#ConvolutionalNeuralNetworks
Explore tagged Tumblr posts
Text
Artificial Neural Networks in a Deep Learning Perspective
Artificial Neural Networks (ANNs) are central to deep learning, revolutionizing industries like healthcare, finance, and automotive. The Computer Science and Engineering department at K. Ramakrishnan College of Technology (KRCT) equips students with cutting-edge skills in ANNs, blending theory and hands-on projects. KRCT's focus on advanced AI ensures graduates are prepared to lead future innovations.

Click here:
#ArtificialNeuralNetworks#top college of technology in trichy#krct the top college of technology in trichy#quality engineering and technical education.#k ramakrishnan college of technology trichy#training and engineering placement#DeepLearning#AIRevolution#MachineLearningModels#FutureOfTechnology#ANNsInIndustry#ConvolutionalNeuralNetworks#ArtificialIntelligenceInsights#RecurrentNeuralNetworks#ExplainableAI#AIInHealthcare#AIInEducation
0 notes
Text
Unlock the secrets of training Convolutional Neural Networks (CNNs) with our user-friendly flowchart. From data preparation to model fine-tuning, grasp each step in building powerful image classifiers. Simplify your deep learning path with our guide, perfect for beginners and experts alike. Stay tuned to Softlabs Group for more AI insights!
#CNNTraining#ConvolutionalNeuralNetworks#DeepLearningTraining#NeuralNetworks#MachineLearningAlgorithms
0 notes
Text
Unveiling the Depths of Intelligence: A Journey into the World of Deep Learning
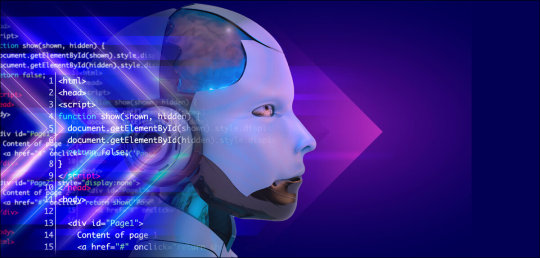
Embark on a captivating exploration into the cutting-edge realm of Deep Learning, a revolutionary paradigm within the broader landscape of artificial intelligence. Read More. https://www.sify.com/ai-analytics/unveiling-the-depths-of-intelligence-a-journey-into-the-world-of-deep-learning/
#DeepLearning#ArtificialIntelligence#AI#ConvolutionalNeuralNetworks#CNNs#GenerativeAdversarialNetworks#GANs#Autoencoders
0 notes
Text
Neural Style Transfer (NST)
Neural Style Transfer (NST) is a captivating intersection of artificial intelligence and artistic creativity. This technology leverages the capabilities of deep learning to merge the essence of one image with the aesthetic style of another.
Basic Concept of Neural Style Transfer (NST)
Combining Content and Style: NST works by taking two images - a content image (like a photograph) and a style image (usually a famous painting) - and combining them. The goal is to produce a new image that retains the original content but is rendered in the artistic style of the second image. Deep Learning at its Core: This process is made possible through deep learning techniques, specifically using Convolutional Neural Networks (CNNs). These networks are adept at recognizing and processing visual information. Content Representation: The CNN captures the content of the target image at its deeper layers, where the network understands higher-level features (like objects and their arrangements). Style Representation: The style of the source image is captured from the correlations between different layers of the CNN. These layers encode textural and color patterns characteristic of the artistic style. Image Transformation: The NST algorithm iteratively adjusts a third, initially random image to minimize the differences in content with the target image and in style with the source image. Resulting Image: The result is a fascinating blend that looks like the original photograph (content) 'painted' in the style of the artwork (style).
How Neural Style Transfer Works with Python Example
Content and Style Images: The process begins with two images: a content image (the subject you want to transform) and a style image (the artistic style to be transferred). Using a Pre-Trained CNN: Typically, a pre-trained CNN like VGG19 is used. This network has been trained on a vast dataset of images and can effectively extract and represent features from these images. Feature Extraction: The CNN extracts content features from the content image and style features from the style image. These features are essentially patterns and textures that define the image's content and style. Combining Features: The NST algorithm then creates a new image that combines the content features of the content image with the style features of the style image. Optimization: This new image is gradually refined through an optimization process, minimizing the loss between its content and the content image, and its style and the style image. Result: The final output is a new image that retains the essence of the content image but is rendered in the style of the style image. Python Code Example: import tensorflow as tf import tensorflow_hub as hub import matplotlib.pyplot as plt import numpy as np # Load content and style images content_image = plt.imread('path_to_content_image.jpg') style_image = plt.imread('path_to_style_image.jpg') # Load a style transfer model from TensorFlow Hub hub_model = hub.load('https://tfhub.dev/google/magenta/arbitrary-image-stylization-v1-256/2') # Preprocess images and run the style transfer content_image = tf.image.convert_image_dtype(content_image, tf.float32) style_image = tf.image.convert_image_dtype(style_image, tf.float32) stylized_image = hub_model(tf.constant(content_image), tf.constant(style_image)) # Display the output plt.imshow(np.squeeze(stylized_image)) plt.show() This code snippet uses TensorFlow and TensorFlow Hub to apply a style transfer model, merging the content of one image with the style of another.
Detailed Section on Content and Style Representations in Neural Style Transfer
Feature Extraction Using Pre-Trained CNN: VGG19, a CNN model pre-trained on a large dataset (like ImageNet), is often used. This model effectively extracts features from images. Content Representation: - The content of an image is represented by the feature maps of higher layers in the CNN. - These layers capture the high-level content of the image, such as objects and their spatial arrangement, but not the finer details or style aspects. Style Representation: - The style of an image is captured by examining the correlations across different layers' feature maps. - These correlations are represented as a Gram matrix, which effectively captures the texture and visual patterns that define the image's style. Combining Content and Style: - NST algorithms aim to preserve the content from the content image while adopting the style of the style image. - This is done by minimizing a loss function that measures the difference in content and style representations between the generated image and the respective content and style images. Python Code Example: import numpy as np import tensorflow as tf from tensorflow.keras.applications import vgg19 from tensorflow.keras.preprocessing.image import load_img, img_to_array # Function to preprocess the image for VGG19 def preprocess_image(image_path, target_size=(224, 224)): img = load_img(image_path, target_size=target_size) img = img_to_array(img) img = np.expand_dims(img, axis=0) img = vgg19.preprocess_input(img) return img # Load your content and style images content_image = preprocess_image('path_to_your_content_image.jpg') style_image = preprocess_image('path_to_your_style_image.jpg') # Load the VGG19 model model = vgg19.VGG19(weights='imagenet', include_top=False) # Define a function to get content and style features def get_features(image, model): layers = { 'content': , 'style': } features = {} outputs = + layers] model = tf.keras.Model(, outputs) image_features = model(image) for name, output in zip(layers + layers, image_features): features = output return features # Extract features content_features = get_features(content_image, model) style_features = get_features(style_image, model) This code provides a basic structure for extracting content and style features using VGG19 in Python. Further steps would involve defining and optimizing the loss functions to generate the stylized image.
Applications of Neural Style Transfer
Video Styling: NST can be applied to video content, allowing filmmakers and content creators to impart artistic styles to their videos. This can transform ordinary footage into visually stunning sequences that resemble paintings or other art forms. Website Design: In web design, NST can be used to create unique, visually appealing backgrounds and elements. Designers can apply specific artistic styles to images, aligning them with the overall aesthetic of the website. Fashion and Textile Design: NST has been explored in the fashion industry for designing fabrics and garments. By transferring artistic styles onto textile patterns, designers can create innovative and unique clothing lines. Augmented Reality (AR) and Virtual Reality (VR): In AR and VR environments, NST can enhance the visual experience by applying artistic styles in real-time, creating immersive and engaging worlds for users. Product Design: NST can be used in product design to create visually appealing prototypes and presentations, allowing designers to experiment with different artistic styles quickly. Therapeutic Settings for Mental Health: There's growing interest in using NST in therapeutic settings. By creating soothing and pleasant images, it can be used as a tool for relaxation and stress relief, contributing positively to mental health and well-being. Educational Tools: NST can also be used as an educational tool in art and design schools, helping students understand the nuances of different artistic styles and techniques. These diverse applications showcase the versatility of NST, demonstrating its potential beyond the realm of digital art creation.
Limitations and Challenges of Neural Style Transfer
Computational Intensity: - NST, especially when using deep learning models like VGG19, is computationally demanding. It requires significant processing power, often necessitating the use of GPUs to achieve reasonable processing times. Balancing Content and Style: - Achieving the right balance between content and style in the output image can be challenging. It often requires careful tuning of the algorithm's parameters and may involve a lot of trial and error. Unpredictability of Results: - The outcome of NST can be unpredictable. The results may vary widely based on the chosen content and style images and the specific configurations of the neural network. Quality of Output: - The quality of the generated image can sometimes be lower than expected, with issues like distortions in the content or the style not being accurately captured. Training Data Limitations: - The effectiveness of NST is also influenced by the variety and quality of images used to train the underlying model. Limited or biased training data can affect the versatility and effectiveness of the style transfer. Overfitting: - There's a risk of overfitting, especially when the style transfer model is trained on a narrow set of images. This can limit the model's ability to generalize across different styles and contents. These challenges highlight the need for ongoing research and development in the field of NST to enhance its efficiency, versatility, and accessibility.
Necessary Hardware Resources for AI and Machine Learning in Art Generation
To effectively work with AI and machine learning algorithms for art generation, which can be computationally intensive, certain hardware resources are essential: High-Performance GPUs: - Graphics Processing Units (GPUs) are crucial for their ability to handle parallel tasks, making them ideal for the intensive computations required in training and running neural networks. - GPUs significantly reduce the time required for training models and generating art, compared to traditional CPUs. Sufficient RAM: - Adequate Random Access Memory (RAM) is important for handling large datasets and the high memory requirements of deep learning models. - A minimum of 16GB RAM is recommended, but 32GB or higher is preferable for more complex tasks. Fast Storage Solutions: - Solid State Drives (SSDs) are preferred over Hard Disk Drives (HDDs) for their faster data access speeds, which is beneficial when working with large datasets and models. High-Performance CPUs: - While GPUs handle most of the heavy lifting, a good CPU can improve overall system performance and efficiency. - Multi-core processors with high clock speeds are recommended. Cloud Computing Platforms: - Cloud computing resources like AWS, Google Cloud Platform, or Microsoft Azure offer powerful hardware for AI and machine learning tasks without the need for local installation. - These platforms provide scalability, allowing you to choose resources as per the project's requirements. Adequate Cooling Solutions: - High computational tasks generate significant heat. Therefore, a robust cooling solution is necessary to maintain optimal hardware performance and longevity. Reliable Power Supply: - A stable and reliable power supply is crucial, especially for desktop setups, to ensure uninterrupted processing and to protect the hardware from power surges. Investing in these hardware resources can greatly enhance the efficiency and capabilities of AI and machine learning algorithms in art generation and other computationally demanding tasks.
Limitations and Challenges of Neural Style Transfer
Neural Style Transfer (NST), despite its innovative applications in art and technology, faces several limitations and challenges: Computational Resource Intensity: - NST is computationally demanding, often requiring powerful GPUs and significant processing power. This can be a barrier for individuals or organizations without access to high-end computing resources. Quality and Resolution of Output: - The quality and resolution of the output images can sometimes be less than satisfactory. High-resolution images may lose detail or suffer from distortions after the style transfer. Balancing Act Between Content and Style: - Achieving a harmonious balance between the content and style in the output image can be challenging. It often requires fine-tuning of parameters and multiple iterations. Generalization and Diversity: - NST models might struggle with generalizing across vastly different styles or content types. This can limit the diversity of styles that can be effectively transferred. Training Data Biases: - The effectiveness of NST can be limited by the biases present in the training data. A model trained on a narrow range of styles may not perform well with radically different artistic styles. Overfitting Risks: - There's a risk of overfitting when the style transfer model is exposed to a limited set of images, leading to reduced effectiveness on a broader range of styles. Real-Time Processing Challenges: - Implementing NST in real-time applications, such as video styling, can be particularly challenging due to the intensive computational requirements. Understanding and addressing these limitations and challenges is crucial for the advancement and wider application of NST technologies.
Trends and Innovations in Neural Style Transfer (NST)
Neural Style Transfer (NST) is an evolving field with continuous advancements and innovations. These developments are broadening its applications and enhancing its efficiency: Improving Efficiency: - Research is focused on making NST algorithms faster and more resource-efficient. This includes optimizing existing neural network architectures and developing new methods to reduce computational requirements. Adapting to Various Artistic Styles: - Innovations in NST are enabling the adaptation to a wider range of artistic styles. This includes the ability to mimic more complex and abstract art forms, providing artists and designers with more diverse creative tools. Extending Applications Beyond Visual Art: - NST is finding applications in areas beyond traditional visual art. This includes video game design, film production, interior design, and even fashion, where NST can be used to create unique patterns and designs. Real-Time Style Transfer: - Advances in real-time processing capabilities are enabling NST to be applied in dynamic environments, such as live video feeds, augmented reality (AR), and virtual reality (VR). Integration with Other AI Technologies: - NST is being combined with other AI technologies like Generative Adversarial Networks (GANs) and reinforcement learning to create more sophisticated and versatile style transfer tools. User-Friendly Tools and Platforms: - The development of more user-friendly NST tools and platforms is democratizing access, allowing artists and non-technical users to experiment with style transfer without deep technical knowledge. These trends and innovations are propelling NST into new realms of creativity and practical application, making it a rapidly growing area in the field of AI and machine learning.
🌐 Sources
- Neural Style Transfer: Trends, Innovations, and Benefits - Challenges and Limitations of Deep Learning for Style Transfer - Neural Style Transfer: A Critical Review - Neural Style Transfer for So Long, and Thanks for all the Fish - Advantages and disadvantages of two methods of Neural Style Transfer - Evaluate and improve the quality of neural style transfer - Neural Style Transfer: Creating Artistic Images with Deep Learning - Classic algorithms, neural style transfer, GAN - Ricardo Corin - Mastering Neural Style Transfer - Neural Style Transfer Papers on GitHub - How to Make Artistic Images with Neural Style Transfer - Artificial Intelligence and Applications: Neural Style Transfer - Neural Style Transfer with Deep VGG model - Style Transfer using Deep Neural Network and PyTorch - Neural Style Transfer on Real Time Video (With Full Implementable Code) - How to Code Neural Style Transfer in Python? - Implementing Neural Style Transfer Using TensorFlow 2.0 - Neural Style Transfer (NST). Using Deep Learning Algorithms - Neural Read the full article
#AI#Art#ArtisticStyle#ConvolutionalNeuralNetworks#Deeplearning#ImageProcessing#machinelearning#NeuralStyleTransfer#OptimizationTechniques#VGG19
0 notes
Text
New Oracle Roving Edge Device Revolutionizes AI At The Edge

Oracle’s second-generation Roving Edge Device (RED), the newest product in the company’s e Edge Cloud line, offers exceptional processing power, smooth connection, and integrated security at network edges and in remote areas. Numerous workloads, including corporate applications, AI, and certain OCI services, may be operated at the edge with Roving Edge Device(RED) because to its easy deployment, great price-performance, and better security, which includes the option to run isolated or air-gapped.
What is Oracle Roving Edge Device?
OCI cloud computing and storage are made possible at network edges and in remote areas via the Oracle Roving Edge Device (RED), a portable hardware platform with cloud-integrated services.
The second version of the Roving Edge Device builds upon a strong foundation created to satisfy the requirements of security applications. It not only improves basic capabilities but also adds customized configurations to satisfy business needs in a range of sectors.Image Credit To Intel
Introducing the second generation Oracle RED
With more OCPUs, RAM, storage, and better GPU performance, the second iteration of the Oracle Roving Edge Device offers significant improvements over the first generation.Image Credit To Intel
RED is available in three configurations to suit diverse business
Where can you deploy Oracle Roving Edge Device?
In your data center or at the edge, RED offers the same OCI development and deployment methods, selectable OCI services, and CPU and GPU forms. This drives development in many industries and technical advancement in today’s fast-paced commercial environment. Due to its unrivaled processing power, smooth connectivity, and steadfast security, the Roving Edge Device is ideal for cutting-edge applications that need speed, dependability, and efficiency.
Improvements in performance with the second-generation RED
Milliseconds matter in the fast-paced world of artificial intelligence. Imagine a future in which your network’s borders are infinite and its edge gets exponentially more intelligent. Customers now have more deployment options with to Oracle Roving Edge Device 2nd Generation (RED), which has a new GPU-optimized configuration with compute- and storage-optimized configuration.
Customers gain from low-latency processing nearer the point of data production and ingestion by utilizing the Intel Xeon 8480+ processor’s capability at the edge, which leads to more timely insights into their data. Oracle and Intel collaborated to perform a number of benchmarks over the first-generation RED in order to test this capability.
It used only Intel Xeon processors to run the Llama 2-7B, Yolov10 model, and Resnet50 convolutional neural network (CNN) for the testing. The Intel Xeon 6230T-based first-generation Roving Edge Device is compared to the Intel Xeon 8480+-based second-generation using the following benchmarks:
Deploying Llama2-7B on RED
An autoregressive, transformer architecture serves as the foundation for the Llama 2 family of pre-trained and optimized text generation models. Three models with seven billion, thirteen billion, and seventy billion parameters are included with Llama 2. Oracle benchmarked the Llama 2 7 billion parameter model for this simulation.
Using the Llama 2-7B model, the second-generation Roving Edge Device may achieve response rates up to 13.6 times faster than RED Gen 1, allowing for lightning-fast performance for edge-based large language model (LLM) inferencing. Enhancement of Throughput Intel Xeon 8480+ Processor.
Using the Llama2-7B paradigm, the RED Gen 2 may achieve up to 12.4 times higher throughput, greatly increasing the edge’s capacity for processing LLM data.
YOLO v10
Real-time object identification and precise, low-latency object category and location prediction in pictures were the goals of the YOLO family of models. Oracle compared using the YOLO v10 model on the two versions of Roving Edge Device in this set of benchmarks.
Up to 60% more performance may be achieved by the new RED generation than by the old one. YOLO v10 increased throughput by 67%.
ResNet-50
A convolutional neural network (CNN) architecture called ResNet-50 is a member of the Residual Networks (ResNet) family, a group of models created to tackle the difficulties involved in deep neural network training. Renowned for its depth and effectiveness in image classification tasks, ResNet-50 was created by researchers at Microsoft Research Asia. There are several levels of ResNet topologies, including ResNet-18 and ResNet-32, with ResNet-50 being a mid-sized version.
Using the ResNet 50 CNN, the second generation achieves a response rate that is up to three times higher than the first.
Why deploy with Oracle Roving Edge Device?
Oracle Roving Edge Device is the best option if you need to deploy application workloads at the edge and need a scalable, secure, and adaptable platform with the advantages of cloud computing and cost-effectiveness. Built to execute time-sensitive, mission-critical applications at the edge in both connected and unconnected areas, it is a powerful cloud-integrated service.
Getting Started
Oracle Roving Edge Device is the perfect infrastructure for anybody seeking a high-security, low-latency data processing and scalable environment at the edge because of its affordable, adaptable configurations and capacity to serve computing, storage, and GPU-intensive applications.
Read more on govindhtech.com
#OracleRovingEdgeDevice#RevolutionizesAI#cloudcomputing#artificialintelligence#Llama2#IntelXeonprocessors#YOLO#GettingStarted#convolutionalneuralnetwork#CNN#EdgeDevice#technology#technews#news#govindhtech
0 notes
Text
What is a Neural Network? A Beginner's Guide
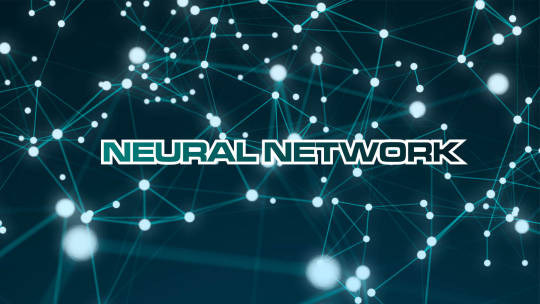
Artificial Intelligence (AI) is everywhere today—from helping us shop online to improving medical diagnoses. At the core of many AI systems is a concept called the neural network, a tool that enables computers to learn, recognize patterns, and make decisions in ways that sometimes feel almost human. But what exactly is a neural network, and how does it work? In this guide, we’ll explore the basics of neural networks and break down the essential components and processes that make them function. The Basic Idea Behind Neural Networks At a high level, a neural network is a type of machine learning model that takes in data, learns patterns from it, and makes predictions or decisions based on what it has learned. It’s called a “neural” network because it’s inspired by the way our brains process information. Imagine your brain’s neurons firing when you see a familiar face in a crowd. Individually, each neuron doesn’t know much, but together they recognize the pattern of a person’s face. In a similar way, a neural network is made up of interconnected nodes (or “neurons”) that work together to find patterns in data. Breaking Down the Structure of a Neural Network To understand how a neural network works, let's take a look at its basic structure. Neural networks are typically organized in layers, each playing a unique role in processing information: - Input Layer: This is where the data enters the network. Each node in the input layer represents a piece of data. For example, if the network is identifying a picture of a dog, each pixel of the image might be one node in the input layer. - Hidden Layers: These are the layers between the input and output. They’re called “hidden” because they don’t directly interact with the outside environment—they only process information from the input layer and pass it on. Hidden layers help the network learn complex patterns by transforming the data in various ways. - Output Layer: This is where the network gives its final prediction or decision. For instance, if the network is trying to identify an animal, the output layer might provide a probability score for each type of animal (e.g., 90% dog, 5% cat, 5% other). Each layer is made up of “neurons” (or nodes) that are connected to neurons in the previous and next layers. These connections allow information to pass through the network and be transformed along the way. The Role of Weights and Biases In a neural network, each connection between neurons has an associated weight. Think of weights as the importance or influence of one neuron on another. When information flows from one layer to the next, each connection either strengthens or weakens the signal based on its weight. - Weights: A higher weight means the signal is more important, while a lower weight means it’s less important. Adjusting these weights during training helps the network make better predictions. - Biases: Each neuron also has a bias value, which can be thought of as a threshold it needs to “fire” or activate. Biases allow the network to make adjustments and refine its learning process. Together, weights and biases help the network decide which features in the data are most important. For example, when identifying an image of a cat, weights and biases might be adjusted to give more importance to features like “fur” and “whiskers.” How a Neural Network Learns: Training with Data Neural networks learn by adjusting their weights and biases through a process called training. During training, the network is exposed to many examples (or “data points”) and gradually learns to make better predictions. Here’s a step-by-step look at the training process: - Feed Data into the Network: Training data is fed into the input layer of the network. For example, if the network is designed to recognize handwritten digits, each training example might be an image of a digit, like the number “5.” - Forward Propagation: The data flows from the input layer through the hidden layers to the output layer. Along the way, each neuron performs calculations based on the weights, biases, and activation function (a function that decides if the neuron should activate or not). - Calculate Error: The network then compares its prediction to the actual result (the known answer in the training data). The difference between the prediction and the actual answer is the error. - Backward Propagation: To improve, the network needs to reduce this error. It does so through a process called backpropagation, where it adjusts weights and biases to minimize the error. Backpropagation uses calculus to “push” the error backwards through the network, updating the weights and biases along the way. - Repeat and Improve: This process repeats thousands or even millions of times, allowing the network to gradually improve its accuracy. Real-World Analogy: Training a Neural Network to Recognize Faces Imagine you’re trying to train a neural network to recognize faces. Here’s how it would work in simple terms: - Input Layer (Eyes, Nose, Mouth): The input layer takes in raw information like pixels in an image. - Hidden Layers (Detecting Features): The hidden layers learn to detect features like the outline of the face, the position of the eyes, and the shape of the mouth. - Output Layer (Face or No Face): Finally, the output layer gives a probability that the image is a face. If it’s not accurate, the network adjusts until it can reliably recognize faces. Types of Neural Networks There are several types of neural networks, each designed for specific types of tasks: - Feedforward Neural Networks: These are the simplest networks, where data flows in one direction—from input to output. They’re good for straightforward tasks like image recognition. - Convolutional Neural Networks (CNNs): These are specialized for processing grid-like data, such as images. They’re especially powerful in detecting features in images, like edges or textures, which makes them popular in image recognition. - Recurrent Neural Networks (RNNs): These networks are designed to process sequences of data, such as sentences or time series. They’re used in applications like natural language processing, where the order of words is important. Common Applications of Neural Networks Neural networks are incredibly versatile and are used in many fields: - Image Recognition: Identifying objects or faces in photos. - Speech Recognition: Converting spoken language into text. - Natural Language Processing: Understanding and generating human language, used in applications like chatbots and language translation. - Medical Diagnosis: Assisting doctors in analyzing medical images, like MRIs or X-rays, to detect diseases. - Recommendation Systems: Predicting what you might like to watch, read, or buy based on past behavior. Are Neural Networks Intelligent? It’s easy to think of neural networks as “intelligent,” but they’re actually just performing a series of mathematical operations. Neural networks don’t understand the data the way we do—they only learn to recognize patterns within the data they’re given. If a neural network is trained only on pictures of cats and dogs, it won’t understand that cats and dogs are animals—it simply knows how to identify patterns specific to those images. Challenges and Limitations While neural networks are powerful, they have their limitations: - Data-Hungry: Neural networks require large amounts of labeled data to learn effectively. - Black Box Nature: It’s difficult to understand exactly how a neural network arrives at its decisions, which can be a drawback in areas like medicine, where interpretability is crucial. - Computationally Intensive: Neural networks often require significant computing resources, especially as they grow larger and more complex. Despite these challenges, neural networks continue to advance, and they’re at the heart of many of the technologies shaping our world. In Summary A neural network is a model inspired by the human brain, made up of interconnected layers that work together to learn patterns and make predictions. With input, hidden, and output layers, neural networks transform raw data into insights, adjusting their internal “weights” over time to improve their accuracy. They’re used in fields as diverse as healthcare, finance, entertainment, and beyond. While they’re complex and have limitations, neural networks are powerful tools for tackling some of today’s most challenging problems, driving innovation in countless ways. So next time you see a recommendation on your favorite streaming service or talk to a voice assistant, remember: behind the scenes, a neural network might be hard at work, learning and improving just for you. Read the full article
#AIforBeginners#AITutorial#ArtificialIntelligence#Backpropagation#ConvolutionalNeuralNetwork#DeepLearning#HiddenLayer#ImageRecognition#InputLayer#MachineLearning#MachineLearningTutorial#NaturalLanguageProcessing#NeuralNetwork#NeuralNetworkBasics#NeuralNetworkLayers#NeuralNetworkTraining#OutputLayer#PatternRecognition#RecurrentNeuralNetwork#SpeechRecognition#WeightsandBiases
0 notes
Link
#ADAS#advanceddriver-assistancesystems#AEB#AI#artificialintelligence#automaticemergencybraking#CNN#convolutionalneuralnetwork#EuroNCAP#FederalMotorVehicleSafetyStandard#Fisker#FMVSS127#Futurride#Level4roboshuttle#MagnaInternational#Mercedes-Benz#Michigan#NationalHighwayTrafficSafetyAdministration#pedestrianAEB#Raytheon#sustainablemanufacturing#sustainablematerials#sustainablemobility#thermalsensing#Troy#U.S.DepartmentofTransportation
0 notes
Text
youtube
Discover how to build a CNN model for skin melanoma classification using over 20,000 images of skin lesions
We'll begin by diving into data preparation, where we will organize, clean, and prepare the data form the classification model.
Next, we will walk you through the process of build and train convolutional neural network (CNN) model. We'll explain how to build the layers, and optimize the model.
Finally, we will test the model on a new fresh image and challenge our model.
Check out our tutorial here : https://youtu.be/RDgDVdLrmcs
Enjoy
Eran
#Python #Cnn #TensorFlow #deeplearning #neuralnetworks #imageclassification #convolutionalneuralnetworks #SkinMelanoma #melonomaclassification
#artificial intelligence#convolutional neural network#deep learning#python#tensorflow#machine learning#Youtube
3 notes
·
View notes
Text
Advance Your Skills in AI and Machine Learning
LAOMUSIC ARTS 2024 presents
I just finished the learning path “Advance Your Skills in AI and Machine Learning”! 16 Courses with 21h 46m and my 18 brand new skills: appliedmachinelearning, machinelearning, deeplearning, reinforcementlearning, neuralnetworks, generativeai, generativeadversarialnetworks, artificialneuralnetworks, convolutionalneuralnetworks, itprocessautomation, artificialintelligence, itautomation, mlops, artificialintelligencefordesign, userexperiencedesign, responsibleai, businessprocessautomation, datagovernance
In the rapidly expanding universe of artificial intelligence and machine learning, there's a lot to learn and to stay on top of. Use the range of courses in this learning path to augment your skills related to AI, ML, and data science by understanding generative models and exploring the fields of MLOps and Responsible AI. Gain hands-on training with generative models. Refine your skills in deep learning and neural networks. Explore the developing fields of MLOps and responsible AI.
#lao #music #laomusic #laomusicarts #laomusicArts #ai #appliedmachinelearning #machinelearning #deeplearning #reinforcementlearning #neuralnetworks #python #generativeai #generativeadversarialnetworks #artificialneuralnetworks #convolutionalneuralnetworks #itprocessautomation #artificialintelligence #itautomation #mlops #artificialintelligencefordesign #userexperiencedesign #responsibleai #businessprocessautomation #datagovernance
Check it out:
1 note
·
View note
Text
Facial Segmentation Service:-
Facial segmentation is the process of separating different parts of a face image into distinct regions or segments. This can be accomplished using machine learning techniques.
Visit: https://gts.ai/
#facialsegmentation#machinelearning#computervision#convolutionalneuralnetworks#facerecognition#artificialintelligence#deeplearning#imageprocessing#precisionsegmentation#faceanalysis#facialfeatures#FacialSegmentation
0 notes
Photo

Today I'm going to talk about Urban Planning and Artifcial Intelligence. Especially about Data Bias and how sloppy labeling can affect design goals massively. This is for a get together called "Cidade Habitação e Participação - politicas publicas para construir uma cidade justa para o seculo XXI" organized by the "Laboratório de Habitación Basica" Please join us on zoom starting 4pm EST: https://us02web.zoom.us/j/84134631331 pw: city #artificialintelligence #architecture #design #agency #authorship #MachineLearning #neuralnetworks #posthuman #postdigital #school #neuralarchitecture #archdaily #spanarch #archinect #archilovers #ConvolutionalNeuralNetworks #3DgraphCNN #settlement #architecture_theory #ML #AI (at Ann Arbor, Michigan) https://www.instagram.com/p/CJJ4FyssSQj/?igshid=nv85cv807sg7
#artificialintelligence#architecture#design#agency#authorship#machinelearning#neuralnetworks#posthuman#postdigital#school#neuralarchitecture#archdaily#spanarch#archinect#archilovers#convolutionalneuralnetworks#3dgraphcnn#settlement#architecture_theory#ml#ai
0 notes
Video
Using Deep Learning to detect a face. It gives much better accuracy as compare to OpenCV's Haar Cascades. ⠀ ⠀ Also, at the same time (in the background) building my custom dataset of images to further perform facial recognition and collect training data.⠀ ⠀ Thanks for Adrian @PyImageSearch for his amazing tutorials. ⠀ ⠀ ⠀ ⠀ #WeekEndGrind #WeekEnd #WeekEndProject #DeepLearning #AI #ArtificalIntelligence #MachineLearning #NerualNetwork #NN #CaffeNet #OpenCV #FaceDetection #FaceRecognition #DeepNeuralNetwork #DNN #ConvolutionalNeuralNetwork #CNN #CaffeNet #OpenCV2 #ComputerVision (at Brampton, Ontario) https://www.instagram.com/p/B5SaITAHJT5/?igshid=7s79ggft6z0u
#weekendgrind#weekend#weekendproject#deeplearning#ai#artificalintelligence#machinelearning#nerualnetwork#nn#caffenet#opencv#facedetection#facerecognition#deepneuralnetwork#dnn#convolutionalneuralnetwork#cnn#opencv2#computervision
2 notes
·
View notes
Video
This painting is my personal favorite. When I was conceiving the idea, I felt a strong drive to achieve beauty in intensity and I kept working on this painting for months till the point when I felt totally satisfied with the result. Here I present before you an output from running style transfer on the same artwork. A lot of appreciation and thanks goes to Ankita @bipolarraf for being so creative in her photographs. May her beauty shine forever. #science #art #neuralstyletransfer #painting #photograph #nst #technology #ai #computer #artificialintelligence #beauty #mumbai #india #styletransfer #computerscience #tensorflow #neuralnetwork #cnn #convolutionalneuralnetwork (at India Mumbai) https://www.instagram.com/p/B3OuYKXlkZ1/?igshid=juuj33mtfbaq
#science#art#neuralstyletransfer#painting#photograph#nst#technology#ai#computer#artificialintelligence#beauty#mumbai#india#styletransfer#computerscience#tensorflow#neuralnetwork#cnn#convolutionalneuralnetwork
2 notes
·
View notes
Photo

Hello India, Welcome to India’s first podacst specialized in artificial intellegnce, machine learning and python programming. You will get one episode everyday on different topic but related to artificial intelligence. . . Follow this page for updates and to get some of our best podcast. . . . Just go to spotify and search for AI Hindi show you will get our podcast. Thank you. . . . . #artificialintelligence #machinelearning #pythonprogramming #india #hindi #pythoncode #pythonindia #indianpython #aihindishow #python3 #artificialintelligenceai #artificialinteligence #matplotlib #seaborn #numpy #linux #convolutionalneuralnetwork #neuralnetworks #neuramisdeep #machinelearningalgorithms #data #datascience #datascienceindia #bigdata #bigdataanalytics (at India) https://www.instagram.com/p/B5VCp0blbVI/?igshid=1qa5ebsmnkffs
#artificialintelligence#machinelearning#pythonprogramming#india#hindi#pythoncode#pythonindia#indianpython#aihindishow#python3#artificialintelligenceai#artificialinteligence#matplotlib#seaborn#numpy#linux#convolutionalneuralnetwork#neuralnetworks#neuramisdeep#machinelearningalgorithms#data#datascience#datascienceindia#bigdata#bigdataanalytics
1 note
·
View note
Text
Intel Geti Platform: Next-Gen Computer Vision AI Software

What is Intel Geti Software?
Intel’s latest software can create computer vision models with less data and in a quarter of the time. Teams can now create unique AI models at scale with to this software, which streamlines time-consuming data labeling, model training, and optimization processes throughout the AI model creation process.
Create Robust AI Models for Computer Vision
Small data sets, active learning, an easy-to-use user interface, and integrated collaboration make training AI models straightforward.
Automate and Digitize Projects More Quickly
Teams can rapidly create vision models for a variety of processes, such as identifying faulty parts in a production line, cutting downtime on the factory floor, automating inventory management, or other digitization and automation projects, by streamlining labor-intensive data upload, labeling, training, model optimization, and retraining tasks. The Intel Geti software simplifies the process and significantly reduces the time-to-value of developing AI models.
Core Capabilities Behind the Next-Generation Computer Vision AI Software
Interactive Model Training
Use as little as 20–30 photos to begin annotating data, and then use active learning to train the model as it learns.
Multiple Computer Vision Tasks
Build models for AI tasks such as anomaly detection, object identification, categorization, and semantic segmentation.
Task Chaining
By chaining two or more tasks together, you may train your model into a multistep, intelligent application without writing extra code.
Smart Annotations
Use professional drawing tools like a pencil, polygon tool, and OpenCV GrabCut to quickly annotate data and segment pictures.
Production-Ready Models
Produce deep learning models as optimized models for the OpenVINO toolkit to operate on Intel architecture CPUs, GPUs, and VPUs, or in TensorFlow or PyTorch formats, if available.
Hyperparameter Optimization
The model’s learning process depends on the hyperparameters being adjusted. The work of a data scientist is made simpler by Intel Geti software’s integrated optimization.
Rotated Bounding Boxes
The accuracy and ease of training are extended to datasets with non-axis-aligned pictures with to support for rotated bounding boxes.
Model Evaluation
Assessment of the Model thorough statistics to evaluate the success of your model.
Flexible Deployment Options to Get You Started
Simply set up your environment and infrastructure and prepare to install Intel Geti software, regardless of whether you want to use your system infrastructure inside your network or benefit from the cloud virtual machine without managing infrastructure.
On Premise
Virtual Machine
Enabling Collaboration that Adds Value
In a single instance, cross-functional AI teams work together to examine outcomes instantly. Team members with little to no familiarity with AI may assist in training computer vision models with to the graphical user interface. Drag-and-drop model training is made easy by enabling features like object identification helpers, drawing features, and annotation assistants.
Intel Geti Platform Use Cases
Convolutional neural network models are retrained using the Intel Geti platform for important computer vision applications, such as:
Semantic and instance segmentation, including counting
Single-label, multi-label, and hierarchical classification
Anomaly classification, detection, and segmentation
Axis-aligned and rotational object detection
Task chaining is also supported, allowing you to create intelligent, multi-step applications.
Manufacturing: Create AI for industrial controls, worker safety systems, autonomous assembly, and defect detection.
Smart Agriculture: Create models for self-governing devices that can assess crop health, detect weeds and pests, apply spot fertilizer and treatment, and harvest crops.
Smart Cities: Create AI-powered traffic-management systems to automatically route traffic, create emergency-recognition and response systems, and utilize video data to enhance safety in real time.
Retail: Create AI for accurate, touchless checkout, better safety and loss prevention, and self-governing inventory management systems.
Video Safety: Create task-specific models for the identification of safety gear, PPE, social distancing, and video analytics.
Medical Care: Create models to help with diagnosis and procedures, assess lab data and count cultures, identify abnormalities in medical pictures, and expedite medical research.
REST APIs and a software development kit (SDK) may be used to incorporate all of these models into your pipeline, or you can use the OpenVINO toolkit to distribute them.
FAQs
What types of data can Intel Geti handle?
Text, photos, videos, and structured data are just a few of the data kinds that Intel Geti can manage. It excels in processing unstructured data, such as audio and visual inputs for deep learning applications.
Read more on govindhtech.com
#IntelGetiPlatform#NextGenComputer#VisionAISoftware#AImodels#ComputerVision#CreateRobustAIModels#DigitizeProjects#OpenVINO#deeplearning#CoreCapabilitiesBehind#data#IntelGeti#technology#TensorFlow#virtualmachine#Convolutionalneuralnetwork#technews#news#govindhtech
0 notes
Text
Nucot training in Data science with python and IT Service management.
#datascience#python#datasciencewithpython#data#programmer#ML#computerscience#artificialintelligence#bigdata#machinelearning#learndatascience#datasciencetraining#dataanalytics#entrepreneur#datascientists#deeplearning#bigdataanalytics#neuralnetworks#naturallanguageprocessing#convolutionalneuralnetwork#softwareengineer#computervision#nocut
1 note
·
View note