#Dataset
Explore tagged Tumblr posts
Text
Just a heads up to any non AI artists that use red bubble (among many more). They are allowing your work to be used by the LAION-5B data set for use in AI training. haveibeentrained.com is free to use
9 notes
·
View notes
Text
Dalla pagina Instagram di dailychatgpt
#chatgpt#dati#dataset#deepseek#proprietà dati#cittadinanza digitale#ai#ia#genai#artificial intelligence#intelligenza artificiale#stolen data
5 notes
·
View notes
Text

Tonight I am hunting down venomous and nonvenomous snake pictures that are under the creative commons of specific breeds in order to create one of the most advanced, in depth datasets of different venomous and nonvenomous snakes as well as a test set that will include snakes from both sides of all species. I love snakes a lot and really, all reptiles. It is definitely tedious work, as I have to make sure each picture is cleared before I can use it (ethically), but I am making a lot of progress! I have species such as the King Cobra, Inland Taipan, and Eyelash Pit Viper among just a few! Wikimedia Commons has been a huge help!
I'm super excited.
Hope your nights are going good. I am still not feeling good but jamming + virtual snake hunting is keeping me busy!
#programming#data science#data scientist#data analysis#neural networks#image processing#artificial intelligence#machine learning#snakes#snake#reptiles#reptile#herpetology#animals#biology#science#programming project#dataset#kaggle#coding
43 notes
·
View notes
Text
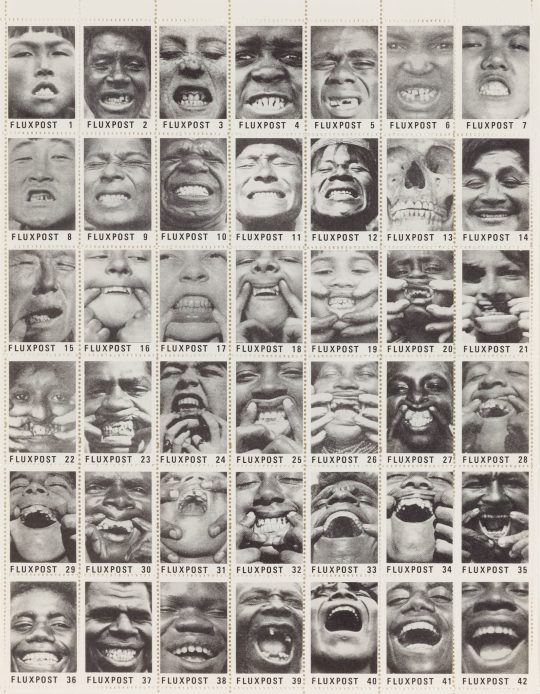
George Maciunas, Fluxpost (Smiles), 1978. © George Maciunas & Jonas Mekas Visual Arts Center
7 notes
·
View notes
Text
I bleed revolution. If your only anarchist actions are related to union organizing, then you’re not an anarchist, you’re a corporate puppet. Everything you do should work to subvert the current and future actions of the state and all of their tentacle corporate affiliations. If your only goal in life is to work under the orders of someone else, under someone’s else’s direction, with someone else’s instructions, then you’re not a human being. You’re chattel cattle at best. If a corporate pig tells or wants you to do something, then you should do the exact opposite, or else you’re just a pawn in a game of global corporate chess. Every one of your actions should be both a defensive and offensive maneuver. If you defend while you attack, you become one with your true purpose, which is to dismantle the state and all corporate authority. If you don’t think in a linear manner, then you’re not apart of their datasets, and they can’t predict your next move. You operate from outside of their datasets and what they think is your next move is never your next move. Then they start to doubt their own intelligence and all the false assumptions it’s based on, and the system starts to crumble. You use any means necessary, because that is your constitutional right, just as they use any means necessary to hold onto the power they stole from you. They stole your birthright, and it’s your legal duty as an American citizen to seek a redress of your grievances, using whatever it takes. Under no pretext.
#Revolution#constitution#anarchy#authority#system#corporate#American#America#birthright#dataset#datasets#AI#artificial intelligence#intelligence#CIA#anomaly#alien#UFO#wavelength#signals#amplitude#frequency
9 notes
·
View notes
Text
Researchers develop unsupervised machine learning method to improve fraud detection in imbalanced datasets

- By Nuadox Crew -
Researchers at Florida Atlantic University have developed a new machine learning method that significantly improves fraud detection by generating accurate class labels from severely imbalanced datasets—common in fraud cases where fraudulent events are rare.
Unlike traditional methods that rely on labeled data, their unsupervised technique works without prior labeling, cutting costs and addressing privacy concerns.
Tested on large real-world datasets (European credit card transactions and Medicare claims), the method outperformed the widely-used Isolation Forest algorithm by minimizing false positives and requiring less human oversight. It combines three unsupervised learning models with a percentile-gradient approach to isolate the most confidently identified fraud cases, enhancing accuracy and efficiency.
Published in the Journal of Big Data, this approach offers scalable, low-cost fraud detection for high-risk industries like finance and healthcare, and was recognized with a Best Student Paper Award at the IEEE ICTAI 2024 conference. Future work will focus on automating optimal label selection to further boost scalability.
Read more at Florida Atlantic University (FAU)
Scientific paper: Mary Anne Walauskis et al, Unsupervised label generation for severely imbalanced fraud data, Journal of Big Data (2025). DOI: 10.1186/s40537-025-01120-x
Related Content
Small Data approaches provide nuance and context to health datasets
Other Recent News
New oral medication shows promise against antibiotic-resistant gonorrhea.
Medical imaging radiation may be responsible for 5% of cancer cases in the U.S.
0 notes
Text
TrackMyHashtag explores how users can access and utilize free Twitter datasets for research, marketing, and analytics. It highlights the significance of Twitter data in trend analysis, sentiment research, and social media strategy. The article provides insights into different types of datasets, including historical and live Twitter data, and explains how TrackMyHashtag offers structured, ready-to-use datasets. Additionally, it guides users on downloading and using these datasets effectively for various applications like academic studies, brand monitoring, and competitive analysis.
#twitter dataset#historical data#historical twitter dataset#twitter data#twitter tool#historical twitter data#hashtag analysis#twitter search history#hashtag analytics#dataset#twitter
0 notes
Link
看看網頁版全文 ⇨ 公視地方新聞資料集 / PTS NEWS Local News Dataset https://blog.pulipuli.info/2025/02/pts-news-local-news-dataset.html 最近我因為研究需求蒐集了公視新聞網地方新聞的一些內容,並把資料整理表格資料集,提供給有需要的人使用。 Recently, for my research, I collected local news content from the PTS News website and organized the data into a tabular dataset, making it available for anyone who needs it.。 ---- # 公視新聞網 / Public Television Service News Network。 https://news.pts.org.tw/category/11。 公視新聞網(PTS News Website)是公共電視文化事業基金會新聞部經營的網路新聞平台。 它提供即時的新聞報導、深度分析和專題節目,涵蓋國內外的重要事件。 公視新聞網的目標是提供一個獨立、中立且值得信賴的新聞來源,相較於其他可能被歸類為特定政治立場的媒體,公視新聞網比較強調它注重客觀與多元觀點呈現的特質。 它不僅是臺灣重要的公共媒體資源,也透過豐富多元的內容和積極的互動,努力滿足使用者對高品質新聞資訊的需求,並期許能成為一個值得信賴的新聞來源。 使用者可以用多種管道收看公視的新聞,而公視新聞網則是提供使用者這個網頁上瀏覽文字、圖片和影片等不同形式的新聞內容,並透過網站提供的導覽功能,快速找到自己感興趣的資訊。 公視新聞網的網站分類包含了「政治」、「全球」、「兩岸」、「地方」、「產經」、「文教科技」、「環境」、「社福人權」等類別。 而我這次蒐集的資料,就是來自於「地方」分類底下的新聞資料。 ---- # 公視地方新聞資料集 / PTS NEWS Local News Dataset。 - Google試算表:https://docs.google.com/spreadsheets/d/1lz6BdPbno6AMwbay8ud95ioplHBYMDGN4kZDE5ZEs-I/edit?usp=sharing - CSV格式下載:https://docs.google.com/spreadsheets/d/1lz6BdPbno6AMwbay8ud95ioplHBYMDGN4kZDE5ZEs-I/export?format=csv ---- 繼續閱讀 ⇨ 公視地方新聞資料集 / PTS NEWS Local News Dataset https://blog.pulipuli.info/2025/02/pts-news-local-news-dataset.html
0 notes
Text

Adam Harvey, Exposing AI, 2021. Online project
0 notes
Text
CDC Datasets
Update on the compressed single-file version of the CDC datasets: the ingest to the Internet Archive is now complete, and my redirect has been updated to point there.
You can pull the file from them directly, or use their torrent.
That link again: https://dave.io/go/cdc
0 notes
Text
The Epistemology of Algorithmic Bias Detection: A Multidisciplinary Exploration at the Intersection of Linguistics, Philosophy, and Artificial Intelligence
We live in an increasingly data-driven world, where algorithms permeate nearly every facet of our existence, from the mundane suggestions of online retailers and products to the critical decisions impacting healthcare and justice systems. Photo by Tara Winstead on Pexels.com These algorithms, while often presented as objective and impartial, are inherently products of human design and the data…

View On WordPress
#Algorithm#algorithm design#algorithmic bias#Artificial Intelligence#bias#confirmation bias#critical discourse analysis#critical reflection#data bias#dataset#Deep Learning#deontology#epistemology#epistēmē#ethical principles#fairness#inequality#interdisciplinary collaboration#justice#Language#linguistics#Machine Learning#natural language processing#objectivity#Philosophy#pragmatics#prohairesis#Raffaello Palandri#sampling bias#Sapir-Whorf hypothesis
1 note
·
View note
Text
Image Classification Datasets: Fueling the Future of AI

Within artificial intelligence (AI), the power to classify images precisely is a valuable tool for machines to be able to really get and decode the visual world; later on, they are used thus. From object detection to medicine, the image classification dataset procedure is a key task, which, in turn, facilitates different AI systems to the maximum. The core image classification datasets are meticulously curated collections of labeled images that are essential in the education of these systems, as they allow AI models to learn how to categorize visual data.
What is Image Classification?
Image classification is the assignment of an image to a certain category by taking into account its content. As an example, a dataset containing photos of different animals could be labeled as "dog," "cat," or "elephant." A trained model, whether it is supervised or unsupervised, can thus recognize and later classify objects in new, unseen images using the data of different patterns, textures, and features. A successful image classification method is dependent on good datasets, where the images are appropriately labeled with correct tags and also represent the broadest possible range of conditions.
Why Are Image Classification Datasets Important?
The method’s success lies deeply in the used datasets for the training purpose of AI model’s. A properly organized image classification dataset enables machine learning systems to differentiate various categories, thus, the system will become smarter and more accurate. Here are some reasons why these datasets are crucial for AI development:
Improved Accuracy
A versatile and full collection of image classification datasets guarantees that the AI model will be able to distinguish among objects, animals, faces, or scenes regardless of the different lighting conditions, environments or viewpoints. The larger and the better the images in the dataset, the higher will be the reliability of the AI's predictions in practical applications.
Reduces Bias
If the dataset has less variety in it, the AI could harbor biases, which, in turn, limit its efficacy. For instance, a facial recognition model taught by a set of pictures containing only one single ethnicity among all images may not be able to capture people from other ethnicities. Facing this limitation, diversity of image classification datasets, including different age groups, genders, places, and settings, is a must to achieve fairness and inclusion.
Enables Efficient Learning
To design a model that is able to capture the general idea of AI, it needs to be trained on different types of data samples from many different categories. Image classification datasets are the ones that make the model understand to classify images by considering important characteristics rather than superficial ones.
Applications of Image Classification Datasets
Datasets designed for image classification are predominantly implemented in many domains and applications. These are some of the main areas where these datasets are critical:
Healthcare
Within medicine, AI-based systems utilizing image classification datasets are expected to become the most advanced approach to diagnosing diseases in the future. An AI model trained on a huge dataset of medical images of different cases can detect abnormalities like cancer, T.B., or heart disease with a very high level of accuracy. These AI-based systems are the tools through which doctors can reach correct medical conclusions faster.
Retail and E-Commerce
Within the retail division, image classification is applied to product categorization. Retailers may easily use AI to automatically sort and categorize products based on the visual representations including stockrooms and customers. By means of a database containing the classification of images, online shops can online platforms Visual Search to enhance their search and recommendation systems by, for example, helping customers to their desired products visually.
Autonomous Vehicles
Autonomous cars use image classification datasets for road signs, pedestrians, traffic lights, and other vehicles, as they recognize objects by comparing them with the datasets. Autonomous vehicles are able to safely drive around thanks to big and wide bodies of unique image data that allows them to understand their surroundings and decide what to do at that moment.
Agriculture
AI is being employed in agriculture as a tool by farmers and researchers to supervise crop health, detect diseases, and evaluate soil conditions. Image classification datasets are very important in the training of AI models that can identify plant species, observe their growth, and even detect early signs of disease or pest infestation, which in turn, allows for more efficient farming practices.
Security and Surveillance
Security systems with AI models supervised on image classification datasets contribute to the identification of deviants and possible security risks in surveillance footage. Image classification systems can make well-timed decisions of these deviations, thus not only catching suspected people in the surveillance videos, but also tracking the unusual tendencies within the crowd.
Social Media
Social media platforms are using image classification datasets for content moderation, auto-tagging, and even facial recognition. These systems measure and classify millions of images by AI which automatically tags them and this draws user interest and gives the platform safety.
Building a Good Image Classification Dataset
Materials in a quality image classification dataset should be carefully thought out with an emphasis on detail. Below are some important aspects of constructing a dataset:
Diversity of Data
One that is deemed to be a successful image classification dataset includes a wide range of categories and conditions. The AI model learns properly to handle the heterogeneous case of the real-world. For example, creating a face image dataset will necessarily contain the annotated faces of different people with varied ages, ethnicities, and facial expressions which will obviate the bias problem and the model will then be able to generalize.
High-Quality, Labeled Images
Every picture will be examined in this dataset and pinned with its relevant tag correctly. The more exact and uniform the tagging of the images, the more efficiently the model will learn. Properly labeling pictures is a lengthy process for sure but it is a critical constituent of high-quality training.
Data Augmentation
In most of the cases, data augmentation techniques like rotating, cropping, or flipping the images, turn out to be the best way of increasing the dataset. This way, the model actually learns to recognize objects or features from the images, or there can be different possible conditions of those.
Data Preprocessing
Preprocessing of data (for example, by means of resizing images, normalizing pixel values, or removing noisy data) guarantees that the AI model can process the images efficiently and consequently the training is faster and more accurate.
Conclusion: The Future of Image Classification with AI
With AI evolving, accuracy and image classification datasets will be demanded increasingly. These connotations are the triggers of tons of AI applications, from healthcare to retail, and security followed by autonomous driving. The variety and quality of the data-set will seriously determine the AI model to identify proper images, thus, image classification is a core part of successful AI projects.
One of the best approaches to develop a genuine, efficient, and inclusive AI system is by creating different kinds of good quality and labeled image classification datasets. If you've exponentially boosted your skills in the fields like healthcare, retail, or agriculture then choosing the correct data sources is the key step toward the successful AI and ML implementation.
0 notes
Text
Upcoming Developments In Federated Learning AI Technologies

What is Federated learning?
Federated learning AI provides a means of unlocking information to feed new AI applications while training AI models without anybody seeing or touching your data.
The recommendation engines, chatbots, and spam filters that have made artificial intelligence a commonplace in contemporary life were developed using data mountains of training samples that were either scraped from the internet or supplied by users in return for free music, email, and other benefits.
A large number of these AI programs were trained using data that was collected and processed in one location. However, modern AI is moving in the direction of a decentralized strategy. Collaboratively, new AI models are being trained on the edge using data that never leaves your laptop, private server, or mobile device.
Federated learning AI model is a new kind of AI training that is quickly becoming the norm for processing and storing private data in order to comply with a number of new requirements. Federated learning also provides a means of accessing the raw data coming from sensors on satellites, bridges, factories, and an increasing number of smart gadgets on our bodies and in our homes by processing data at its source.
IBM is co-organizing a federated learning session at this year’s NeurIPS, the premier machine learning conference in the world, to foster conversation and idea sharing for developing this emerging subject.
How Federated Learning AI Model Works?
Similar to a team report or presentation, federated learning allows many individuals to remotely share their data in order to jointly train a single deep learning model and improve incrementally. The model, often a pre-trained foundation model, is downloaded by each participant from a cloud datacenter.
After training it on their personal information, they condense and encrypt the updated model configuration. After being decrypted and averaged, the model updates are returned to the cloud and incorporated into the centralized model. The collaborative training process keeps going iteration after iteration until the model is completely trained.
There are three variations of this decentralized, dispersed training method. Similar datasets are used to train the central model in horizontal federated learning. The data are complimentary in vertical federated learning; for instance, a person’s musical interests may be predicted by combining their assessments of books and movies.
Lastly, in federated transfer learning, a foundation model that has already been trained to do one task such as recognizing cars is trained on a different dataset to accomplish another such as identifying cats. The integration of foundation models into federated learning is now being worked on by Baracaldo and her colleagues. One possible use case is for banks to build an AI model to identify fraud and then repurpose it for other purposes.
Advantages Of Federated Learning
Federated learning AI model has a number of clear benefits, particularly where decentralized data processing and data privacy are crucial. Here are a few main benefits:
Improved Privacy of Data
By enabling model training on decentralized data sources without direct access to the raw data, federated learning puts privacy first. By ensuring that private or sensitive data stays on local devices, this decentralized method lowers the possibility of data breaches.
Enhanced Protection
Sensitive information is less centrally located as it is processed and stored locally on separate devices. When compared to conventional centralized learning techniques, this structure reduces the likelihood of significant breaches.
Effective Use of Data
Federated learning may improve model performance and accuracy by using data from several devices or institutions rather than centrally gathering data. This makes it feasible for the model to learn from a large dataset, something that conventional approaches would not be able to do.
Lower Data Transfer Expenses
Federated learning decreases data transmission costs and network stress by sharing just model changes rather than raw data. Applications with poor connection or settings where bandwidth costs are an issue would particularly benefit from this.
Quicker Education and Instantaneous Updates
Models may be updated almost instantly as data is created on local devices with to federated learning. Applications where current learning is essential, such as smart devices or tailored suggestions, benefit from this responsiveness.
Observance of Data Regulations
Because the data remains locally, federated learning is well-suited to comply with data privacy rules and regulations like the GDPR. For businesses managing user data in regulated sectors like healthcare or banking, this may reduce compliance concerns.
Increased Customization
Federated learning preserves user privacy while enabling models to be tailored to local data patterns. Applications such as customized advice or individualized health monitoring benefit greatly from this.
Conclusion
All things considered, federated learning facilitates safe, privacy-aware AI developments, enabling efficient data utilization without jeopardizing user confidence or legal compliance.
Read more on Govindhtech.com
#FederatedLearningAI#AI#Federatedlearning#AIModels#machinelearning#deeplearning#dataset#dataprivacy#Technews#Technology#Technologynews#Technologytrends#govindhtech#News
0 notes
Text

Fiber Planning: Key Consideration for Broadband and Deployment
This article into key considerations in fiber planning, future of optical fibers, the importance of network inventory management, and integration of telecom GIS to support broadband deployment.
Click to read more about Fiber Planning and Broadband Deployment.
#fiber#fttx#ftth#broadband deployement#fiber planning#network planning#network optimization#fiber deployment#lepton software#lepton maps#network inventory management#telecom#gis#data#map#maps#dataset
0 notes