable content.
Top Strategies to Leverage Query Deserves Freshness
Create Timely Content Stay ahead of industry trends by crafting content on emerging topics, breaking news, or hot discussions. This proactive approach ensures your content is primed for trending searches.
Update Existing Content Regular updates keep older posts relevant while boosting their SEO value. For instance, incorporate new statistics, insights, or case studies into evergreen content to maintain its freshness.
Use Multimedia for Engagement Adding videos, infographics, and podcasts not only enhances user experience but also improves your chances of ranking in featured snippets or video results.
Challenges of Implementing Query Deserves Freshness
While QDF offers clear benefits, there are challenges to consider:
Content Saturation: Competitive industries may require extra effort to stand out amidst similar content.
Resource Management: Keeping content fresh demands time and resources, especially for businesses with extensive material.
Over-Optimization Risks: Avoid stuffing trendy keywords or forcing updates that feel unnatural. Always prioritize value for your audience.
Conclusion: Staying Fresh for SEO Success
Optimizing for Query Deserves Freshness is a continuous effort that requires strategic planning and execution. For businesses looking to maintain an edge, partnering with the right SEO agency can make all the difference.
If you’re looking for results-driven solutions, consider working with an experienced team to help you create fresh, engaging content that resonates with your audience. Whether you’re targeting new trends or refining your existing strategy, QDF is your ticket to staying competitive in the fast-paced digital landscape.
For more tips and SEO strategies, connect with us today and take the first step toward a stronger, fresher digital presence!
0 notes
Text
Understanding SQL Query Execution: A Data Engineer’s Guide
As data engineers, we work with SQL daily, but how many of us truly understand the inner workings of a SQL query? Knowing the order of execution can significantly improve the way you write SQL queries. Let’s dive into the process with a practical example.
For more information, visit Teklink International LLC
0 notes