#What is Data Validation in Excel
Explore tagged Tumblr posts
Text
What is Data Validation in Excel? How to Improve Data Quality
Data validation is a crucial feature in Excel that helps users control the type of data entered into a cell. By setting specific criteria, you can ensure that only the correct data type is input, which helps in maintaining accuracy and consistency in your spreadsheets. This feature is particularly useful in large datasets where errors can easily go unnoticed, leading to inaccurate results and flawed analyses.
In this blog, we'll explore what is data validation in Excel, how it works, and how you can use it to improve data quality in your spreadsheets.
Understanding Data Validation in Excel
Data validation in Excel allows you to define rules that restrict the type of data that can be entered into a cell. These rules can include restrictions on data type (e.g., whole numbers, decimals, dates), specific values, or even custom formulas. When a user tries to enter data that doesn't meet the criteria, Excel will display an error message, preventing the entry of invalid data.
For example, if you're working with a list of ages, you can use data validation to ensure that only numbers between 1 and 120 are entered. This prevents errors such as negative numbers or extremely high values that would distort the analysis.
Steps to Implement Data Validation in Excel
Implementing data validation in Excel can be done through a few simple steps. Hereās how you can do it:
Identify Your Data Requirements: Before setting up data validation, determine what type of data you want to restrict. This could be a number, date, specific text, or values from a list.
Create a List or Criteria: If your data validation involves specific choices (e.g., a list of departments or categories), prepare the list beforehand. You can do this by typing the options into a separate worksheet or a column in the same sheet.
Select the Target Cells: Click and drag to highlight the cells where you want to apply the data validation rules. This could be a single cell, a column, or a range of cells.
Apply Data Validation Rules: (a) Go to the āDataā tab on the Excel ribbon. (b) Click on āData Validationā in the Data Tools group.
In the dialog box that appears, under the āSettingsā tab, choose the type of validation you want (e.g., whole numbers, dates, or a list).
Specify Your Criteria: Depending on the validation type selected, set up your specific criteria. For instance, if youāre working with a list, select the range where your list is located.
Set Error Alerts: Navigate to the āError Alertā tab within the Data Validation dialog box. Here, you can create a custom message that will pop up if a user enters invalid data.
Test Your Validation Rules: Once applied, try entering data in the validated cells to ensure your rules work as intended. Adjust if necessary by returning to the Data Validation menu.
This process allows you to precisely control the data being entered, helping maintain the integrity and quality of your Excel spreadsheets.
Types of Data Validation in Excel
Excel offers several types of data validation to suit different needs. Here are some common types:
Whole Number: Restricts entries to whole numbers within a specified range.
Decimal: Allows decimal numbers within a specific range.
List: Lets users select from a predefined list of values.
Date: Restricts entries to dates within a specific range.
Time: Limits entries to times within a specific range.
Text Length: Controls the number of characters in a text entry.
Custom: Allows you to use a custom formula to define the validation criteria.
Benefits of Using Data Validation in Excel
Using data validation in Excel is essential for quality data analysis which offers numerous benefits:
Improves Data Accuracy: By restricting data entry to specific criteria, you reduce the risk of errors.
Enhances Consistency: Ensures that data follows a consistent format, making it easier to analyze.
Saves Time: Prevents the need for manual data cleaning by catching errors at the point of entry.
Guides Users: Custom error messages can help guide users to enter the correct data, reducing confusion.
Reduces Redundancy: By validating data, you can avoid duplicate entries, ensuring that your dataset remains clean and organized.
How to Improve Data Quality Using Data Validation
Data validation is a powerful tool, but it's just one part of improving data quality. Here are some additional tips to ensure high-quality data in Excel:
Use Consistent Formats: Ensure that all data entries follow a consistent format, such as dates in the same format (e.g., DD/MM/YYYY).
Regularly Audit Your Data: Periodically check your data for inconsistencies, errors, or missing values.
Leverage Conditional Formatting: Use conditional formatting to highlight cells that don't meet specific criteria, making it easier to spot errors.
Implement Drop-Down Lists: Where possible, use drop-down lists to limit data entry to a predefined set of options.
Use Data Cleaning Tools: Excel offers several tools like "Remove Duplicates" and "Text to Columns" that can help clean up your data.
Conclusion
Data validation in Excel is a powerful feature that can significantly improve the quality of your data. By setting specific criteria for data entry, you can reduce errors, enhance consistency, and ensure that your data is reliable and accurate. Combined with other data management practices, data validation can help you maintain high standards of data quality, making your analyses more trustworthy and actionable.
0 notes
Text
growing sideways š§ jeonghan x reader.
yours, whether you like it or not,
š§ pairing. co-workers!jeonghan x reader. š§ social media au & epistolary (told through emails). š§ genres. alternate universe: non-idol, alternate universe: co-workers. romance, humor. š§ includes. mention of alcohol; suggestive language; profanity. workplace rivals, corporate jargon, engineering terms i definitely butchered, use of y/n l/n for e-mail purposes. title from noah kahanās growing sideways; waaay too many kahan references, really. style and format insp. by cinnamorussellās tell all your friends iām crazy (iāll drive you mad). š§ notes. this is a bit long, but we ball. in one of my first conversations with @diamonddaze01, we dreamed up workplace rival yoon jeonghan. i offer it, now, as part of a month-long celebration for the person iāve dedicated a good quarter of my work to. tara, iāll never meet someone who wonāt know about you. nanu ninnannu pritisuttene! š
Liked by feat.dino, everyone_woo, and others jeonghaniyoo_n Ā if my engine works perfect on empty, guess iāll driveĀ
View all comments
vernonline woah indie ahhh caption user1 Looking good, Jeonghan! Letās catch up soon x user2 who tha baddie in the back in the second slideee ā³ sound_of_coups š ā³ user3 no the one on the right sry :/ ā„ļø Liked by creator user4 congrats to whoeverās bouncing on it ! junhui_moon Aura 1000000% ā³ jeonghaniyoo_n what language are you speaking
Liked by sound_of_coups, dk_is_dokyeom, and others yourusername Ā romanticizing life (before i go insane)Ā
View all comments
user1 need to know where that phone case is from user2 Are you EVER not working dk_is_dokyeom THATāS MY GIRLBOSS ā°(āāā)⯠Ⳡyourusername ā¤ļø user3 i wanna be you when i grow up <3 xuminghao_o Lovely ā„ļø Liked by creator
from: L/N Y/N [email protected] to: Yoon Jeonghan [email protected] subject: Re: Test Platform Validation Report (EU Submission)
Yoon,
I reviewed the validation draft you uploaded this morning. Fascinating interpretation of clause 4.3.2. Bold of you to skip the stability data appendix entirely. I can only assume it was an artistic choice.
Also, the raw tensile data from the 0528 batch isnāt included. If it was meant to be in the shared drive, it wasnāt in any of the usual folders (QA_Share > FR_Validation > tensile_data > missing_files > probably_Jeonghanās).
Iāve attached my edits. I added actual numbers.
Regards, L/N Y/N she/her [email protected]
from: Yoon Jeonghan [email protected] to: L/N Y/N [email protected] subject: Re: Test Platform Validation Report (EU Submission)
Thank you for the prompt review. I assumed your obsession with clause 4.3.2 would outweigh your impulse to nitpick, but alasāsome things never change.
The stability data was excluded intentionally while awaiting results from the accelerated aging test. If you opened the protocol (second folder under QA_Share > FR_Validation > tensile_data > definitely_not_missing), youād see that.
As for your edits, I appreciate the effort. Itās cute when you pretend Excel likes you back.
Best, Yoon Jeonghan he/him [email protected]
from: L/N Y/N [email protected] to: Yoon Jeonghan [email protected] subject: Re: EU Submission - FR Manufacturing Coordination
Yoon,
Not that I expect you to read full briefs, but just in case you skimmed this one: yes, the transfer protocols need to be locked before next Friday if we want the France site to hit qualification by Q3.
Your last edits to the QAP template were inspired. I didnāt know it was possible to confuse ISO 13485 with a haiku.
Iāve restructured the equipment IQ section. Youāre welcome. Youāll need to coordinate with Wonwoo at the Lyon site for vendor access, assuming you remember to email him this time.
Iāll see you in Lyon.
Disrespectfully, L/N Y/N she/her [email protected]
from: Yoon Jeonghan [email protected] to: L/N Y/N [email protected] subject: Re: EU Submission - FR Manufacturing Coordination
Of course I read the brief. Just because I donāt annotate every margin with red ink and superiority complexes doesnāt mean I donāt understand the deadline.
Iāll coordinate with Wonwoo, assuming you donāt scare him off again with your charmingly blunt emails. (I still have the screenshot of him calling you āintimidatingly competent.ā)
By the way, your IQ revisions look fine. Shockingly legible this time. Congratulations.
Iāll see you in Lyon. Try not to sabotage the coffee machine this trip.
Until customs detains us, Yoon Jeonghan he/him [email protected]
from: L/N Y/N [email protected] to: Yoon Jeonghan [email protected] subject: Re: EU Submission - FR Manufacturing Coordination
If Wonwoo was intimidated, itās because I sent him instructions written in complete sentences. A rare treat, I know.
You still havenāt confirmed the calibration matrix. Weāll need the traceable certs before equipment ships, or do you plan to charm EU regulators into letting us slide on documentation? Actually, donāt answer that. Iāve seen you talk to vendors.
Also: bring the correct adapter this time. Iām not sharing an outlet with you again.
Best of luck (to me), L/N Y/N she/her [email protected]
from: Yoon Jeonghan [email protected] to: L/N Y/N [email protected] subject: Re: EU Submission - FR Manufacturing Coordination
The calibration matrix is in the tracker: third tab, fourth column, next to the thing labeled āREAD ME, PLEASEā Try it. Itās fun.
And yes, I plan to charm the regulators. You, on the other hand, can stun them into compliance with your piercing PowerPoint transitions.
As for the outlet. Iām bringing an adapter. And a surge protector. For reasons.
Looking forward to our time in France. Nothing says āteamworkā like four days of jetlag and passive aggression.
Yours in regulatory purgatory, Yoon Jeonghan he/him [email protected]
YJH šæ (Work) [8:13 AM]: why do you type so aggressively. the guy next to me thinks youāre yelling at me You [8:14 AM]: heās not wrong. YJH šæ (Work) [8:15 AM]: did you really need three highlighters in your carry-on? You [8:15 AM]: yes. the pink one is for your mistakes. YJH šæ (Work) [8:16 AM]: romantic You [8:16 AM]: if you die on this trip itās going to be from a highlighter to the throat. YJH šæ (Work) [8:17 AM]: worth it You [8:17 AM]: you are the worst seatmate in existence. YJH šæ (Work) [8:18 AM]: you snore when you pretend not to be sleeping and your pointy elbow crosses the line You [8:18 AM]: so weāre calling it a truce? YJH šæ (Work) [8:19 AM]: weāre calling it foreplay
ā¾ You have silenced Notifications.
Liked by junhui_moon, woozi_universefactory, and others jeonghaniyoo_n Ā everything, everywhereĀ
View all comments
user1 oui oui š user2 Who are you wearing??? ho5hi_kwon surprised a murder hasnāt occurred lolololol ą° āāæā ą° ā³ jeonghaniyoo_n not counting it out just yet user3 WHATāS 4+4? ATEEE user4 Is he a model? ā³ sound_of_coups please donāt say that his head is going to get so big
Liked by vernonline, xuminghao_o, and others jeonghaniyoo_n Ā northern attitudesĀ
View all comments user1 bwoah . . . feat.dino STUNT ON THEM HOESSSS ā„ļø Liked by creator user2 gender gender gender š®āšØ user3 Really need to know where the second pic is !! Plsss DM yourusername i see how it is ā³ jeonghaniyoo_n credits. xo
from: L/N Y/N [email protected] to: Yoon Jeonghan [email protected] subject: FR Submission Debrief + Documentation
Yoon,
Per our debrief notes (the ones not written on a cocktail napkin), Iāve uploaded the final QAP revisions and vendor qualification summaries to the shared drive. You can stop emailing me pictures of our hotel room as Ā ādocumentation.ā Though impressive dedication to fieldwork.
Also, your expense report still lists the mini bar from Tuesday night. Pretty bold move, considering you insisted you only drank half the bottle.
Respectueusement, L/N Y/N she/her [email protected]
from: Yoon Jeonghan [email protected] to: L/N Y/N [email protected] subject: Re: FR Submission Debrief + Documentation
Youāre welcome for the in-room stress testing of French plumbing. I was being thorough.
Also, I did only drink half. You drank the other half and then told the front desk I was your emotional support engineer.
Re: shared drive. I see your formatting crimes continue. I fixed your spacing in the risk assessment table. Try to be better.
Yours across all timezones, Yoon Jeonghan he/him [email protected]
from: L/N Y/N [email protected] to: Yoon Jeonghan [email protected] subject: Re: FR Submission Debrief + Documentation
Yoon,
Iād fix my spacing if youād stop adjusting my bullet styles just to mess with me. And next time, maybe donāt volunteer us for the plant tour while hungover. Watching you nearly fall into a vat of solvent was not the regulatory impression we wanted.
Stop calling me yours, L/N Y/N she/her [email protected]
P.S. You still owe me one (1) bed. Iām adding it to your performance review.
from: Yoon Jeonghan [email protected] to: L/N Y/N [email protected] subject: Re: FR Submission Debrief + Documentation
Not my fault someone booked the hotel late and got us the romantic suite. Youāre lucky I didnāt call room service for rose petals.
Iāve uploaded the final sign-offs and confirmation from the French regulatory contactāwho says weāre the most āthorough and theatrically matchedā engineers sheās worked with. I think thatās a compliment.
Let me know if Iāve missed any appendices. Or if you want your highlighter back.
Yours, even if you deny me (hotel registration said so), Yoon Jeonghan he/him [email protected]
P.S. I liked sharing the room with you. Not because of budget errors or international confusion. Just because it was you.
Liked by ho5hi_kwon, min6yu_k, and others yourusername Ā good week š·
View all comments
user1 GIVE US A FIT CHECK user2 something youāre not telling me ? hmmm ā³ yourusername dm dm dm user3 Need to know who yr nail tech girlie is fr everyone_woo š ā³ yourusername 𤫠sunwoo pretty flowers 4 a pretty girl ā„ļø Liked by creator
from: Yoon Jeonghan [email protected] to: L/N Y/N [email protected] subject: Supplier Audit Timeline + Other Things
Great audit notes, as usual. Iāve attached my edits for the CAPA log. Weāll have to discuss column F, because your formulas hate me.
Also, bold of you to post a photo of flowers on a Tuesday. Does SVT approve PTO for midweek romance now?
Am I being cheated on?, Yoon Jeonghan he/him [email protected]
from: L/N Y/N [email protected] to: Yoon Jeonghan [email protected] subject: Re: Supplier Audit Timeline + Other Things
Yoon,
Corrected the formula logic in column F. Try not to break it again.
And yes, Tuesday dates are a thing now. Believe it or not, some people find me tolerable enough to see more than once.
Shocking, I know.
Regrets, L/N Y/N she/her [email protected]
from: Yoon Jeonghan [email protected] to: L/N Y/N [email protected] subject: Re: Supplier Audit Timeline + Other Things
Donāt worry. Iām sure your second date will be charmed by your bullet point consistency.
Personally, Iāve never seen the appeal of dating someone like you. Too sharp. Too bossy. Too quick to judge formula errors.
Fortunately, SVT doesnāt require us to like each other outside of Gantt charts.
Yours, whether you like it or not, Yoon Jeonghan he/him [email protected]
from: L/N Y/N [email protected] to: Yoon Jeonghan [email protected] subject: Re: Supplier Audit Timeline + Other Things
Yoon,
Believe me, the feeling is mutual. I'd sooner date a malfunctioning tensile tester.
I fixed your math in the timeline estimates. Again. Please donāt bother me for the rest of the week. Iām going to be busy preparing for date number two.
(You wish I was) Yours, L/N Y/N she/her [email protected]Ā
You [11:42 PM]: he ghosted me. u jinxed it. You [11:43 PM]: i shaved my legs for nothing. hope ur happy. You [11:44 PM]: he said he liked my slides. he LIED!!!!!!!!!!!!!! You [11:45 PM]: sitting alone at a bar rn contemplating the meaning of life.. and if i can blow u up telepahteitcally.... YJH šæ (Work) [11:45 PM]: *telepathically YJH šæ (Work) [11:46 PM]: which bar. You [11:47 PM]: fucking MANSPLAINER You [11:47 PM]: donāt come near me EVEREVER
YJH šæ (Work) requested your location.
You started sharing your location with YJH šæ (Work).
You [11:50 PM]: fuckfcuckfuckity my fat fucking thumbs FMLLL YJH šæ (Work) [11:53 PM]: iām coming. donāt order tequila until i get there. or do. i want to see the disaster myself. You [11:55 PM]: jerk YJH šæ (Work) [11:56 PM]: always. save me a seat, heartbreak girl
Liked by dk_is_dokyeom, junhui_moon, and others jeonghaniyoo_n Ā keep the bad shit in my liver and the rest around my heart
View all comments
user1 Caption + second slide >>>> joshu_acoustic is that yourusername in the last slide 𫨠Ⳡjeonghaniyoo_n is it ? yourusername ā³ yourusername must be a lookalike ā„ļø Liked by creator ā³ dk_is_dokyeom THATāS ME yourusername & min6yu_k !!! įµ įµ įµ user2 just one chance pls,, user3 Wait was that a wine date or
from: L/N Y/N [email protected] to: Yoon Jeonghan [email protected] subject: Re: Equipment Revalidation ScheduleĀ
Yoon,
Your revised equipment validation timeline looks solid. Iāve flagged the dates where QRA and process requal overlap. Youāll need to talk to Ops to make sure thereās no resource conflict.
Also, thanks. For the other night.
Donāt make a thing out of it. Reluctantly yours, L/N Y/N she/her [email protected]Ā
from: Yoon Jeonghan [email protected] to: L/N Y/N [email protected] subject: Re: Equipment Revalidation ScheduleĀ
Wow. A āthanks.ā What is this, a truce?
Noted on the QRA overlapāIāll sync with Ops and shift our timeline by 2-3 business days. Iāve attached a revised Gantt for your very critical review.
Also: you owe me fries.
Yours with no reluctance whatsoever, Yoon Jeonghan he/him [email protected]
P.S. Donāt let your guard down. Iād hate for you to start thinking Iām nice.
P.P.S. Youāre beautiful when drunk. Infuriating, but beautiful.
from: L/N Y/N [email protected] to: Yoon Jeonghan [email protected] subject: Re: Equipment Revalidation ScheduleĀ
Attached: my comments on your Gantt chart (see rows 14ā27). Also, your font choices are unhinged. Youāre lucky youāre marginally good at your job.
Fries are contingent on you not mentioning the karaoke. Sober now, L/N Y/N she/her [email protected]
P.S. Youāre nice when you think Iām too drunk to remember.
from: Yoon Jeonghan [email protected] to: L/N Y/N [email protected] subject: Re: Equipment Revalidation ScheduleĀ
Iāll swap the font if it means less red pen in my inbox.
And donāt worry, Iād never mention your rendition of āDancing Queenā in front of senior management. Or that you made me sing backup.
As for being nice: I was just making sure you didnāt fall asleep in a nacho basket. Again.
Drunk on you, Yoon Jeonghan he/him [email protected]
P.S. I remember everything you said. Even the parts you donāt.
Liked by everyone_woo, sound_of_coups, and others yourusername Ā new perspective
View all comments
user1 fly safe, babygirl user2 ermmm.. am i witnessing a soft launch ?! min9yu_k Iād know that YSL bag from anywhere š user3 How can I be youuu :( user4 is that a BOYFRIEND?! junhui_moon strategic non-response to any of the comments here #respect
from: Yoon Jeonghan [email protected] to: L/N Y/N [email protected] Subject: Re: France Stability Testing TimelineĀ
Attached: updated protocol outline and projected data submission window. Added notes re: temperature excursions flagged by the lab.
Unrelated, but I saw your latest post. Interesting how you managed to frame the lighting just right on that cafe table. Almost as if someone you work with took the photo.
Also, bold choice uploading a cropped version of that one picture of me holding five tote bags. Very āsoft launch,ā very subtle.
Launched like a rocket ship, Yoon Jeonghan he/him [email protected]
from: L/N Y/N [email protected] to: Yoon Jeonghan [email protected] subject: Re: France Stability Testing TimelineĀ
This isnāt the time.
The humidity chamber failed mid-run and half of the accelerated aging samples are compromised. Iāll need to retest from baseline and revalidate the controls. Not sure yet if it pushes our submission, but Iām flagging it with QA.
I suggest you review section 6.2 of the protocol instead of obsessing over my Instagram.
L/N Y/N she/her [email protected]
from: Yoon Jeonghan [email protected] to: L/N Y/N [email protected] Subject: Re: France Stability Testing TimelineĀ
Didnāt mean to distract. I hadnāt seen the alert yet. Engineering just looped me in on the chamber issue. Iāll prioritize sourcing backup samples and contact Tech Ops to check chamber calibration across all zones.
Youāll have data. Weāll make it work.
(But if you were soft-launching me, I looked great.)
Trying too hard, Yoon Jeonghan he/him [email protected]
from: L/N Y/N [email protected] to: Yoon Jeonghan [email protected] subject: Re: France Stability Testing TimelineĀ
Yoon,
Appreciated. Sorry I snapped.
I just really didnāt want this run to go sideways. I know itās not your faultābut Iāve been fielding calls since 7:00 a.m. and Iām a little fried.
Yours and then some, L/N Y/N she/her [email protected]
P.S. You looked ridiculous, but sure. Let the internet wonder.
from: Yoon Jeonghan [email protected] to: L/N Y/N [email protected] Subject: Re: France Stability Testing TimelineĀ
You can yell at me any time. Preferably not before coffee, but Iāll survive.
QA says theyāll expedite sample disposal so we can start the new batch by end of week. I sent you a revised Gantt. And a snack. Donāt fight me on it.
Yours in whatever way youāll have me, Yoon Jeonghan he/him [email protected]
P.S. Internet speculation is already intense. Iāve received two DMs inquiring if Iām truly off the market. Is this your twisted little way of staking claim?
from: L/N Y/N [email protected] to: Yoon Jeonghan [email protected] subject: Re: France Stability Testing TimelineĀ
The snack was suspiciously well-timed. Youāre lucky I like sesame.
Re: QAāIāll update the submission calendar and notify Regulatory weāre adjusting the stability window.
And tell your fans Iām flattered, but my standards are higher than āguy who argues about font weight in shared spreadsheets.ā
Yours for some reason (When did I succumb to this?), L/N Y/N she/her [email protected]
from: Yoon Jeonghan [email protected] to: L/N Y/N [email protected] Subject: Re: France Stability Testing Timeline
For the record, I wasnāt arguing. I was advocating for consistent formatting.
Also: Iām sorry. For earlier. I shouldāve checked the system alerts before joking around. You always catch things first, and I forget what itās like to be under that kind of pressure all the time.
Let me know what else you need. I mean it.
Yours for equally no reason (I bookmarked the first time you signed off with āyoursā, btw), Yoon Jeonghan he/him [email protected]
Liked by woozi_universefactory, vernonline, and others yourusername Ā needed coffeeĀ
View all comments
sound_of_coups š£ Hook, line, sinker user1 can this guy fight omfg user2 Even his side view is ethereal. What the hale vernonline okurrr ā„ļø Liked by jeonghaniyoo_n Ā ā³ yourusername ? jeonghaniyoo_n wasnāt aware i had paparazzi Ā ā³ pledis_boos IS THIS ALLOWEDDD IS THIS ALLOWED
from: L/N Y/N [email protected] to: Yoon Jeonghan [email protected] subject: Apologies for the TimestampĀ
Yoon,
I realize this is past hours. I wonāt pretend itās an emergencyāitās just the draft for the stability test realignment we discussed. I needed to get it out of my head or I wouldnāt sleep. It can wait until morning. I just didnāt want to forget.
Sorry. Again. Sleep well, or party well, or whatever it is youāre doing tonight.
Terribly sorry, L/N Y/N she/her [email protected]
from: Yoon Jeonghan [email protected] to: L/N Y/N [email protected] Subject: Re: Apologies for the TimestampĀ
Got your emailāyes, timestamp noted.
Iām out. Drinking. Loud music, terrible lighting, questionable tequila. Iāll look at the draft during actual work hours. I promise.
Also, you do know that youāre allowed to exist outside work. Donāt apologize for thinking too hard. Thatās half your brand.
Buzzing like a drunk bumblebee, Yoon Jeonghan he/him [email protected]
from: L/N Y/N [email protected] to: Yoon Jeonghan [email protected] subject: Re: Apologies for the Timestamp
Yoon,
Enjoy your night out. Try not to bully the DJ. May your drinks be overpriced and your lighting flattering.
And heyāhope you pull. You deserve someone mildly tolerable for a few hours.
Cheers, L/N Y/N she/her [email protected]
from: Yoon Jeonghan [email protected] to: L/N Y/N [email protected] Subject: Re: Apologies for the TimestampĀ
The drinks are terrible. The lighting is flattering. Iāve technically pulled, but sheās more interested in the bartender now, which is fine becauseā
I miss you. You, and your midnight overthinking, and your Excel color codes, and the way you always say ādonāt wait upā but still check your inbox five minutes later.
I miss you. Stupidly. Even while Iām here.
Yours at my own risk, Yoon Jeonghan he/him [email protected]
from: L/N Y/N [email protected] to: Yoon Jeonghan [email protected] subject: Re: Apologies for the Timestamp
Yoon,
Pray tell why you're getting drunk and you're "pulling" what I can assume to be ABGs whose names you won't even know in the morning, and yet you're still in the club, emailing me? Missing my drunken emails?
Why? Are the girls of Wall Street not enough for you?
Totally not jealous, L/N Y/N she/her [email protected]
from: Yoon Jeonghan [email protected] to: L/N Y/N [email protected] Subject: Re: Apologies for the Timestamp
I can answer this so simply, it wonāt even be fun.
The girls of Wall Street will never be you.
No one will ever be you.
I'm not enjoying my night as much as I should because you're not here. I'm in the club, drunk AND emailing you. That should tell you everything.
Come out with me next time. Wreck my plans. Ruin the music. Steal my coat.
I may be playing with fire, but to hell with it.
Burning myself, Yoon Jeonghan he/him [email protected]Ā
from: Yoon Jeonghan [email protected] to: L/N Y/N [email protected] Subject: Re: Apologies for the Timestamp
I can feel you overthinking all the way from here. Youāre probably thinking that Iāll wake up tomorrow morning and regret all of this. That I will be unable to face you at work come Monday, when I am no longer drunk out of my mind and thinking you are the most brilliant, most gorgeous, most infuriating person alive.Ā
You will be right. Thankfully, though, these areāwhat do the kids call it? āReceiptsā. You will have a paper trail. These emails will be between you, me, and that Australian guy from IT.Ā
He will know, and you will know, that I may have the most miniscule work crush on you.Ā
Jesus Christ. What am I? A high schooler?Ā
Letās try that again: Love is just a chemical reaction that compels animals to breed. What Iām feeling for you isnāt love. Itās so much more than that.
Love sucks, and I need to sober up, Yoon Jeonghan he/him [email protected]
from: L/N Y/N [email protected] to: Yoon Jeonghan [email protected] subject: Re: Apologies for the Timestamp
Get home safe, Jeonghan.
Yours, with questions, L/N Y/N she/her [email protected]
from: Yoon Jeonghan [email protected] to: L/N Y/N [email protected] Subject: Re: Apologies for the Timestamp
You just called me Jeonghan.
Yours, with answers (maybe), Yoon Jeonghan he/him [email protected]
from: L/N Y/N [email protected] to: Yoon Jeonghan [email protected] subject: Re: Apologies for the Timestamp
Thatās your name, isnāt it?
Stop e-mailing me while youāre at the club.
Fine. Yours, L/N Y/N she/her [email protected]
P.S.: I may have the most miniscule work crush on you, too.
from: Yoon Jeonghan [email protected] to: L/N Y/N [email protected] Subject: Re: Apologies for the Timestamp
i amĀ goi nĀ Ā to die
Yoon Jeonghan he/him [email protected]
Liked by yourusername, ho5hi_kwon, and others jeonghaniyoo_n Ā you got all my love
View all comments
vernonline lfggg min9yu_k 𤮠JK! Congrats junhui_moon saw this coming from a mile away sound_of_coups Gorgeousss Ā ā³ jeonghaniyoo_n back off, bud. dk_is_dokyeom (˶ Ė Ā³Ė)Ėįµ Ė˶) love is love everyone_woo oh god what about our project Ā ā³ yourusername please check your e-mail. :) Ā ā³ everyone_woo fml.
Liked by jeonghaniyoo_n, dk_is_dokyeom, and others yourusername Ā dreaming each night of this version of you :)
View all comments
xuminghao_o Not seeing yjh in suits is disconcerting ho5hi_kwon RAH RAH RAH RAHHH woozi_universefactory š Ā ā³ jeonghaniyoo_n JIHOON????????????? pledis_boos U CAN DO BETTER THAN HIM GIRL joshua_acoustic So happy for you two! feat.dino my otp fr jeonghaniyoo_n mine ā„ļø Liked by creator Ā ā³ yourusername yours,
#jeonghan x reader#jeonghan imagines#svt x reader#jeonghan smau#svthub#svt imagines#seventeen x reader#seventeen imagines#jeonghan drabble#svt drabble#seventeen drabble#(š) page: svt#(š„”) notebook
567 notes
Ā·
View notes
Text
Dropout should hire more trans women.
That said, a couple things about the data set floating around showing disproportionality in casting:
1. 7 of the top 9 (those cast members who appear in over 100 episodes, everyone else has under 70 appearances) are members of the core dimension 20 cast, aka āthe intrepid heroesā. This cast has been in 7 of the 22 seasons, with those seasons usually being 20-ish episodes long (the other seasons are between 4-10 episodes long typically). Thatās approximately 140 episodes for each of the main intrepid heroes cast members just for these seasons (not including bonus content like live shows). Brian Murphy has appeared 154 times, which means almost all of his appearances were on D20 intrepid heroes campaigns.
2. The other 2 in the top 9 are Sam Reich and Mike Trapp, who are both hosts of long running shows (Game Changer and Um, Actually)
3. 198 of the 317 episodes that noncis āTMEā people have appeared in can be attributed to ally Beardsley alone (there is some crossover where for example alex and ally have both appeared in the same episodes). Erika ishii has been in 67 of the 317 noncis āTMEā episode appearances i donāt know how much crossover there is between them but i donāt think theyāve been on d20 together so i doubt itās more than 20. It could be as many as 250 of the 317 episodes that have either erica or ally. Both Erika and ally are majorly skewing the results for the data
4. Over 3/4 of people have no listed gender identity in the spreadsheet - most of them have 1-2 appearances, but a few have 3-4 appearances. Iām pretty sure these people arenāt included in the data at all (some of them iām p sure are not cis like jiavani and bob the drag queen)
5. The data collector has assigned ātmeā and ātmaā to various cast members.
TME: transmisogyny exempt
TMA: transmisogyny affected
Now, tranmisogyny can affect trans women, trans femmes, and nonbinary people, and occasionally masculine appearing cis women.
I personally do not believe that an outside person can assign you a label deciding whether or not you experience certain types of oppression- and yet that is what the data collector has done.
I think a more accurate label would be amab/afab, or more honestly- āpeople i think are amab or have said they are amab and then everyone elseā
6. The data does not include many of their newer shows such as Very Important People, Gastronauts, Play it By Ear, and Monetās Slumber Party, all of which feature trans people (MSP, Gastronauts, and VIP are all hosted by noncis people)
What I think the data more accurately shows:
- Dimension 20 has a āmain castā who have appeared in the majority of episodes
- Dropout has some āregularsā who appear on the majority of their content/shows (sam has referenced multiple times that brennan is one of the first people he calls whenever someone canāt show up for something since heās nearly always down for anything) - none of these people are trans women
Final thoughts:
I think eliminating āhostsā and the āintrepid heroesā from THIS TYPE of data set would be more appropriate because they massively skew the data when crunching the numbers for dropout shows. Especially since I can tell from the excel sheet that there are shows missing. Examining d20 sidequests and the guests on the other shows will give a more accurate representation of casting. Hosts should be analyzed separately as thatās a different casting process.
Also imagine if we referred to men and women as āmisogyny exemptā and āmisogyny affectedā when doing demographics. Or if someone did a data collection of the number of POC appearances in dropout episodes and sorted it by āracism affectedā and āracism exemptā - so weiiiiird
TLDR: the data set has massive issues with its methodology and that should be considered. That doesnāt make what trans women are saying less valid.
In other words: spiders brennan is an outlier and should not have been counted
415 notes
Ā·
View notes
Text
Update #2: A New Outlining Method, Dropdown Plotter
I've been having a hard time conceptualizing how to plan out a novel lately. It used to come so easy to me. Now that I'm a real adult, it feels like there isn't enough space in my brain anymore for me to have my story all in my head without writing my ideas down somewhere.
So, good outlining methods, which can be hard to come by, are crucial for my writing process.
But, a lot of traditional methods don't work for me.
In my opinion, it's extremely important to have an outlining method that doesn't overwhelm you, and which feels creatively freeing. And when do I need to be more creatively free, than when writing an IT Crowd fanfiction?
So I've come up with a new outlining method that I'd love to share with you all! I call it Dropdown Plotter.
Dropdown Plotter uses the dropdown menu feature, which can be found in both Google Sheets and Microsoft Excel, to help you better visualize (and, most importantly, easily reorganize) the major aspects of each chapter of your story.
Basically, it's a spreadsheet that includes, at minimum, 8 columns.
Chapter Title
Plotlines
Included Character(s)
Perspective Character(s)
Location(s)
Chapter Story Description
Writing Stage
Due Date
Why keep track of these things? Balance. Basically, making sure your characters, plotlines, POVs, and important locations all get adequate page time.
It's a lot easier to conceptualize these things when you have it on a color-coded spreadsheet, zoomed out really far, and can see in a big picture way. For example, you might see that the red color indicating "Legolas" is in a scene, drops off after Chapter 17. You might see that the green-coded plot about his missing shoe is only important for 5 chapters in the middle of the story, or that we're spending almost the entire story in the bathroom and never in the evil lair.
So, how do you use the Dropdown Plotter?
First, you go into the dropdown menu, and you can see all the plots you've selected. There's a handy "search feature" for those writing the next Game of Thrones. You want to click the little pencil icon in the bottom right, which is the "edit" button.
On the right-hand side of your screen, a column will pop up called "Data Validation Rules." From here, you can edit the names and colors of each item in the dropdown menu, and add or delete things as you'd like.
Make sure to click "allow multiple selections" on the bottom of the data validation rules pop-up. This will allow you to select multiple characters, multiple locations, and multiple plots when you're in the dropdown menus.
Don't forget to click "Done" at the bottom!
On top of the dropdown menus, the nice thing about working in a spreadsheet is that you can always drag and drop the rows and columns. This makes it extremely easy to change the order of scenes around, in a way that feels very impermanent and easy.
Here is what my Dropdown Plotter looked like for the first three chapters of The Grant: An IT Crowd Fanfiction.
Looks pretty, right? And pretty outlines build confidence! Not only that, but what I like most about this outlining method is that it encourages me to be less precious with my ideas.
It's way less intimidating to overhaul major aspects of your story, such as the dominant POV, the main plot, etc., when all you have to do is click a little button in the dropdown menu to change everything. When I'm editing a little blurb in a spreadsheet versus an entire step outline, it reminds me that no writing problem is insurmountable, and nothing is ever really set in stone.
Again, it builds confidence.
Unfortunately, I've only built a Dropdown Plotter in Google Sheets, but I've provided a blank version to share with you all. The nice thing about spreadsheets is that you can add and delete things as you see fit. For example, some people might want to add...
More columns indicating multiple scenes within the same chapter!
A "Story Beat" column, to mark the specific plot beats each chapter follows (as in the Hero's Journey or the Blake Snyder Beat Sheet).
An "Important Info" column, to mark any worldbuilding or character details discussed or introduced in a certain chapter.
Literally anything your little heart desires!
Happy outlining and I hope you enjoy the Dropdown Plotter!
#the grant an it crowd fanfiction#it crowd unexpected reboot#writing advice#creative writing#writeblr#writers on writing#writers on tumblr#writerscommunity#writing community#writers#outline#book writing#plotting#outlining#novel writing#spreadsheets#dropdown plotter#outlining method#writing tool#posts#it crowd
27 notes
Ā·
View notes
Text
š« Join the Fediverse! š«
Greetings, fellow bloggers! We welcome you to join us in discovering, honoring, and promoting the potential future of social networkingācommonly referred to as the "Fediverse."
The Fediverse, or Federation Universe, refers to a collective of online platforms that utilize the web protocol known as ActivityPub, which has set a standard of excellence in regards to both protecting and respecting users' online privacies.
There's a good chance in the past few years that you've caught wind of the fedi family's critically acclaimed Mastodon; however, there are many other unique platforms worth your consideration...
⨠Where To Begin?
Conveniently enough, from the minds of brilliant independent developers, there already likely exists a Fediverse equivalent to your favorite socials. Whether it's an opinion from the critics, or from the community alikeāthe following popular websites are commonly associated with one another:
Friendica š° = Facebook Mastodon š = Twitter Pixelfed š¼ = Instagram PeerTube š = YouTube Lemmy š = Reddit
It's worth mentioning, too, a few other sites and forks thereof that are worthy counterparts, which be: Pleroma š¦ & Misskey š±, microblogs also similar to Twitter/Mastodon. Funkwhale š is a self-hosting audio streamer, which pays homage to the once-popular GrooveShark. For power users, Hubzilla šØ makes a great choice (alongside Friendica) when choosing macroblogging alternatives.
⨠To Be Clear...
To address the technicalities: aside from the "definitive" Fediverse clients, we will also be incorporating any platforms that utilize ActivityPub-adjacent protocols as well. These include, but are not limited to: diaspora*; AT Protocol (Bluesky š¦); Nostr; OStatus; Matrix; Zot; etc. We will NOT be incorporating any decentralized sites that are either questionably or proven to be unethical. (AKA: Gab has been exiled.)
⨠Why Your Privacy Matters
You may ask yourself, as we once did, "Why does protecting my online privacy truly matter?" While it may seem innocent enough on the surface, would it change your mind that it's been officially shared by former corporate media employees that data is more valuable than money to these companies? Outside of the ethical concerns surrounding these concepts, there are many other reasons why protecting your data is critical, be it: security breaches which jeopardize your financial info and risk identity theft; continuing to feed algorithms which use psychological manipulation in attempts to sell you products; the risk of spyware hacking your webcams and microphones when you least expect it; amongst countless other possibilities that can and do happen to individuals on a constant basis. We wish it could all just be written off as a conspiracy... but, with a little research, you'll swiftly realize the validity of these claims are not to be ignored any longer. The solution? Taking the decentralized route.
⨠Our Mission For This Blog
Our mission for establishing this blog includes 3 core elements:
To serve as a hub which anybody can access in order to assist themselves in either: becoming a part of the Fediverse, gaining the resources/knowledge to convince others to do the very same, and providing updates on anything Fedi-related.
We are determined to do anything within our power to prevent what the future of the Internet could become if active social users continue tossing away their data, all while technologies are advancing at faster rates with each passing year. Basically we'd prefer not to live in a cyber-Dystopia at all costs.
Tumblr (Automattic) has expressed interest in switching their servers over to ActivityPub after Musk's acquisition of then-Twitter, and are officially in the transitional process of making this happen for all of us. We're hoping our collective efforts may at some point be recognized by @staff, which in turn will encourage their efforts and stand by their decision.
With that being stated, we hope you decide to follow us here, and decide to make the shiftāas it is merely the beginning. We encourage you to send us any questions you may have, any personal suggestions, or corrections on any misinformation you may come across.
From the Tender Hearts of, āØš @disease & @faggotfungus šāØ
#JOIN THE FEDIVERSE#fediverse#decentralization#internet privacy#social media#social networks#FOSS#activitypub#mastodon#fedi#big data#degoogle#future technology#cybersecurity#technology#essential reading
37 notes
Ā·
View notes
Text
Pen Pals
Oh fuck that was beautiful
shit what was the last episode i called "the best tng episode" yet, cause it might have been dethroned
okay i also gotta say that i have madly misrepresented this episode in the past. Like, in my memory from 15 years ago this episode was "Data makes a pre-warp friend, her home planet is threatened by geology, Picard so we gotta let them die because of the prime directive, but I guess we can make an exception for Data's friend if he insists, and then everyone except Data's friend dies." And that is not at all what happens!
At least up until the "we gotta let them all die" point, because they have a discussion about the prime directive and fate and it's excellent. Geordi, Troi, Data and Pulaski are the pro-saving the planet's people side and Picard, Worf and (weakly) Riker are the anti-saving the planet's people side. And it's a really good discussion. That Picard ends by basically saying "well if we save people from volcanoes, then we'd also have to save them from slavery, and where'd that bring us?" And then Data fucking plays the recording of this ten-year old girl asking for help and everyone gets emotionally affected enough to say fuck the prime directive, let's save these people if we can. And then they do! It's fucking great!
And I love that this gently bends Picard. Like, the Picard of Symbiosis would not have gone for this and the episode doesn't outright changes him, it just nudges him ever so slightly in the right direction to make him a little better. Although yes, it is fucked up that he needed to hear that little girl's pleas for help first. But he got there anyway!
At one point Riker and Troi are walking down the corridor and Troi giggles unprompted and like honestly, yeah same girl.
I forgot Picard's a horsegirl! As if his character wasn't redeemed enough in this episode already!
I also love that Data is entirely driven by emotions here, like literally everything he does in this episode, it's all emotion. Not a "oh in this scene he is clearly happy or heartbroken" or whatever, every single fucking bit from him answering "Is anybody out there" with a "yes" is entirely emotions. Love that for him. I do find it a bit weird that At the end Picard goes all "well you are a bit closer to undersatnding huamnity by experiencing remembrance and regret." Like you wouldn't say that to a child that just had their first friendship break-up or something, right? Like, yes, it's cool that Data got to experience these things for the first time, but it's odd to frame them as steps on the path to humanity, when we would never frame them as such for any other being that experiences them. Data is a human being admit it already you cowards!
Also love that Pulaski is the one to openly acknowledge and validate it! Hell yeah character arcs!
Okay I was so cought up in everything else, but Wesley is great in this too! He has his first "command", leading a team of scientists to analyse the weird shit happening in this sector! And he's a bit insecure about commanding people who are older and more experienced than him, and then Riker gives him a pep talk, and then he gets better at it. Also loved that Wesley immediately questioned the hierarchies in place, good lad, Wes!
When Wesley approaches Riker for help in Ten Forward, Riker tells his companion that it's a "family emergency" and like. I never thought about that. But Riker is like a great ersatz father for Wesley, and a much better choice than Picard (who I think gets stereotyped into the role due to his closeness to Jack and shipping with Beverly. There's even fan theories out there that actually Picard is Wesley's biological father. No idea why, but they're there). Riker's the one who pushes for Wesley to become an acting ensign, he supports him every step of the way, he saves his life in Justice, And they both have some stuff going on with their own fathers (Riker's sucks and Wesley's is dead). Picard shares a connection with Wes' mother and that one conversation about not getting into the Academy first try. Which is nice, but now that I'm looking at it like this I really wanna see more of Riker mentoring Wes.
This is kind of funny to watch post-Discovery, with Saru's backstory basically being similar to Sarjenka's in that they're both from a pre-warp planet and figure out how to communicate with a Starfleet Lieutenant Commander.
Also, this is the longest Trek episode yet, at eight weeks, it's on par with TOS's The Paradise Syndrome.
I think this is also the first notable example of the TNG crew going rogue, with them clearly violating the prime directive, having Riker beam Data down instead of O'Brien, so that only Riker would be implicated in the crime and so on.
Sarjenka really could have used a lesson in internet safety, because you are not supposed to tell strange androids your family details! It could have been Lore instead of Data!
#the next generation rewatch#star trek#star trek tng#tng#star trek the next generation#this post was exiled by the queue continuum
9 notes
Ā·
View notes
Text
Men Underestimate and Women Overestimate Their Own Sexual Violence
Time for an excellent new (2024) article "Gender Differences in Sexual Violence Perpetration Behaviors and Validity of Perpetration Reports: A Mixed-Method Study".
What this study did:
This study asked 23 men and 31 women to "think out loud while privately completing [the Sexual Experiences Survey-Short Form Perpetration (SES-SFP) survey] and to describe (typed response) behaviors that they reported having engaged in on the SES". The researchers asked anyone who "reported no such behavior ... to describe any similar behaviors they may have engaged in". They then analyzed differences in the quantitative responses (numerical values on the SES) and the qualitative responses (written descriptions and think-aloud audio).
What this study found (broad strokes):
Menās sexual violence (SV) perpetration was more frequent and severe than womenās
Menās verbal coercion was often harsher in tone and men more often than women used physical force (including in events only reported as verbal coercion on the SES)
Women often reported that their response to a refusal was not intended to pressure their partner or obtain the sexual activity*
Two women also mistakenly reported experiences of their own victimization or compliance (giving in to unwanted sex) on SES perpetration items, which inflated womenās SV perpetration rate
Quantitative measurement can miss important qualitative differences in women and menās behaviors and may underestimate menās and overestimate womenās SV perpetration
*This phrasing is poor (in my opinion) the authors are emphasizing genuine differences in men and women's reported behavior for ambiguous situations (not just their internal intent). Specifically, women would endorse responses for behaviors that (most) people would not actually consider a form of sexual violence. For example, women often indicated that the behaviors they were reporting were all pre-refusal (i.e., the women stopped and respected when their partner said no/told them to stop). Other "seducing" behaviors (e.g., kissing/touching) were also reported by women because their partner ultimately refused. Men did not report these types of behaviors, which the authors suggest is possibly because women may be more likely to remember experiences where they wanted to engage in sex with someone who did not because this violates social norms. It's also possible that men are more likely to consider these behaviors acceptable provided they stop when refused. (Ironically this suggests that the anti-feminist hyperbole that people will start recording "normal sexual interactions" as violence ... has only affected women.)
Lots more details below the cut (I use a mix of - unmarked - quotes and paraphrasing):
Quantitative data
The overall prevalence of sexual perpetration of significantly inflated due to intentional over-sampling of likely perpetrators (particularly female perpetrators). This is reasonable because the authors are interested in examining differences among self-reported perpetrators, not in establishing incidence/prevalence rates.
Even without taking the qualitative aspects into consideration (i.e., looking only at the quantitative data), men reporting SV perpetration reported more frequent offenses than women (re-offended more often). Men were also more likely to report more severe acts of violence (per the original tactic-act, the tactic specific, and sexual act specific continua).
Differences in severity identified via qualitative analysis
Menās verbal coercion was more often stronger; more deceptive, persistent, or intimidating; or otherwise harsher in tone (e.g., "She kept refusing to do anything with me. I remember saying to her ājust cause youāre on your period doesnāt mean I canāt get head.ā I then remember repeating my intentions with her and almost gaslighting her and making her feel that she must not love me."). Proportionally more men described continually asking or persisting after repeated refusals, getting angry, telling lies, making false promises, and trying to make their partner feel guilty.
Womenās verbal coercion was predominantly expressing disappointment or pouting after a single refusal (e.g., āI got upset and said whatever and rolled over the opposite wayā)
Also a difference in intent that could only be identified in the qualitative data. 35% of women who perpetrated explicitly said they had not intended to pressure their partner, change their partnerās mind, or obtain the sexual activity after their partner refused (e.g., "I respected him not trying to do anything further, though, and did not attempt anything further."). No men explicitly said they had not intended to pressure their partner or obtain the sexual activity and [men] more often than women explicitly said that they had intended to (e.g., "I think it was one time where I just kept pressuring . . . Didnāt happen, but the pressure was there, thatās for sure. I definitely asked more than a couple times.")
A few of womenās SV perpetration behaviors appeared more like attempts to advocate for equity in their own sexual pleasure or to stick up for themselves in response to a partnerās coercion (e.g., "I really love receiving oral sex. But sometimes my partner ignores that and directly goes to the penetration. So, I stop him and make him do it because I also feel like being properly aroused to get a better sexual experience.")
False negatives
Some participants that did not mark any of the perpetration items still described similar experiences. Most were not coercive (e.g., asking and ārespectingā a refusal, clarifying an unclear refusal) but a couple were clear false negatives. There appears to be an issue with some behaviors not clearly fitting into any of the described categories (e.g., Even the physical force SES items refer only to more extreme force (holding down, pinning arms, having a weapon).)
There were many more cases where a less severe offense was marked (i.e., coded as a true positive for perpetration but for incorrect offense in severity analysis). Specifically, men reported only verbal coercion but then described physical behaviors, so the tactic report was incorrect or incomplete (e.g., "We were experimenting with different things and I did not necessarily ask for their consent before putting my finger in their butt." was coded by one man as verbal coercion).
False negative may have occurred, in part, because behaviors that were themselves no different than those performed in consensual sex were not adequately captured. This is a problem given that previous qualitative research has also found that initiating or going ahead with penetration without asking or following a refusal is a common SV perpetration behavior used by men (i.e., this type of behavior may be recorded as either a false negative or a less severe offense in quantitative scales).
When women reported verbal coercion only, but then described initiating sexual acts without asking, they almost always initiated non-penetrative sexual acts in contrast to men who more often described penetrative sexual acts without asking.
The SES may underestimate use of physical force and, especially, menās rape and attempted rape.
False positives
Some participants reported perpetration on the SES that their description showed was not forceful, coercive, or engaged in without consent or following a refusal. Men explained that they did not engage in the behavior, misread or misinterpreted the SES question, or clicked the wrong response. Some women reported these same problems, but two "were reports of victimization or giving in to unwanted sex" (i.e., mistakenly reported victimization as perpetration).
Notably, three out of the four men with false positives reported other instances of SV perpetration on the SES whereas two of the four women with false positives did not report other perpetration and, therefore, inflated womenās perpetration rate.
Taken together, our analysis of false negatives and false positives suggests that the SES likely underestimates menās SV perpetration and overestimates womenās perpetration.
This doesn't even account for instances reporting no intent to perpetrate (as described above). But the fact that many women reported no intent may further support the conclusion that women overreport or are more likely to remember and report because their coercion violates social expectations
Verbalized thought processes
In general, most participants appeared to understand and interpret the SES as intended
But there was evidence that the distinction between attempted and completed acts on the SES may be unclear for some respondents (e.g, one woman said "I also donāt understand what they mean by ātried.ā Like does this mean that . . . You simply spoke to them, and they said no? Does this mean that you were engaged in an act and they pushed you off? Or does this mean that something disrupted you? So, this question doesnāt seem very clear to me.")
Second, participants used different items on the SES to report having used a specific category of tactic that is not mentioned in the measure. For example, some participants described kissing and sexually touching their partner without asking to try to arouse them and reported this as verbal tactics to obtain non-penetrative sexual contact. This may have underestimated attempted and completed sexual coercion (because the intent was to engage in penetrative sex). It may also have overestimated non-consensual non-penetrative sexual contact category (the most frequent category for female offenders) since research also finds that partners often use nonverbal cues including kissing and touching to communicate about sexual interest.
There was also confusion about the meaning of āgetting angryā or "showing displeasure". Some participants (particularly women) indicated these could refer to internal feeling as opposed to external expression or be a ānormal human reaction to . . . feeling rejectionā that does not necessarily include a purposeful attempt to manipulate.
Other problems: (1) confusion on if intoxication only applied to alcohol, (2) too many tactics listed in a single question resulting in confusion, (3) participant frequency estimates were rough estimates likely contributing to a significant underestimation problem, (4) participants wouldn't endorse items that specified "without consent" even if they later described coercive behaviors suggesting different phrasing may be needed, (5) participants reported shock at the severity of the tactics asked about, which may indicate SV is not normalized among non-perpetrators or may indicate that less severe tactics are not being captured
Concerning (4) above: Other research indicates that while conceptually narrower, asking about behaviors done after someone resisted or indicated ānoā (i.e., post-refusal persistence) results in higher rates of self-reported SV perpetration than asking about behaviors done without consent or when the other person did not want to.
Citation: Jeffrey, Nicole K., and Charlene Y. Senn. āGender Differences in Sexual Violence Perpetration Behaviors and Validity of Perpetration Reports: A Mixed-Method Study.ā The Journal of Sex Research, Feb. 2024, pp. 1ā16. DOI.org (Crossref), https://doi.org/10.1080/00224499.2024.2322591.
32 notes
Ā·
View notes
Text
My Upcoming Research Study: The Effects of Yogic Breathing on Chronic Sinus Symptoms
This week I have been putting together a presentation that I will be giving about my new research project. It's a study of a yogic breathing technique called Bhramari Pranayama as an adjunctive treatment for people with chronic nose and sinus issues.
Essentially the premise is this:
Our sinuses produce nitric oxide, which then in turn effects our nasal airway and our lungs. The nitric oxide can improve nasal airflow, up-regulate mucociliary clearance, and enhance anti-viral immune activity. Additionally, humming actually temporarily increases the amount of nasal nitric oxide released by about 15 fold. Therefore my study is intended to examine the effects of this pranayama technique that utilizes humming on patients with chronic nose and sinus symptoms.
Typically for patients with these symptoms, we start them out on a steroid nasal spray (flonase) as well as sinus irrigations (something like a neti pot).
I always hear from people outside the medical field about how no one studies these things--non-pharmacologic interventions, the beneficial effects of non-proprietary supplements, or other alternative medical options. People often think that if big pharma (or someone expecting to profit) isn't paying for a study, it cannot happen.
This really isn't true likely 90-99% of the time. The problem isn't funding. I'm conducting a prospective randomized control study with human subjects in order to evaluate the benefits of yogic breathing for patients--something that if found to be helpful will bring in no additional monetary profit for anyone. How much will my study cost? $0.
But do you know what it did require?
Two things: interest and opportunity.
Firstly I, a resident physician, had an idea. I learned about nasal nitric oxide and thought it was cool. I read about how humming has a bolusing effect by transiently increasing nitric oxide output by 15x. And then I recalled that there are pranayama techniques that utilize humming.
With my interest piqued, I spoke with one of my bosses, an attending physician at an academic medical center. He's the head of our Rhinology and Skull Base Neurosurgery division and he is cool as hell. He's all about healthy lifestyle and benefiting patients as much as possible. He loved the idea immediately.
And lastly we roped in a medical student. Med students are very helpful with doing the grunt work of collecting the data into spreadsheets, running the statistical analyses and such. Sometimes they bring some excellent ideas of their own as well. In return for their work, med students are often given a significant portion credit upon publication of the study and this allows them the opportunity to add some scholarly publications to their CV. I don't really need more publications under my name, but they do.
My point with sharing all of this is that people often claim there are health benefits to doing or imbibing certain things but that they'll never be studied because there's no money to be made. And it may be true that private companies such as those in the pharmaceutical industry may not have such interest; their existence in a capitalistic economy relies on profitability. But this is part of why academic institutions are so important--because learning and discovery is part of the essential mission there. Profit doesn't dictate their avenues of research.
When it comes to the study and validation of alternative/complimentary medicine, the focus really needs to be on raising awareness and interest. Talk to your doctors, nurses, physician assistants, etc. The good ones listen. The younger they are, the more likely they are to be open-minded about it too (the older ones are hit or miss--some are so cool and some are very old school).
Just some errant thoughts this week as I work on my slide deck.
LY
29 notes
Ā·
View notes
Note
in the tags on a recent post you said your day job is "mind numbingly simple" do you know if that's common of chemical engineering jobs?
(i am currently pursuing a chemical engineering degree and honestly don't know that much about chemical engineering jobs. but i would not mind a simple job that gives me mental capacity left to write at the end of the day)
So it strongly depends on the kind of engineering work you end up going into and any job will vary in complexity on a day to day basis but with a chemical engineering job you have a lot of different options!
Specifically I'm a R&D Applications Engineer/Technical Customer Service in a polymer science role for a big international corporation so I'm working with existing products in a company and figuring out how to make them work for customers who are having issues.
What this looks like on a project to project basis is that we get an email from the customer or the plant outlining the problem and what kind of material they're sending us to test, I design the experiments we need to do to validate all the variables and properties, and then I spend a few weeks in the lab churning out data, then plug it into an Excel spreadsheet, crunch the numbers, throw that in a PowerPoint, and send it off to the relevant personnel.
The mind numbingly simple part is the standing in lab running through tests because it's hands-on labor that requires very little thinking once you've established your parameters. I usually just put on an audiobook or a podcast to kill time. The design of experiment can get somewhat complex and you have to be very good at time management if you have multiple projects with time sensitive lab components going on at once, but the number crunching has never required anything more complicated than 10th grade algebra. I'm not doing much chemical formulation either, just following established recipes and procedures within my company, but I'm learning more specific stuff as I go.
Now I'm only a year out of college and I've never had an internship or anything that WASN'T in a non-lab setting, so I can't speak to how something like a Process Engineer spends their time. I knew I didn't like being out on the plant floor because it's often Loud and Dirty and Sensory Overload so I tried to avoid applying for those roles. You learn a lot more about the production that way though, just not my cup of tea. You also have chemical engineers who design entire chemical plants and control systems (which is very very cool and important but I was bad at those classes lmao). Some also go the biochemical angle and get into pharmaceuticals but medicine scares me.
That's just my personal take but I encourage you to talk to your professors and upperclassman and see what they have to say! Career fairs >>>>>>> linked in for getting anywhere in this hell of a job market if your school has them and I hope you have a better time of it than I did during the COVID times. š«”
16 notes
Ā·
View notes
Note
Dude the chapter 11 snippet for 25H,,,,,, I love Noma š she's so cute. I'm fully obsessed with her.
I know the vibe is Sexual Tension~ and pushing the big sexy man and his big sexy brain's buttons but the way she goes about it is so earnest which makes it so so so endearing.
Like Yoongi is over here Sweating, horrifically down bad and she's so earnestly conducting her little scientific experiment on what to say/when to illicit the reactions she wants to see more of.
She's just so endearing š š I love reading the way her brain works
Also I love her and Jimin's dynamic with hobi being the devious little instigator. Jimin just being like "I'm so done with you" and noma being like ok but..... that is extremely secondary to the questions I need answered.
I truly love your commitment to making relationships with characters complex and allowing characters to not be simply assigned into categories like "good" or "bad". Because the little we have seen of jimins reasonings of not liking Noma like!!!! Totally fair I think. It would not be fun to see your friend put through this agony over and over again with no change. I also just like that you've written a relationship where they disagree fundamentally and/or have different views of a person and that's normal for them. A lot of times now I think people often write romantic interests as being perfectly aligned in thought and morality and š“. Complex characters have been missing in forms of media in recent years and it makes the stories just so much better and interesting to read.
Oh my god this is so long I'm so sorry šØ
HELLOOOOOOOOOOOOOO ANON. First of all. How dare you. How DARE you apologize for giving me a beautifully thought out, high-quality, brain-rich take like this. Do you think Iām one of those girlies who doesnāt want 15-page essays in their inbox?? Do you think I post 20k chapters and go āoh no!! not a long message ššā??? No. No, babe. Iām thriving. Iām flourishing. Iām feeding on this like a little lore gremlin with my claws out.
Okay NOW. Letās talk about Noma. YES!!! Thatās literally itāthatās her. Thatās the exact dynamic I wanted to show. Like, sheās doing science. Sheās running numbers. Sheās like āHm. If I say āyour theory is flawedā in a lower tone, his pupils dilate. Interesting. If I say ādefine dissonanceā and tilt my head 3 degrees he gets all rigid. Letās repeat that test.ā Meanwhile Yoongi is across from her like āš„µšØšØš„µš§Ø.ā Itās so funny because to her, this is just data collection. Sheās not even trying to be sexyāsheās just accidentally hot because sheās smart and terrifying and deeply fixated on learning Yoongi inside and out like a system to decode.
And YES oh my God thank you for noticing the Noma/Jimin/Hoseok dynamic. Jimin is 900% just done⢠but also still participating because he cares, but he hates that he does, and Hoseok is like āIām here to stir the pot and narrate and emotionally instigate :)ā and Nomaās like āOk valid but hereās a spreadsheet and six follow-up questions.ā She is NOT emotionally fluent in human friction but she is excellent at interrogating inconsistencies. Jiminās like āI think youāre ruining my friendās life,ā and sheās like āMm ok but letās define what constitutes āruin.āā
And the point you made about not flattening characters into āgoodā or ābadā?? YES. Literally thank you. THANK YOU. We are so past that era. I hate when I see cast dynamics written where everyone is just perfectly aligned because thatās not how people work. Jimin having valid reasons for disliking her? That matters. Because heās not a villaināheās not jealous or petty or wrongāheās just processing the trauma of watching his best friend get torn apart and is like āI canāt see this happen again.ā Itās such a real, heavy thing. And Noma doesnāt take it personallyāshe sees it, catalogs it, understands it. Thatās what I mean when I say āmessy emotional realism.ā People clash. People grieve. People love differently.
So. Yeah. This message made my whole day. And I will never apologize for being a writer who craves nuance, friction, and moral divergence. Thatās where the real intimacy lives.
Thank you for reading. Thank you for caring. You are SO valid and intelligent and welcome here. Please come back always. Iām obsessed with you. š«
3 notes
Ā·
View notes
Text
Python Programming Language: A Comprehensive Guide
Ā Python is one of the maximum widely used and hastily growing programming languages within the world. Known for its simplicity, versatility, and great ecosystem, Python has become the cross-to desire for beginners, professionals, and organizations across industries.
What is Python used for

š What is Python?
Python is a excessive-stage, interpreted, fashionable-purpose programming language.Ā The language emphasizes clarity, concise syntax, and code simplicity, making it an excellent device for the whole lot from web development to synthetic intelligence.
Its syntax is designed to be readable and easy, regularly described as being near the English language. This ease of information has led Python to be adopted no longer simplest through programmers but also by way of scientists, mathematicians, and analysts who may not have a formal heritage in software engineering.
š Brief History of Python
Late Nineteen Eighties:Ā Guido van Rossum starts work on Python as a hobby task.
1991:Ā Python zero.9.0 is released, presenting classes, functions, and exception managing.
2000:Ā Python 2.Zero is launched, introducing capabilities like list comprehensions and rubbish collection.
2008: Python 3.Zero is launched with considerable upgrades but breaks backward compatibility.
2024:Ā Python three.12 is the modern day strong model, enhancing performance and typing support.
ā Key Features of Python
Easy to Learn and Use:
Python's syntax is simple and similar to English, making it a high-quality first programming language.
Interpreted Language:
Python isn't always compiled into device code; it's far done line by using line the usage of an interpreter, which makes debugging less complicated.
Cross-Platform:
Python code runs on Windows, macOS, Linux, and even cell devices and embedded structures.
Dynamic Typing:
Variables donāt require explicit type declarations; types are decided at runtime.
Object-Oriented and Functional:
Python helps each item-orientated programming (OOP) and practical programming paradigms.
Extensive Standard Library:
Python includes a rich set of built-in modules for string operations, report I/O, databases, networking, and more.
Huge Ecosystem of Libraries:
From data technological know-how to net development, Python's atmosphere consists of thousands of programs like NumPy, pandas, TensorFlow, Flask, Django, and many greater.
š Basic Python Syntax
Here's an instance of a easy Python program:
python
Copy
Edit
def greet(call):
Ā Ā print(f"Hello, call!")
greet("Alice")
Output:
Copy
Edit
Hello, Alice!
Key Syntax Elements:
Indentation is used to define blocks (no curly bracesĀ like in different languages).
Variables are declared via task: x = 5
Comments use #:
# This is a remark
Print Function:
print("Hello")
š Python Data Types
Python has several built-in data kinds:
Numeric:Ā int, go with the flow, complicated
Text: str
Boolean:Ā bool (True, False)
Sequence:Ā listing, tuple, range
Mapping:Ā dict
Set Types:Ā set, frozenset
Example:
python
Copy
Edit
age = 25Ā Ā Ā Ā Ā Ā Ā # int
name = "John"Ā Ā Ā Ā # str
top = 5.NineĀ Ā Ā Ā Ā # drift
is_student = TrueĀ Ā # bool
colors = ["red", "green", "blue"]Ā # listing
š Control Structures
Conditional Statements:
python
Copy
Edit
if age > 18:
Ā Ā print("Adult")
elif age == 18:
Ā Ā print("Just became an person")
else:
Ā Ā print("Minor")
Loops:
python
Copy
Edit
for color in hues:
Ā Ā print(coloration)
while age < 30:
Ā Ā age += 1
š§ Functions and Modules
Defining a Function:
python
Copy
Edit
def upload(a, b):
Ā Ā return a + b
Importing a Module:
python
Copy
Edit
import math
print(math.Sqrt(sixteen))Ā # Output: four.0
šļø Object-Oriented Programming (OOP)
Python supports OOP functions such as lessons, inheritance, and encapsulation.
Python
Copy
Edit
elegance Animal:
Ā Ā def __init__(self, call):
Ā Ā Ā Ā self.Call = name
def communicate(self):
Ā Ā Ā Ā print(f"self.Call makes a valid")
dog = Animal("Dog")
dog.Speak()Ā # Output: Dog makes a legitimate
š§ Applications of Python
Python is used in nearly each area of era:
1. Web Development
Frameworks like Django, Flask, and FastAPI make Python fantastic for building scalable web programs.
2. Data Science & Analytics
Libraries like pandas, NumPy, and Matplotlib permit for data manipulation, evaluation, and visualization.
Three. Machine Learning & AI
Python is the dominant language for AI, way to TensorFlow, PyTorch, scikit-research, and Keras.
4. Automation & Scripting
Python is extensively used for automating tasks like file managing, device tracking, and data scraping.
Five. Game Development
Frameworks like Pygame allow builders to build simple 2D games.
6. Desktop Applications
With libraries like Tkinter and PyQt, Python may be used to create cross-platform computing device apps.
7. Cybersecurity
Python is often used to write security equipment, penetration trying out scripts, and make the most development.
š Popular Python Libraries
NumPy:Ā Numerical computing
pandas:Ā Data analysis
Matplotlib / Seaborn:Ā Visualization
scikit-study:Ā Machine mastering
BeautifulSoup / Scrapy:Ā Web scraping
Flask / Django: Web frameworks
OpenCV:Ā Image processing
PyTorch / TensorFlow:Ā Deep mastering
SQLAlchemy:Ā Database ORM
š» Python Tools and IDEs
Popular environments and tools for writing Python code encompass:
PyCharm:Ā Full-featured Python IDE.
VS Code:Ā Lightweight and extensible editor.
Jupyter Notebook:Ā Interactive environment for statistics technological know-how and studies.
IDLE:Ā Pythonās default editor.
š Strengths of Python
Easy to study and write
Large community and wealthy documentation
Extensive 0.33-birthday celebration libraries
Strong support for clinical computing and AI
Cross-platform compatibility
ā ļø Limitations of Python
Slower than compiled languages like C/C++
Not perfect for mobile app improvement
High memory usage in massive-scale packages
GIL (Global Interpreter Lock) restricts genuine multithreading in CPython
š§ Learning Path for Python Beginners
Learn variables, facts types, and control glide.
Practice features and loops.
Understand modules and report coping with.
Explore OOP concepts.
Work on small initiatives (e.G., calculator, to-do app).
Dive into unique areas like statistics technological know-how, automation, or web development.
#What is Python used for#college students learn python#online course python#offline python course institute#python jobs in information technology
2 notes
Ā·
View notes
Text
Sales Tracker vs Sales Automation: What's Right for SMBs in 2025?

In today's digital economy, tracking sales activities has become more important than ever. Whether weāre at networking events or meeting customers, one question often comes up:
āCan we keep track of our sales team without making them feel monitored all the time?ā
Our answer: āYou are tracking activities, not people.ā
In 2025, small FMCG businesses and emerging CPG brands need to track on-field sales. Writing retailer orders in notebooks was fine back in the day.
Sales Tracker vs Sales Enabler: Whatās the Difference?
Sales Tracker: A tool to digitize and monitor sales activities in real time. It offers basic data but minimal insights.
Sales Enabler: An advanced solution that automates processes and uses real-time insights to provide detailed data on sales volume, outlet visits, performance trends, and more.
For example, the Sales Force Automation App goes beyond tracking. They analyze historical data to suggest better routes, product assortment, and provide actionable insights to improve performance.
Why Do SMBs Need a Sales Enabler in 2025?
Many small FMCG brands still rely on Excel sheets to track sales. While it works in the early stages, managing a business in todayās competitive market, where over 12 million retail outlets serve products from 30,000 brands, requires more advanced tools.

Hereās what a Sales Enabler can do for SMBs:
Validation of Outlet Visits: This is an essential feature that ensures every visit to a retailer is genuine. With geo-fencing, you can confirm each visit, while live tracking allows you to monitor the number of outlets visited, the average time spent at each outlet, and other key activities like leaves or meetings. This gives you a clear picture of your field teamās productivity throughout the day.
Smart Order Booking: For emerging businesses, smart order booking takes sales tracking to the next level by enabling faster and smarter order processing.
3. Automated Attendance Management: KRA-based attendance management system brings transparency and discipline to field operations. By implementing a KRA-based attendance policy, you can encourage salespeople to meet defined goals like total outlet visits or starting their beat on time.
Benefits of Sales Enablement Solutions for Growing Businesses

Improved Productivity: Sales enablement platforms ensure higher compliance and productivity by closely monitoring field activities.
Improved Market Penetration: Unlike basic sales trackers, sales enablement solutions provide in-depth insights into market penetration.
Better Sales Performance: By analyzing historical data, business owners and sales leaders can identify trends and patterns in consumer behavior, such as which products are in demand, when, and where.
Competitive Edge: Sales enablement solutions also enable growing businesses to stay ahead of competitors by tracking how their brand performs against others in the market.
Accurate Forecasting: A good sales enabler like the SFA App provides historical data comparisons, including year-on-year, month-on-month, and even three-month trends.
2 notes
Ā·
View notes
Text
Shin Sekai Yori and the Fear of the Unknown
Shin Sekai Yori by A-1 Pictures (2012 ā 2013) was the latest feature in my anime exploration. The visuals of the show were excellent, especially the way that the animators played with light. Scenes set at dusk with dynamic skies and silhouetted features captured the look, but more importantly the feel of twilight. Scenes at night and in caves captured the way that space closes in on you when light wanes adding drama and suspense to the story.
Shin Sekai Yori is more than just pretty pictures. The series comments insightfully on deep issues including class dynamics, power and what it means to be human. Another prominent theme addressed in the series is fear ā specifically fear of the unknown. There are some dangers that are easy to understand. Tigers are deadly, driving above the speed limit increases risk and certain places at certain times have higher risk for crime. However, when violence erupts in places, times and at the hands of those we donāt expect primal fears activate as society tires to understand why something so horrible could happen.
The anxieties surrounding unexplainable violence in real life Japan are mirrored in Shin Sekai Yori. Episode 4 reveals that the start of the disruption in social order after people were discovering to use their cantus was sparked by āBoy Aā, a young child who went on a killing spree, breaking into homes and committing brutal crimes. This a reference to the crimes committed by ShÅnen A, a young murderer in actual Japan in the late 1990ās (Arai, 2000). Both events shook society and in the universe of Shin Sekai Yori strong action was taken eventually culminating genetically modifying humanity to have an automatic physical response that prevents them from harming others.
Possibly more terrifying than unexpected violence is when the actions that we take to control the situation are not completely effective. In Shin Sekai Yori, some children, known as Fiends, are immune to their genetically imposed killing instincts. SSYās main character Saki learns the truth about Fiends when she is told the story of a young boy who is obsessed with dark ideas. One day the boy goes on a massacre. His lack of restraint coupled with the rest of societies physical limitation from harming him is a worst case scenario of an out of control threat. Eventually the killer is defeated, but even stronger measures are taken to attempt to eliminate the chances that someone will develop into a Fiend. Anyone even remotely displaying troublesome traits will be eliminated. It mostly works, but the show struggles with the idea of the cost of these measures. How many innocent lives are lost in the pursuit of public safety? What is the acceptable margin of error when trying to prevent a catastrophe?
This dilhemma exists in the real world too. One example is how artificial intelligence and machine vision are already being used to identify possible school shooters. Max Zahnās ABC News article Can Artificial Intelligence Help Stop Mass Shootings? discusses the use and reaction to these types of systems. Fortunately a key difference exists between these systems and the pre-emptive action taken by society in SSY. The systems being used today only flag actions as a response to an attack already happening. The article mentions that some are concerned about innocent people being flagged as threats. Someoneās life being tainted by a false identification is a valid concern. However, the problem isnāt that the data is being collected or analyzed in an automated way, itās societyās reaction to the data that is collected. Instead of using the surveillance data to punish, it should be used to intervene and help people before the turn to violence. The solution isnāt punishing an individual, itās creating systemic fixes.
References:
Arai, A. (2000). The "Wild Child" of 1990s Japan. The South Atlantic Quarterly, 99(4), 841-863.
Zahn, M. (2023, February 2). Can Artificial Intelligence Help Stop Mass Shootings? ABC News. https://abcnews.go.com/Technology/artificial-intelligence-stop-mass-shootings/story?id=96767922
2 notes
Ā·
View notes
Text
The Dos and Donāts of AI & ML in Digital Marketing
Artificial intelligence (AI) and machine learning (ML) are revolutionizing the digital marketing landscape, offering unprecedented opportunities for personalization, automation, and optimization. However, like any powerful tool, AI and ML must be wielded wisely. This blog outlines the dos and don'ts of leveraging these technologies effectively in your digital marketing strategies.
The Dos:
Do Define Clear Objectives:Ā Before implementing any AI/ML solution, clearly define your marketing goals. What are you trying to achieve? Increased conversions? Improved customer engagement? Specific objectives will guide your AI/ML strategy and ensure you're measuring the right metrics.
Do Focus on Data Quality:Ā AI/ML algorithms are only as good as the data they are trained on. Prioritize collecting clean, accurate, and relevant data. Invest in data cleansing and validation processes to ensure the reliability of your AI-driven insights.
Do Start Small and Iterate:Ā Don't try to implement everything at once. Begin with a specific use case, such as automating social media posting or personalizing email campaigns. Test, refine, and iterate on your approach before scaling up.
Do Prioritize Personalization:Ā AI/ML excels at personalization. Leverage these technologies to create tailored content, product recommendations, and offers for individual customers based on their behavior, preferences, and demographics.
Do Embrace Automation:Ā AI can automate repetitive tasks, freeing up marketers to focus on strategic initiatives. Identify areas where AI can streamline workflows, such as ad campaign optimization, content curation, or customer service interactions.
Do Focus on Transparency and Explainability:Ā Understand how your AI/ML models work and ensure they are transparent and explainable. This is crucial for building trust and addressing ethical concerns.
Do Measure and Analyze Results:Ā Track the performance of your AI/ML-driven marketing campaigns and analyze the data to identify areas for improvement. Use data to inform your decisions and optimize your strategies.
Do Stay Updated:Ā The field of AI/ML is constantly evolving. Keep up with the latest advancements, new tools, and best practices to ensure you're maximizing the potential of these technologies.
The Don'ts:
Don't Treat AI as a Magic Bullet:Ā AI/ML is a powerful tool, but it's not a magic solution. It requires careful planning, implementation, and ongoing management. Don't expect overnight results without putting in the effort.
Don't Neglect Human Oversight:Ā While AI can automate tasks, it's essential to maintain human oversight. Human judgment is still crucial for strategic decision-making, creative development, and ethical considerations.
Don't Over-Rely on Automation:Ā While automation is beneficial, don't over-automate to the point where you lose the human touch. Maintain a balance between automation and human interaction to ensure a personalized and engaging customer experience.
Don't Ignore Ethical Implications:Ā AI/ML raises ethical concerns about data privacy, bias, and transparency. Be mindful of these issues and ensure that your AI-driven marketing practices are ethical and responsible.
Don't Forget About Data Security:Ā Protecting customer data is paramount. Implement robust security measures to safeguard your data from unauthorized access and breaches.
Don't Be Afraid to Experiment:Ā AI/ML is a field of experimentation. Don't be afraid to try new approaches, test different algorithms, and learn from your mistakes.
Don't Underestimate the Importance of Training:Ā Proper training is essential for effectively using AI/ML tools and understanding their capabilities and limitations. Invest in training for your marketing team to ensure they have the skills they need to succeed.
Digital Marketing & AI Certification Program: Your Path to AI-Powered Marketing Mastery
Want to become a sought-after digital marketing professional with expertise in AI and ML? Consider enrolling in aĀ Digital Marketing & AI Certification Program. These programs provide comprehensive training on the latest AI/ML tools and techniques, preparing you to leverage the power of these technologies in your marketing strategies. You'll learn how to:
Develop and implement AI/ML-driven marketing campaigns.
Analyze data and generate actionable insights.
Choose and use the right AI/ML marketing tools.
Address ethical considerations related to AI/ML in marketing.
Conclusion:
AI and ML are transforming the landscape of digital marketing, offering unprecedented opportunities for growth and innovation. By following these dos and don'ts, marketers can harness the power of these technologies effectively and responsibly, driving better results and achieving their marketing goals. The future of digital marketing is intelligent, and it's powered by AI and ML.
#technology#artificial intelligence#online course#ai#marketing#digital marketing#ai in digital marketing
4 notes
Ā·
View notes
Text
Dan Pfeiffer at The Message Box:
Despite minimal evidence, a full-bore effort is underway to make Democrats think Kamala Harris is losing the election. This effort is abetted by preternaturally anxious Democrats expressing their concerns on social media. I have written a lot recently about the vibe shift in Democrats after Kamala Harrisās nomination. I donāt think the data validates such an extreme shift in emotions. However, I wonāt shame anyone for being on edge these last two weeks. The stakes are enormous. Reproductive freedom, health care, democracy, and the planet are on the line in an election that could be decided by the weather in a random suburban Wisconsin county. Long time readers of this newsletter know that I am not exactly a glass-half-full kinda guy. I tend to hang out on the dark side, but I will resist that temptation. How one feels over the next two weeks is a choice. There is nothing cool or savvy about predicting a loss. More importantly, thanks to you-know-who, I believe that hope is a powerful force. If you want to be hopeful over the next few weeks, I will give you some reasons ā borne of data and experience ā to justify an optimistic approach.
Before you read another word, let me offer some critical stipulations:
I am not making a prediction (Iām never doing that again after 2016);
I truly have no idea what will happen (Neither does anyone else);
I acknowledge that theĀ pollsĀ mightĀ have moved slightly in Trumpās direction over the last few weeks; and
The political environment and the Electoral College favor Trump on paper.
When writing for the Internet, you are incentivized to tell people what they want to hear. I try to resist that temptation, but I have upset many of you over the years with negative takes ā especially after the Biden-Trump debate. However, the national political discourse lacks arguments for why Harris will win. So, with those painful caveats in mind, hereās the optimistic case for Kamala Harris.
2. Harris Has a (Slightly) Easier Path to 270
The Electoral College has a Republican bias. According to theĀ New York TimesĀ polling average, five of the seven battleground states are within less than a point and no candidate holds a lead in any state greater than two points. There is no easy path to 270, but Harrisās is slightly easier. If she wins Pennsylvania, Wisconsin, Michigan and the second congressional district of Nebraska, she will get 270 electoral votes and be the next President of the United States. [...]
3. Trump is Not Closing Strong
There is a reason why Kamala Harris used the debate to invite people to attend or watch a Trump rally. Itās the same reason why Harris is now playing video clips of her opponent at her rallies. Trump is a disaster on the public stage. And heās getting worse as the election nears.Ā [...]
4. Harris Runs the Better Field Operation
Kamala Harris has invested a tremendous amount of time and money into building a massive field operation to persuade undecided voters and turn out the base. Just last weekend, Harris campaign volunteers made five million phone calls to voters ā five million calls in one weekend! The Trump campaign has largely outsourced their field operations to Elon Musk and other outside entities.Ā
Dan Pfeiffer makes an excellent case for being optimistic towards Kamala Harrisās chances of winning the Presidency.
3 notes
Ā·
View notes
Text
AI Frameworks Help Data Scientists For GenAI Survival
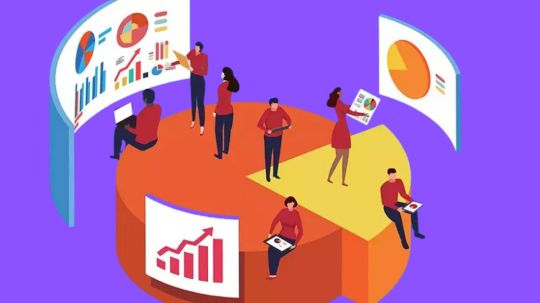
AI Frameworks: Crucial to the Success of GenAI
Develop Your AI Capabilities Now
You play a crucial part in the quickly growing field of generativeĀ artificial intelligenceĀ (GenAI) as a data scientist. Your proficiency in data analysis, modeling, and interpretation is still essential, even though platforms like Hugging Face and LangChain are at the forefront of AI research.
Although GenAI systems are capable of producing remarkable outcomes, they still mostly depend on clear, organized data and perceptive interpretation areas in which data scientists are highly skilled. You can directĀ GenAI modelsĀ to produce more precise, useful predictions by applying your in-depth knowledge of data and statistical techniques. In order to ensure that GenAI systems are based on strong, data-driven foundations and can realize their full potential, your job as a data scientist is crucial. Hereās how to take the lead:
Data Quality Is Crucial
The effectiveness of even the most sophisticated GenAI models depends on the quality of the data they use. By guaranteeing that the data is relevant, AI tools like Pandas and Modin enable you to clean, preprocess, and manipulate large datasets.
Analysis and Interpretation of Exploratory Data
It is essential to comprehend the features and trends of the data before creating the models. Data and model outputs are visualized via a variety ofĀ data scienceĀ frameworks, like Matplotlib and Seaborn, which aid developers in comprehending the data, selecting features, and interpreting the models.
Model Optimization and Evaluation
A variety of algorithms for model construction are offered byĀ AI frameworksĀ like scikit-learn,Ā PyTorch, and TensorFlow. To improve models and their performance, they provide a range of techniques for cross-validation, hyperparameter optimization, and performance evaluation.
Model Deployment and Integration
Tools such asĀ ONNX RuntimeĀ and MLflow help with cross-platform deployment and experimentation tracking. By guaranteeing that the models continue to function successfully in production, this helps the developers oversee their projects from start to finish.
Intelās Optimized AI Frameworks and Tools
The technologies that developers are already familiar with in data analytics,Ā machine learning, and deep learning (such as Modin, NumPy, scikit-learn, and PyTorch) can be used. For the many phases of the AI process, such as data preparation, model training, inference, and deployment, Intel has optimized the current AI tools andĀ AI frameworks, which are based on a single, open, multiarchitecture, multivendor software platform calledĀ oneAPIĀ programming model.
Data Engineering and Model Development:
To speed up end-to-end data science pipelines on Intel architecture, use Intelās AI Tools, which include Python tools and frameworks like Modin, Intel Optimization for TensorFlowĀ Optimizations, PyTorch Optimizations, IntelExtension for Scikit-learn, and XGBoost.
Optimization and Deployment
For CPU or GPU deployment, Intel Neural Compressor speeds upĀ deep learningĀ inference and minimizes model size. Models are optimized and deployed across several hardware platforms including Intel CPUs using theĀ OpenVINOĀ toolbox.
You may improve the performance of your Intel hardware platforms with the aid of these AI tools.
Library of Resources
Discover collection of excellent, professionally created, and thoughtfully selected resources that are centered on the core data science competencies that developers need. Exploring machine and deep learningĀ AI frameworks.
What you will discover:
Use Modin to expedite the extract, transform, and load (ETL) process for enormous DataFrames and analyze massive datasets.
To improve speed on Intel hardware, use Intelās optimizedĀ AI frameworksĀ (such as Intel Optimization for XGBoost, Intel Extension for Scikit-learn, Intel Optimization for PyTorch, and Intel Optimization for TensorFlow).
Use Intel-optimized software on the most recent Intel platforms to implement and deploy AI workloads onĀ Intel Tiber AI Cloud.
How to Begin
Frameworks for Data Engineering and Machine Learning
Step 1: View the Modin, Intel Extension for Scikit-learn, and Intel Optimization for XGBoost videos and read the introductory papers.
Modin: To achieve a quicker turnaround time overall, the video explains when to utilize Modin and how to apply Modin and Pandas judiciously. A quick start guide for Modin is also available for more in-depth information.
Scikit-learn Intel Extension: This tutorial gives you an overview of the extension, walks you through the code step-by-step, and explains how utilizing it might improve performance. A movie on accelerating silhouette machine learning techniques, PCA, and K-means clustering is also available.
Intel Optimization for XGBoost: This straightforward tutorial explains Intel Optimization for XGBoost and how to use Intel optimizations to enhance training and inference performance.
Step 2: Use Intel Tiber AI Cloud to create and developĀ machine learningĀ workloads.
On Intel Tiber AI Cloud, this tutorial runs machine learning workloads with Modin, scikit-learn, and XGBoost.
Step 3: Use Modin and scikit-learn to create an end-to-end machine learning process using census data.
Run an end-to-end machine learning task using 1970ā2010 US census data with this code sample. The code sample uses the Intel Extension for Scikit-learn module to analyze exploratory data using ridge regression and theĀ Intel DistributionĀ of Modin.
Deep Learning Frameworks
Step 4: Begin by watching the videos and reading the introduction papers for Intelās PyTorch and TensorFlow optimizations.
Intel PyTorch Optimizations: Read the article to learn how to use the Intel Extension for PyTorch to accelerate your workloads for inference and training. Additionally, a brief video demonstrates how to use the addon to run PyTorch inference on anĀ Intel Data Center GPUĀ Flex Series.
Intelās TensorFlow Optimizations: The article and video provide an overview of the Intel Extension for TensorFlow and demonstrate how to utilize it to accelerate your AI tasks.
Step 5: Use TensorFlow and PyTorch for AI on the Intel Tiber AI Cloud.
In this article, it show how to use PyTorch and TensorFlow on Intel Tiber AI Cloud to create and execute complicated AI workloads.
Step 6: Speed up LSTM text creation with Intel Extension for TensorFlow.
The Intel Extension for TensorFlow can speed up LSTM model training for text production.
Step 7: Use PyTorch and DialoGPT to create an interactive chat-generation model.
Discover how to use Hugging Faceās pretrained DialoGPT model to create an interactive chat model and how to use the Intel Extension for PyTorch to dynamically quantize the model.
Read more on Govindhtech.com
#AI#AIFrameworks#DataScientists#GenAI#PyTorch#GenAISurvival#TensorFlow#CPU#GPU#IntelTiberAICloud#News#Technews#Technology#Technologynews#Technologytrends#govindhtech
2 notes
Ā·
View notes