#iot big data machine learning
Explore tagged Tumblr posts
Text
Big Data and the Internet of Things (IoT): The Power of Analytics
In today’s hyperconnected world, the intersection of the Internet of Things (IoT) and Big Data analytics is reshaping industries, providing businesses with unprecedented insights, and fueling a new wave of innovation. The vast amount of data generated by IoT devices offers immense opportunities to derive actionable insights. By leveraging IoT Big Data solutions, companies can optimize processes, enhance customer experiences, and drive business growth.

This article explores how IoT Big Data analytics, IoT Big Data architecture, and machine learning are transforming industries and providing valuable solutions.
The Explosion of IoT Data
The Internet of Things refers to the network of physical devices connected to the internet, gathering and sharing data. These devices include everything from smart home appliances and wearable health monitors to industrial sensors and autonomous vehicles. According to Statista, the number of IoT-connected devices is projected to reach 30.9 billion by 2025, generating a massive amount of data.
This data deluge presents significant challenges but also immense opportunities for organizations. By implementing IoT Big Data solutions, companies can collect, store, analyze, and act on this vast amount of information to improve decision-making, efficiency, and innovation.
IoT Big Data Analytics: Turning Data Into Insights
One of the most significant advantages of combining IoT with Big Data analytics is the ability to transform raw data into actionable insights. IoT Big Data analytics involves analyzing large volumes of data generated by IoT devices to identify patterns, trends, and anomalies that can inform business decisions.
Real-World Application: In the automotive industry, companies like Tesla use IoT sensors embedded in vehicles to monitor real-time data related to performance, maintenance needs, and driving patterns. This data is then processed through Big Data analytics to improve vehicle performance, anticipate maintenance issues, and even enhance autonomous driving features. Tesla’s ability to leverage IoT Big Data is a key factor in its innovative approach to automotive technology.
Moreover, GE Aviation uses IoT sensors in aircraft engines to monitor real-time performance data. By leveraging Big Data analytics, GE predicts engine failures and schedules proactive maintenance, improving safety and reducing downtime.
IoT Big Data Architecture: The Backbone of Data Processing
To efficiently process and analyze data from millions of IoT devices, businesses need a scalable and robust IoT Big Data architecture. This architecture typically includes:
Data Collection Layer: Sensors and devices collect and transmit data.
Data Ingestion Layer: Middleware solutions or platforms like Apache Kafka are used to ingest data in real-time, handling the large influx of information from various IoT sources.
Data Storage Layer: Data is stored in cloud-based or on-premise databases. Solutions like AWS IoT or Azure IoT are popular choices for storing and managing vast amounts of IoT data.
Data Processing and Analytics Layer: Advanced analytics platforms, such as Hadoop or Apache Spark, process large datasets to extract insights.
Visualization Layer: Insights are presented through dashboards or visualization tools, allowing stakeholders to make informed decisions.
This architecture supports the seamless flow of data from collection to actionable insights, enabling organizations to scale their IoT initiatives.
IoT and Machine Learning: Driving Smarter Solutions
The integration of machine learning with IoT Big Data creates smarter, more predictive systems. Machine learning models analyze the vast datasets generated by IoT devices to detect patterns, learn from them, and predict future outcomes. This combination unlocks powerful IoT Big Data solutions for industries ranging from healthcare to manufacturing.
Practical Example: In healthcare, IoT medical devices such as wearable fitness trackers and smart medical sensors monitor patients’ vitals, including heart rate, blood pressure, and oxygen levels. By feeding this data into machine learning models, healthcare providers can predict potential health risks and intervene early. For instance, machine learning algorithms can detect irregular heart patterns in real-time and alert doctors before a critical event occurs, ultimately saving lives.
In manufacturing, IoT sensors on equipment monitor real-time performance and detect potential failures. By integrating machine learning, manufacturers can predict when machinery is likely to fail and schedule maintenance ahead of time. This proactive approach reduces downtime and increases efficiency.
IoT Big Data Solutions: Real-World Impact
Industries are already reaping the benefits of IoT Big Data solutions, transforming how they operate and deliver value to customers.
Smart Cities: Cities like Barcelona and Singapore have deployed IoT sensors to monitor traffic patterns, optimize waste management, and manage energy consumption. With Big Data analytics, city administrators can improve urban planning and enhance the quality of life for residents. Smart traffic systems use IoT data to reduce congestion, while smart lighting systems adjust brightness based on real-time data to conserve energy.
Retail: IoT sensors in stores can monitor customer behavior, including how long they spend in certain areas or which products they interact with the most. Retailers like Amazon leverage this data to personalize in-store experiences, manage inventory more efficiently, and optimize store layouts. Amazon Go stores, for example, use IoT sensors to track what customers pick up, allowing for a seamless checkout-free shopping experience.
Agriculture: IoT devices in agriculture monitor soil conditions, weather patterns, and crop health. IoT Big Data analytics helps farmers optimize water usage, improve crop yields, and reduce waste. Companies like John Deere use IoT data from smart farming equipment to provide farmers with real-time insights on field conditions, enabling more precise and efficient farming practices.
Overcoming IoT Big Data Challenges
While the potential of IoT Big Data is vast, there are challenges that businesses need to overcome to fully realize its value.
Data Security: With the large volume of sensitive data being collected, organizations must prioritize the security of their IoT Big Data architecture. Ensuring data encryption, secure authentication, and regular vulnerability assessments are essential to safeguarding IoT data.
Data Quality: The sheer amount of data generated by IoT devices can lead to issues with data quality. Companies need to implement systems that filter out irrelevant or redundant data to ensure that only valuable insights are derived.
Scalability: As the number of connected devices grows, so does the complexity of managing IoT Big Data solutions. Businesses need scalable architectures that can handle exponential growth in data while maintaining efficiency.
The Future of IoT and Big Data
The convergence of IoT and Big Data analytics is set to drive significant advancements in many sectors, including healthcare, manufacturing, smart cities, and retail. As IoT devices become more ubiquitous, businesses will increasingly rely on IoT Big Data solutions to make data-driven decisions, improve efficiency, and create personalized experiences.
Looking ahead, the integration of artificial intelligence (AI) and machine learning with IoT will further enhance predictive capabilities, enabling even more accurate forecasting and decision-making. For instance, autonomous vehicles will rely heavily on IoT and Big Data analytics to process vast amounts of real-time data from sensors, allowing for safer and more efficient driving experiences.
Conclusion
The fusion of the Internet of Things and Big Data analytics offers unprecedented opportunities for businesses to harness the power of real-time data and make more informed, timely decisions. By implementing robust IoT Big Data architectures and integrating machine learning models, companies can derive actionable insights that lead to greater operational efficiency, improved customer experiences, and innovation across industries.
As IoT continues to evolve, businesses that invest in the right IoT Big Data solutions will be well-positioned to lead in a data-driven future.
Browse Related Blogs –
Revolutionize Your Healthcare Strategy with Big Data: What Every CXO Needs to Know
The Power of Customer Journey Mapping: Lessons from Amazon, Starbucks, Netflix and Disney
#iot and big data#iot data analytics#iot big data solutions#internet of things big data analytics#internet of things data analytics#iot big data architecture#iot big data machine learning#big data#internet of things
0 notes
Text
AI Consulting Business in Construction: Transforming the Industry
The construction industry is experiencing a profound transformation due to the integration of artificial intelligence (AI). The AI consulting business is at the forefront of this change, guiding construction firms in optimizing operations, enhancing safety, and improving project outcomes. This article explores various applications of AI in construction, supported by examples and statistics that…
#AI Consulting Business#AI in Construction#AI Technologies#artificial intelligence#Big Data Analytics#Construction Automation#construction efficiency#construction industry#Construction Safety#construction sustainability#Data Science#Generative Design#IoT Technologies#Labor Optimization#Machine Learning#Predictive Analytics#project management#quality control#Robotics#Safety Monitoring
0 notes
Text
Effiziente Lieferkettenoptimierung durch Datenanalyse-Techniken
Die Optimierung von Lieferketten ist ein entscheidender Faktor für die Wettbewerbsfähigkeit von Unternehmen im globalen Markt. Angesichts der zunehmenden Komplexität und Dynamik von Lieferketten ist die Anwendung von Datenanalyse-Techniken von zentraler Bedeutung, um Effizienzgewinne und Kostensenkungen zu realisieren. In diesem Artikel werden wir untersuchen, wie Data Analytics zur Verbesserung…
#Big Data#Data Analytics#Datenanalyse#Effizienzsteigerung#IoT#Kundenzufriedenheit#Machine Learning#Predictive Analytics#Prozessoptimierung#Risikomanagement#Transparenz#Unternehmenskultur
0 notes
Text
Big Data vs. Traditional Data: Understanding the Differences and When to Use Python

In the evolving landscape of data science, understanding the nuances between big data and traditional data is crucial. Both play pivotal roles in analytics, but their characteristics, processing methods, and use cases differ significantly. Python, a powerful and versatile programming language, has become an indispensable tool for handling both types of data. This blog will explore the differences between big data and traditional data and explain when to use Python, emphasizing the importance of enrolling in a data science training program to master these skills.
What is Traditional Data?
Traditional data refers to structured data typically stored in relational databases and managed using SQL (Structured Query Language). This data is often transactional and includes records such as sales transactions, customer information, and inventory levels.
Characteristics of Traditional Data:
Structured Format: Traditional data is organized in a structured format, usually in rows and columns within relational databases.
Manageable Volume: The volume of traditional data is relatively small and manageable, often ranging from gigabytes to terabytes.
Fixed Schema: The schema, or structure, of traditional data is predefined and consistent, making it easy to query and analyze.
Use Cases of Traditional Data:
Transaction Processing: Traditional data is used for transaction processing in industries like finance and retail, where accurate and reliable records are essential.
Customer Relationship Management (CRM): Businesses use traditional data to manage customer relationships, track interactions, and analyze customer behavior.
Inventory Management: Traditional data is used to monitor and manage inventory levels, ensuring optimal stock levels and efficient supply chain operations.
What is Big Data?
Big data refers to extremely large and complex datasets that cannot be managed and processed using traditional database systems. It encompasses structured, unstructured, and semi-structured data from various sources, including social media, sensors, and log files.
Characteristics of Big Data:
Volume: Big data involves vast amounts of data, often measured in petabytes or exabytes.
Velocity: Big data is generated at high speed, requiring real-time or near-real-time processing.
Variety: Big data comes in diverse formats, including text, images, videos, and sensor data.
Veracity: Big data can be noisy and uncertain, requiring advanced techniques to ensure data quality and accuracy.
Use Cases of Big Data:
Predictive Analytics: Big data is used for predictive analytics in fields like healthcare, finance, and marketing, where it helps forecast trends and behaviors.
IoT (Internet of Things): Big data from IoT devices is used to monitor and analyze physical systems, such as smart cities, industrial machines, and connected vehicles.
Social Media Analysis: Big data from social media platforms is analyzed to understand user sentiments, trends, and behavior patterns.
Python: The Versatile Tool for Data Science
Python has emerged as the go-to programming language for data science due to its simplicity, versatility, and robust ecosystem of libraries and frameworks. Whether dealing with traditional data or big data, Python provides powerful tools and techniques to analyze and visualize data effectively.
Python for Traditional Data:
Pandas: The Pandas library in Python is ideal for handling traditional data. It offers data structures like DataFrames that facilitate easy manipulation, analysis, and visualization of structured data.
SQLAlchemy: Python's SQLAlchemy library provides a powerful toolkit for working with relational databases, allowing seamless integration with SQL databases for querying and data manipulation.
Python for Big Data:
PySpark: PySpark, the Python API for Apache Spark, is designed for big data processing. It enables distributed computing and parallel processing, making it suitable for handling large-scale datasets.
Dask: Dask is a flexible parallel computing library in Python that scales from single machines to large clusters, making it an excellent choice for big data analytics.
When to Use Python for Data Science
Understanding when to use Python for different types of data is crucial for effective data analysis and decision-making.
Traditional Data:
Business Analytics: Use Python for traditional data analytics in business scenarios, such as sales forecasting, customer segmentation, and financial analysis. Python's libraries, like Pandas and Matplotlib, offer comprehensive tools for these tasks.
Data Cleaning and Transformation: Python is highly effective for data cleaning and transformation, ensuring that traditional data is accurate, consistent, and ready for analysis.
Big Data:
Real-Time Analytics: When dealing with real-time data streams from IoT devices or social media platforms, Python's integration with big data frameworks like Apache Spark enables efficient processing and analysis.
Large-Scale Machine Learning: For large-scale machine learning projects, Python's compatibility with libraries like TensorFlow and PyTorch, combined with big data processing tools, makes it an ideal choice.
The Importance of Data Science Training Programs
To effectively navigate the complexities of both traditional data and big data, it is essential to acquire the right skills and knowledge. Data science training programs provide comprehensive education and hands-on experience in data science tools and techniques.
Comprehensive Curriculum: Data science training programs cover a wide range of topics, including data analysis, machine learning, big data processing, and data visualization, ensuring a well-rounded education.
Practical Experience: These programs emphasize practical learning through projects and case studies, allowing students to apply theoretical knowledge to real-world scenarios.
Expert Guidance: Experienced instructors and industry mentors offer valuable insights and support, helping students master the complexities of data science.
Career Opportunities: Graduates of data science training programs are in high demand across various industries, with opportunities to work on innovative projects and drive data-driven decision-making.
Conclusion
Understanding the differences between big data and traditional data is fundamental for any aspiring data scientist. While traditional data is structured, manageable, and used for transaction processing, big data is vast, varied, and requires advanced tools for real-time processing and analysis. Python, with its robust ecosystem of libraries and frameworks, is an indispensable tool for handling both types of data effectively.
Enrolling in a data science training program equips you with the skills and knowledge needed to navigate the complexities of data science. Whether you're working with traditional data or big data, mastering Python and other data science tools will enable you to extract valuable insights and drive innovation in your field. Start your journey today and unlock the potential of data science with a comprehensive training program.
#Big Data#Traditional Data#Data Science#Python Programming#Data Analysis#Machine Learning#Predictive Analytics#Data Science Training Program#SQL#Data Visualization#Business Analytics#Real-Time Analytics#IoT Data#Data Transformation
0 notes
Text
How to Create Stunning Graphics with Adobe Photoshop
Introduction
Adobe Photoshop is the preferred software for graphic designers, photographers, and digital artists worldwide. Its powerful tools and versatile features lead to the foundation of an essential application that one needs to create the best kind of graphics. Mastering Photoshop can improve your creative-level projects, whether you are a beginner or an experienced user. In this tutorial, we will walk you through the basics and advanced techniques so you can create stunning graphics with the help of Adobe Photoshop. Read to continue
#Technology#Science#business tech#Adobe cloud#Trends#Nvidia Drive#Analysis#Tech news#Science updates#Digital advancements#Tech trends#Science breakthroughs#Data analysis#Artificial intelligence#Machine learning#Ms office 365#Quantum computing#virtual lab#fashion institute of technology#solid state battery#elon musk internet#Cybersecurity#Internet of Things (IoT)#Big data#technology applications
0 notes
Text
The Future of Market Research: Unveiling the Top 10 Emerging Trends
The landscape of market research is undergoing a transformative shift, driven by the convergence of technology, consumer behavior, and data-driven insights. Embracing these six emerging trends empowers businesses to connect with their target audiences on a deeper level, adapt to changing market dynamics, and make informed decisions that drive success
#Artificial intelligence (AI)#Augmented reality (AR) and virtual reality (VR)#Automation#Big data#Blockchain technology#Consumer behavior#Customer experience (CX)#Data analytics#Digital transformation#Emerging trends#Ethnographic research#Future of market research#Internet of Things (IoT)#Machine learning#market research#market xcel#Mobile market research#Personalization#Predictive analytics#Social media analytics#Voice of the customer (VoC)
0 notes
Text
An Introduction to Cybersecurity
I created this post for the Studyblr Masterpost Jam, check out the tag for more cool masterposts from folks in the studyblr community!
What is cybersecurity?
Cybersecurity is all about securing technology and processes - making sure that the software, hardware, and networks that run the world do exactly what they need to do and can't be abused by bad actors.
The CIA triad is a concept used to explain the three goals of cybersecurity. The pieces are:
Confidentiality: ensuring that information is kept secret, so it can only be viewed by the people who are allowed to do so. This involves encrypting data, requiring authentication before viewing data, and more.
Integrity: ensuring that information is trustworthy and cannot be tampered with. For example, this involves making sure that no one changes the contents of the file you're trying to download or intercepts your text messages.
Availability: ensuring that the services you need are there when you need them. Blocking every single person from accessing a piece of valuable information would be secure, but completely unusable, so we have to think about availability. This can also mean blocking DDoS attacks or fixing flaws in software that cause crashes or service issues.
What are some specializations within cybersecurity? What do cybersecurity professionals do?
incident response
digital forensics (often combined with incident response in the acronym DFIR)
reverse engineering
cryptography
governance/compliance/risk management
penetration testing/ethical hacking
vulnerability research/bug bounty
threat intelligence
cloud security
industrial/IoT security, often called Operational Technology (OT)
security engineering/writing code for cybersecurity tools (this is what I do!)
and more!
Where do cybersecurity professionals work?
I view the industry in three big chunks: vendors, everyday companies (for lack of a better term), and government. It's more complicated than that, but it helps.
Vendors make and sell security tools or services to other companies. Some examples are Crowdstrike, Cisco, Microsoft, Palo Alto, EY, etc. Vendors can be giant multinational corporations or small startups. Security tools can include software and hardware, while services can include consulting, technical support, or incident response or digital forensics services. Some companies are Managed Security Service Providers (MSSPs), which means that they serve as the security team for many other (often small) businesses.
Everyday companies include everyone from giant companies like Coca-Cola to the mom and pop shop down the street. Every company is a tech company now, and someone has to be in charge of securing things. Some businesses will have their own internal security teams that respond to incidents. Many companies buy tools provided by vendors like the ones above, and someone has to manage them. Small companies with small tech departments might dump all cybersecurity responsibilities on the IT team (or outsource things to a MSSP), or larger ones may have a dedicated security staff.
Government cybersecurity work can involve a lot of things, from securing the local water supply to working for the big three letter agencies. In the U.S. at least, there are also a lot of government contractors, who are their own individual companies but the vast majority of what they do is for the government. MITRE is one example, and the federal research labs and some university-affiliated labs are an extension of this. Government work and military contractor work are where geopolitics and ethics come into play most clearly, so just… be mindful.
What do academics in cybersecurity research?
A wide variety of things! You can get a good idea by browsing the papers from the ACM's Computer and Communications Security Conference. Some of the big research areas that I'm aware of are:
cryptography & post-quantum cryptography
machine learning model security & alignment
formal proofs of a program & programming language security
security & privacy
security of network protocols
vulnerability research & developing new attack vectors
Cybersecurity seems niche at first, but it actually covers a huge range of topics all across technology and policy. It's vital to running the world today, and I'm obviously biased but I think it's a fascinating topic to learn about. I'll be posting a new cybersecurity masterpost each day this week as a part of the #StudyblrMasterpostJam, so keep an eye out for tomorrow's post! In the meantime, check out the tag and see what other folks are posting about :D
#studyblrmasterpostjam#studyblr#cybersecurity#masterpost#ref#I love that this challenge is just a reason for people to talk about their passions and I'm so excited to read what everyone posts!
47 notes
·
View notes
Text
AI and Neural Networks: Transforming the Future
The COVID-19 pandemic has pushed the incorporation of Artificial Intelligence in the education industry, changing it into a powerful tool for learning and adaptation. In education, AI and Neural Networks are directing a significant revolution, adding to personalized learning, automating tasks, and offering insights into student performance amid global and Indian challenges like access variations and advancing teaching methods. AI in education draws inspiration from psychological studies on learning in humans and animals, utilizing machine learning to derive knowledge from data, which is particularly beneficial in the complex educational environment due to the diverse data distribution and vast datasets available for exploration.
In personalized and adaptive learning environments, the learning path adjusts continuously based on individual student characteristics and knowledge levels, optimizing learning outcomes conveniently. Integrating AI, big data, and network technologies is remodelling traditional classrooms into dynamic smart classrooms, increasing interactive learning experiences, and facilitating personalized education tailored to individual student needs. Neural networks in education boost feedback, assessment, and personalized learning by analyzing student data and fostering collaboration through online tools and platforms. Neural networks also support, intelligent tutoring systems like Knewton, and educational games such as DragonBox, fostering interactive and immersive learning environments.
Bringing some facts into light, the AI in the education market is expected to peak, from $3.79 U.S. dollars in 2022 to a whopping $20.54 U.S. dollars in 2027. Until today, the worldwide market for AI in education has witnessed significant growth, expanding from USD 537.3 million in 2018 to USD 3,683.5 million by 2023. According to a market forecast by Statista, in 2019, the worldwide e-learning market was valued at nearly $200 billion.
Citing few examples, in the process of theme logo design in a research study based on a digital network learning platform, AI computer-aided art teaching model has played an important role. A convolutional neural network (CNN) model for fuzzy classification, classifies gesture images into four blur categories: motion, defocus, Gaussian, and box blur. Furthermore, a Google scholar proposed an online oral English teaching platform based on the Internet of Things (IoT) to overcome the problems of low fluency and operability of the current online oral English teaching platform. A virtual teaching environment is constructed. A spoken English teaching system is used to correct the user's pronunciation and mouth movements.
AI has significantly strengthened education through applications like personalized learning, chatbots, virtual tutors, content recommendation systems, automated grading, and language processing tools. In India, platforms like SWAYAM has adopted AI to provide personalized learning experiences, expanding access to high-quality education. Founded in 2011, BYJU's has transformed the Indian edtech sector by leveraging Neural Networks (NN) and Artificial Intelligence (AI) to deliver personalized learning experiences, evaluating student interactions to adapt content dynamically and provide targeted support across K-12 education and competitive exam preparation globally. Founded in 2012, Coursera leverages Artificial Intelligence (AI) and Neural Networks (NN) to lift global online learning experiences through automated grading and personalized course recommendations tailored to individual interests and career goals, thereby raising grading proficiency and student engagement while improving course completion rates. Duolingo has reformed global language education with its AI-driven platform that personalizes learning through neural networks, offering interactive features like pronunciation analysis, grammar checking, and customized practice sessions to elevate user proficiency and engagement.
From online textbooks to remote lectures, AI is upgrading and automating various aspects of education. This progress holds huge potential for building learning outcomes for both students and educators. With AI as a valuable tool, the future of education looks promising, fostering a more efficient and inclusive learning environment for all. In conclusion, the integration of AI and NN has led the way for a new era of education, offering creative solutions to challenges, modifying the learning experience. As we navigate the future, responsible adoption, ethical considerations, and a commitment to equity will be essential in realizing the altering power of these technologies.
2 notes
·
View notes
Text
What is artificial intelligence (AI)?
Imagine asking Siri about the weather, receiving a personalized Netflix recommendation, or unlocking your phone with facial recognition. These everyday conveniences are powered by Artificial Intelligence (AI), a transformative technology reshaping our world. This post delves into AI, exploring its definition, history, mechanisms, applications, ethical dilemmas, and future potential.
What is Artificial Intelligence? Definition: AI refers to machines or software designed to mimic human intelligence, performing tasks like learning, problem-solving, and decision-making. Unlike basic automation, AI adapts and improves through experience.
Brief History:
1950: Alan Turing proposes the Turing Test, questioning if machines can think.
1956: The Dartmouth Conference coins the term "Artificial Intelligence," sparking early optimism.
1970s–80s: "AI winters" due to unmet expectations, followed by resurgence in the 2000s with advances in computing and data availability.
21st Century: Breakthroughs in machine learning and neural networks drive AI into mainstream use.
How Does AI Work? AI systems process vast data to identify patterns and make decisions. Key components include:
Machine Learning (ML): A subset where algorithms learn from data.
Supervised Learning: Uses labeled data (e.g., spam detection).
Unsupervised Learning: Finds patterns in unlabeled data (e.g., customer segmentation).
Reinforcement Learning: Learns via trial and error (e.g., AlphaGo).
Neural Networks & Deep Learning: Inspired by the human brain, these layered algorithms excel in tasks like image recognition.
Big Data & GPUs: Massive datasets and powerful processors enable training complex models.
Types of AI
Narrow AI: Specialized in one task (e.g., Alexa, chess engines).
General AI: Hypothetical, human-like adaptability (not yet realized).
Superintelligence: A speculative future AI surpassing human intellect.
Other Classifications:
Reactive Machines: Respond to inputs without memory (e.g., IBM’s Deep Blue).
Limited Memory: Uses past data (e.g., self-driving cars).
Theory of Mind: Understands emotions (in research).
Self-Aware: Conscious AI (purely theoretical).
Applications of AI
Healthcare: Diagnosing diseases via imaging, accelerating drug discovery.
Finance: Detecting fraud, algorithmic trading, and robo-advisors.
Retail: Personalized recommendations, inventory management.
Manufacturing: Predictive maintenance using IoT sensors.
Entertainment: AI-generated music, art, and deepfake technology.
Autonomous Systems: Self-driving cars (Tesla, Waymo), delivery drones.
Ethical Considerations
Bias & Fairness: Biased training data can lead to discriminatory outcomes (e.g., facial recognition errors in darker skin tones).
Privacy: Concerns over data collection by smart devices and surveillance systems.
Job Displacement: Automation risks certain roles but may create new industries.
Accountability: Determining liability for AI errors (e.g., autonomous vehicle accidents).
The Future of AI
Integration: Smarter personal assistants, seamless human-AI collaboration.
Advancements: Improved natural language processing (e.g., ChatGPT), climate change solutions (optimizing energy grids).
Regulation: Growing need for ethical guidelines and governance frameworks.
Conclusion AI holds immense potential to revolutionize industries, enhance efficiency, and solve global challenges. However, balancing innovation with ethical stewardship is crucial. By fostering responsible development, society can harness AI’s benefits while mitigating risks.
2 notes
·
View notes
Text
Why Python Will Thrive: Future Trends and Applications
Python has already made a significant impact in the tech world, and its trajectory for the future is even more promising. From its simplicity and versatility to its widespread use in cutting-edge technologies, Python is expected to continue thriving in the coming years. Considering the kind support of Python Course in Chennai Whatever your level of experience or reason for switching from another programming language, learning Python gets much more fun.
Let's explore why Python will remain at the forefront of software development and what trends and applications will contribute to its ongoing dominance.
1. Artificial Intelligence and Machine Learning
Python is already the go-to language for AI and machine learning, and its role in these fields is set to expand further. With powerful libraries such as TensorFlow, PyTorch, and Scikit-learn, Python simplifies the development of machine learning models and artificial intelligence applications. As more industries integrate AI for automation, personalization, and predictive analytics, Python will remain a core language for developing intelligent systems.
2. Data Science and Big Data
Data science is one of the most significant areas where Python has excelled. Libraries like Pandas, NumPy, and Matplotlib make data manipulation and visualization simple and efficient. As companies and organizations continue to generate and analyze vast amounts of data, Python’s ability to process, clean, and visualize big data will only become more critical. Additionally, Python’s compatibility with big data platforms like Hadoop and Apache Spark ensures that it will remain a major player in data-driven decision-making.
3. Web Development
Python’s role in web development is growing thanks to frameworks like Django and Flask, which provide robust, scalable, and secure solutions for building web applications. With the increasing demand for interactive websites and APIs, Python is well-positioned to continue serving as a top language for backend development. Its integration with cloud computing platforms will also fuel its growth in building modern web applications that scale efficiently.
4. Automation and Scripting
Automation is another area where Python excels. Developers use Python to automate tasks ranging from system administration to testing and deployment. With the rise of DevOps practices and the growing demand for workflow automation, Python’s role in streamlining repetitive processes will continue to grow. Businesses across industries will rely on Python to boost productivity, reduce errors, and optimize performance. With the aid of Best Online Training & Placement Programs, which offer comprehensive training and job placement support to anyone looking to develop their talents, it’s easier to learn this tool and advance your career.
5. Cybersecurity and Ethical Hacking
With cyber threats becoming increasingly sophisticated, cybersecurity is a critical concern for businesses worldwide. Python is widely used for penetration testing, vulnerability scanning, and threat detection due to its simplicity and effectiveness. Libraries like Scapy and PyCrypto make Python an excellent choice for ethical hacking and security professionals. As the need for robust cybersecurity measures increases, Python’s role in safeguarding digital assets will continue to thrive.
6. Internet of Things (IoT)
Python’s compatibility with microcontrollers and embedded systems makes it a strong contender in the growing field of IoT. Frameworks like MicroPython and CircuitPython enable developers to build IoT applications efficiently, whether for home automation, smart cities, or industrial systems. As the number of connected devices continues to rise, Python will remain a dominant language for creating scalable and reliable IoT solutions.
7. Cloud Computing and Serverless Architectures
The rise of cloud computing and serverless architectures has created new opportunities for Python. Cloud platforms like AWS, Google Cloud, and Microsoft Azure all support Python, allowing developers to build scalable and cost-efficient applications. With its flexibility and integration capabilities, Python is perfectly suited for developing cloud-based applications, serverless functions, and microservices.
8. Gaming and Virtual Reality
Python has long been used in game development, with libraries such as Pygame offering simple tools to create 2D games. However, as gaming and virtual reality (VR) technologies evolve, Python’s role in developing immersive experiences will grow. The language’s ease of use and integration with game engines will make it a popular choice for building gaming platforms, VR applications, and simulations.
9. Expanding Job Market
As Python’s applications continue to grow, so does the demand for Python developers. From startups to tech giants like Google, Facebook, and Amazon, companies across industries are seeking professionals who are proficient in Python. The increasing adoption of Python in various fields, including data science, AI, cybersecurity, and cloud computing, ensures a thriving job market for Python developers in the future.
10. Constant Evolution and Community Support
Python’s open-source nature means that it’s constantly evolving with new libraries, frameworks, and features. Its vibrant community of developers contributes to its growth and ensures that Python stays relevant to emerging trends and technologies. Whether it’s a new tool for AI or a breakthrough in web development, Python’s community is always working to improve the language and make it more efficient for developers.
Conclusion
Python’s future is bright, with its presence continuing to grow in AI, data science, automation, web development, and beyond. As industries become increasingly data-driven, automated, and connected, Python’s simplicity, versatility, and strong community support make it an ideal choice for developers. Whether you are a beginner looking to start your coding journey or a seasoned professional exploring new career opportunities, learning Python offers long-term benefits in a rapidly evolving tech landscape.
#python course#python training#python#technology#tech#python programming#python online training#python online course#python online classes#python certification
2 notes
·
View notes
Text
How-To IT
Topic: Core areas of IT
1. Hardware
• Computers (Desktops, Laptops, Workstations)
• Servers and Data Centers
• Networking Devices (Routers, Switches, Modems)
• Storage Devices (HDDs, SSDs, NAS)
• Peripheral Devices (Printers, Scanners, Monitors)
2. Software
• Operating Systems (Windows, Linux, macOS)
• Application Software (Office Suites, ERP, CRM)
• Development Software (IDEs, Code Libraries, APIs)
• Middleware (Integration Tools)
• Security Software (Antivirus, Firewalls, SIEM)
3. Networking and Telecommunications
• LAN/WAN Infrastructure
• Wireless Networking (Wi-Fi, 5G)
• VPNs (Virtual Private Networks)
• Communication Systems (VoIP, Email Servers)
• Internet Services
4. Data Management
• Databases (SQL, NoSQL)
• Data Warehousing
• Big Data Technologies (Hadoop, Spark)
• Backup and Recovery Systems
• Data Integration Tools
5. Cybersecurity
• Network Security
• Endpoint Protection
• Identity and Access Management (IAM)
• Threat Detection and Incident Response
• Encryption and Data Privacy
6. Software Development
• Front-End Development (UI/UX Design)
• Back-End Development
• DevOps and CI/CD Pipelines
• Mobile App Development
• Cloud-Native Development
7. Cloud Computing
• Infrastructure as a Service (IaaS)
• Platform as a Service (PaaS)
• Software as a Service (SaaS)
• Serverless Computing
• Cloud Storage and Management
8. IT Support and Services
• Help Desk Support
• IT Service Management (ITSM)
• System Administration
• Hardware and Software Troubleshooting
• End-User Training
9. Artificial Intelligence and Machine Learning
• AI Algorithms and Frameworks
• Natural Language Processing (NLP)
• Computer Vision
• Robotics
• Predictive Analytics
10. Business Intelligence and Analytics
• Reporting Tools (Tableau, Power BI)
• Data Visualization
• Business Analytics Platforms
• Predictive Modeling
11. Internet of Things (IoT)
• IoT Devices and Sensors
• IoT Platforms
• Edge Computing
• Smart Systems (Homes, Cities, Vehicles)
12. Enterprise Systems
• Enterprise Resource Planning (ERP)
• Customer Relationship Management (CRM)
• Human Resource Management Systems (HRMS)
• Supply Chain Management Systems
13. IT Governance and Compliance
• ITIL (Information Technology Infrastructure Library)
• COBIT (Control Objectives for Information Technologies)
• ISO/IEC Standards
• Regulatory Compliance (GDPR, HIPAA, SOX)
14. Emerging Technologies
• Blockchain
• Quantum Computing
• Augmented Reality (AR) and Virtual Reality (VR)
• 3D Printing
• Digital Twins
15. IT Project Management
• Agile, Scrum, and Kanban
• Waterfall Methodology
• Resource Allocation
• Risk Management
16. IT Infrastructure
• Data Centers
• Virtualization (VMware, Hyper-V)
• Disaster Recovery Planning
• Load Balancing
17. IT Education and Certifications
• Vendor Certifications (Microsoft, Cisco, AWS)
• Training and Development Programs
• Online Learning Platforms
18. IT Operations and Monitoring
• Performance Monitoring (APM, Network Monitoring)
• IT Asset Management
• Event and Incident Management
19. Software Testing
• Manual Testing: Human testers evaluate software by executing test cases without using automation tools.
• Automated Testing: Use of testing tools (e.g., Selenium, JUnit) to run automated scripts and check software behavior.
• Functional Testing: Validating that the software performs its intended functions.
• Non-Functional Testing: Assessing non-functional aspects such as performance, usability, and security.
• Unit Testing: Testing individual components or units of code for correctness.
• Integration Testing: Ensuring that different modules or systems work together as expected.
• System Testing: Verifying the complete software system’s behavior against requirements.
• Acceptance Testing: Conducting tests to confirm that the software meets business requirements (including UAT - User Acceptance Testing).
• Regression Testing: Ensuring that new changes or features do not negatively affect existing functionalities.
• Performance Testing: Testing software performance under various conditions (load, stress, scalability).
• Security Testing: Identifying vulnerabilities and assessing the software’s ability to protect data.
• Compatibility Testing: Ensuring the software works on different operating systems, browsers, or devices.
• Continuous Testing: Integrating testing into the development lifecycle to provide quick feedback and minimize bugs.
• Test Automation Frameworks: Tools and structures used to automate testing processes (e.g., TestNG, Appium).
19. VoIP (Voice over IP)
VoIP Protocols & Standards
• SIP (Session Initiation Protocol)
• H.323
• RTP (Real-Time Transport Protocol)
• MGCP (Media Gateway Control Protocol)
VoIP Hardware
• IP Phones (Desk Phones, Mobile Clients)
• VoIP Gateways
• Analog Telephone Adapters (ATAs)
• VoIP Servers
• Network Switches/ Routers for VoIP
VoIP Software
• Softphones (e.g., Zoiper, X-Lite)
• PBX (Private Branch Exchange) Systems
• VoIP Management Software
• Call Center Solutions (e.g., Asterisk, 3CX)
VoIP Network Infrastructure
• Quality of Service (QoS) Configuration
• VPNs (Virtual Private Networks) for VoIP
• VoIP Traffic Shaping & Bandwidth Management
• Firewall and Security Configurations for VoIP
• Network Monitoring & Optimization Tools
VoIP Security
• Encryption (SRTP, TLS)
• Authentication and Authorization
• Firewall & Intrusion Detection Systems
• VoIP Fraud DetectionVoIP Providers
• Hosted VoIP Services (e.g., RingCentral, Vonage)
• SIP Trunking Providers
• PBX Hosting & Managed Services
VoIP Quality and Testing
• Call Quality Monitoring
• Latency, Jitter, and Packet Loss Testing
• VoIP Performance Metrics and Reporting Tools
• User Acceptance Testing (UAT) for VoIP Systems
Integration with Other Systems
• CRM Integration (e.g., Salesforce with VoIP)
• Unified Communications (UC) Solutions
• Contact Center Integration
• Email, Chat, and Video Communication Integration
2 notes
·
View notes
Text
Revolutionizing Healthcare: The Role of Cloud Computing in Modern Healthcare Technologies
In today’s digital era, cloud computing is transforming industries, and healthcare is no exception. The integration of cloud computing healthcare technologies is reshaping patient care, medical research, and healthcare management. Let’s explore how cloud computing is revolutionizing healthcare and the benefits it brings.
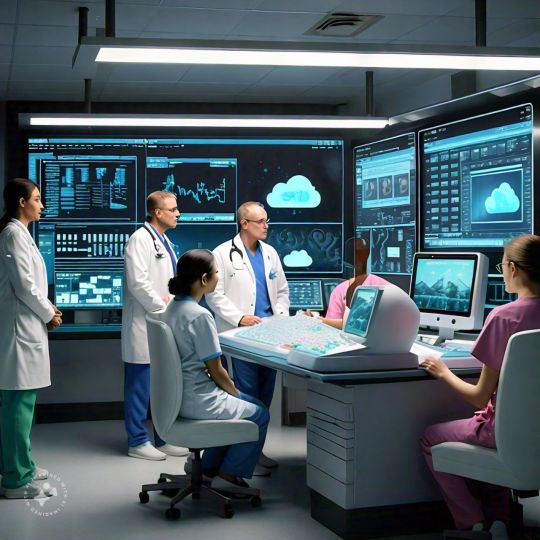
What is Cloud Computing in Healthcare?
Cloud computing in healthcare refers to the use of remote servers to store, manage, and process healthcare data, rather than relying on local servers or personal computers. This technology allows healthcare organizations to access vast amounts of data, collaborate with other institutions, and scale operations seamlessly.
Download PDF Brochure
Key Benefits of Cloud Computing in Healthcare
Enhanced Data Storage and Accessibility Cloud technology allows healthcare providers to store massive volumes of patient data, including medical records, images, and test results, securely. Clinicians can access this data from anywhere, ensuring that patient information is available for timely decision-making.
Improved Collaboration Cloud-based healthcare platforms enable easy sharing of patient data between healthcare providers, specialists, and labs. This facilitates better collaboration and more accurate diagnoses and treatment plans, especially in multi-disciplinary cases.
Cost Efficiency The cloud reduces the need for expensive hardware, software, and in-house IT teams. Healthcare providers only pay for the resources they use, making it a cost-effective solution. Additionally, the scalability of cloud systems ensures they can grow as healthcare organizations expand.
Better Data Security Protecting sensitive patient information is critical in healthcare. Cloud computing providers invest heavily in data security measures such as encryption, multi-factor authentication, and regular audits, ensuring compliance with regulatory standards like HIPAA.
Telemedicine and Remote Patient Monitoring Cloud computing powers telemedicine platforms, allowing patients to consult with doctors virtually, from the comfort of their homes. It also enables remote patient monitoring, where doctors can track patients' health metrics in real time, improving outcomes for chronic conditions.
Advanced Data Analytics The cloud supports the integration of advanced data analytics tools, including artificial intelligence (AI) and machine learning (ML), which can analyze large datasets to predict health trends, track disease outbreaks, and personalize treatment plans based on individual patient data.
Use Cases of Cloud Computing in Healthcare
Electronic Health Records (EHRs): Cloud-based EHRs allow healthcare providers to access and update patient records instantly, improving the quality of care.
Genomics and Precision Medicine: Cloud computing accelerates the processing of large datasets in genomics, supporting research and development in personalized medicine.
Hospital Information Systems (HIS): Cloud-powered HIS streamline hospital operations, from patient admissions to billing, improving efficiency.
Challenges in Cloud Computing for Healthcare
Despite its numerous benefits, there are challenges to implementing cloud computing in healthcare. These include:
Data Privacy Concerns: Although cloud providers offer robust security measures, healthcare organizations must ensure their systems are compliant with local and international regulations.
Integration with Legacy Systems: Many healthcare institutions still rely on outdated technology, making it challenging to integrate cloud solutions smoothly.
Staff Training: Healthcare professionals need adequate training to use cloud-based systems effectively.
Request Sample Pages
The Future of Cloud Computing in Healthcare
The future of healthcare will be increasingly cloud-centric. With advancements in AI, IoT, and big data analytics, cloud computing will continue to drive innovations in personalized medicine, population health management, and patient care. Additionally, with the growing trend of wearable devices and health apps, cloud computing will play a crucial role in integrating and managing data from diverse sources to provide a comprehensive view of patient health.
Conclusion
Cloud computing is not just a trend in healthcare; it is a transformative force driving the industry towards more efficient, secure, and patient-centric care. As healthcare organizations continue to adopt cloud technologies, we can expect to see improved patient outcomes, lower costs, and innovations that were once thought impossible.
Embracing cloud computing in healthcare is essential for any organization aiming to stay at the forefront of medical advancements and patient care.
Content Source:
2 notes
·
View notes
Text
From Novice to Pro: Master the Cloud with AWS Training!
In today's rapidly evolving technology landscape, cloud computing has emerged as a game-changer, providing businesses with unparalleled flexibility, scalability, and cost-efficiency. Among the various cloud platforms available, Amazon Web Services (AWS) stands out as a leader, offering a comprehensive suite of services and solutions. Whether you are a fresh graduate eager to kickstart your career or a seasoned professional looking to upskill, AWS training can be the gateway to success in the cloud. This article explores the key components of AWS training, the reasons why it is a compelling choice, the promising placement opportunities it brings, and the numerous benefits it offers.
Key Components of AWS Training
1. Foundational Knowledge: Building a Strong Base
AWS training starts by laying a solid foundation of cloud computing concepts and AWS-specific terminology. It covers essential topics such as virtualization, storage types, networking, and security fundamentals. This groundwork ensures that even individuals with little to no prior knowledge of cloud computing can grasp the intricacies of AWS technology easily.
2. Core Services: Exploring the AWS Portfolio
Once the fundamentals are in place, AWS training delves into the vast array of core services offered by the platform. Participants learn about compute services like Amazon Elastic Compute Cloud (EC2), storage options such as Amazon Simple Storage Service (S3), and database solutions like Amazon Relational Database Service (RDS). Additionally, they gain insights into services that enhance performance, scalability, and security, such as Amazon Virtual Private Cloud (VPC), AWS Identity and Access Management (IAM), and AWS CloudTrail.
3. Specialized Domains: Nurturing Expertise
As participants progress through the training, they have the opportunity to explore advanced and specialized areas within AWS. These can include topics like machine learning, big data analytics, Internet of Things (IoT), serverless computing, and DevOps practices. By delving into these niches, individuals can gain expertise in specific domains and position themselves as sought-after professionals in the industry.
Reasons to Choose AWS Training
1. Industry Dominance: Aligning with the Market Leader
One of the significant reasons to choose AWS training is the platform's unrivaled market dominance. With a staggering market share, AWS is trusted and adopted by businesses across industries worldwide. By acquiring AWS skills, individuals become part of the ecosystem that powers the digital transformation of numerous organizations, enhancing their career prospects significantly.
2. Comprehensive Learning Resources: Abundance of Educational Tools
AWS training offers a wealth of comprehensive learning resources, ranging from extensive documentation, tutorials, and whitepapers to hands-on labs and interactive courses. These resources cater to different learning preferences, enabling individuals to choose their preferred mode of learning and acquire a deep understanding of AWS services and concepts.
3. Recognized Certifications: Validating Expertise
AWS certifications are globally recognized credentials that validate an individual's competence in using AWS services and solutions effectively. By completing AWS training and obtaining certifications like AWS Certified Solutions Architect or AWS Certified Developer, individuals can boost their professional credibility, open doors to new job opportunities, and command higher salaries in the job market.
Placement Opportunities
Upon completing AWS training, individuals can explore a multitude of placement opportunities. The demand for professionals skilled in AWS is soaring, as organizations increasingly migrate their infrastructure to the cloud or adopt hybrid cloud strategies. From startups to multinational corporations, industries spanning finance, healthcare, retail, and more seek talented individuals who can architect, develop, and manage cloud-based solutions using AWS. This robust demand translates into a plethora of rewarding career options and a higher likelihood of finding positions that align with one's interests and aspirations.
In conclusion, mastering the cloud with AWS training at ACTE institute provides individuals with a solid foundation, comprehensive knowledge, and specialized expertise in one of the most dominant cloud platforms available. The reasons to choose AWS training are compelling, ranging from the industry's unparalleled market position to the top ranking state.
9 notes
·
View notes
Text
Navigating Java's Promising Future: A Closer Look at the Ever-Expanding Horizons of Technology
In the fast-paced world of technology, Java stands tall as a resilient language with boundless potential. Its enduring significance and robust ecosystem position it as a cornerstone of software development across various domains. Let's explore the myriad factors that contribute to Java's promising trajectory in the evolving landscape of technology.
1. Unmatched Versatility and Reach
Java's versatility knows no bounds, making it a preferred choice for developers across diverse industries. From web and mobile app development to enterprise solutions and big data processing, Java's adaptability ensures a wide spectrum of career opportunities. Its widespread adoption and proven track record make it a dependable foundation for building scalable and resilient applications.
2. Thriving Ecosystem and Community Support
At the core of Java's success lies its thriving ecosystem and vibrant community. With a vast array of libraries, frameworks, and tools, the Java ecosystem empowers developers to streamline development processes and create cutting-edge solutions. Furthermore, the active Java community fosters collaboration and innovation, driving the language's evolution to new heights.
3. Platform Agnosticism
Java's renowned "write once, run anywhere" principle remains a key advantage in today's multi-platform landscape. Developers can craft Java applications that seamlessly operate across different platforms and devices, ensuring interoperability and accessibility. This platform-agnostic approach simplifies development efforts and broadens the reach of Java-powered solutions.
4. Dominance in Enterprise Solutions
Java continues to maintain its stronghold in the enterprise sector, powering critical systems for numerous Fortune 500 companies. Its reliability, scalability, and robust security features make it the preferred choice for building large-scale enterprise applications. As businesses increasingly rely on digital solutions, the demand for skilled Java developers remains steadfast, offering lucrative prospects in corporate environments.
5. Seamless Integration with Emerging Technologies
Java's adaptability extends to emerging technologies such as cloud computing, artificial intelligence, machine learning, and the Internet of Things (IoT). Leveraging Java's robust frameworks and libraries, developers can innovate and build solutions that harness the potential of these cutting-edge technologies. Java's compatibility with emerging trends ensures its relevance and longevity in a rapidly evolving tech landscape.
6. Continuous Evolution and Enhancement
The Java platform undergoes continual evolution to stay at the forefront of industry trends and technological advancements. With each new release, such as Java 15 and beyond, developers gain access to enhanced features, improved performance, and strengthened security measures. Java's commitment to innovation ensures its competitiveness and relevance in the dynamic software development sphere.
7. Focus on Performance Optimization
Performance optimization remains a top priority for Java developers, driving efforts to enhance the language's efficiency and speed. Through bytecode enhancements, garbage collection improvements, and runtime optimizations, Java delivers exceptional performance in diverse computing environments. This relentless focus on performance ensures that Java remains a top choice for resource-intensive applications.
8. Accessibility and Education Initiatives
Java's popularity extends beyond professional development, making it a cornerstone in educational institutions and training programs worldwide. Abundant resources, tutorials, and online courses enable aspiring developers to learn Java and embark on rewarding careers in software development. Java's widespread use in academia fosters a steady influx of new talent, enriching the industry with fresh perspectives and ideas.
In summary, Java's future looks promising, driven by its unparalleled versatility, robust ecosystem, enterprise dominance, integration with emerging technologies, continuous evolution, performance optimization, and accessibility initiatives. As Java continues to adapt and innovate, it will remain a vital force in software development for years to come.
2 notes
·
View notes
Text
Future of data science?
Exciting developments await the future of data science as it evolves. Data scientists can expect to see greater integration of artificial intelligence (AI) and machine learning, facilitated by automation and AutoML, which will make data analysis more accessible. Proficiency in big data and real-time analytics will be crucial as the volume and complexity of data continue to expand. Additionally, ethical considerations such as privacy protection, bias detection, and responsible AI implementation will remain important focal points. Furthermore, data science will involve increased interdisciplinary collaboration that bridges technical expertise with domain knowledge and ethics.
Explainable AI will be necessary for the development of trust and compliance, while edge and IoT analytics will cater for the increased demand of IoT devices. In this way, data visualisation and storytelling skills will still be vital. Data scientists will need to adapt as organisations shift to hybrid and multi-cloud environments. This field will require continuous learning for success. The next quantum leap for data science with quantum computing. Customization and personalization will also define data science; this will deliver specific solutions for particular business needs. In the data-driven era, it’s the data scientists that will excel.
If you are considering preparing for this promising future, Edure provides a wide variety of comprehensive data science courses designed for students of all skill levels. Edure has a comprehensive curriculum that includes introductory courses in basic data science concepts, tools and techniques for beginners and advanced programs targeted for experienced professionals. These courses will give you the knowledge and the skills that will enable you to succeed in the data-driven world of tomorrow. Therefore, whether a beginner or an expert desiring to remain up to date in data science, Edure courses are valuable for launching into or deepening one’s involvement in this promising domain.
For more information contact as
Edure | Learn to Earn
Aristo Junction, Thampanoor,
Thiruvananthapuram, Kerala , 695001
+91 9746211123 +91 9746711123
2 notes
·
View notes
Text
How to Optimize Your Workflow with Task Management Software
Introduction
The world has become too fast-moving; hence, the demand for organization and productivity is higher than ever. From multiple tasks at hand to meeting deadlines, the pressure is always there to make things easier. Task management software provides a powerful solution for this issue. This guide will walk you through optimizing your workflow with task management software to bring about efficiency and effectiveness in both personal and professional aspects of life. Read to continue...
#Technology#Science#business tech#Adobe cloud#Trends#Nvidia Drive#Analysis#Tech news#Science updates#Digital advancements#Tech trends#Science breakthroughs#Data analysis#Artificial intelligence#Machine learning#Ms office 365#Quantum computing#virtual lab#fashion institute of technology#solid state battery#elon musk internet#Cybersecurity#Internet of Things (IoT)#Big data#technology applications
0 notes