#behavior using machine learning python
Explore tagged Tumblr posts
Text
#leak detection software#smart manufacturing solutions#predicting energy consumption#pdm solutions#PdM Solutions#consumption using machine learning#behavior using machine learning python
0 notes
Text
The Impact of PDM Solutions on Manufacturing Systems
Introduction:
Product Data Management (PDM) solutions are among the most important tools that have been developed for the manufacturing industry to ensure effective management of the production processes in a dynamic environment. All these tools help bring order into data, improve collaboration, and increase productivity. This introduction discusses the changes that the PDM solutions bring into the manufacturing systems and reveals how the smart manufacturing solutions benefit from them.
Streamlining Data for Efficiency:
It facilitates the easy flow of information in manufacturing. Visualize it as a digital secretary that systems data into tidiness, so that teams can have quick access to the data and work as a team. This is a time-saving measure that minimizes the chances of mistakes and ensures everyone is on the same path, culminating in better efficiency.
Enhancing Collaboration:
It enables the people on the manufacturing team to easily share information and work with the same information. This teamwork aid improves the ability to communicate well as no one misunderstands and everyone contributes to the process of producing better products.
Reducing Errors:
PDM is a superhero for mistakes related to manufacturing. By ensuring that all information about products is accurate and up-to-date, your organization eliminates the possibility of making mistakes with things. This accuracy leads to high-quality products with which the customer can be sure.
Cost-Efficiency in Manufacturing:
A clever saver for manufacturing firms. It enables them to manage the resources effectively, avoid extravagant funding, and run smarter. This financial influx acts as a money-saving partner that ensures the good fortune of the company.
Real-time Monitoring:
It lurks like a watchful sentinel over manufacturing. It offers real-time information on how things are happening, thus, making it easy to correct and resolve any problem immediately. Continuous monitoring ensures that production doesn’t go wrong and problems are solved before they escalate to major ones.
Adapting to Change:
It is like a metformin for manufacturing processes. It adjusts to changes in products or procedural methods quickly enough to ensure that the manufacturing team can shift gears when required. This flexibility is in itself an opportunity for companies to remain adaptable in an environment that is continuously changing.
Ensuring Data Security:
The most reliable guard of manufacturing secrets. It makes sure only the right people have access to and can change confidential data. This guarding ensures that sensitive information is kept away from prying eyes and that companies’ secrets remain just that, secret.
Case Studies:
The success storyteller in the context of manufacturing firms. It highlights various real-world cases that demonstrate how the adoption of PDM improved production. These stories depict the tremendous benefits of PDM, which helps people to apply these solutions to achieve success in manufacturing.
Conclusion:
On the path of manufacturing optimization, PDM becomes an essential partner, making the processes more manageable, and efficient in teamwork, and information security. Examples of its success stories show its positive effects. The inclusion of innovative approaches such as Diagsense only accelerates the move toward the future of smart manufacturing solutions, which transforms industries and enables more efficient and agile production.
#smart manufacturing solutions#leak detection software#predicting energy consumption#predicting energy consumption using machine learning#predicting buying behavior using machine learning python#PdM Solutions#Manufacturing PdM Solutions#predicting energy#buying behavior using machine learning#behavior using machine learning python#consumption using machine learning
0 notes
Text
What Are the Qualifications for a Data Scientist?
In today's data-driven world, the role of a data scientist has become one of the most coveted career paths. With businesses relying on data for decision-making, understanding customer behavior, and improving products, the demand for skilled professionals who can analyze, interpret, and extract value from data is at an all-time high. If you're wondering what qualifications are needed to become a successful data scientist, how DataCouncil can help you get there, and why a data science course in Pune is a great option, this blog has the answers.
The Key Qualifications for a Data Scientist
To succeed as a data scientist, a mix of technical skills, education, and hands-on experience is essential. Here are the core qualifications required:
1. Educational Background
A strong foundation in mathematics, statistics, or computer science is typically expected. Most data scientists hold at least a bachelor’s degree in one of these fields, with many pursuing higher education such as a master's or a Ph.D. A data science course in Pune with DataCouncil can bridge this gap, offering the academic and practical knowledge required for a strong start in the industry.
2. Proficiency in Programming Languages
Programming is at the heart of data science. You need to be comfortable with languages like Python, R, and SQL, which are widely used for data analysis, machine learning, and database management. A comprehensive data science course in Pune will teach these programming skills from scratch, ensuring you become proficient in coding for data science tasks.
3. Understanding of Machine Learning
Data scientists must have a solid grasp of machine learning techniques and algorithms such as regression, clustering, and decision trees. By enrolling in a DataCouncil course, you'll learn how to implement machine learning models to analyze data and make predictions, an essential qualification for landing a data science job.
4. Data Wrangling Skills
Raw data is often messy and unstructured, and a good data scientist needs to be adept at cleaning and processing data before it can be analyzed. DataCouncil's data science course in Pune includes practical training in tools like Pandas and Numpy for effective data wrangling, helping you develop a strong skill set in this critical area.
5. Statistical Knowledge
Statistical analysis forms the backbone of data science. Knowledge of probability, hypothesis testing, and statistical modeling allows data scientists to draw meaningful insights from data. A structured data science course in Pune offers the theoretical and practical aspects of statistics required to excel.
6. Communication and Data Visualization Skills
Being able to explain your findings in a clear and concise manner is crucial. Data scientists often need to communicate with non-technical stakeholders, making tools like Tableau, Power BI, and Matplotlib essential for creating insightful visualizations. DataCouncil’s data science course in Pune includes modules on data visualization, which can help you present data in a way that’s easy to understand.
7. Domain Knowledge
Apart from technical skills, understanding the industry you work in is a major asset. Whether it’s healthcare, finance, or e-commerce, knowing how data applies within your industry will set you apart from the competition. DataCouncil's data science course in Pune is designed to offer case studies from multiple industries, helping students gain domain-specific insights.
Why Choose DataCouncil for a Data Science Course in Pune?
If you're looking to build a successful career as a data scientist, enrolling in a data science course in Pune with DataCouncil can be your first step toward reaching your goals. Here’s why DataCouncil is the ideal choice:
Comprehensive Curriculum: The course covers everything from the basics of data science to advanced machine learning techniques.
Hands-On Projects: You'll work on real-world projects that mimic the challenges faced by data scientists in various industries.
Experienced Faculty: Learn from industry professionals who have years of experience in data science and analytics.
100% Placement Support: DataCouncil provides job assistance to help you land a data science job in Pune or anywhere else, making it a great investment in your future.
Flexible Learning Options: With both weekday and weekend batches, DataCouncil ensures that you can learn at your own pace without compromising your current commitments.
Conclusion
Becoming a data scientist requires a combination of technical expertise, analytical skills, and industry knowledge. By enrolling in a data science course in Pune with DataCouncil, you can gain all the qualifications you need to thrive in this exciting field. Whether you're a fresher looking to start your career or a professional wanting to upskill, this course will equip you with the knowledge, skills, and practical experience to succeed as a data scientist.
Explore DataCouncil’s offerings today and take the first step toward unlocking a rewarding career in data science! Looking for the best data science course in Pune? DataCouncil offers comprehensive data science classes in Pune, designed to equip you with the skills to excel in this booming field. Our data science course in Pune covers everything from data analysis to machine learning, with competitive data science course fees in Pune. We provide job-oriented programs, making us the best institute for data science in Pune with placement support. Explore online data science training in Pune and take your career to new heights!
#In today's data-driven world#the role of a data scientist has become one of the most coveted career paths. With businesses relying on data for decision-making#understanding customer behavior#and improving products#the demand for skilled professionals who can analyze#interpret#and extract value from data is at an all-time high. If you're wondering what qualifications are needed to become a successful data scientis#how DataCouncil can help you get there#and why a data science course in Pune is a great option#this blog has the answers.#The Key Qualifications for a Data Scientist#To succeed as a data scientist#a mix of technical skills#education#and hands-on experience is essential. Here are the core qualifications required:#1. Educational Background#A strong foundation in mathematics#statistics#or computer science is typically expected. Most data scientists hold at least a bachelor’s degree in one of these fields#with many pursuing higher education such as a master's or a Ph.D. A data science course in Pune with DataCouncil can bridge this gap#offering the academic and practical knowledge required for a strong start in the industry.#2. Proficiency in Programming Languages#Programming is at the heart of data science. You need to be comfortable with languages like Python#R#and SQL#which are widely used for data analysis#machine learning#and database management. A comprehensive data science course in Pune will teach these programming skills from scratch#ensuring you become proficient in coding for data science tasks.#3. Understanding of Machine Learning
3 notes
·
View notes
Text
Why Predictive Maintenance Is the Key to Future-Proofing Your Operations
The capacity to foresee and avoid equipment breakdowns is not just a competitive advantage but also a need in today's fast-paced industrial scene. PdM solutions are becoming a vital tactic for businesses looking to ensure their operations are future-proof. Businesses may anticipate equipment failure and take preventative measures to minimize costly downtime, prolong equipment life, and maximize operational efficiency by utilizing cutting-edge technology and data analytics.
The Evolution of Maintenance Strategies
Conventional maintenance approaches have generally been either proactive or reactive. Reactive maintenance, sometimes known as "run-to-failure," is the practice of repairing equipment after it malfunctions, which can result in unplanned downtime and possible safety hazards. Contrarily, preventive maintenance plans routine maintenance activities independent of the equipment's state, which occasionally leads to needless effort and additional expenses.
By utilizing real-time data and sophisticated algorithms to anticipate equipment breakdowns before they occur, predictive maintenance provides a more sophisticated method. This approach optimizes resources and lowers total maintenance costs by preventing downtime and ensuring that maintenance is only done when necessary.
How Predictive Maintenance Works
Big data analytics, machine learning, and the Internet of Things (IoT) are some of the technologies that are essential to predictive maintenance. Large volumes of data, such as temperature, vibration, pressure, and other performance indicators, are gathered by IoT devices from equipment. After that, machine learning algorithms are used to examine this data to find trends and anticipate future problems.
Benefits of Predictive Maintenance:
Decreased Downtime: Reducing unscheduled downtime is one of predictive maintenance's most important benefits. Businesses can minimize operational disturbance by scheduling maintenance during off-peak hours by anticipating when equipment is likely to break.
Cost savings: By lowering the expenses of emergency repairs and equipment replacements, predictive maintenance helps save money. It also cuts labor expenses by avoiding unneeded maintenance operations.
Increased Equipment Life: Businesses may minimize the frequency of replacements and prolong the life of their gear by routinely checking the operation of their equipment and performing maintenance only when necessary.
Increased Safety: By averting major equipment breakdowns that can endanger employees, predictive maintenance can also increase workplace safety.
Optimal Resource Allocation: Businesses may maximize their use of resources, including manpower and spare parts, by concentrating maintenance efforts on machinery that requires them.
Predictive Maintenance's Future
Predictive maintenance is becoming more widely available and reasonably priced for businesses of all sizes as technology develops. Predictive models will be further improved by the combination of artificial intelligence (AI) and machine learning, increasing their accuracy and dependability.
Predictive maintenance will likely become a more autonomous process in the future, with AI-driven systems scheduling and carrying out maintenance chores in addition to predicting faults. Businesses will be able to function with never-before-seen dependability and efficiency because of this degree of automation.
Conclusion:
PdM Solutions has evolved from an abstract concept to a workable solution that is revolutionizing the way companies run their operations. Businesses may future-proof their operations by using predictive maintenance, which will help them stay ahead of any problems, cut expenses, and keep a competitive advantage in the market. Those who embrace predictive maintenance will be well-positioned to prosper in the future as the industrial landscape continues to change.
#pdm solutions#predicting buying behavior using machine learning python#predicting energy consumption#predicting energy consumption using machine learning#leak detection software#smart manufacturing solutions
0 notes
Text
Challenges and Limitations for Leak Detection Software
Technology is continuously changing, and we need to follow the changes to be part of the market. One of these is leak detection software, which companies use for financial gain. While leak detection software provides important advantages and breakthroughs in locating and containing leaks, it is important to be aware of the difficulties and constraints associated with its use. Here are a few typical issues and restrictions with leak detection software:
Let's read it out:
Complexity and Integration
Leak detection software implementation can be difficult and time-consuming on current infrastructure. It could be necessary to have specific knowledge to integrate the program with various sensor technologies, control systems, and data processing platforms.
Costs
The cost of acquiring, implementing, and maintaining high-quality leak detection software can be high. The initial cost may be a major deterrent to adoption for some companies or governments with tight budgets.
Sensor Limitations
The precision and dependability of the sensors have a significant impact on how well the leak detection program works. Different kinds of sensors have certain restrictions, such as their sensitivity to environmental factors or particular kinds of leakage.
False Positives and False Negatives
Systems for finding leaks may produce false alarms (false positives) or not find any leaks at all (false negatives). Unneeded shutdowns and investigations might result from false alarms, while significant damage and safety issues can be brought about by leaks that go unnoticed.
Flexibility in a Variety of Environments
Different sectors and settings have distinctive qualities that might have an impact on how well leak detection software works. The software's capacity to adjust to various conditions and leak scenarios may differ.
Human Expertise and Training
To properly use and comprehend the data produced by leak detection software, operators and maintenance employees must get the necessary training. The system's potential might not be completely realized without adequate training.
Geographical Difficulties
It might be logistically difficult to find leaks in specific areas of large and remote infrastructure networks or pipelines. It may take a long time and be expensive to reach remote places for maintenance and inspection.
Detection Limits and Reaction Time
It's critical to establish proper detection levels to reduce false alarms and guarantee the prompt identification of real breaches. Finding the ideal balance may be difficult.
Compatibility with Legacy Infrastructure
In other instances, outdated infrastructure might not work with cutting-edge leak detection systems, necessitating further improvements or retrofits.
Reliability and System Performance
Software for leak detection must be reliable, especially in crucial applications. Building faith in the system's capabilities requires ensuring that it operates consistently and properly over time.
Conclusion
Although leak detection software significantly improves the ability to locate and stop leaks, it is not without its difficulties and restrictions. When choosing and putting leak detection systems in place, organizations must carefully take these elements into account. The effectiveness of leak detection software may be further enhanced by addressing these issues through continued research, improvements in sensor technology, and increased data analytics capabilities, eventually assisting businesses and municipalities in protecting their assets and the environment.
#leak detection software#smart manufacturing solutions#predicting energy consumption#pdm solution#predicting energy consumption using machine learning#predicting buying behavior using machine learning python#energy consumption#detection software#machine learning python#machine learning
0 notes
Text
#leak detection software#predicting buying behavior using machine learning python#predicting energy consumption#predicting energy consumption using machine learning#smart manufacturing solutions#leak detection software israel#predicting energy consumption israel#pdm solutions
0 notes
Text
Honestly, I think we need to talk more often about the exotic pet trade. It’s such a threat to wild populations of animals all over the world. Anytime i see a post on here that’s like “look at this cute animal you probably haven’t heard of!” I weep over the truth that the more people hear about it, the more they will poach it from the land.
And I ask myself…why are people doing this? Why are we trying to take these often endangered animals from their habitat even as we know it won’t help them?
And I think to my own self. When I was 19, working in an exotic pet store, having just left a childhood full of times in the woods and farm animals. I came to a city that felt like it had none of that. The little glass boxes felt like encapsulations of the nature I had grown up around and sorely come to miss. If I couldn’t have it outside, I thought, I would have it inside. My coworkers and I all talked and watched videos about building little ecosystems for our little pets. It felt exciting. It felt connecting.
But the issue is that these aren’t ecosystems. They are often perpetual death machines of capture, and they often lead to disruption of the native ecosystems you’re actually yearning for. Hell, look at Florida. Hundreds of invasive animals, including everything from great large pythons to little isopods, all brought in through the pet trade. Look at Australia. Species decimated by poaching. Read about all the little monkeys of the world, and what we have done by owning them.
There are entire industries wrapped up in the poaching of wild animals, the breeding of them, the housing of them, the feeding of them. Even some of the most well respected and supposedly ethical people in the trade still think there are different ethics for breeders and normal keepers. They store their animals in minimalistic tiny enclosures. Thousands upon thousands of rats and crickets are bred just to maintain the feed supply to the trade and its customers, creating an endless wheel of suffering and disease conditions. And I must ask, why? For what purpose? It’s not conservation. These aren’t concerned groups creating sanctuary populations for wildlife reintroduction. They aren’t growing things for food, or leather, or research. All of those we can discuss the ethics of, sure, but at least then there is a reason. What is the reason here? Just to have a hollow stare at a sad creature and pretend that is love? Just to propagate more environmental destruction with our ongoing thievery of peat moss and orchids for our tanks?
And still then are the collectors. I’ve met people with hundreds of animals placed in shoe storage racks, living their lives for no purpose other than the occasional glance and the feeling of having a hoard.
We all feel so disconnected from the environment that we are willing to rob other areas of the world, we are willing to further endanger these species we love, we are willing to terrorize the ecosystems we are unknowingly in, all due to our incredible and violent loneliness.
But this is a solvable problem.
Learn to live where you are. Learn to love the things you can find around you. Plant native plants, you will see native birds and wildlife. If you don’t have a yard, offer to care for the yard of an older neighbor, if they will let you use native plant landscaping in exchange. If you truly cannot connect where you are, to its people and to its ecosystems, then research somewhere and move. But don’t live your life with one foot out the door, hating where you are and doing nothing, dreaming or elsewhere and never leaving.
You do not need to collect rare morphs of isopods. My friend, I see so many beautiful native ones outside in the habitat I have helped manage for them. I see swallowtail butterflies and chimney swifts and solitary bees and little snakes and salamanders and anoles. I learn their species names and watch their behaviors. I see what they eat, where they hide, what times of year they are around. And I feel, finally, connected. Everything I was looking for in the commodity of exotic pets, I found in the reality just outside my door. I have nurtured it and it has nurtured me.
Owning that cute monkey won’t fix you. But having a relationship with the ecology around you just might begin to.
#rose baker#text post#ecology#environment#enviromentalism#ecosystems#pets#pet trade#exotic pets#conservation#native plants
19 notes
·
View notes
Text
Short-Term vs. Long-Term Data Analytics Course in Delhi: Which One to Choose?
In today’s digital world, data is everywhere. From small businesses to large organizations, everyone uses data to make better decisions. Data analytics helps in understanding and using this data effectively. If you are interested in learning data analytics, you might wonder whether to choose a short-term or a long-term course. Both options have their benefits, and your choice depends on your goals, time, and career plans.
At Uncodemy, we offer both short-term and long-term data analytics courses in Delhi. This article will help you understand the key differences between these courses and guide you to make the right choice.
What is Data Analytics?
Data analytics is the process of examining large sets of data to find patterns, insights, and trends. It involves collecting, cleaning, analyzing, and interpreting data. Companies use data analytics to improve their services, understand customer behavior, and increase efficiency.
There are four main types of data analytics:
Descriptive Analytics: Understanding what has happened in the past.
Diagnostic Analytics: Identifying why something happened.
Predictive Analytics: Forecasting future outcomes.
Prescriptive Analytics: Suggesting actions to achieve desired outcomes.
Short-Term Data Analytics Course
A short-term data analytics course is a fast-paced program designed to teach you essential skills quickly. These courses usually last from a few weeks to a few months.
Benefits of a Short-Term Data Analytics Course
Quick Learning: You can learn the basics of data analytics in a short time.
Cost-Effective: Short-term courses are usually more affordable.
Skill Upgrade: Ideal for professionals looking to add new skills without a long commitment.
Job-Ready: Get practical knowledge and start working in less time.
Who Should Choose a Short-Term Course?
Working Professionals: If you want to upskill without leaving your job.
Students: If you want to add data analytics to your resume quickly.
Career Switchers: If you want to explore data analytics before committing to a long-term course.
What You Will Learn in a Short-Term Course
Introduction to Data Analytics
Basic Tools (Excel, SQL, Python)
Data Visualization (Tableau, Power BI)
Basic Statistics and Data Interpretation
Hands-on Projects
Long-Term Data Analytics Course
A long-term data analytics course is a comprehensive program that provides in-depth knowledge. These courses usually last from six months to two years.
Benefits of a Long-Term Data Analytics Course
Deep Knowledge: Covers advanced topics and techniques in detail.
Better Job Opportunities: Preferred by employers for specialized roles.
Practical Experience: Includes internships and real-world projects.
Certifications: You may earn industry-recognized certifications.
Who Should Choose a Long-Term Course?
Beginners: If you want to start a career in data analytics from scratch.
Career Changers: If you want to switch to a data analytics career.
Serious Learners: If you want advanced knowledge and long-term career growth.
What You Will Learn in a Long-Term Course
Advanced Data Analytics Techniques
Machine Learning and AI
Big Data Tools (Hadoop, Spark)
Data Ethics and Governance
Capstone Projects and Internships
Key Differences Between Short-Term and Long-Term Courses
FeatureShort-Term CourseLong-Term CourseDurationWeeks to a few monthsSix months to two yearsDepth of KnowledgeBasic and Intermediate ConceptsAdvanced and Specialized ConceptsCostMore AffordableHigher InvestmentLearning StyleFast-PacedDetailed and ComprehensiveCareer ImpactQuick Entry-Level JobsBetter Career Growth and High-Level JobsCertificationBasic CertificateIndustry-Recognized CertificationsPractical ProjectsLimitedExtensive and Real-World Projects
How to Choose the Right Course for You
When deciding between a short-term and long-term data analytics course at Uncodemy, consider these factors:
Your Career Goals
If you want a quick job or basic knowledge, choose a short-term course.
If you want a long-term career in data analytics, choose a long-term course.
Time Commitment
Choose a short-term course if you have limited time.
Choose a long-term course if you can dedicate several months to learning.
Budget
Short-term courses are usually more affordable.
Long-term courses require a bigger investment but offer better returns.
Current Knowledge
If you already know some basics, a short-term course will enhance your skills.
If you are a beginner, a long-term course will provide a solid foundation.
Job Market
Short-term courses can help you get entry-level jobs quickly.
Long-term courses open doors to advanced and specialized roles.
Why Choose Uncodemy for Data Analytics Courses in Delhi?
At Uncodemy, we provide top-quality training in data analytics. Our courses are designed by industry experts to meet the latest market demands. Here’s why you should choose us:
Experienced Trainers: Learn from professionals with real-world experience.
Practical Learning: Hands-on projects and case studies.
Flexible Schedule: Choose classes that fit your timing.
Placement Assistance: We help you find the right job after course completion.
Certification: Receive a recognized certificate to boost your career.
Final Thoughts
Choosing between a short-term and long-term data analytics course depends on your goals, time, and budget. If you want quick skills and job readiness, a short-term course is ideal. If you seek in-depth knowledge and long-term career growth, a long-term course is the better choice.
At Uncodemy, we offer both options to meet your needs. Start your journey in data analytics today and open the door to exciting career opportunities. Visit our website or contact us to learn more about our Data Analytics course in delhi.
Your future in data analytics starts here with Uncodemy!
2 notes
·
View notes
Text
Object-Oriented Programming (OOP) Explaine
Object-Oriented Programming (OOP) is a programming paradigm based on the concept of "objects," which represent real-world entities. Objects combine data (attributes) and functions (methods) into a single unit. OOP promotes code reusability, modularity, and scalability, making it a popular approach in modern software development.
Core Concepts of Object-Oriented Programming
Classes and Objects
Class: A blueprint or template for creating objects. It defines properties (attributes) and behaviors (methods).
Object: An instance of a class. Each object has unique data but follows the structure defined by its
Encapsulations
Encapsulation means bundling data (attributes) and methods that operate on that data within a class. It protects object properties by restricting direct access.
Access to attributes is controlled through getter and setter methods.Example: pythonCopyEditclass Person: def __init__(self, name): self.__name = name # Private attribute def get_name(self): return self.__name person = Person("Alice") print(person.get_name()) # Output: Alice
Inheritance
Inheritance allows a class (child) to inherit properties and methods from another class (parent). It promotes code reuse and hierarchical relationships.Example: pythonCopyEditclass Animal: def speak(self): print("Animal speaks") class Dog(Animal): def speak(self): print("Dog barks") dog = Dog() dog.speak() # Output: Dog barks
Polymorphism
Polymorphism allows methods to have multiple forms. It enables the same function to work with different object types.
Two common types:
Method Overriding (child class redefines parent method).
Method Overloading (same method name, different parameters – not natively supported in Python).Example: pythonCopyEditclass Bird: def sound(self): print("Bird chirps") class Cat: def sound(self): print("Cat meows") def make_sound(animal): animal.sound() make_sound(Bird()) # Output: Bird chirps make_sound(Cat()) # Output: Cat meows
Abstraction
Abstraction hides complex implementation details and shows only the essential features.
In Python, this is achieved using abstract classes and methods (via the abc module).Example: pythonCopyEditfrom abc import ABC, abstractmethod class Shape(ABC): @abstractmethod def area(self): pass class Circle(Shape): def __init__(self, radius): self.radius = radius def area(self): return 3.14 * self.radius * self.radius circle = Circle(5) print(circle.area()) # Output: 78.5
Advantages of Object-Oriented Programming
Code Reusability: Use inheritance to reduce code duplication.
Modularity: Organize code into separate classes, improving readability and maintenance.
Scalability: Easily extend and modify programs as they grow.
Data Security: Protect sensitive data using encapsulation.
Flexibility: Use polymorphism for adaptable and reusable methods.
Real-World Applications of OOP
Software Development: Used in large-scale applications like operating systems, web frameworks, and databases.
Game Development: Objects represent game entities like characters and environments.
Banking Systems: Manage customer accounts, transactions, and security.
E-commerce Platforms: Handle products, users, and payment processing.
Machine Learning: Implement models as objects for efficient training and prediction.
Conclusion
Object-Oriented Programming is a powerful paradigm that enhances software design by using objects, encapsulation, inheritance, polymorphism, and abstraction. It is widely used in various industries to build scalable, maintainable, and efficient applications. Understanding and applying OOP principles is essential for modern software development.
: pythonCopyEdit
class Car: def __init__(self, brand, model): self.brand = brand self.model = model def display_info(self): print(f"Car: {self.brand} {self.model}") my_car = Car("Toyota", "Camry") my_car.display_info() # Output: Car: Toyota Camry
Encapsulation
2 notes
·
View notes
Text
Why Tableau is Essential in Data Science: Transforming Raw Data into Insights
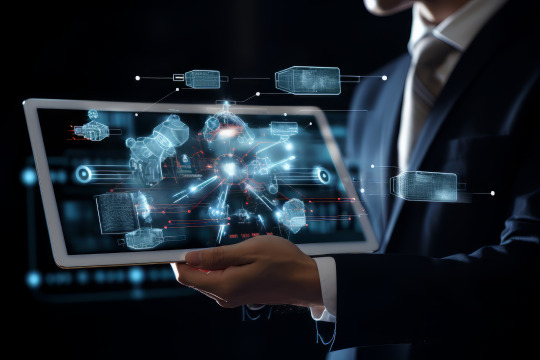
Data science is all about turning raw data into valuable insights. But numbers and statistics alone don’t tell the full story—they need to be visualized to make sense. That’s where Tableau comes in.
Tableau is a powerful tool that helps data scientists, analysts, and businesses see and understand data better. It simplifies complex datasets, making them interactive and easy to interpret. But with so many tools available, why is Tableau a must-have for data science? Let’s explore.
1. The Importance of Data Visualization in Data Science
Imagine you’re working with millions of data points from customer purchases, social media interactions, or financial transactions. Analyzing raw numbers manually would be overwhelming.
That’s why visualization is crucial in data science:
Identifies trends and patterns – Instead of sifting through spreadsheets, you can quickly spot trends in a visual format.
Makes complex data understandable – Graphs, heatmaps, and dashboards simplify the interpretation of large datasets.
Enhances decision-making – Stakeholders can easily grasp insights and make data-driven decisions faster.
Saves time and effort – Instead of writing lengthy reports, an interactive dashboard tells the story in seconds.
Without tools like Tableau, data science would be limited to experts who can code and run statistical models. With Tableau, insights become accessible to everyone—from data scientists to business executives.
2. Why Tableau Stands Out in Data Science
A. User-Friendly and Requires No Coding
One of the biggest advantages of Tableau is its drag-and-drop interface. Unlike Python or R, which require programming skills, Tableau allows users to create visualizations without writing a single line of code.
Even if you’re a beginner, you can:
✅ Upload data from multiple sources
✅ Create interactive dashboards in minutes
✅ Share insights with teams easily
This no-code approach makes Tableau ideal for both technical and non-technical professionals in data science.
B. Handles Large Datasets Efficiently
Data scientists often work with massive datasets—whether it’s financial transactions, customer behavior, or healthcare records. Traditional tools like Excel struggle with large volumes of data.
Tableau, on the other hand:
Can process millions of rows without slowing down
Optimizes performance using advanced data engine technology
Supports real-time data streaming for up-to-date analysis
This makes it a go-to tool for businesses that need fast, data-driven insights.
C. Connects with Multiple Data Sources
A major challenge in data science is bringing together data from different platforms. Tableau seamlessly integrates with a variety of sources, including:
Databases: MySQL, PostgreSQL, Microsoft SQL Server
Cloud platforms: AWS, Google BigQuery, Snowflake
Spreadsheets and APIs: Excel, Google Sheets, web-based data sources
This flexibility allows data scientists to combine datasets from multiple sources without needing complex SQL queries or scripts.
D. Real-Time Data Analysis
Industries like finance, healthcare, and e-commerce rely on real-time data to make quick decisions. Tableau’s live data connection allows users to:
Track stock market trends as they happen
Monitor website traffic and customer interactions in real time
Detect fraudulent transactions instantly
Instead of waiting for reports to be generated manually, Tableau delivers insights as events unfold.
E. Advanced Analytics Without Complexity
While Tableau is known for its visualizations, it also supports advanced analytics. You can:
Forecast trends based on historical data
Perform clustering and segmentation to identify patterns
Integrate with Python and R for machine learning and predictive modeling
This means data scientists can combine deep analytics with intuitive visualization, making Tableau a versatile tool.
3. How Tableau Helps Data Scientists in Real Life
Tableau has been adopted by the majority of industries to make data science more impactful and accessible. This is applied in the following real-life scenarios:
A. Analytics for Health Care
Tableau is deployed by hospitals and research institutions for the following purposes:
Monitor patient recovery rates and predict outbreaks of diseases
Analyze hospital occupancy and resource allocation
Identify trends in patient demographics and treatment results
B. Finance and Banking
Banks and investment firms rely on Tableau for the following purposes:
✅ Detect fraud by analyzing transaction patterns
✅ Track stock market fluctuations and make informed investment decisions
✅ Assess credit risk and loan performance
C. Marketing and Customer Insights
Companies use Tableau to:
✅ Track customer buying behavior and personalize recommendations
✅ Analyze social media engagement and campaign effectiveness
✅ Optimize ad spend by identifying high-performing channels
D. Retail and Supply Chain Management
Retailers leverage Tableau to:
✅ Forecast product demand and adjust inventory levels
✅ Identify regional sales trends and adjust marketing strategies
✅ Optimize supply chain logistics and reduce delivery delays
These applications show why Tableau is a must-have for data-driven decision-making.
4. Tableau vs. Other Data Visualization Tools
There are many visualization tools available, but Tableau consistently ranks as one of the best. Here’s why:
Tableau vs. Excel – Excel struggles with big data and lacks interactivity; Tableau handles large datasets effortlessly.
Tableau vs. Power BI – Power BI is great for Microsoft users, but Tableau offers more flexibility across different data sources.
Tableau vs. Python (Matplotlib, Seaborn) – Python libraries require coding skills, while Tableau simplifies visualization for all users.
This makes Tableau the go-to tool for both beginners and experienced professionals in data science.
5. Conclusion
Tableau has become an essential tool in data science because it simplifies data visualization, handles large datasets, and integrates seamlessly with various data sources. It enables professionals to analyze, interpret, and present data interactively, making insights accessible to everyone—from data scientists to business leaders.
If you’re looking to build a strong foundation in data science, learning Tableau is a smart career move. Many data science courses now include Tableau as a key skill, as companies increasingly demand professionals who can transform raw data into meaningful insights.
In a world where data is the driving force behind decision-making, Tableau ensures that the insights you uncover are not just accurate—but also clear, impactful, and easy to act upon.
#data science course#top data science course online#top data science institute online#artificial intelligence course#deepseek#tableau
3 notes
·
View notes
Text
$AIGRAM - your AI assistant for Telegram data
Introduction
$AIGRAM is an AI-powered platform designed to help users discover and organize Telegram channels and groups more effectively. By leveraging advanced technologies such as natural language processing, semantic search, and machine learning, AIGRAM enhances the way users explore content on Telegram.
With deep learning algorithms, AIGRAM processes large amounts of data to deliver precise and relevant search results, making it easier to find the right communities. The platform seamlessly integrates with Telegram, supporting better connections and collaboration. Built with scalability in mind, AIGRAM is cloud-based and API-driven, offering a reliable and efficient tool to optimize your Telegram experience.
Tech Stack
AIGRAM uses a combination of advanced AI, scalable infrastructure, and modern tools to deliver its Telegram search and filtering features.
AI & Machine Learning:
NLP: Transformer models like BERT, GPT for understanding queries and content. Machine Learning: Algorithms for user behavior and query optimization. Embeddings: Contextual vectorization (word2vec, FAISS) for semantic search. Recommendation System: AI-driven suggestions for channels and groups.
Backend:
Languages: Python (AI models), Node.js (API). Databases: PostgreSQL, Elasticsearch (search), Redis (caching). API Frameworks: FastAPI, Express.js.
Frontend:
Frameworks: React.js, Material-UI, Redux for state management.
This tech stack powers AIGRAM’s high-performance, secure, and scalable platform.
Mission
AIGRAM’s mission is to simplify the trading experience for memecoin traders on the Solana blockchain. Using advanced AI technologies, AIGRAM helps traders easily discover, filter, and engage with the most relevant Telegram groups and channels.
With the speed of Solana and powerful search features, AIGRAM ensures traders stay ahead in the fast-paced memecoin market. Our platform saves time, provides clarity, and turns complex information into valuable insights.
We aim to be the go-to tool for Solana traders, helping them make better decisions and maximize their success.
Our socials:
Website - https://aigram.software/ Gitbook - https://aigram-1.gitbook.io/ X - https://x.com/aigram_software Dex - https://dexscreener.com/solana/baydg5htursvpw2y2n1pfrivoq9rwzjjptw9w61nm25u
2 notes
·
View notes
Text
Diagsense revolutionizing manufacturing unveils Cutting-Edge Smart Manufacturing Solutions
#leak detection software#predicting energy consumption#smart manufacturing solutions#pdm solutions#predicting energy consumption using machine learning#predicting buying behavior using machine learning python
0 notes
Text
How Israel’s Smart Manufacturing Solutions are Shaping the Future of Global Industry in 2024
Introduction:
Israel is rapidly emerging as a leader in smart manufacturing solutions Israel, leveraging advanced technologies to drive efficiency, innovation, and sustainability in production processes. From robotics and IoT integration to AI-driven analytics and cybersecurity, Israeli companies are developing cutting-edge solutions that are transforming industries worldwide. As global manufacturing faces challenges like supply chain resilience, energy efficiency, and labor shortages, Israel’s tech-driven approach provides valuable answers. This blog explores the latest advancements in Israel’s smart manufacturing sector, highlighting how these innovations are setting new standards for industrial excellence and shaping the future of global manufacturing in 2024 and beyond.
IoT-Enabled Smart Factories
Israeli companies are leading the way in IoT integration, where connected devices and sensors enable real-time monitoring of production processes. This smart infrastructure helps manufacturers track machine health, identify bottlenecks, and optimize energy use. Israeli firms like Augury provide IoT-based solutions for predictive maintenance, helping companies prevent equipment failures, reduce downtime, and extend machine lifespan. With IoT-enabled factories, businesses can achieve a higher level of operational efficiency and reduce costs.
AI and Machine Learning for Process Optimization
Artificial intelligence is at the core of Israel’s smart manufacturing innovations. By using machine learning algorithms, manufacturers can analyze vast amounts of data to improve quality control, optimize production speeds, and reduce waste. Companies like Diagsense offer AI-driven platforms that provide real-time insights, allowing manufacturers to adjust operations swiftly. These predictive analytics systems are crucial for industries aiming to minimize waste and maximize productivity, enabling smarter, data-driven decisions.
Advanced Robotics for Precision and Flexibility
Robotics has transformed manufacturing in Israel, with smart robotic solutions that enhance both precision and flexibility on the production line. Israeli robotics firms, such as Roboteam and Elbit Systems, develop robots that assist with high-precision tasks, making production more agile. These robots adapt to varying tasks, which is particularly valuable for industries like electronics and automotive manufacturing, where precision and customization are crucial.
Cybersecurity for Manufacturing
As manufacturing becomes more digital, cybersecurity is a pressing need. Israel, known for its cybersecurity expertise, applies these strengths to secure manufacturing systems against cyber threats. Solutions from companies like Claroty and SCADAfence protect IoT devices and critical infrastructure in factories, ensuring data integrity and operational continuity. This focus on cybersecurity helps manufacturers defend against costly cyberattacks, safeguard intellectual property, and maintain secure operations.
Sustainable and Energy-Efficient Solutions
Sustainability is a growing focus in Israeli smart manufacturing, with innovations designed to reduce resource consumption and emissions. Companies such as ECOncrete are developing environmentally friendly materials and processes, supporting the global push for greener industries. From energy-efficient machinery to sustainable building materials, these innovations align with global efforts to reduce environmental impact, making manufacturing both profitable and responsible.
Conclusion
Israel’s smart manufacturing solutions Israel are redefining the global industrial landscape, setting benchmarks in efficiency, precision, and sustainability. By embracing IoT, AI, robotics, cybersecurity, and eco-friendly practices, Israeli innovators are helping industries worldwide become more resilient and adaptive to changing market demands. These advancements equip manufacturers with the tools they need to operate efficiently while minimizing environmental impact. For organizations looking to integrate these smart technologies, the expertise of partners like Diagsense can provide invaluable insights and tailored solutions, making it easier to navigate the smart manufacturing revolution and ensure long-term success in a competitive global market.
#pdm solutions#leak detection software#predicting energy#smart manufacturing solutions#predicting buying behavior using machine learning python#predicting energy consumption
0 notes
Text
Explaining Complex Models to Business Stakeholders: Understanding LightGBM
As machine learning models like LightGBM become more accurate and efficient, they also tend to grow in complexity, making them harder to interpret for business stakeholders. This challenge arises as these advanced models, often referred to as "black-box" models, provide superior performance but lack transparency in their decision-making processes. This lack of interpretability can hinder model adoption rates, impede the evaluation of feature impacts, complicate hyper-parameter tuning, raise fairness concerns, and make it difficult to identify potential vulnerabilities within the model.
To explain a LightGBM (Light Gradient Boosting Machine) model, it's essential to understand that LightGBM is a gradient boosting ensemble method based on decision trees. It is optimized for high performance with distributed systems and can be used for both classification and regression tasks. LightGBM creates decision trees that grow leaf-wise, meaning that only a single leaf is split based on the gain. This approach can sometimes lead to overfitting, especially with smaller datasets. To prevent overfitting, limiting the tree depth is recommended.
One of the key features of LightGBM is its histogram-based method, where data is bucketed into bins using a histogram of the distribution. Instead of each data point, these bins are used to iterate, calculate the gain, and split the data. This method is efficient for sparse datasets. LightGBM also employs exclusive feature bundling to reduce dimensionality, making the algorithm faster and more efficient.
LightGBM uses Gradient-based One Side Sampling (GOSS) for dataset sampling. GOSS assigns higher weights to data points with larger gradients when calculating the gain, ensuring that instances contributing more to training are prioritized. Data points with smaller gradients are randomly removed, while some are retained to maintain accuracy. This sampling method is generally more effective than random sampling at the same rate.
As machine learning models like LightGBM become more accurate and efficient, they also tend to grow in complexity, making them harder to interpret for business stakeholders. This challenge arises as these advanced models, often referred to as "black-box" models, provide superior performance but lack transparency in their decision-making processes. This lack of interpretability can hinder model adoption rates, impede the evaluation of feature impacts, complicate hyper-parameter tuning, raise fairness concerns, and make it difficult to identify potential vulnerabilities within the model.
Global and Local Explainability:
LightGBM, a tree-based boosting model, is known for its precision in delivering outcomes. However, its complexity can present challenges in understanding the inner workings of the model. To address this issue, it is crucial to focus on two key aspects of model explainability: global and local explainability.
- Global Explainability: Global explainability refers to understanding the overall behavior of the model and how different features contribute to its predictions. Techniques like feature importance analysis can help stakeholders grasp which features are most influential in the model's decision-making process.
- Local Explainability: Local explainability involves understanding how the model arrives at specific predictions for individual data points. Methods like SHAP (SHapley Additive exPlanations) can provide insights into the contribution of each feature to a particular prediction, enhancing the interpretability of the model at a granular level.
Python Code Snippet for Model Explainability:
To demonstrate the explainability of a LightGBM model using Python, we can utilize the SHAP library to generate local explanations for individual predictions. Below is a sample code snippet showcasing how SHAP can be applied to interpret the predictions of a LightGBM model:
```python
# Import necessary libraries
import shap
import lightgbm as lgb
# Load the LightGBM model
model = lgb.Booster(model_file='model.txt') # Load the model from a file
# Load the dataset for which you want to explain predictions
data = ...
# Initialize the SHAP explainer with the LightGBM model
explainer = shap.TreeExplainer(model)
# Generate SHAP values for a specific data point
shap_values = explainer.shap_values(data)
# Visualize the SHAP values
shap.initjs()
shap.force_plot(explainer.expected_value, shap_values[0], data) ,,,
In this code snippet, we first load the LightGBM model and the dataset for which we want to explain predictions. We then initialize a SHAP explainer with the model and generate SHAP values for a specific data point. Finally, we visualize the SHAP values using a force plot to provide a clear understanding of how each feature contributes to the model's prediction for that data point.
Examples of Using LightGBM in Industries
LightGBM, with its high performance and efficiency, finds applications across various industries, providing accurate predictions and valuable insights. Here are some examples of how LightGBM is utilized in different sectors:
1. Finance Industry:
- Credit Scoring: LightGBM is commonly used for credit scoring models in the finance sector. By analyzing historical data and customer behavior, financial institutions can assess creditworthiness and make informed lending decisions.
- Risk Management: LightGBM helps in identifying and mitigating risks by analyzing market trends, customer data, and other relevant factors to predict potential risks and optimize risk management strategies.
2. Healthcare Industry:
- Disease Diagnosis: LightGBM can be employed for disease diagnosis and prognosis prediction based on patient data, medical history, and diagnostic tests. It aids healthcare professionals in making accurate and timely decisions for patient care.
- Drug Discovery: In pharmaceutical research, LightGBM can analyze molecular data, drug interactions, and biological pathways to accelerate drug discovery processes and identify potential candidates for further testing.
3. E-commerce and Retail:
- Recommendation Systems: LightGBM powers recommendation engines in e-commerce platforms by analyzing user behavior, purchase history, and product preferences to provide personalized recommendations, enhancing user experience and increasing sales.
- Inventory Management: By forecasting demand, optimizing pricing strategies, and managing inventory levels efficiently, LightGBM helps e-commerce and retail businesses reduce costs, minimize stockouts, and improve overall operational efficiency.
4. Manufacturing and Supply Chain:
- Predictive Maintenance: LightGBM can predict equipment failures and maintenance needs in manufacturing plants by analyzing sensor data, production metrics, and historical maintenance records, enabling proactive maintenance scheduling and minimizing downtime.
- Supply Chain Optimization: LightGBM assists in optimizing supply chain operations by forecasting demand, identifying bottlenecks, and streamlining logistics processes, leading to cost savings and improved supply chain efficiency.
5. Marketing and Advertising:
- Customer Segmentation: LightGBM enables marketers to segment customers based on behavior, demographics, and preferences, allowing targeted marketing campaigns and personalized messaging to enhance customer engagement and retention.
- Click-Through Rate Prediction: In digital advertising, LightGBM is used to predict click-through rates for ad placements, optimize ad targeting, and maximize advertising ROI by showing relevant ads to the right audience.
These examples illustrate the versatility and effectiveness of LightGBM in addressing diverse challenges and driving value across industries. By leveraging its capabilities for predictive modeling, optimization, and decision-making, organizations can harness the power of LightGBM to gain a competitive edge and achieve business objectives efficiently.
By leveraging tools like SHAP, data scientists can enhance the explainability of complex models like LightGBM, enabling better communication with business stakeholders and fostering trust in the model's decision-making process.
In the era of advanced machine learning models, achieving model explainability is crucial for ensuring transparency, trust, and compliance with regulatory requirements. By employing techniques like SHAP and focusing on global and local explainability, data scientists can bridge the gap between complex models like LightGBM and business stakeholders, facilitating informed decision-making and fostering a deeper understanding of the model's inner workings.
In summary, LightGBM is a powerful machine learning algorithm that leverages gradient boosting and decision trees to achieve high performance and efficiency in both classification and regression tasks. Its unique features like leaf-wise tree growth, histogram-based data processing, exclusive feature bundling, and GOSS sampling contribute to its effectiveness in handling complex datasets and producing accurate predictions.
2 notes
·
View notes
Text
How PdM Solutions are Revolutionizing the Manufacturing Sector?
In this technological era, businesses are moving towards automation; one of them is PdM solutions. That is why, if you belong to the same industry, you must understand it. Predictive maintenance (PdM) technologies are revolutionizing the industrial industry by providing notable benefits over conventional maintenance methodologies. In this article, we will discuss how PdM solutions are revolutionizing manufacturing.
Let's read it out:
Minimizing Downtime and Production Losses
PdM solutions forecast when equipment is likely to fail by using machine learning algorithms and sensor data.
Manufacturers can minimize production delays by scheduling maintenance during scheduled downtime and diagnosing faults before they lead to failures.
Increasing the Life of Equipment
Proactive maintenance based on the real state of the machinery, as opposed to random scheduling, is made possible by PdM.
PdM solutions help expensive equipment last longer by preventing significant malfunctions and resolving problems early on, which lowers the need for pricey replacements.
Optimizing Maintenance Costs
Higher repair and replacement expenses are frequently the result of traditional reactive maintenance.
By enabling manufacturers to more effectively allocate resources and concentrate on equipment that requires repair, PdM lowers maintenance costs.
Improving the Consistency and Quality of Products
Unexpected equipment breakdowns might result in flaws in manufactured goods.
PdM lowers the possibility of defective manufacturing and makes sure that equipment is running at peak efficiency, which contributes to maintaining consistent quality.
Boosting Overall Operational Efficiency
PdM systems offer insightful data on the functionality and usage trends of equipment.
With the use of this data, manufacturers may streamline their manufacturing procedures, consume less energy, and allocate resources more wisely.
Summary
Maintenance solutions are transforming the manufacturing industry. by offering accurate decreases in downtime, increasing equipment longevity, maximizing maintenance costs, improving product quality, and increasing overall operational efficiency. Embracing PdM gives manufacturers a competitive edge by increasing output, cutting expenses, and releasing better products onto the market. PdM's influence on manufacturing is anticipated to increase with the advancement of technology, resulting in more sustainable and productive operations. If you are looking for PdM Solutions services, you can connect with us here. We have experienced staff to offer you the most comprehensive services. We are committed to making the business journey easy.
#leak detection software#predicting buying behavior using machine learning python#predicting energy consumption#predicting energy consumption using machine learning#smart manufacturing solutions
0 notes
Text
i'm working on a new security tool called dbe.
dbe is designed to simulate a cybersecurity scenario in which an agent learns to perform various actions in order to infect machines, perform self-healing, and propagate to other machines. The agent uses a Q-learning algorithm to learn which actions to take based on the current state of the environment.
The script takes a list of IP addresses as input and scans them to see if they are vulnerable to a specific exploit. If a vulnerable machine is found, the agent tries to infect it by connecting to a remote server and executing a payload. The agent also performs periodic self-healing actions to ensure that it is running smoothly, and propagates to other machines in order to spread the infection.
The script uses a Q-table to keep track of the expected rewards for each action in each state, and updates the Q-table based on the rewards received for each action taken. The agent also uses a decaying exploration probability to balance exploration and exploitation of the environment.
The script is written in Python and uses various libraries such as subprocess, threading, and numpy to perform its functions. It can be run from the command line with various options to customize its behavior.
In simpler terms, the script is like a game where the agent learns to take actions in order to achieve a goal (in this case, infecting machines and spreading the infection). The agent uses a special kind of learning algorithm called Q-learning to figure out which actions are the best to take in each situation. The script also includes some safety measures to make sure the agent doesn't cause any harm to itself or others.
https://github.com/geeknik/dbe
#open source#python#github#geeknik#writing#blog#tumblr#twitter#foss#machine learning#cybersecurity#infosec#malware
2 notes
·
View notes