#DataCollection
Explore tagged Tumblr posts
Text
Why do online surveys produce bad data? Learn about common pitfalls, their impact on research, and actionable solutions for cleaner, more reliable data.
To know more: data analytics services
fraud prevention solution
2 notes
·
View notes
Text
The Ultimate Data Collection Handbook: Exploring Methods, Types, and Advantages
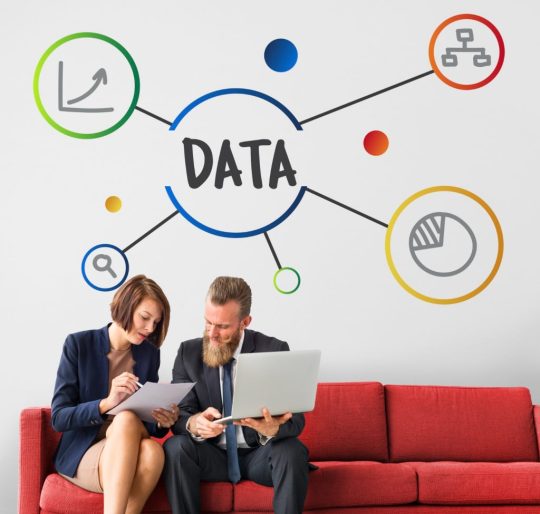
Data collection is a fundamental part of any research, business strategy, or decision-making process. Whether you're a student, a professional, or just curious about how data is gathered and used, understanding the basics of data collection can be incredibly useful. In this guide, we'll explore the methods, types, and benefits of data collection in a way that’s easy to understand.
What is Data Collection?
Data collection is the process of gathering information to answer specific questions or to support decision-making. This information, or data, can come from various sources and can be used to make informed decisions, conduct research, or solve problems.
Methods of Data Collection
Surveys and Questionnaires
What Are They? Surveys and questionnaires are tools used to gather information from people. They can be distributed in person, by mail, or online.
How Do They Work? Respondents answer a series of questions that provide insights into their opinions, behaviors, or experiences.
When to Use Them? Use surveys and questionnaires when you need to gather opinions or experiences from a large group of people.
Interviews
What Are They? Interviews involve asking questions to individuals in a one-on-one setting or in a group discussion.
How Do They Work? The interviewer asks questions and records the responses, which can be either structured (with set questions) or unstructured (more conversational).
When to Use Them? Use interviews when you need detailed, qualitative insights or when you want to explore a topic in depth.
Observations
What Are They? Observations involve watching and recording behaviors or events as they happen.
How Do They Work? The observer notes what is happening without interfering or influencing the situation.
When to Use Them? Use observations when you need to see actual behavior or events in their natural setting.
Experiments
What Are They? Experiments involve manipulating variables to see how changes affect outcomes.
How Do They Work? Researchers control certain variables and observe the effects on other variables to establish cause-and-effect relationships.
When to Use Them? Use experiments when you need to test hypotheses and understand the relationships between variables.
Secondary Data Analysis
What Is It? This method involves analyzing data that has already been collected by someone else.
How Does It Work? Researchers use existing data from sources like government reports, research studies, or company records.
When to Use It? Use secondary data analysis when you need historical data or when primary data collection is not feasible.
Types of Data
Quantitative Data
What Is It? Quantitative data is numerical and can be measured or counted.
Examples: Age, income, number of products sold.
Use It When: You need to quantify information and perform statistical analysis.
Qualitative Data
What Is It? Qualitative data is descriptive and involves characteristics that can be observed but not measured numerically.
Examples: Customer feedback, interview responses, descriptions of behavior.
Use It When: You need to understand concepts, opinions, or experiences.
Benefits of Data Collection
Informed Decision-Making
Data provides insights that help individuals and organizations make informed decisions based on evidence rather than guesswork.
Identifying Trends and Patterns
Collecting data allows you to identify trends and patterns that can inform future actions or strategies.
Improving Services and Products
By understanding customer needs and preferences through data, businesses can improve their products and services to better meet those needs.
Supporting Research and Development
Data is crucial for researchers to test hypotheses, validate theories, and advance knowledge in various fields.
Enhancing Efficiency
Data helps in streamlining processes and improving operational efficiency by highlighting areas that need attention or improvement.
Conclusion
Understanding the methods, types, and benefits of data collection can greatly enhance your ability to gather useful information and make informed decisions. Whether you're conducting research, running a business, or just curious about the world around you, mastering data collection is a valuable skill. Use this guide to get started and explore the many ways data can help you achieve your goals.
To know more: A Guide to Data Collection: Methods, Types, and Benefits
Outsource Data Collection Services
5 notes
·
View notes
Text
Your Guide to Success in Quantitative Research: 8 Practical Tips
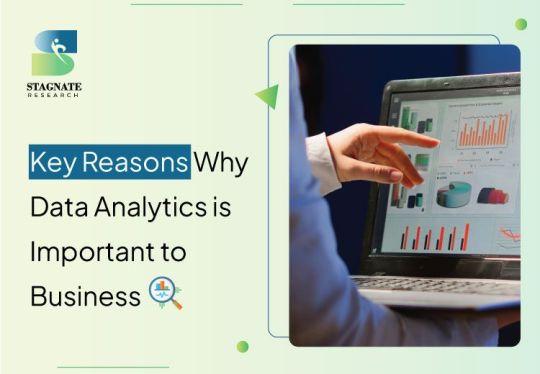
Quantitative research plays a crucial role in fields like social sciences, business, healthcare, and education. It provides numerical data that can be analyzed statistically to identify patterns, relationships, and trends. However, excelling in quantitative research requires more than just crunching numbers.
1. Start with a Clear Research Question
The foundation of any successful research is a well-defined research question. This question guides the entire study, determining your methodology, data collection, and analysis. Ensure that your research question is specific, measurable, and aligned with the purpose of your study.
For example, instead of asking, "How do students perform in school?" a clearer question might be, "What is the relationship between study hours and academic performance in high school students?"
Tip: Before starting, spend time refining your question. This will save you time and effort during the research process.
2. Choose the Right Research Design
Quantitative research can take many forms, including experiments, surveys, and observational studies. Choosing the right design depends on your research objectives and the type of data you need. Are you testing a hypothesis?
Tip: Match your research design with your objectives to ensure you’re collecting the right kind of data.
3. Use Valid and Reliable Instruments
The tools you use to gather data—whether they’re questionnaires, tests, or measuring devices—must be both valid (measuring what you intend to measure) and reliable (producing consistent results over time).
Tip: If you’re developing your own instrument, pilot it first with a small group to check its validity and reliability. If using an existing tool, review past studies to confirm it works well for your research population.
4. Select an Appropriate Sample Size
A common mistake in quantitative research is working with a sample size that’s too small, which can lead to unreliable or inconclusive results. On the other hand, excessively large samples can waste resources. To avoid these pitfalls, conduct a power analysis to determine the optimal sample size for your study.
Tip: Use tools like G*Power to calculate the right sample size based on your research goals and the expected effect size. This ensures your findings are statistically significant and applicable to a larger population.
5. Ensure Random Sampling for Representativeness
Your findings will only be meaningful if your sample represents the broader population you’re studying. Random sampling ensures that every individual in the population has an equal chance of being selected, reducing bias and increasing the generalizability of your results.
Tip: Use random sampling methods (e.g., simple random sampling, stratified random sampling) to ensure your data is as representative as possible.
6. Minimize Bias in Data Collection
Bias can creep into any research process, affecting the accuracy and fairness of your results. To reduce bias, carefully design your data collection process. For example, avoid leading questions in surveys and standardize how data is collected across all participants to prevent interviewer or observer bias.
Tip: Blind or double-blind studies can help minimize bias, especially in experiments where participants or researchers might be influenced by expectations.
7. Analyze Data Properly with the Right Statistical Tools
Once you’ve collected your data, the next step is analysis. Choosing the right statistical tests is essential to interpret your findings correctly. Descriptive statistics (like means and frequencies) give a broad overview, while inferential statistics (like t-tests, chi-squares, or regression analyses) help determine whether your findings are statistically significant.
Tip: If you’re unsure which test to use, consult a statistician or use resources like statistical decision trees to guide your choice based on your data type and research questions.
8. Interpret Results with Context and Caution
After analyzing your data, it’s tempting to jump to conclusions. However, quantitative research is not just about the numbers; it’s about what those numbers mean in context. Always interpret your results in relation to your research question and the existing body of knowledge.
Be cautious when generalizing your findings, especially if your sample size is small or non-representative. Additionally, consider the limitations of your study—were there any confounding variables, measurement errors, or external factors that might have influenced your results?
Tip: Be transparent about the limitations of your study. Acknowledging them strengthens the credibility of your research.
Conclusion
Mastering quantitative research requires attention to detail, a solid understanding of statistical methods, and a commitment to rigor throughout the process. By following these 8 practical tips—starting with a clear question, choosing the right design, using valid instruments, selecting the appropriate sample, minimizing bias, analyzing correctly, and interpreting results carefully—you’ll be well on your way to conducting successful and impactful quantitative research.
Read more: https://stagnateresearch.com/blog/how-to-excel-in-quantitative-research-8-essential-tips-for-success/
Also read: Project Management Service Company
data processing in research services
#onlineresearch#marketresearch#datacollection#project management#survey research#data collection company#business
3 notes
·
View notes
Text
EXPLANATION OF DATA SCIENCE
Data science
In today's data-driven world, the term "data science" has become quite the buzzword. At its core, data science is all about turning raw data into valuable insights. It's the art of collecting, analyzing, and interpreting data to make informed decisions. Think of data as the ingredients, and data scientists as the chefs who whip up delicious insights from them.
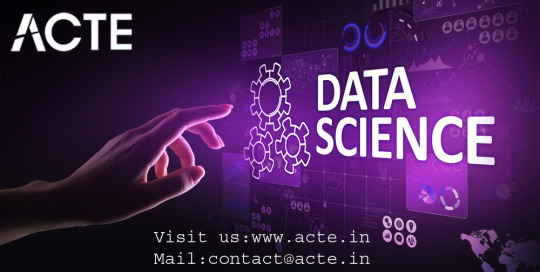
The Data Science Process
Data Collection: The journey begins with collecting data from various sources. This can include anything from customer surveys and social media posts to temperature readings and financial transactions.
Data Cleaning: Raw data is often messy and filled with errors and inconsistencies. Data scientists clean, preprocess, and organize the data to ensure it's accurate and ready for analysis.
Data Analysis: Here's where the real magic happens. Data scientists use statistical techniques and machine learning algorithms to uncover patterns, trends, and correlations in the data. This step is like searching for hidden gems in a vast treasure chest of information.
Data Visualization: Once the insights are extracted, they need to be presented in a way that's easy to understand. Data scientists create visualizations like charts and graphs to communicate their findings effectively.
Decision Making: The insights obtained from data analysis empower businesses and individuals to make informed decisions. For example, a retailer might use data science to optimize their product inventory based on customer preferences.
Applications of Data Science
Data science has a wide range of applications in various industries.
Business: Companies use data science to improve customer experiences, make marketing strategies more effective, and enhance operational efficiency.
Healthcare: Data science helps in diagnosing diseases, predicting patient outcomes, and even drug discovery.
Finance: In the financial sector, data science plays a crucial role in fraud detection, risk assessment, and stock market predictions.
Transportation: Transportation companies use data science for route optimization, predicting maintenance needs, and even developing autonomous vehicles.
Entertainment: Streaming platforms like Netflix use data science to recommend movies and TV shows based on your preferences.
Why Data Science Matters
Data science matters for several reasons:
Informed Decision-Making: It enables individuals and organizations to make decisions based on evidence rather than guesswork.
Innovation: Data science drives innovation by uncovering new insights and opportunities.
Efficiency: Businesses can streamline their operations and reduce costs through data-driven optimizations.
Personalization: It leads to personalized experiences for consumers, whether in the form of product recommendations or targeted advertisements.
In a nutshell, data science is the process of turning data into actionable insights. It's the backbone of modern decision-making, fueling innovation and efficiency across various industries. So, the next time you hear the term "data science," you'll know that it's not just a buzzword but a powerful tool that helps shape our data-driven world.
Overall, data science is a highly rewarding career that can lead to many opportunities. If you're interested in this field and have the right skills, you should definitely consider it as a career option. If you want to gain knowledge in data science, then you should contact ACTE Technologies. They offer certifications and job placement opportunities. Experienced teachers can help you learn better. You can find these services both online and offline. Take things step by step and consider enrolling in a course if you’re interested.
Thanks for reading.
2 notes
·
View notes
Text
Market Research Services
Are you looking to gain a competitive edge in your industry? 🚀
Marketing research services provide invaluable insights into consumer behavior, market trends, and competitor analysis. With this information, you can tailor your marketing strategies to target the right audience, optimize your campaigns, and identify lucrative opportunities for growth. As a Marketing outsourcing company, Navigant offers a full suite of market research operations and data analytics solutions to clients.
Just let us know where you need support, and we’ll step in and take care of the rest.
Book A Meeting: https://meetings.hubspot.com/sonal-arora Web: www.navigant.in Email us at: [email protected] Cell: +91 9354739641
#Navigant#DataDrivenStrategies#BusinessGrowth#marketresearch#marketing#business#research#digitalmarketing#marketingstrategy#branding#market#entrepreneur#startup#dataanalytics#data#insights#marketanalysis#technology#datacollection#socialmediamarketing#entrepreneurship#customerexperience#leadgeneration
3 notes
·
View notes
Text
Get your data-gathering groove on with our "Collecting Data Is My Cardio" shirt! This tee is the perfect gift for behavior analysts, BCBA-certified professionals, and special education teachers. Embrace your passion for data-driven insights and show your dedication to autism awareness and ABA therapy. Wear it proudly as a statement of your commitment to making a positive impact!
#datacollection#behavioranalyst#BCBA#specialeducation#autismawareness#ABATherapist#tshirt#giftideas#datadriven#passion#dedication
2 notes
·
View notes
Text
With the proliferation of cloud native applications, organizations today are dealing with an abundance of data from various sources. Collecting and analyzing this data effectively has become crucial for businesses to gain insights and make data-driven decisions. In this blog post, we will explore the best practices for data collection and analysis in the age of numerous cloud native applications, and how Crest Data Systems’ solutions can help organizations optimize their data strategies in this evolving landscape.
2 notes
·
View notes
Text
Haptic Feedback in Data Exploration: Feeling the Pulse of Market Trends

Discover the potential of haptic feedback in data exploration. Learn how analysts are using VR gloves and tactile displays to physically "feel" market trends and identify outliers in consumer behavior datasets.
Link : https://maction.com/haptic-feedback-in-data-exploration-feeling-the-pulse-of-market-trends/
#marketresearch#marketing#business#research#digitalmarketing#marketingstrategy#branding#market#entrepreneur#startup#covid#survey#dataanalytics#data#insights#marketanalysis#technology#surveys#datacollection#socialmediamarketing#entrepreneurship#paidsurveys#customerexperience#leadgeneration#dataanalysis#businessstrategy#socialmedia#contentmarketing#emailmarketing#marketingdigital
0 notes
Text
How To Scrape Airbnb Listing Data Using Python And Beautiful Soup: A Step-By-Step Guide

The travel industry is a huge business, set to grow exponentially in coming years. It revolves around movement of people from one place to another, encompassing the various amenities and accommodations they need during their travels. This concept shares a strong connection with sectors such as hospitality and the hotel industry.
Here, it becomes prudent to mention Airbnb. Airbnb stands out as a well-known online platform that empowers people to list, explore, and reserve lodging and accommodation choices, typically in private homes, offering an alternative to the conventional hotel and inn experience.
Scraping Airbnb listings data entails the process of retrieving or collecting data from Airbnb property listings. To Scrape Data from Airbnb's website successfully, you need to understand how Airbnb's listing data works. This blog will guide us how to scrape Airbnb listing data.
What Is Airbnb Scraping?

Airbnb serves as a well-known online platform enabling individuals to rent out their homes or apartments to travelers. Utilizing Airbnb offers advantages such as access to extensive property details like prices, availability, and reviews.
Data from Airbnb is like a treasure trove of valuable knowledge, not just numbers and words. It can help you do better than your rivals. If you use the Airbnb scraper tool, you can easily get this useful information.
Effectively scraping Airbnb’s website data requires comprehension of its architecture. Property information, listings, and reviews are stored in a database, with the website using APIs to fetch and display this data. To scrape the details, one must interact with these APIs and retrieve the data in the preferred format.
In essence, Airbnb listing scraping involves extracting or scraping Airbnb listings data. This data encompasses various aspects such as listing prices, locations, amenities, reviews, and ratings, providing a vast pool of data.
What Are the Types of Data Available on Airbnb?

Navigating via Airbnb's online world uncovers a wealth of data. To begin with, property details, like data such as the property type, location, nightly price, and the count of bedrooms and bathrooms. Also, amenities (like Wi-Fi, a pool, or a fully-equipped kitchen) and the times for check-in and check-out. Then, there is data about the hosts and guest reviews and details about property availability.
Here's a simplified table to provide a better overview:
Property Details Data regarding the property, including its category, location, cost, number of rooms, available features, and check-in/check-out schedules.
Host Information Information about the property's owner, encompassing their name, response time, and the number of properties they oversee.
Guest Reviews Ratings and written feedback from previous property guests.
Booking Availability Data on property availability, whether it's available for booking or already booked, and the minimum required stay.
Why Is the Airbnb Data Important?

Extracting data from Airbnb has many advantages for different reasons:
Market Research
Scraping Airbnb listing data helps you gather information about the rental market. You can learn about prices, property features, and how often places get rented. It is useful for understanding the market, finding good investment opportunities, and knowing what customers like.
Getting to Know Your Competitor
By scraping Airbnb listings data, you can discover what other companies in your industry are doing. You'll learn about their offerings, pricing, and customer opinions.
Evaluating Properties
Scraping Airbnb listing data lets you look at properties similar to yours. You can see how often they get booked, what they charge per night, and what guests think of them. It helps you set the prices right, make your property better, and make guests happier.
Smart Decision-Making
With scraped Airbnb listing data, you can make smart choices about buying properties, managing your portfolio, and deciding where to invest. The data can tell you which places are popular, what guests want, and what is trendy in the vacation rental market.
Personalizing and Targeting
By analyzing scraped Airbnb listing data, you can learn what your customers like. You can find out about popular features, the best neighborhoods, or unique things guests want. Next, you can change what you offer to fit what your customers like.
Automating and Saving Time
Instead of typing everything yourself, web scraping lets a computer do it for you automatically and for a lot of data. It saves you time and money and ensures you have scraped Airbnb listing data.
Is It Legal to Scrape Airbnb Data?
Collecting Airbnb listing data that is publicly visible on the internet is okay, as long as you follow the rules and regulations. However, things can get stricter if you are trying to gather data that includes personal info, and Airbnb has copyrights on that.
Most of the time, websites like Airbnb do not let automatic tools gather information unless they give permission. It is one of the rules you follow when you use their service. However, the specific rules can change depending on the country and its policies about automated tools and unauthorized access to systems.
How To Scrape Airbnb Listing Data Using Python and Beautiful Soup?

Websites related to travel, like Airbnb, have a lot of useful information. This guide will show you how to scrape Airbnb listing data using Python and Beautiful Soup. The information you collect can be used for various things, like studying market trends, setting competitive prices, understanding what guests think from their reviews, or even making your recommendation system.
We will use Python as a programming language as it is perfect for prototyping, has an extensive online community, and is a go-to language for many. Also, there are a lot of libraries for basically everything one could need. Two of them will be our main tools today:
Beautiful Soup — Allows easy scraping of data from HTML documents
Selenium — A multi-purpose tool for automating web-browser actions
Getting Ready to Scrape Data
Now, let us think about how users scrape Airbnb listing data. They start by entering the destination, specify dates then click "search." Airbnb shows them lots of places.
This first page is like a search page with many options. But there is only a brief data about each.
After browsing for a while, the person clicks on one of the places. It takes them to a detailed page with lots of information about that specific place.
We want to get all the useful information, so we will deal with both the search page and the detailed page. But we also need to find a way to get info from the listings that are not on the first search page.
Usually, there are 20 results on one search page, and for each place, you can go up to 15 pages deep (after that, Airbnb says no more).
It seems quite straightforward. For our program, we have two main tasks:
looking at a search page, and getting data from a detailed page.
So, let us begin writing some code now!
Getting the listings
Using Python to scrape Airbnb listing data web pages is very easy. Here is the function that extracts the webpage and turns it into something we can work with called Beautiful Soup.
def scrape_page(page_url): """Extracts HTML from a webpage""" answer = requests.get(page_url) content = answer.content soup = BeautifulSoup(content, features='html.parser') return soup
Beautiful Soup helps us move around an HTML page and get its parts. For example, if we want to take the words from a “div” object with a class called "foobar" we can do it like this:
text = soup.find("div", {"class": "foobar"}).get_text()
On Airbnb's listing data search page, what we are looking for are separate listings. To get to them, we need to tell our program which kinds of tags and names to look for. A simple way to do this is to use a tool in Chrome called the developer tool (press F12).
The listing is inside a "div" object with the class name "8s3ctt." Also, we know that each search page has 20 different listings. We can take all of them together using a Beautiful Soup tool called "findAll.
def extract_listing(page_url): """Extracts listings from an Airbnb search page""" page_soup = scrape_page(page_url) listings = page_soup.findAll("div", {"class": "_8s3ctt"}) return listings
Getting Basic Info from Listings
When we check the detailed pages, we can get the main info about the Airbnb listings data, like the name, total price, average rating, and more.
All this info is in different HTML objects as parts of the webpage, with different names. So, we could write multiple single extractions -to get each piece:
name = soup.find('div', {'class':'_hxt6u1e'}).get('aria-label') price = soup.find('span', {'class':'_1p7iugi'}).get_text() ...
However, I chose to overcomplicate right from the beginning of the project by creating a single function that can be used again and again to get various things on the page.
def extract_element_data(soup, params): """Extracts data from a specified HTML element"""
# 1. Find the right tag
if 'class' in params: elements_found = soup.find_all(params['tag'], params['class']) else: elements_found = soup.find_all(params['tag'])
# 2. Extract text from these tags
if 'get' in params: element_texts = [el.get(params['get']) for el in elements_found] else: element_texts = [el.get_text() for el in elements_found]
# 3. Select a particular text or concatenate all of them tag_order = params.get('order', 0) if tag_order == -1: output = '**__**'.join(element_texts) else: output = element_texts[tag_order] return output
Now, we've got everything we need to go through the entire page with all the listings and collect basic details from each one. I'm showing you an example of how to get only two details here, but you can find the complete code in a git repository.
RULES_SEARCH_PAGE = { 'name': {'tag': 'div', 'class': '_hxt6u1e', 'get': 'aria-label'}, 'rooms': {'tag': 'div', 'class': '_kqh46o', 'order': 0}, } listing_soups = extract_listing(page_url) features_list = [] for listing in listing_soups: features_dict = {} for feature in RULES_SEARCH_PAGE: features_dict[feature] = extract_element_data(listing, RULES_SEARCH_PAGE[feature]) features_list.append(features_dict)
Getting All the Pages for One Place
Having more is usually better, especially when it comes to data. Scraping Airbnb listing data lets us see up to 300 listings for one place, and we are going to scrape them all.
There are different ways to go through the pages of search results. It is easiest to see how the web address (URL) changes when we click on the "next page" button and then make our program do the same thing.
All we have to do is add a thing called "items_offset" to our initial URL. It will help us create a list with all the links in one place.
def build_urls(url, listings_per_page=20, pages_per_location=15): """Builds links for all search pages for a given location""" url_list = [] for i in range(pages_per_location): offset = listings_per_page * i url_pagination = url + f'&items_offset={offset}' url_list.append(url_pagination) return url_list
We have completed half of the job now. We can run our program to gather basic details for all the listings in one place. We just need to provide the starting link, and things are about to get even more exciting.
Dynamic Pages
It takes some time for a detailed page to fully load. It takes around 3-4 seconds. Before that, we could only see the base HTML of the webpage without all the listing details we wanted to collect.
Sadly, the "requests" tool doesn't allow us to wait until everything on the page is loaded. But Selenium does. Selenium can work just like a person, waiting for all the cool website things to show up, scrolling, clicking buttons, filling out forms, and more.
Now, we plan to wait for things to appear and then click on them. To get information about the amenities and price, we need to click on certain parts.
To sum it up, here is what we are going to do:
Start up Selenium.
Open a detailed page.
Wait for the buttons to show up.
Click on the buttons.
Wait a little longer for everything to load.
Get the HTML code.
Let us put them into a Python function.
def extract_soup_js(listing_url, waiting_time=[5, 1]): """Extracts HTML from JS pages: open, wait, click, wait, extract""" options = Options() options.add_argument('--headless') options.add_argument('--no-sandbox') driver = webdriver.Chrome(options=options) driver.get(listing_url) time.sleep(waiting_time[0]) try: driver.find_element_by_class_name('_13e0raay').click() except: pass # amenities button not found try: driver.find_element_by_class_name('_gby1jkw').click() except: pass # prices button not found time.sleep(waiting_time[1]) detail_page = driver.page_source driver.quit() return BeautifulSoup(detail_page, features='html.parser')
Now, extracting detailed info from the listings is quite straightforward because we have everything we need. All we have to do is carefully look at the webpage using a tool in Chrome called the developer tool. We write down the names and names of the HTML parts, put all of that into a tool called "extract_element_data.py" and we will have the data we want.
Running Multiple Things at Once
Getting info from all 15 search pages in one location is pretty quick. When we deal with one detailed page, it takes about just 5 to 6 seconds because we have to wait for the page to fully appear. But, the fact is the CPU is only using about 3% to 8% of its power.
So. instead of going to 300 webpages one by one in a big loop, we can split the webpage addresses into groups and go through these groups one by one. To find the best group size, we have to try different options.
from multiprocessing import Pool with Pool(8) as pool: result = pool.map(scrape_detail_page, url_list)
The Outcome
After turning our tools into a neat little program and running it for a location, we obtained our initial dataset.
The challenging aspect of dealing with real-world data is that it's often imperfect. There are columns with no information, many fields need cleaning and adjustments. Some details turned out to be not very useful, as they are either always empty or filled with the same values.
There's room for improving the script in some ways. We could experiment with different parallelization approaches to make it faster. Investigating how long it takes for the web pages to load can help reduce the number of empty columns.
To Sum It Up
We've mastered:
Scraping Airbnb listing data using Python and Beautiful Soup.
Handling dynamic pages using Selenium.
Running the script in parallel using multiprocessing.
Conclusion
Web scraping today offers user-friendly tools, which makes it easy to use. Whether you are a coding pro or a curious beginner, you can start scraping Airbnb listing data with confidence. And remember, it's not just about collecting data – it's also about understanding and using it.
The fundamental rules remain the same, whether you're scraping Airbnb listing data or any other website, start by determining the data you need. Then, select a tool to collect that data from the web. Finally, verify the data it retrieves. Using this info, you can make better decisions for your business and come up with better plans to sell things.
So, be ready to tap into the power of web scraping and elevate your sales game. Remember that there's a wealth of Airbnb data waiting for you to explore. Get started with an Airbnb scraper today, and you'll be amazed at the valuable data you can uncover. In the world of sales, knowledge truly is power.
0 notes
Text
7 Key Principles to Drive Success in Market Research
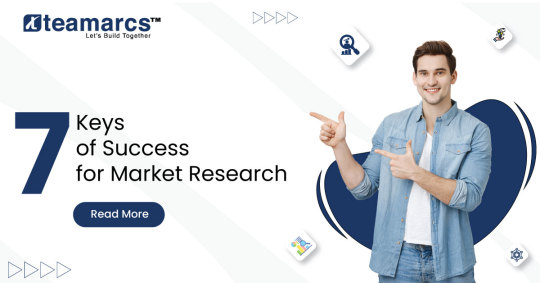
Market research plays a crucial role in guiding business strategies and decision-making. Here are seven key principles to ensure success in your market research efforts:
1. Define Clear Objectives
Start with well-defined goals. Understand what insights you need and how they will support your business decisions.
2. Know Your Audience
Identify and segment your target audience effectively. Tailor your research methods to align with their preferences and behaviors.
3. Choose the Right Methodology
Select the most suitable research approach, whether qualitative, quantitative, or a hybrid model, to ensure meaningful results.
4. Leverage Advanced Tools and Technology
Incorporate AI, big data, and analytics tools to enhance data accuracy and speed. Modern technology can streamline data collection and interpretation.
5. Ensure Data Quality
Prioritize data accuracy, relevance, and reliability. Scrutinize data sources and methodologies to avoid biased or incomplete insights.
6. Adhere to Ethical Standards
Respect privacy and comply with regulations like GDPR. Ethical practices build trust and credibility with your audience.
7. Translate Insights into Action
Insights are valuable only when applied. Create actionable recommendations and integrate them into your strategy to drive results.
By following these keys, businesses can elevate their market research practices and gain a competitive edge in their industry.
To know more: online market research platforms
online panel management platform
fraud detection and reporting tool
#market research#onlineresearch#samplemanagement#panelmanagement#communitypanel#datacollection#onlinesurvey#fraud detection#datainsights
3 notes
·
View notes
Text
The Next Wave: Business AI, Privacy, and Navigation Industry Evolution

As technology continues to advance, the interplay between artificial intelligence (AI), privacy, and the navigation industry is reshaping the business landscape. Here's a glimpse into how these elements are driving transformation:
1. AI's Expanding Role in Business
AI is revolutionizing industries with its ability to analyze vast datasets, predict trends, and automate processes. In navigation, AI enables real-time traffic updates, predictive routing, and autonomous vehicle advancements. Businesses leveraging AI gain a competitive edge by offering smarter, more efficient solutions.
2. Privacy Challenges and Innovations
With AI's reliance on data, privacy concerns are escalating. Regulations like GDPR and CCPA are setting stricter standards for data usage, urging companies to prioritize transparency and ethical practices. Balancing personalized services with data protection is becoming a critical challenge for businesses.
3. Navigation Industry Transformation
The navigation industry is evolving with AI-driven innovations, such as augmented reality (AR) mapping, enhanced geolocation services, and integration with IoT devices. These advancements not only improve user experiences but also open new avenues for businesses to deliver value in logistics, travel, and urban planning.
Conclusion
The convergence of AI, privacy considerations, and navigation technology signals a future filled with opportunities and challenges. Businesses that embrace AI while respecting privacy and adapting to industry changes will be well-positioned to thrive in this evolving landscape.
To know more: Survey Programming & Hosting Company
Outsource Data Collection Services
2 notes
·
View notes
Text
Safeguarding Privacy and Security in Fast-Paced Data Processing
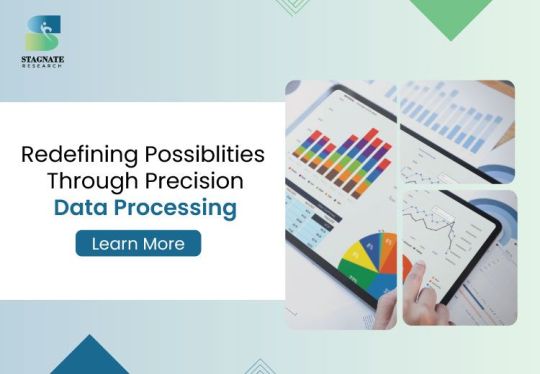
In the current era of data-centric operations, rapid data processing is essential across many industries, fostering innovation, improving efficiency, and offering a competitive advantage.
However, as the velocity and volume of data processing increase, so do the challenges related to data privacy and security. This article explores the critical issues and best practices in maintaining data integrity and confidentiality in the era of rapid data processing.
The Importance of Data Privacy and Security
Data privacy ensures that personal and sensitive information is collected, stored, and used in compliance with legal and ethical standards, safeguarding individuals' rights. Data security, on the other hand, involves protecting data from unauthorized access, breaches, and malicious attacks. Together, they form the foundation f trust in digital systems and processes.
Challenges in Rapid Data Processing
Volume and Velocity: The sheer amount of data generated and processed in real-time poses significant security risks.
Complex Data Environments: Modern data processing often involves distributed systems, cloud services, and multiple third-party vendors, creating a complex ecosystem that is challenging to secure comprehensively.
Regulatory Compliance: With stringent regulations like GDPR, CCPA, and HIPAA, organizations must ensure that their rapid data processing activities comply with data privacy laws.
Anonymization and De-identification: Rapid data processing systems must implement robust anonymization techniques to protect individual identities.
Best Practices for Ensuring Data Privacy and Security
Data Encryption: Encrypting data at rest and in transit is crucial to prevent unauthorized access.
Access Controls: Role-based access controls (RBAC) and multi-factor authentication (MFA) are effective measures.
Regular Audits and Monitoring: Continuous monitoring and regular security audits help identify and mitigate vulnerabilities in data processing systems.
Data Minimization: Collecting and processing only the necessary data reduces exposure risks.
Compliance Management: Staying updated with regulatory requirements and integrating compliance checks into the data processing workflow ensures adherence to legal standards.
Robust Anonymization Techniques: Employing advanced anonymization methods and regularly updating them can reduce the risk of re-identification.
Conclusion
As organizations leverage rapid data processing for competitive advantage, prioritizing data privacy and security becomes increasingly critical. By adopting best practices and staying vigilant against evolving threats, businesses can safeguard their data assets, maintain regulatory compliance, and uphold the trust of their customers and stakeholders.
To know more: project management service company
data processing services
Also read: https://stagnateresearch.com/blog/data-privacy-and-security-in-rapid-data-processing-a-guide-for-market-research-professionals/
#onlineresearch#marketresearch#datacollection#project management#survey research#data collection company
3 notes
·
View notes
Text

Empowering Equipment Health with Shop Floor Data Collection Software Boost equipment health with Shop Floor Data Collection Software for real-time insights, enhanced efficiency, and reduced downtime
For more details read our blog :
#machinemonitoring#machinemonitoringsystem#EquipmentHealthMonitoring#machinemonitoringsoftware#productionmonitoringsystem#oeemonitoringsystem#productionmonitoringsoftware#equipmentmonitoring#oeemanufacturing#smartmanufacturing#factorymonitoringsystem#datacollection#dataautomation#dataanalysistools
0 notes
Text
How Does AI Generate Human-Like Voices? 2025
How Does AI Generate Human-Like Voices? 2025
Artificial Intelligence (AI) has made incredible advancements in speech synthesis. AI-generated voices now sound almost indistinguishable from real human speech. But how does this technology work? What makes AI-generated voices so natural, expressive, and lifelike? In this deep dive, we’ll explore: ✔ The core technologies behind AI voice generation. ✔ How AI learns to mimic human speech patterns. ✔ Applications and real-world use cases. ✔ The future of AI-generated voices in 2025 and beyond.
Understanding AI Voice Generation
At its core, AI-generated speech relies on deep learning models that analyze human speech and generate realistic voices. These models use vast amounts of data, phonetics, and linguistic patterns to synthesize speech that mimics the tone, emotion, and natural flow of a real human voice. 1. Text-to-Speech (TTS) Systems Traditional text-to-speech (TTS) systems used rule-based models. However, these sounded robotic and unnatural because they couldn't capture the rhythm, tone, and emotion of real human speech. Modern AI-powered TTS uses deep learning and neural networks to generate much more human-like voices. These advanced models process: ✔ Phonetics (how words sound). ✔ Prosody (intonation, rhythm, stress). ✔ Contextual awareness (understanding sentence structure). 💡 Example: AI can now pause, emphasize words, and mimic real human speech patterns instead of sounding monotone.




2. Deep Learning & Neural Networks AI speech synthesis is driven by deep neural networks (DNNs), which work like a human brain. These networks analyze thousands of real human voice recordings and learn: ✔ How humans naturally pronounce words. ✔ The pitch, tone, and emphasis of speech. ✔ How emotions impact voice (anger, happiness, sadness, etc.). Some of the most powerful deep learning models include: WaveNet (Google DeepMind) Developed by Google DeepMind, WaveNet uses a deep neural network that analyzes raw audio waveforms. It produces natural-sounding speech with realistic tones, inflections, and even breathing patterns. Tacotron & Tacotron 2 Tacotron models, developed by Google AI, focus on improving: ✔ Natural pronunciation of words. ✔ Pauses and speech flow to match human speech patterns. ✔ Voice modulation for realistic expression. 3. Voice Cloning & Deepfake Voices One of the biggest breakthroughs in AI voice synthesis is voice cloning. This technology allows AI to: ✔ Copy a person’s voice with just a few minutes of recorded audio. ✔ Generate speech in that person’s exact tone and style. ✔ Mimic emotions, pitch, and speech variations. 💡 Example: If an AI listens to 5 minutes of Elon Musk’s voice, it can generate full speeches in his exact tone and speech style. This is called deepfake voice technology. 🔴 Ethical Concern: This technology can be used for fraud and misinformation, like creating fake political speeches or scam calls that sound real.
How AI Learns to Speak Like Humans
AI voice synthesis follows three major steps: Step 1: Data Collection & Training AI systems collect millions of human speech recordings to learn: ✔ Pronunciation of words in different accents. ✔ Pitch, tone, and emotional expression. ✔ How people emphasize words naturally. 💡 Example: AI listens to how people say "I love this product!" and learns how different emotions change the way it sounds. Step 2: Neural Network Processing AI breaks down voice data into small sound units (phonemes) and reconstructs them into natural-sounding speech. It then: ✔ Creates realistic sentence structures. ✔ Adds human-like pauses, stresses, and tonal changes. ✔ Removes robotic or unnatural elements. Step 3: Speech Synthesis Output After processing, AI generates speech that sounds fluid, emotional, and human-like. Modern AI can now: ✔ Imitate accents and speech styles. ✔ Adjust pitch and tone in real time. ✔ Change emotional expressions (happy, sad, excited).
Real-World Applications of AI-Generated Voices
AI-generated voices are transforming multiple industries: 1. Voice Assistants (Alexa, Siri, Google Assistant) AI voice assistants now sound more natural, conversational, and human-like than ever before. They can: ✔ Understand context and respond naturally. ✔ Adjust tone based on conversation flow. ✔ Speak in different accents and languages. 2. Audiobooks & Voiceovers Instead of hiring voice actors, AI-generated voices can now: ✔ Narrate entire audiobooks in human-like voices. ✔ Adjust voice tone based on story emotion. ✔ Sound different for each character in a book. 💡 Example: AI-generated voices are now used for animated movies, YouTube videos, and podcasts. 3. Customer Service & Call Centers Companies use AI voices for automated customer support, reducing costs and improving efficiency. AI voice systems: ✔ Respond naturally to customer questions. ✔ Understand emotional tone in conversations. ✔ Adjust voice tone based on urgency. 💡 Example: Banks use AI voice bots for automated fraud detection calls. 4. AI-Generated Speech for Disabled Individuals AI voice synthesis is helping people who have lost their voice due to medical conditions. AI-generated speech allows them to: ✔ Type text and have AI speak for them. ✔ Use their own cloned voice for communication. ✔ Improve accessibility for those with speech impairments. 💡 Example: AI helped Stephen Hawking communicate using a computer-generated voice.
The Future of AI-Generated Voices in 2025 & Beyond
AI-generated speech is evolving fast. Here’s what’s next: 1. Fully Realistic Conversational AI By 2025, AI voices will sound completely human, making robots and AI assistants indistinguishable from real humans. 2. Real-Time AI Voice Translation AI will soon allow real-time speech translation in different languages while keeping the original speaker’s voice and tone. 💡 Example: A Japanese speaker’s voice can be translated into English, but still sound like their real voice. 3. AI Voice in the Metaverse & Virtual Worlds AI-generated voices will power realistic avatars in virtual worlds, enabling: ✔ AI-powered characters with human-like speech. ✔ AI-generated narrators in VR experiences. ✔ Fully voiced AI NPCs in video games.
Final Thoughts
AI-generated voices have reached an incredible level of realism. From voice assistants to deepfake voice cloning, AI is revolutionizing how we interact with technology. However, ethical concerns remain. With the ability to clone voices and create deepfake speech, AI-generated voices must be used responsibly. In the future, AI will likely replace human voice actors, power next-gen customer service, and enable lifelike AI assistants. But one thing is clear—AI-generated voices are becoming indistinguishable from real humans. Read Our Past Blog: What If We Could Live Inside a Black Hole? 2025For more information, check this resource.
How Does AI Generate Human-Like Voices? 2025 - Everything You Need to Know
Understanding ai in DepthRelated Posts- How Does AI Generate Human-Like Voices? 2025 - How Does AI Generate Human-Like Voices? 2025 - How Does AI Generate Human-Like Voices? 2025 - How Does AI Generate Human-Like Voices? 2025 Read the full article
#1#2#2025-01-01t00:00:00.000+00:00#3#4#5#accent(sociolinguistics)#accessibility#aiaccelerator#amazonalexa#anger#animation#artificialintelligence#audiodeepfake#audiobook#avatar(computing)#blackhole#blog#brain#chatbot#cloning#communication#computer-generatedimagery#conversation#customer#customerservice#customersupport#data#datacollection#deeplearning
0 notes
Text
surveysurvey.com – The ideal domain for a survey company, data collection service, or research platform. Secure it now! https://www.godaddy.com/en-uk/domainsearch/find?domainToCheck=surveysurvey.com
0 notes
Text
15 Free Online Survey Tools & Apps You Should Try Today
Discover the best free survey tools and apps to collect customer insights. Get detailed comparisons to select the perfect solution.
#SurveyTools#OnlineSurveys#FreeSurveyApps#CustomerFeedback#SurveySoftware#DataCollection#MarketResearch#AIChatbot
0 notes