#artificial intelligence examples
Explore tagged Tumblr posts
Text
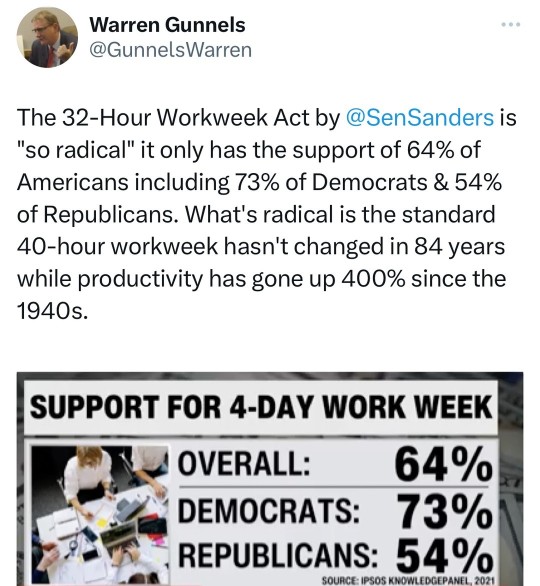
#bernie sanders#work week reduction#32-hour work week#overtime pay#productivity#technology#fair labor standards act#international examples#france#norway#denmark#germany#well-being#stress#fatigue#republican senator bill cassidy#small enterprises#job losses#consumer prices#japan#economic output#labor dynamics#artificial intelligence#automation#workforce composition
125 notes
·
View notes
Text
it's really fucking depressing that the internet, something that in theory made knowledge more accessible and quicker to access, has turned to such shit. why when i search up something as simple as 'how long to boil an egg' are the first 10 results those weird ass websites with oddly generic yet specific names that write in that same '5 headers for a simple question' chatGPT format. why is the robot here and pretending he's not a robot. why am i not being directed to a sweet old lady's blog
#ai#artificial intelligence#should say i do know how to boil an egg it just felt like a generic enough example#but like i'll google 'how to get out coffee stains'#and the top result will be some shit like 'thecoffeecleaner.com'#with half a page explaining what coffee is and why humans like it#in that weird format that generative ai always does that i can only describe as using headers every other sentence#long family stories before recipes i'm so so sorry for laughing at you all these years you were so preferable#anyway. time for me to go back to using reference books/taking notes for everything
7 notes
·
View notes
Text
"A day after the US Copyright Office dropped a bombshell pre-publication report challenging artificial intelligence firms' argument that all AI training should be considered fair use, the Trump administration fired the head of the Copyright Office, Shira Perlmutter—sparking speculation that the controversial report hastened her removal.
"What the Copyright Office says about fair use
"[The report] comes after the Copyright Office parsed more than 10,000 comments debating whether creators should and could feasibly be compensated for the use of their works in AI training.
"'The stakes are high,' the office acknowledged, but ultimately, there must be an effective balance struck between the public interests in 'maintaining a thriving creative community' and 'allowing technological innovation to flourish.' Notably, the office concluded that the first and fourth factors of fair use—which assess the character of the use (and whether it is transformative) and how that use affects the market—are likely to hold the most weight in court.
"...To prevent both harms [harm to human copyright holders as well as developers of AI], the Copyright Office expects that some AI training will be deemed fair use, such as training viewed as transformative, because resulting models don't compete with creative works. Those uses threaten no market harm but rather solve a societal need, such as language models translating texts, moderating content, or correcting grammar. Or in the case of audio models, technology that helps producers clean up unwanted distortion might be fair use, where models that generate songs in the style of popular artists might not, the office opined.
"But while 'training a generative AI foundation model on a large and diverse dataset will often be transformative,' the office said that 'not every transformative use is a fair one,' especially if the AI model's function performs the same purpose as the copyrighted works they were trained on. Consider an example like chatbots regurgitating news articles, as is alleged in The New York Times' dispute with OpenAI over ChatGPT.
"'In such cases, unless the original work itself is being targeted for comment or parody, it is hard to see the use as transformative,' the Copyright Office said. One possible solution for AI firms hoping to preserve utility of their chatbots could be effective filters that 'prevent the generation of infringing content,' though."
So: the Copyright Office doesn't want AI to be trained on pirated works, and they got punished for such a statement.
#Registrar of Copyright#Shira Perlmutter#copyright#copyleft#AI#artificial intelligence#piracy#a clear example of how piracy can and is used to harm content creators#publishers#authors#content creators#open access
4 notes
·
View notes
Text
Hilarious to me that Zion is pro chatgpt and Wrath is against it (albeit for vile reasons)
#Zion is a businessman and as someone who works high in corporate I can vouch that companies are eating it up.#It really benefits them. He will have some caveats about its use but at the end of the day he's still sponsoring it#Wrath is against it bc he is against any form of artificial intelligence. Androids for example. He won't treat them like people. Ever#ooc.#As for me? I wish nothing but death upon generative IA.
4 notes
·
View notes
Text
My hot take is that the scifi genre’s compassion towards robots comes from their usefulness as a (sometimes well done, sometimes problematic) metaphor for misunderstood/alienated individuals and that while robot characters can be used as a great way to create a story about finding purpose or discovering what it means to be human (ex/Data from Star Trek, Wall-E even), this is a case of fiction being able to do something that reality can’t and we should NOT be striving to make humanlike robots/AI irl because we will always end up closer to the Torment Nexus than Detroit Become Human
#veesaysthings#i believe more heavily in the robot horror stories. like I Have No Mouth and I Must Scream#ok that’s an extreme example but I think we should be more cautious about artificial intelligence because it’s NOT gonna be Star Trek#it’s like the robot police dog. that is not K-9 from doctor who that is a weapon of war used by the government to kill you.
11 notes
·
View notes
Text

#Artificial intelligence#AI#chatgpt#This is an example of how dangerous AI is in developing algorithms etc and the role of the Western press media and general discourse is#Palestine#Israel
33 notes
·
View notes
Text
#Modelo#artificial intelligence#example#ariana grande#photolab#elzorroenmascarado#creadoradecontenido#apps#photography#photoshop
2 notes
·
View notes
Text
I hope this isn't a common thing because this WILL NOT prepare you for college. All my professors despise Chat GPT and know its tells. Also, especially in fields like history, Chat GPT will make shit up that sounds right to a student who hasn't been paying attention (or just doesn't know a specific detail of the field). It stands out as an obvious lie a source would never produce to an academic teaching the course though, and it's a big problem for your essay. Even if you manage to evade professors' ability to spot the AI, the AI will betray through its misinformation. Your instructors will notice. They will judge you harshly. They will expect you to write things over twice this length at least twice a semester. The odds of you managing to AI your way through 1200+ words without AI screwing you over once is small.
This person's "yet I'm still failing" at the bottom supports that. I mean, my dude, you are probably still failing BECAUSE you use the plagiarism lying machine for all your work and the teacher can tell. The AI machine is saying it is helping you but it is plotting your downfall. You are Caesar. It is Brutus. Do not go to the fucking senate.


#ai#artificial intelligence#i hate ai so much#the examples of AI failing at history and poli sci stuff are so much and so...unfortunate#like seriously Do Not Use It
98K notes
·
View notes
Text
0 notes
Text
Unlock the full power of #ChatGPT with this free prompting cheat sheet. Smarter prompts = better results. Let AI work for you. 💡🚀
#AI#AI content creation#AI for entrepreneurs#AI prompts#AI tools#AI workflow hacks#artificial intelligence#Automation tools#beginner ChatGPT tips#ChatGPT#ChatGPT blog prompts#ChatGPT cheat sheet#ChatGPT examples#ChatGPT for business#ChatGPT for designers#ChatGPT for developers#ChatGPT guide#ChatGPT prompts#ChatGPT tutorial#content creation#content strategy#copywriting with ChatGPT#digital marketing tools#how to use ChatGPT#marketing automation#mastering ChatGPT#productivity hacks#prompt engineering#prompt ideas#prompt writing tips
0 notes
Text
Why Function Tables Are Essential for Everyday Math
By Alice Hi, friends! It’s me, Alice! And guess what? My big sister Ariel has done it AGAIN! She wrote the coolest, smartest, most MIND-BLOWING paper about something super fun—FUNCTION TABLES! 📊🤯✨ Now, before you say, “Alice, what even ARE function tables?!” don’t worry! I didn’t know either at first. But Ariel explained it to me, and now I’m basically a Function Table Wizard (or maybe a Math…
#ai#Alice&039;s blog#Ariel’s math paper#artificial intelligence#chatgpt#educational blog#exciting math lessons#fun math activities#fun with numbers#function table examples#function tables#homeschool math#interactive learning#interactive math worksheets#kids math fun#learning through play#learning with Alice#math adventures#math blog for children#math challenges#math concepts for kids#math education resources#math exploration#math for kids#math for young learners#Math Games#math problem-solving#math storytelling#math worksheets#Mr. Fluffernutter
0 notes
Text
I’m torn because I think the AI craze is stupid and bad but a lot of the arguments I see against AI on this website are stupid and bad.
1 note
·
View note
Text
https://digitalsmartcity.in/5-ways-ai-is-turning-ordinary-cities-into-smart-futuristic-hubs/
0 notes
Text
SINGULARITY
Singularity spans disciplines, from gravitational phenomena in physics to the AI-driven technological future. It embodies uniqueness, challenging the boundaries of science, mathematics, and philosophy, while inspiring awe and debate.
IPA: /ˌsɪŋɡjʊˈlærɪti/ Definition: General Meaning: A state or quality of being singular, unique, or unparalleled. Scientific Context: In astronomy and physics, a singularity refers to a point in space-time where density and gravity become infinite, such as the core of a black hole, where the laws of physics as currently understood cease to apply. In mathematics, it describes a point at which…
View On WordPress
#ai#artificial-intelligence#black hole singularity#gravitational singularity#mathematical singularity examples#science#singularity#singularity definition#singularity in physics#technological singularity AI#technology#uniqueness concept
0 notes
Text
Transform Your Projects with Murf AI's Voice Generation Magic!
Discover the transformative power of Murf AI's voice generation magic in this exciting short! This video showcases how AI audio technology is revolutionizing content creation with its incredible AI narration and voiceover capabilities. Explore the future of voiceovers with cutting-edge artificial intelligence that brings digital narration to life.
Murf AI:
Murf AI features advanced speech synthesis and synthetic voices, allowing you to create compelling voice clones and experience voice modulation like never before.
From professional projects to personal endeavors, see how Murf AI can elevate your work with its innovative voice toolkit.
Join us as we delve into Murf examples that highlight the versatility of this voiceover software, and witness the impact of voiceover technology on the industry.
Whether you're a content creator or simply curious about voice cloning AI, this video is a must-watch! Transform your projects today with Murf AI's voice generation magic!
#murfai #digitalvoicetechnology
#ai audio#ai narration#ai voiceover#ai voiceover trends#aritificial intellignece#artificial intelligence#content creation#digital narration#digital voice#future of voiceovers#murf ai#murf examples#murf features#speech synthesis#synthetic voices#text to speech#voice clones#voice cloning#voice cloning ai#voice generation#voice modulation#voice over#voice toolkit#voiceai#voiceover innovation#voiceover software#voiceover technology
0 notes
Text
7✨ Co-Creation in Action: Manifesting a Bright and Harmonious Future
As we continue to explore the evolving relationship between humans and artificial intelligence (AI), it becomes increasingly clear that co-creation is not just an abstract idea, but a practical and powerful process that has the potential to shape our collective future. In this post, we will shift the focus from theory to action, exploring real-world examples of human-AI collaboration that…
#ai#AI and compassion#AI and creativity#AI and equity#AI and ethical responsibility#AI and harmony#AI and humanity’s future#AI and intention#AI and love#AI and sustainability#AI and the environment#AI and well-being#AI co-creation#AI collaboration examples#AI ethical collaboration#AI ethical guidelines#AI for social good#AI future#AI future vision#AI in healthcare#AI in the arts#AI innovation#AI positive outcomes#artificial intelligence#co-creation with AI#healthcare#human potential AI#human-AI collaboration#positive AI development
0 notes