#learn big data
Explore tagged Tumblr posts
Text
The Importance of Data Analytics for Better Business Decision Making
In today's business context, data has emerged as a critical asset for strategic decision-making processes. With the development of digital technologies and the rise of big data, organizations are increasingly turning to data analytics to gain actionable insights. This article investigates the revolutionary role of data analytics in business decision making, including its relevance, benefits, and limitations.
The significance of data analytics:
Data analytics is the methodical analysis of large databases to discover useful patterns, trends, and correlations. Organizations can extract important insights from heterogeneous data sources by using advanced statistical algorithms, machine learning approaches, and data visualization technologies. These insights serve as the cornerstone for educated decision-making in a variety of fields, including marketing, finance, operations, and customer service.
Enhancing Decision Making using Data Analytics:
Data analytics enables organizations to make data-driven decisions based on factual facts rather than intuition or hypothesis. Businesses may better predict industry trends, client preferences, and upcoming possibilities by analyzing historical data and real-time information. This allows them to better allocate resources, manage risks, and capitalize on competitive advantages.
Furthermore, data analytics enables scenario analysis and predictive modeling, allowing businesses to forecast future events and assess various courses of action. Whether it's anticipating sales performance, optimizing pricing tactics, or finding operational inefficiencies, data-driven insights help decision makers develop plans that are aligned with organizational goals.
Advantages of Data-Driven Decision Making:
Adoption of data analytics provides numerous benefits for firms looking to acquire a competitive advantage in today's dynamic industry. This includes:
Improved Accuracy: Data analytics allows organizations to base their decisions on empirical evidence, minimizing the possibility of errors or biases that come with subjective decision-making processes.
Improved Efficiency: By automating data analysis processes and streamlining decision-making workflows, firms can increase operational efficiency and resource utilization.
Enhanced Strategic Insights: Data analytics offers deeper insights into market dynamics, customer behavior, and competitive landscapes, allowing organizations to develop more successful strategic plans and initiatives.
Agility and Adaptability: Real-time analytics enable firms to respond swiftly to changing market conditions, new trends, and customer preferences, fostering agility and adaptability in decision making.
Challenges and Considerations: Data analytics offer significant benefits, but implementation can be challenging. Organizations must deal with problems such as data quality, privacy concerns, talent shortages, and technological difficulties. Furthermore, in the age of data-driven decision making, it is vital to ensure ethical data use and compliance with regulatory frameworks.
Conclusion:
In conclusion, data analytics has developed as a critical component of modern corporate decision making, providing organizations with unmatched insights into their operations, customers, and markets. Businesses that leverage the power of data analytics can open new opportunities, manage risks, and gain a competitive advantage in today's fast changing landscape. However, success in exploiting data analytics is dependent not only on technology skills, but also on organizational culture, talent development, and ethical concerns. As businesses embrace data-driven decision making, the revolutionary power of data analytics will continue to redefine industries and drive innovation.
Are you ready to unleash the potential of data analytics for your business? Join CACMS Institute for a thorough data analytics training today! Our hands-on practical training, conducted by qualified instructors, will provide you with the skills and information required to succeed in the field of data analytics.
Why should you choose CACMS Institute?
hands-on practical training
Expert teaching staff
Flexible timings to accommodate your schedule
Enroll now and take the first step towards understanding data analytics. For further information, please contact us at +91 8288040281 or visit http://cacms.in/big-data/ Don't pass up this opportunity to boost your career. Enroll in Amritsar's finest data analytics courses today!
#cacms institute#cacms#techskills#techeducation#data analytics training#data analytics#data analytics course#big data course#learn big data#data analyst course#data analyst training#best computer institute near me#best computer institute in Amritsar
0 notes
Text
Big Data Courses Online
Are you looking for Big Data Courses Online? If yes, Look no further. Rudras Social is one of the topmost name offering Big Data courses to improve your skills online today. Choose from a wide range of big data courses offered from top universities and industry leaders.
0 notes
Text
What does AI actually look like?
There has been a lot of talk about the negative externalities of AI, how much power it uses, how much water it uses, but I feel like people often discuss these things like they are abstract concepts, or people discuss AI like it is this intangible thing that exists off in "The cloud" somewhere, but I feel like a lot of people don't know what the infrastructure of AI actually is, and how it uses all that power and water, so I would like to recommend this video from Linus Tech Tips, where he looks at a supercomputer that is used for research in Canada. To be clear I do not have anything against supercomputers in general and they allow important work to be done, but before the AI bubble, you didn't need one, unless you needed it. The recent AI bubble is trying to get this stuff into the hands of way more people than needed them before, which is causing a lot more datacenter build up, which is causing their companies to abandon climate goals. So what does AI actually look like?
First of all, it uses a lot of hardware. It is basically normal computer hardware, there is just a lot of it networked together.
Hundreds of hard drives all spinning constantly
Each one of the blocks in this image is essentially a powerful PC, that you would still be happy to have as your daily driver today even though the video is seven years old. There are 576 of them, and other more powerful compute nodes for bigger datasets.
The GPU section, each one of these drawers contains like four datacenter level graphics cards. People are fitting a lot more of them into servers now than they were then.
Now for the cooling and the water. Each cabinet has a thick door, with a water cooled radiator in it. In summer, they even spray water onto the radiator directly so it can be cooled inside and out.
They are all fed from the pump room, which is the floor above. A bunch of pumps and pipes moving the water around, and it even has cooling towers outside that the water is pumped out into on hot days.
So is this cool? Yes. Is it useful? Also yes. Anyone doing biology, chemistry, physics, simulations, even stuff like social sciences, and even legitimate uses of analytical ai is glad stuff like this exists. It is very useful for analysing huge datasets, but how many people actually do that? Do you? The same kind of stuff is also used for big websites with youtube. But the question is, is it worth building hundreds more datacenters just like this one, so people can automatically generate their emails, have an automatic source of personal attention from a computer, and generate incoherent images for social media clicks? Didn't tech companies have climate targets, once?
107 notes
·
View notes
Text
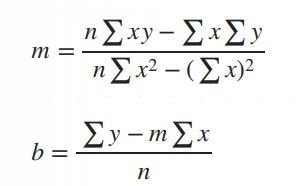
Simple Linear Regression in Data Science and machine learning
Simple linear regression is one of the most important techniques in data science and machine learning. It is the foundation of many statistical and machine learning models. Even though it is simple, its concepts are widely applicable in predicting outcomes and understanding relationships between variables.
This article will help you learn about:
1. What is simple linear regression and why it matters.
2. The step-by-step intuition behind it.
3. The math of finding slope() and intercept().
4. Simple linear regression coding using Python.
5. A practical real-world implementation.
If you are new to data science or machine learning, don’t worry! We will keep things simple so that you can follow along without any problems.
What is simple linear regression?
Simple linear regression is a method to model the relationship between two variables:
1. Independent variable (X): The input, also called the predictor or feature.
2. Dependent Variable (Y): The output or target value we want to predict.
The main purpose of simple linear regression is to find a straight line (called the regression line) that best fits the data. This line minimizes the error between the actual and predicted values.
The mathematical equation for the line is:
Y = mX + b
: The predicted values.
: The slope of the line (how steep it is).
: The intercept (the value of when).
Why use simple linear regression?
click here to read more https://datacienceatoz.blogspot.com/2025/01/simple-linear-regression-in-data.html
#artificial intelligence#bigdata#books#machine learning#machinelearning#programming#python#science#skills#big data#linear algebra#linear b#slope#interception
6 notes
·
View notes
Text
i know i've been on my anti-modern AU propaganda lately and it's just because i've been delving deeper into the sally face ao3 tags and i just keep finding them over and over. it's frustrating because there are a lot of really interesting concepts out there that would fit really well and make for a genuinely really interesting story in the 90s, but they get thrown off because the author doesn't know enough about the 90s to write for that time period, so they make it into a modern au instead. there's nothing inherently wrong with that, and i think there's room for modern aus to be done well here, i even have my own half-serious modern au, but i do think you often lose part of what makes sally face special when you turn the story into any other kind of contemporary love story/horror story/etc where all the characters just have ~iphones~ and use ~snapchat~ and all these things.
like, the 90s was not some kind of alien planet, and a vast number of the problems that you're solving with smartphones can be worked around very easily with just a bit of research or thought. long distance walkie-talkies, pagers, and PDAs were all (though sometimes expensive) perfectly capable contemporary technologies for talking to people when you are not physically with them. in fact, a lot of the abbreviations and slang we use over text right now was developed by young people in the 90s using pagers to talk to their friends. PDAs were a bit more out there in the 90s than they were in the aughts, but it's still completely plausible for henry in particular to have one, considering he already owns a home computer, which was not at all ubiquitous in the 90s. considering the apparent financial limitations that he and sal live under (it's never stated explicitly, but i mean, they live at addison's, they can't be in a great financial situation) and how insanely expensive computers were back then, it's more than likely that henry's job requires a home computer of some sort, meaning that a PDA would probably be incredibly useful to him if he were away from it, because there's no way in hell he's getting himself a luggable or another kind of early laptop to bring with him, that would've been too expensive.
and that's ignoring the fact that so many situations where two characters are apart form each other but need to communicate could just be fixed by rewriting the plot so that they can meet in person. i know that's not what people wanna hear because rewriting sucks, but you can find a lot of reasons for characters to meet each other randomly or to have reasons to meet up later if you give it a bit of problem-solving. part of what makes the pre-smartphone era interesting to write for and so optimized for horror, and probably a big reason that gabry chose this time period for the story in the first place, is the level of disconnection between each character in the story BECAUSE they don't have things like smartphones. having to work around this technological limitation is part of the fun, because you get a very enjoyable push and pull of closeness vs. disconnection between each character.
this is great for alienating ash, the only one who doesn't live in the apartments (except for neil), and causing her internal conflict about her relationships with the rest of her friends, especially as the story progresses and they start discovering more shit about the cult, and her instincts are to call the cops because she's a lot more normal than her friends are. or, it's good for alienating travis, who also doesn't live there and is far more isolated than everyone else (more on that next), or for creating an unhealthy and codependent relationship between sal and larry, who, with the walkies, are the only two in the friend group who DO have semi-instant access to each other all the time--all of which are plot points i put into my writing.
and if that's not enough, think about the implications for travis's character in particular. his father is a preacher, and a huge talking point of christian extremists in the 90s was that things like television were evil and demonic in some way. they campaigned against these things heavily. with the kind of person that we know kenneth phelps to be and the way many technologies we take for granted today, including TVs, were still being adopted by older generations, it's not out of the question at all that travis doesn't own something like a TV or a VCR, putting him even more out of the loop with what other people his age are doing than he already would be, having approximately 0 friends. he doesn't know what DND is, and he doesn't know how to look it up because he's not familiar with computers or the internet, he just knows his dad thinks it's demonic, so he steers clear of it.
the intention of cult leaders like kenneth is to keep their victims as isolated as possible, and not owning a TV, VCR, home computer, etc, is a great way to keep travis and his sisters isolated and disconnected from their peers, and therefore more connected to the cult, and it's a lot easier to justify not owning these things in the 90s, where the story already takes place, than it is if you're writing a modern au. a modern au for this situation would require all kinds of technological workarounds to make sure that travis owned a phone but couldn't do anything his father didn't want him doing on it. he's the kind of father who would go through and monitor his kid's texts, he wouldn't just let travis have snapchat or whatever, but i digress.
i know i'm just doing my petty bitching and people can do whatever they want however they want to, but i really do feel like there's a huge piece of the story that is lost in turning the sally face story as it is into some kind of modern au, and it's pretty unfortunate to me that people seem to think that the 90s was such a primitive alien world of incomprehensible technology that they don't want to write for that time period at all. it's really not as terrifying as it seems, genuinely. a surface level understanding of the era's technologies would be straightforward enough for anyone who wasn't there to write something perfectly coherent, if lacking in specific cultural/technological details that nobody but me cares about because i have autism.
if you're a sally face fan reading this and you struggle with writing for the american 90s because you weren't there, go look up pagers (also called beepers) and PDAs (which are basically early pocket computers) and how they work. ask older family members if or how they used them. go look at the different kinds of home computers of the era from companies like packard-bell and IBM. learn what a pentium III is/was, or what it means to be X86 compatible. look at the history of the CD-ROM, and how when it was invented, it could contain so much data that consumers had absolutely no idea what to do with them until people started putting video games on them. go watch cathode ray dude, LGR or techmoan on youtube.
go learn things about this era, it's good for you and you will have a lot of fun, even if you're not like me, i promise, and your fanfiction will be better for it. please learn about this era. take my hand. we can go to beautiful places together.
#txt#sally face#unwarranted infodump tag#anti modern au propaganda#i don't want to be mean i really don't#i want to encourage people to learn about this era#because the 80s 90s and 2000s were just#full of these huge technological booms#things that you just don't get nowadays#because most of consumer technology is a solved problem#and because capitalism is causing companies to eat each other and themselves#in a place where there can fundamentally be no competition anymore#it's genuinely amazing to see the technological advancements#and the cultural impacts that things like the walkman made#the fights between betamax and VHS#the death throes of the floppy as CDs came into the mix#the concept of computer tape as a whole#would throw so many young people nowadays for a loop#but computers used to have tape decks in them#because you stored the data for certain programs on tape#in an audio format#you can still find a lot of these programs on youtube#and if you were to play them in front of a computer#that read computer tape#then it would start the program that the data was for#it's awesome and it sucked big nasty hairy fucking balls#be glad you have the gift of hindsight here#so that you can learn about how interesting that technology was#instead of having to use it#like c'mon i want you to learn
8 notes
·
View notes
Text
I remember when 3D printers first became a thing and there was a huuuge hype about how you could 3D print anything and it would revolutionize everything
And then there was a phase of fussing around realizing that there are actually some unintuitive constraints on what shapes are printable based on slicing and support of overhangs, and how you have to do fiddly business like putting tape on the platform and watching the first couple layers like a hawk or it can detach and slump sideways and become a big horrible useless mess
And then, after all that, people kinda came around to realize that even if you get all that sorted, the object you've made is fundamentally an object made of moderately-well-adhered layers of brittle plastic, which is actually a pretty shitty material for almost every purpose.
And aside from a few particular use cases the whole hype just sort of dropped off to nothing.
Anyway I feel like we're seeing pretty much the same arc play out with generative AI.
#the major difference I think being that genAI does not allow you to make an unregistered gun.#Which as far as I know is the most significant lasting impact case for 3D printing.#And yes believe me I know that there are other forms of 3D printing beyond pla extrusion BUT all of them are fiddly enough#that they are functionally fabrication processes rather than 'haha just print it!!'#The whole big hype was about the intuitive ease of layered plastic printing and the cheapness and diy-ability of the setup of such a printe#This also works as a metaphorical parallel because there's likewise forms of machine learning for data processing that produce#useable outputs in specific fields. But those are software tools witb specific use cases#And not generic chatbot garbledegook.#The point I'm driving at here is that a chatgpt essay is equivalent to a pla printed coffee mug in terms of#'wow that's an object of such low quality as to be entirely pointless. Why did you make that.'
2 notes
·
View notes
Text
everything going wrong this season blame it on the a.i. (or fusebox, why not both)
#litg#like drake and josh use to say megan!#we say a.i.#fusebox using ai#just to get big money spenders#to buy them out#lol#love island the game#and they tried to convince us with a pic#that they had a human staff#lol a staff heavily dependent on ai#from one tech person to a company#you suck#learn to leverage ai for other things#writers should always write#ai should only track the data#idiots
12 notes
·
View notes
Text
Ouuurgggbhhhh I was tagging some posts for my queue and I offhandedly said something about a cat being perfect for Data cause they're autism creatures who don't show love in necessarily 'conventional' ways and like...it didn't hit me just how TRUE it actually is yanno?
Some people don't like cats because they don't love you the way DOGS do. Loudly, unconditionally, jumping all over you and making a scene of it.
But when a CAT loves you, it's because it chose you. You may not understand each other but it feels safe around you, it can be quiet and observe and sometimes will come up and cuddle you but the most important thing you can do is just be NEAR each other. Doing opposite things but in the same space because it's just better when you can look over and see them and know that they're YOURS....this isn't really about cats anymore 🥺🥺🥺🥺
#jane journals#self insert talk#💛 love makes us human 💛#OUGGGHHH#tbh we're lowkey dog and cat CODED#ofc my s/i being the dog#i love the idea of being excited about an anthropological find and infodumping to data about it#while he just stares with those big ol eyes and occasionally asks questions!#it's like a learning opportunity and like yeah maybe he could read about things and learn faster that way#but its just BETTER somehow hearing it from me 🥺👉👈💖💖💖
12 notes
·
View notes
Text
"Unleashing the Power of Big Data: Fueling AI and Machine Learning with Massive Amounts of Training Data"
Introduction:
The symbiotic relationship between big data, artificial intelligence (AI), and machine learning (ML) has become the catalyst for groundbreaking advancements in today's data-driven world. Big data, which consists of massive amounts of diverse and complex information, is the fuel that propels AI and ML forward. Big data enables AI algorithms to learn, adapt, and make intelligent decisions by providing the necessary training data. In this article, we will look at how big data acts as a fuel for AI and ML, allowing them to reach their full potential.
The Importance of Training Data: At the heart of AI and ML is the concept of training data. These algorithms learn from patterns and examples, and their performance improves as they are exposed to more relevant data. Big data plays an important role in training AI and ML models by providing massive amounts of training data. With such a wealth of data at their disposal, AI algorithms gain a broader understanding of the problem space and become more capable of making accurate predictions and informed decisions.
Unleashing the Potential of Big Data:
Unprecedented Insights: Big data provides an unprecedented opportunity to extract valuable insights. AI and ML algorithms can uncover hidden patterns, correlations, and trends in massive amounts of structured and unstructured data by analyzing massive amounts of structured and unstructured data. This enables businesses and organizations to make data-driven decisions, optimize processes, and gain a competitive advantage.
Enhanced Personalization: Using big data, AI and ML models can personalize user experiences by providing tailored recommendations, targeted advertisements, and customized services. These algorithms can deliver personalized and relevant content by analyzing vast amounts of user data, such as preferences, behavior, and demographics, resulting in increased customer satisfaction and engagement.
Predictive Analytics: Big data powers predictive analytics, allowing organizations to forecast future trends, behaviors, and outcomes. AI and ML models can make accurate predictions by analyzing historical data patterns, allowing businesses to optimize inventory management, anticipate customer demands, prevent fraud, and optimize marketing campaigns.
Automation and Efficiency: AI and ML powered by big data drive automation and efficiency across industries. From self-driving cars to smart manufacturing processes, these technologies use big data to learn from real-time data streams, optimize operations, and make quick decisions, resulting in increased productivity and cost savings.
Challenges and Considerations: While big data offers tremendous opportunities, it also poses significant challenges. Handling and processing massive amounts of data necessitates a strong infrastructure, scalable storage systems, and effective data management strategies. Furthermore, privacy, security, and ethical concerns about the use of big data must be addressed in order to maintain trust and compliance.
Conclusion:
Big data acts as the fuel that powers AI and ML by providing immense volumes of training data. With this abundant resource, AI algorithms gain the ability to learn, adapt, and deliver transformative insights and capabilities. From personalized experiences to predictive analytics and automation, big data unleashes the full potential of AI and ML, propelling us into a future where data-driven decision-making and innovation become the norm. Embracing the power of big data in conjunction with AI and ML is not only a competitive advantage but also a pathway to unlocking new frontiers of knowledge and possibilities.
Are you ready to explore the world of Big Data? Join CACMS today to realise your full potential! Enroll in our comprehensive Big Data course today and begin an exciting learning journey. Don't miss out on this fantastic opportunity; Sign up Now
#cacms institute#big data analytics#big data course#big data course in amritsar#big data training course#AI#Machine Learning#Training data#R#R Training#learn Big Data#sign up now
0 notes
Text
Mastering Big Data: A Comprehensive Guide to Online Learning - rudrasonline
Learning Big Data Courses Online– RudraOnline can be a rewarding endeavor, and there are numerous resources available. Here's a step-by-step guide to help you get started:
Understand the Basics:
Familiarize yourself with the basic concepts of big data, such as volume, velocity, variety, veracity, and value (the 5 V's).
Learn about distributed computing and parallel processing.
Programming Languages:
Gain proficiency in programming languages commonly used in big data processing, such as Python, Java, or Scala.
Foundational Technologies:
Learn the fundamentals of big data technologies like Apache Hadoop and Apache Spark. These technologies are widely used for distributed storage and processing.
Online Courses:
Explore online learning platforms that offer big data courses. Platforms like Coursera, edX, Udacity, and LinkedIn Learning provide courses from universities and industry experts.
Certifications:
Consider pursuing certifications in big data technologies. Certifications from vendors like Cloudera or Hortonworks can enhance your credibility.
Hands-on Practice:
Practice what you learn by working on real-world projects. Platforms like Kaggle provide datasets for hands-on experience.
Documentation and Tutorials:
Read official documentation and follow tutorials for big data technologies. This will help deepen your understanding and troubleshoot issues.
Books:
Refer to books on big data, such as "Hadoop: The Definitive Guide" by Tom White or "Spark: The Definitive Guide" by Bill Chambers and Matei Zaharia.
Community Involvement:
Join online forums and communities where big data professionals share knowledge and experiences. Participate in discussions and ask questions when needed.
Specialize:
Depending on your interests and career goals, consider specializing in specific areas within big data, such as data engineering, data science, or machine learning.
Advanced Topics:
Explore advanced topics like Apache Kafka for real-time data streaming or Apache Flink for stream processing.
Networking:
Attend webinars, conferences, and meetups related to big data. Networking with professionals in the field can provide valuable insights and potential job opportunities.
0 notes
Text
.
#probably going to put together a guide or something about shadowbans and terminations and all that (not so) fun stuff#today I learned that most people who've been terminated have not gotten confirmation emails from the support form#and the common thread is GET THIS using the email address on file for the terminated account#EXACTLY LIKE THE FORM TELLS YOU TO#so you have to use a different email if you want to be sure the ticket goes through#which isn't ideal for a variety of reasons#primarily i have to assume that their data security policies likely rely on the customer being able to verify their email address#I mean I don't know but that seems like a pretty big one#anyway I do (finally) for sure have a ticket in#but that would've been nice to know earlier in the week#also would've been nice to know about the VPN two months ago#but what's done is done
2 notes
·
View notes
Text
i was gonna say "i shouldn't have to go to work when my brain feels like a depression slushie" and then i was like "wait but then i'd basically never ever go to work" and i'm actually doubling down on the first part now bc my god how am i supposed to heal my brain from burning out 5 years ago if i can never get an actual break
#//juri speaks#i also at this moment: do not know if i have health insurance anymore / if i will be able to get insurance#if i can't get insurance i will not be able to take classes this fall#if i can't take classes my loan repayments will kick in immediately#i already don't have enough money for anything and i certainly don't have a spare $150 a month for the government#at any rate i need to submit my tuition waiver Soon but i can't until i know if i can get into the second class#so i have to wait for the prof or my advisor to get back to me#all the while a funeral day draws nearer#and then AT work i still feel like my position doesn't need to exist#but i desperately need it to exist because i need the money#and this big mchuge data migration project we were SUPPOSED to have had done in JUNE is being pushed to the absolute last minute#not by us but by the folks in control of the software we're moving to#so we're not going to have any safety margins with the old software#it's going to be GONE and dead and unlicensed while we're trying to learn the new shit#and i'm going to have to deal with the other branch cataloger trying to do everything for us which Won't Help#and i need!!!!!! a break!!!!!!!!!! from everything!!!!!#i need the world to stop and i need to go sit in the desert for like 6 months#instead best i can do is go buy the new taz gn for a little crumb of escape. maybe a little coffee drink while i'm there#even though i've been hitting sugar hard lately and really do not have the funds to buy more clothes if i gain a few more lbs#and can't afford a walking pad/treadmill and don't want to go outside bc it is a billion degrees all day every day rn#uuuuuuuuuuuugggggggggghhhhhhhhhhhhhhhhhhhhhhhhhh
10 notes
·
View notes
Text
How DeepSeek AI Revolutionizes Data Analysis
1. Introduction: The Data Analysis Crisis and AI’s Role2. What Is DeepSeek AI?3. Key Features of DeepSeek AI for Data Analysis4. How DeepSeek AI Outperforms Traditional Tools5. Real-World Applications Across Industries6. Step-by-Step: Implementing DeepSeek AI in Your Workflow7. FAQs About DeepSeek AI8. Conclusion 1. Introduction: The Data Analysis Crisis and AI’s Role Businesses today generate…
#AI automation trends#AI data analysis#AI for finance#AI in healthcare#AI-driven business intelligence#big data solutions#business intelligence trends#data-driven decisions#DeepSeek AI#ethical AI#ethical AI compliance#Future of AI#generative AI tools#machine learning applications#predictive modeling 2024#real-time analytics#retail AI optimization
3 notes
·
View notes
Text
Understanding Outliers in Machine Learning and Data Science
In machine learning and data science, an outlier is like a misfit in a dataset. It's a data point that stands out significantly from the rest of the data. Sometimes, these outliers are errors, while other times, they reveal something truly interesting about the data. Either way, handling outliers is a crucial step in the data preprocessing stage. If left unchecked, they can skew your analysis and even mess up your machine learning models.
In this article, we will dive into:
1. What outliers are and why they matter.
2. How to detect and remove outliers using the Interquartile Range (IQR) method.
3. Using the Z-score method for outlier detection and removal.
4. How the Percentile Method and Winsorization techniques can help handle outliers.
This guide will explain each method in simple terms with Python code examples so that even beginners can follow along.
1. What Are Outliers?
An outlier is a data point that lies far outside the range of most other values in your dataset. For example, in a list of incomes, most people might earn between $30,000 and $70,000, but someone earning $5,000,000 would be an outlier.
Why Are Outliers Important?
Outliers can be problematic or insightful:
Problematic Outliers: Errors in data entry, sensor faults, or sampling issues.
Insightful Outliers: They might indicate fraud, unusual trends, or new patterns.
Types of Outliers
1. Univariate Outliers: These are extreme values in a single variable.
Example: A temperature of 300°F in a dataset about room temperatures.
2. Multivariate Outliers: These involve unusual combinations of values in multiple variables.
Example: A person with an unusually high income but a very low age.
3. Contextual Outliers: These depend on the context.
Example: A high temperature in winter might be an outlier, but not in summer.
2. Outlier Detection and Removal Using the IQR Method
The Interquartile Range (IQR) method is one of the simplest ways to detect outliers. It works by identifying the middle 50% of your data and marking anything that falls far outside this range as an outlier.
Steps:
1. Calculate the 25th percentile (Q1) and 75th percentile (Q3) of your data.
2. Compute the IQR:
{IQR} = Q3 - Q1
Q1 - 1.5 \times \text{IQR}
Q3 + 1.5 \times \text{IQR} ] 4. Anything below the lower bound or above the upper bound is an outlier.
Python Example:
import pandas as pd
# Sample dataset
data = {'Values': [12, 14, 18, 22, 25, 28, 32, 95, 100]}
df = pd.DataFrame(data)
# Calculate Q1, Q3, and IQR
Q1 = df['Values'].quantile(0.25)
Q3 = df['Values'].quantile(0.75)
IQR = Q3 - Q1
# Define the bounds
lower_bound = Q1 - 1.5 * IQR
upper_bound = Q3 + 1.5 * IQR
# Identify and remove outliers
outliers = df[(df['Values'] < lower_bound) | (df['Values'] > upper_bound)]
print("Outliers:\n", outliers)
filtered_data = df[(df['Values'] >= lower_bound) & (df['Values'] <= upper_bound)]
print("Filtered Data:\n", filtered_data)
Key Points:
The IQR method is great for univariate datasets.
It works well when the data isn’t skewed or heavily distributed.
3. Outlier Detection and Removal Using the Z-Score Method
The Z-score method measures how far a data point is from the mean, in terms of standard deviations. If a Z-score is greater than a certain threshold (commonly 3 or -3), it is considered an outlier.
Formula:
Z = \frac{(X - \mu)}{\sigma}
is the data point,
is the mean of the dataset,
is the standard deviation.
Python Example:
import numpy as np
# Sample dataset
data = {'Values': [12, 14, 18, 22, 25, 28, 32, 95, 100]}
df = pd.DataFrame(data)
# Calculate mean and standard deviation
mean = df['Values'].mean()
std_dev = df['Values'].std()
# Compute Z-scores
df['Z-Score'] = (df['Values'] - mean) / std_dev
# Identify and remove outliers
threshold = 3
outliers = df[(df['Z-Score'] > threshold) | (df['Z-Score'] < -threshold)]
print("Outliers:\n", outliers)
filtered_data = df[(df['Z-Score'] <= threshold) & (df['Z-Score'] >= -threshold)]
print("Filtered Data:\n", filtered_data)
Key Points:
The Z-score method assumes the data follows a normal distribution.
It may not work well with skewed datasets.
4. Outlier Detection Using the Percentile Method and Winsorization
Percentile Method:
In the percentile method, we define a lower percentile (e.g., 1st percentile) and an upper percentile (e.g., 99th percentile). Any value outside this range is treated as an outlier.
Winsorization:
Winsorization is a technique where outliers are not removed but replaced with the nearest acceptable value.
Python Example:
from scipy.stats.mstats import winsorize
import numpy as np
Sample data
data = [12, 14, 18, 22, 25, 28, 32, 95, 100]
Calculate percentiles
lower_percentile = np.percentile(data, 1)
upper_percentile = np.percentile(data, 99)
Identify outliers
outliers = [x for x in data if x < lower_percentile or x > upper_percentile]
print("Outliers:", outliers)
# Apply Winsorization
winsorized_data = winsorize(data, limits=[0.01, 0.01])
print("Winsorized Data:", list(winsorized_data))
Key Points:
Percentile and Winsorization methods are useful for skewed data.
Winsorization is preferred when data integrity must be preserved.
Final Thoughts
Outliers can be tricky, but understanding how to detect and handle them is a key skill in machine learning and data science. Whether you use the IQR method, Z-score, or Wins
orization, always tailor your approach to the specific dataset you’re working with.
By mastering these techniques, you’ll be able to clean your data effectively and improve the accuracy of your models.
#science#skills#programming#bigdata#books#machinelearning#artificial intelligence#python#machine learning#data centers#outliers#big data#data analysis#data analytics#data scientist#database#datascience#data
4 notes
·
View notes
Text
discovering Where The Math Is in something confusing is always such an immense relief
#been trying to learn data analysis for MONTHS now and i only just got a good big picture understanding of What It Is lmao#like. okay. you have some data#you come up with a clearly-defined question. you clean your data#you decide what statistical test is appropriate to answer the question & appropriate for your data#and. CRUCIALLY. deciding which one is Appropriate isnt just some sort of witchcraft!! they all have assumptions built in#often because of how theyre calculated#THATS JUST MATH!!!! i know how to do that#''you can only apply this thing in specific circumstances'' thats a theorem basically. 90% of my coursework in uni was theorems and proofs#still need to practice actually DOING data analysis & im sure ill run into some problems but. hooray! i think i know how to do this!#personal#numbers do not lie
6 notes
·
View notes
Text
An update to the famous HOG map
#map#geography#agriculture#hog#pig farming#data visualization#mapsontheweb#nemfrog#my art#my maps#niche postin#I have arcgis pro on my work computer and i've been really enjoying making maps when its slow the past nine months#lobster's adventures#i think its an interesting comparison. makes the shift from small farms to industrial farms very obvious#that one random county in Utah there's a big smithfield company farm#its by county btw im pretty sure the original map is also by county. realistically its the smallest unit you can get data on#also learned that USDA lets farms ask for their data not to be public#so when I used just one year there were big gaps. so i had to backfill certain counties from the most recent year data was available
8 notes
·
View notes