#big data course
Explore tagged Tumblr posts
Text
#post graduate#data analytics#big data course#big data#big data analytics courses#data analytics courses
2 notes
·
View notes
Text
Master Big Data in Kochi: Transform Data into Real-Time Insights
Step into the future of data-driven decision making with our comprehensive Big Data course in Kochi. Designed for aspiring data professionals and tech enthusiasts, this program covers the full spectrum—from Hadoop and Spark to data lakes, real-time analytics, and machine learning integration. Learn to handle massive datasets, optimize storage, and extract actionable insights that drive business success.
Get hands-on training with real-world projects, industry-grade tools, and practical challenges that simulate the data environments of leading companies. Whether you're a fresher or a working professional looking to upgrade your skills, this course equips you with everything needed to thrive in a rapidly evolving data landscape.
Conclusion:This immersive learning experience is brought to you by Zoople Technologies, one of Kochi’s most trusted tech institutes, known for its expert faculty, personalized mentorship, and 100% placement assistance.
0 notes
Text
Big Data, Big Opportunities: A Beginner's Guide
Big Data is a current trend and the number of specialists in the field of Big Data is growing rapidly. If you are a beginner looking to enter the world of Big Data, you've come to the right place! This Beginner’s Guide will help you understand the basics of Big Data, Data Science, Data Analysis, and Data Engineering, and highlight the skills you need to build a career in this field.
What is Big Data?
Big Data refers to the massive volumes of structured and unstructured data that are too complex for traditional processing software. These Big Data concepts form the foundation for data professionals to extract valuable insights.
While the term might sound intimidating, think of Big Data as just a collection of data that's too large to be processed by conventional databases. Imagine the millions of transactions happening on Amazon or the vast amounts of data produced by a single flight from an airline. These are examples of Big Data in action. Learning the fundamentals will help you understand the potential of this massive resource
Why Big Data Matters
Big Data enables companies to uncover trends, improve decision-making, and gain a competitive edge. This demand has created a wealth of opportunities in Data Science careers, Data Analysis, and Data Engineering.
Key Big Data Concepts
Some key Big Data concepts include:
Volume, Velocity, and Variety: Large volume of data, generated rapidly in various formats.
Structured vs. Unstructured Data: Organized data in databases versus raw, unstructured data.
Tools like Hadoop and Spark are crucial in handling Big Data efficiently.
Data Engineering: The Backbone of Big Data
Data Engineering is the infrastructure behind Big Data. Data Engineering basics involve creating pipelines and processing systems to store and manage massive datasets. Learning these fundamentals is critical for those aspiring to Data Engineering jobs.
Big Data Applications Across Industries
Big Data applications span across industries, from healthcare and finance to marketing and manufacturing. In healthcare, Big Data is used for predictive analytics and improving patient care. In finance, it helps detect fraud, optimize investment strategies, and manage risks. Marketing teams use Big Data to understand customer preferences, personalize experiences, and create targeted campaigns. The possibilities are endless, making Big Data one of the most exciting fields to be a part of today.
As a beginner, you might wonder how Big Data fits into everyday life. Think of online streaming services like Netflix, which recommend shows based on your previous viewing patterns, or retailers who send personalized offers based on your shopping habits. These are just a couple of ways Big Data is being applied in the real world.
Building a Career in Big Data
The demand for Big Data professionals is on the rise, and there are a variety of career paths you can choose from:
Data Science Career: As a Data Scientist, you'll focus on predictive modeling, machine learning, and advanced analytics. This career often involves a strong background in mathematics, statistics, and coding.
Data Analysis Jobs: As a Data Analyst, you’ll extract meaningful insights from data to support business decisions. This role emphasizes skills in statistics, communication, and data visualization.
Data Engineering Jobs: As a Data Engineer, you’ll build the infrastructure that supports data processing and analysis, working closely with Data Scientists and Analysts to ensure that data is clean and ready for use.
Whether you're interested in Data Science, Data Analysis, or Data Engineering, now is the perfect time to jumpstart your career. Each role has its own unique challenges and rewards, so finding the right fit will depend on your strengths and interests.
Career Opportunities in Big Data and Their Salaries
As the importance of Big Data continues to grow, so does the demand for professionals skilled in handling large data sets. Let’s check the different career paths in Big Data, their responsibilities, and average salaries:
Data Scientist
Role: Data Scientists develop models and algorithms to extract insights from large data sets. They work on predictive analytics, machine learning, and statistical modeling.
Average Salary: $120,000 to $150,000 per year in the U.S.
Skills Needed: Strong background in math, statistics, programming (Python, R), and machine learning.
Data Analyst
Role: Data Analysts interpret data to provide actionable insights for decision-making. They focus on generating reports, dashboards, and business insights.
Average Salary: $60,000 to $90,000 per year in the U.S.
Skills Needed: Proficiency in SQL, Excel, Python, data visualization tools like Tableau or Power BI, and statistical analysis.
Data Engineer
Role: Data Engineers build and maintain the architecture (databases, pipelines, etc.) necessary for data collection, storage, and analysis.
Average Salary: $100,000 to $140,000 per year in the U.S.
Skills Needed: Knowledge of cloud platforms (AWS, Google Cloud), database management, ETL tools, and programming languages like Python, Scala, or Java.
Big Data Architect
Role: Big Data Architects design the infrastructure that supports Big Data solutions, ensuring scalability and performance.
Average Salary: $140,000 to $180,000 per year in the U.S.
Skills Needed: Expertise in cloud computing, distributed systems, database architecture, and technologies like Hadoop, Spark, and Kafka.
Machine Learning Engineer
Role: Machine Learning Engineers create algorithms that allow systems to automatically improve from experience, which is key in processing and analyzing large data sets.
Average Salary: $110,000 to $160,000 per year in the U.S.
Skills Needed: Proficiency in machine learning libraries (TensorFlow, PyTorch), programming (Python, R), and experience with large datasets.
Learn Big Data with Guruface
Guruface, an online learning platform, offers different Big Data courses. Whether you’re looking for an Introduction to Big Data, a Data Science tutorial, or Data Engineering basics, Guruface provides beginner-friendly resources to guide your learning. Their courses are ideal for those looking to learn Big Data concepts and practical applications in Data Science, Data Analysis, and Data Engineering.
Conclusion
With data being the driving force in today’s society, understanding the Big Data concepts, tools as well as applications, is a key step towards an exciting Big Data Career. Platforms like Guruface provide the ideal starting point for beginners interested in Big Data, Data Science, Data Analysis, or Data Engineering. Start your journey today and explore the vast potential of Big Data.
0 notes
Text
The Importance of Data Analytics for Better Business Decision Making
In today's business context, data has emerged as a critical asset for strategic decision-making processes. With the development of digital technologies and the rise of big data, organizations are increasingly turning to data analytics to gain actionable insights. This article investigates the revolutionary role of data analytics in business decision making, including its relevance, benefits, and limitations.
The significance of data analytics:
Data analytics is the methodical analysis of large databases to discover useful patterns, trends, and correlations. Organizations can extract important insights from heterogeneous data sources by using advanced statistical algorithms, machine learning approaches, and data visualization technologies. These insights serve as the cornerstone for educated decision-making in a variety of fields, including marketing, finance, operations, and customer service.
Enhancing Decision Making using Data Analytics:
Data analytics enables organizations to make data-driven decisions based on factual facts rather than intuition or hypothesis. Businesses may better predict industry trends, client preferences, and upcoming possibilities by analyzing historical data and real-time information. This allows them to better allocate resources, manage risks, and capitalize on competitive advantages.
Furthermore, data analytics enables scenario analysis and predictive modeling, allowing businesses to forecast future events and assess various courses of action. Whether it's anticipating sales performance, optimizing pricing tactics, or finding operational inefficiencies, data-driven insights help decision makers develop plans that are aligned with organizational goals.
Advantages of Data-Driven Decision Making:
Adoption of data analytics provides numerous benefits for firms looking to acquire a competitive advantage in today's dynamic industry. This includes:
Improved Accuracy: Data analytics allows organizations to base their decisions on empirical evidence, minimizing the possibility of errors or biases that come with subjective decision-making processes.
Improved Efficiency: By automating data analysis processes and streamlining decision-making workflows, firms can increase operational efficiency and resource utilization.
Enhanced Strategic Insights: Data analytics offers deeper insights into market dynamics, customer behavior, and competitive landscapes, allowing organizations to develop more successful strategic plans and initiatives.
Agility and Adaptability: Real-time analytics enable firms to respond swiftly to changing market conditions, new trends, and customer preferences, fostering agility and adaptability in decision making.
Challenges and Considerations: Data analytics offer significant benefits, but implementation can be challenging. Organizations must deal with problems such as data quality, privacy concerns, talent shortages, and technological difficulties. Furthermore, in the age of data-driven decision making, it is vital to ensure ethical data use and compliance with regulatory frameworks.
Conclusion:
In conclusion, data analytics has developed as a critical component of modern corporate decision making, providing organizations with unmatched insights into their operations, customers, and markets. Businesses that leverage the power of data analytics can open new opportunities, manage risks, and gain a competitive advantage in today's fast changing landscape. However, success in exploiting data analytics is dependent not only on technology skills, but also on organizational culture, talent development, and ethical concerns. As businesses embrace data-driven decision making, the revolutionary power of data analytics will continue to redefine industries and drive innovation.
Are you ready to unleash the potential of data analytics for your business? Join CACMS Institute for a thorough data analytics training today! Our hands-on practical training, conducted by qualified instructors, will provide you with the skills and information required to succeed in the field of data analytics.
Why should you choose CACMS Institute?
hands-on practical training
Expert teaching staff
Flexible timings to accommodate your schedule
Enroll now and take the first step towards understanding data analytics. For further information, please contact us at +91 8288040281 or visit http://cacms.in/big-data/ Don't pass up this opportunity to boost your career. Enroll in Amritsar's finest data analytics courses today!
#cacms institute#cacms#techskills#techeducation#data analytics training#data analytics#data analytics course#big data course#learn big data#data analyst course#data analyst training#best computer institute near me#best computer institute in Amritsar
0 notes
Text
i liked the little data and spock exchange in unification and it made sense what w their opposite directions wrt desire to associate with 'humanity'. that said i think worf is a more direct parallel to spock in that respect
#p#tng#identity is at play for data of course! but culture/nation arent and theyre A Big Fucking Deal for the other two#they share the experience of being surrounded by humans and prob the only individual of their species their colleagues had met#And of having tenuous/difficult/painful relationships to their place and emphasizing their ~foreignness to bait their colleagues#into reifying/validating that identity through. well through xenophobia#harder to get a three minute conversation out of that then out of data's frank questions though lol#spock#worf#data
14 notes
·
View notes
Text
I have like. Three friends in school I guess. The Sri Lankan girl and white guy from my program and that one brown guy who's not in my program but is in some of my classes. I think I might be befriending those two DS undergrads, that guy who sat next to me yesterday and the older retired gay man with the delightful southern accent. And there's the Chinese girl who's my partner for my project in database systems who's not even from the comp sci department (she's from math which is way cooler). I think I don't need to be nervous.
#T#I am fighting the urge to take a fifth class I know it will end in failure#But I saw the online ML class had an open seat#And my friend is in that class#I also thought about staying in Big Data programming bc the guy from my program is in that class#But I think it would be better to take after I take these other courses
1 note
·
View note
Text
What are some challenging concepts for beginners learning data science, such as statistics and machine learning?
Hi,
For beginners in data science, several concepts can be challenging due to their complexity and depth.
Here are some of the most common challenging concepts in statistics and machine learning:
Statistics:
Probability Distributions: Understanding different probability distributions (e.g., normal, binomial, Poisson) and their properties can be difficult. Knowing when and how to apply each distribution requires a deep understanding of their characteristics and applications.
Hypothesis Testing: Hypothesis testing involves formulating null and alternative hypotheses, selecting appropriate tests (e.g., t-tests, chi-square tests), and interpreting p-values. The concepts of statistical significance and Type I/Type II errors can be complex and require careful consideration.
Confidence Intervals: Calculating and interpreting confidence intervals for estimates involves understanding the trade-offs between precision and reliability. Beginners often struggle with the concept of confidence intervals and their implications for statistical inference.
Regression Analysis: Multiple regression analysis, including understanding coefficients, multicollinearity, and model assumptions, can be challenging. Interpreting regression results and diagnosing issues such as heteroscedasticity and autocorrelation require a solid grasp of statistical principles.
Machine Learning:
Bias-Variance Tradeoff: Balancing bias and variance to achieve a model that generalizes well to new data can be challenging. Understanding overfitting and underfitting, and how to use techniques like cross-validation to address these issues, requires careful analysis.
Feature Selection and Engineering: Selecting the most relevant features and engineering new ones can significantly impact model performance. Beginners often find it challenging to determine which features are important and how to transform raw data into useful features.
Algorithm Selection and Tuning: Choosing the appropriate machine learning algorithm for a given problem and tuning its hyperparameters can be complex. Each algorithm has its own strengths, limitations, and parameters that need to be optimized.
Model Evaluation Metrics: Understanding and selecting the right evaluation metrics (e.g., accuracy, precision, recall, F1 score) for different types of models and problems can be challenging.
Advanced Topics:
Deep Learning: Concepts such as neural networks, activation functions, backpropagation, and hyperparameter tuning in deep learning can be intricate. Understanding how deep learning models work and how to optimize them requires a solid foundation in both theoretical and practical aspects.
Dimensionality Reduction: Techniques like Principal Component Analysis (PCA) and t-Distributed Stochastic Neighbor Embedding (t-SNE) for reducing the number of features while retaining essential information can be difficult to grasp and apply effectively.
To overcome these challenges, beginners should focus on building a strong foundation in fundamental concepts through practical exercises, online courses, and hands-on projects. Seeking clarification from mentors or peers and engaging in data science communities can also provide valuable support and insights.
#bootcamp#data science course#datascience#data analytics#machinelearning#big data#ai#data privacy#python
3 notes
·
View notes
Text
#Business Analytics#Colleges in India#Data Analytics#Top Colleges in India#Business Analytics Courses#Management#Colleges for Business Analytics#Big Data Analytics#Management Programs in India#Data Visualization
2 notes
·
View notes
Text
MIT researchers discover the universe’s oldest stars in our own galactic backyard
New Post has been published on https://thedigitalinsider.com/mit-researchers-discover-the-universes-oldest-stars-in-our-own-galactic-backyard/
MIT researchers discover the universe’s oldest stars in our own galactic backyard
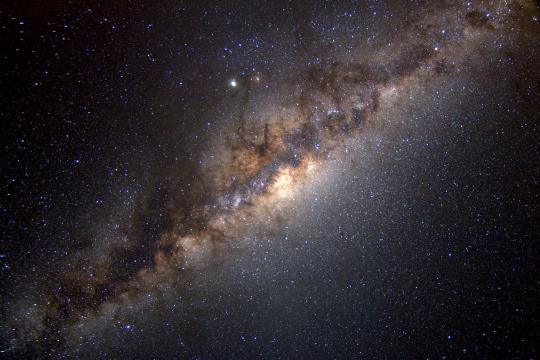
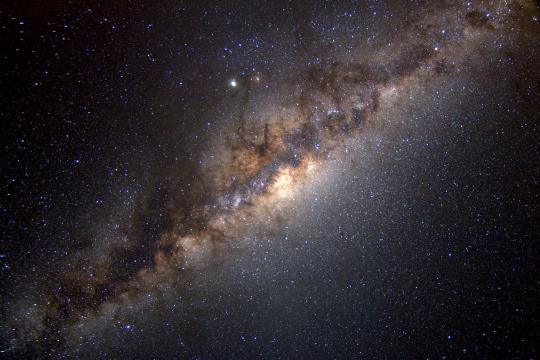
MIT researchers, including several undergraduate students, have discovered three of the oldest stars in the universe, and they happen to live in our own galactic neighborhood.
The team spotted the stars in the Milky Way’s “halo” — the cloud of stars that envelopes the entire main galactic disk. Based on the team’s analysis, the three stars formed between 12 and 13 billion years ago, the time when the very first galaxies were taking shape.
The researchers have coined the stars “SASS,” for Small Accreted Stellar System stars, as they believe each star once belonged to its own small, primitive galaxy that was later absorbed by the larger but still growing Milky Way. Today, the three stars are all that are left of their respective galaxies. They circle the outskirts of the Milky Way, where the team suspects there may be more such ancient stellar survivors.
“These oldest stars should definitely be there, given what we know of galaxy formation,” says MIT professor of physics Anna Frebel. “They are part of our cosmic family tree. And we now have a new way to find them.”
As they uncover similar SASS stars, the researchers hope to use them as analogs of ultrafaint dwarf galaxies, which are thought to be some of the universe’s surviving first galaxies. Such galaxies are still intact today but are too distant and faint for astronomers to study in depth. As SASS stars may have once belonged to similarly primitive dwarf galaxies but are in the Milky Way and as such much closer, they could be an accessible key to understanding the evolution of ultrafaint dwarf galaxies.
“Now we can look for more analogs in the Milky Way, that are much brighter, and study their chemical evolution without having to chase these extremely faint stars,” Frebel says.
She and her colleagues have published their findings today in the Monthly Notices of the Royal Astronomical Society (MNRAS). The study’s co-authors are Mohammad Mardini, at Zarqa University, in Jordan; Hillary Andales ’23; and current MIT undergraduates Ananda Santos and Casey Fienberg.
Stellar frontier
The team’s discoveries grew out of a classroom concept. During the 2022 fall semester, Frebel launched a new course, 8.S30 (Observational Stellar Archaeology), in which students learned techniques for analyzing ancient stars and then applied those tools to stars that had never been studied before, to determine their origins.
“While most of our classes are taught from the ground up, this class immediately put us at the frontier of research in astrophysics,” Andales says.
The students worked from star data collected by Frebel over the years from the 6.5-meter Magellan-Clay telescope at the Las Campanas Observatory. She keeps hard copies of the data in a large binder in her office, which the students combed through to look for stars of interest.
In particular, they were searching ancient stars that formed soon after the Big Bang, which occurred 13.8 billion years ago. At this time, the universe was made mostly of hydrogen and helium and very low abundances of other chemical elements, such as strontium and barium. So, the students looked through Frebel’s binder for stars with spectra, or measurements of starlight, that indicated low abundances of strontium and barium.
Their search narrowed in on three stars that were originally observed by the Magellan telescope between 2013 and 2014. Astronomers never followed up on these particular stars to interpret their spectra and deduce their origins. They were, then, perfect candidates for the students in Frebel’s class.
The students learned how to characterize a star in order to prepare for the analysis of the spectra for each of the three stars. They were able to determine the chemical composition of each one with various stellar models. The intensity of a particular feature in the stellar spectrum, corresponding to a specific wavelength of light, corresponds to a particular abundance of a specific element.
After finalizing their analysis, the students were able to confidently conclude that the three stars did hold very low abundances of strontium, barium, and other elements such as iron, compared to their reference star — our own sun. In fact, one star contained less than 1/10,000 the amount of iron to helium compared to the sun today.
“It took a lot of hours staring at a computer, and a lot of debugging, frantically texting and emailing each other to figure this out,” Santos recalls. “It was a big learning curve, and a special experience.”
“On the run”
The stars’ low chemical abundance did hint that they originally formed 12 to 13 billion years ago. In fact, their low chemical signatures were similar to what astronomers had previously measured for some ancient, ultrafaint dwarf galaxies. Did the team’s stars originate in similar galaxies? And how did they come to be in the Milky Way?
On a hunch, the scientists checked out the stars’ orbital patterns and how they move across the sky. The three stars are in different locations throughout the Milky Way’s halo and are estimated to be about 30,000 light years from Earth. (For reference, the disk of the Milky Way spans 100,000 light years across.)
As they retraced each star’s motion about the galactic center using observations from the Gaia astrometric satellite, the team noticed a curious thing: Relative to most of the stars in the main disk, which move like cars on a racetrack, all three stars seemed to be going the wrong way. In astronomy, this is known as “retrograde motion” and is a tipoff that an object was once “accreted,” or drawn in from elsewhere.
“The only way you can have stars going the wrong way from the rest of the gang is if you threw them in the wrong way,” Frebel says.
The fact that these three stars were orbiting in completely different ways from the rest of the galactic disk and even the halo, combined with the fact that they held low chemical abundances, made a strong case that the stars were indeed ancient and once belonged to older, smaller dwarf galaxies that fell into the Milky Way at random angles and continued their stubborn trajectories billions of years later.
Frebel, curious as to whether retrograde motion was a feature of other ancient stars in the halo that astronomers previously analyzed, looked through the scientific literature and found 65 other stars, also with low strontium and barium abundances, that appeared to also be going against the galactic flow.
“Interestingly they’re all quite fast — hundreds of kilometers per second, going the wrong way,” Frebel says. “They’re on the run! We don’t know why that’s the case, but it was the piece to the puzzle that we needed, and that I didn’t quite anticipate when we started.”
The team is eager to search out other ancient SASS stars, and they now have a relatively simple recipe to do so: First, look for stars with low chemical abundances, and then track their orbital patterns for signs of retrograde motion. Of the more than 400 billion stars in the Milky Way, they anticipate that the method will turn up a small but significant number of the universe’s oldest stars.
Frebel plans to relaunch the class this fall, and looks back at that first course, and the three students who took their results through to publication, with admiration and gratitude.
“It’s been awesome to work with three women undergrads. That’s a first for me,” she says. “It’s really an example of the MIT way. We do. And whoever says, ‘I want to participate,’ they can do that, and good things happen.”
This research was supported, in part, by the National Science Foundation.
#000#2022#Analysis#archaeology#Astronomy#Astrophysics#big bang#billion#Cars#chemical#chemical elements#chemistry#classes#Cloud#Composition#computer#course#data#Discoveries#dwarf#dwarf galaxies#earth#Evolution#Foundation#Gaia#galaxies#Galaxy#galaxy formation#helium#how
3 notes
·
View notes
Text
Seedling is such an interesting little story that i love, but i´d be lying if it wasnt almost completely sprouted (hehe) from the visual of those character duos that are a small child + big scary looking creature who acts as their guard, top tier trope frfr
(also huge shoutout to @ashrayus for hearing me out and adding onto the rambles so long ago, bestie the robot story wasnt working, we got plants now 🌱)
#but also said big scary creature is her brother having an identity crisis and being chased by the horrors :D#the story is 50% strangers to (step) siblings that trust each other with their life#and 50% truths of the world conspiracy with a witch hunt on plant people + aliens doing their thing (of course)#bruhh bc what if there were aliens replacing people at a young age and using them to collect data on the overall population#and “harvesting” them when said purpose was fulfilled#thing is; when the harvesting happens its supposed to be like a switch is flipped; and the seedling just up and leaves to be collected#*but* an infinite amount of things can make this process fail; which happened with the mc; who now clings to his family like a lifeline#while also knowing the general population´s view on seedlings is abyssmal#<- even if the family has no porblem w it; government or a bad neighbour catches you? you´re *gone*#anyways; fun times for lil Vera hshshahsha#seedling#oc talk#shut up sheo#edit: hey ash remember Riven? ik hes your oc can i work with thet concept perhaps?#his color scheme and character role work soooo well!!!!!#planning on making him be one of the few who escaped the harvest but could never quite shake the switch flip
3 notes
·
View notes
Text
Big Data, Big Opportunities: A Beginner's Guide
Big Data is a current trend and the number of specialists in the field of Big Data is growing rapidly. If you are a beginner looking to enter the world of Big Data, you've come to the right place! This Beginner’s Guide will help you understand the basics of Big Data, Data Science, Data Analysis, and Data Engineering, and highlight the skills you need to build a career in this field.
What is Big Data?
Big Data refers to the massive volumes of structured and unstructured data that are too complex for traditional processing software. These Big Data concepts form the foundation for data professionals to extract valuable insights.
While the term might sound intimidating, think of Big Data as just a collection of data that's too large to be processed by conventional databases. Imagine the millions of transactions happening on Amazon or the vast amounts of data produced by a single flight from an airline. These are examples of Big Data in action. Learning the fundamentals will help you understand the potential of this massive resource
Why Big Data Matters
Big Data enables companies to uncover trends, improve decision-making, and gain a competitive edge. This demand has created a wealth of opportunities in Data Science careers, Data Analysis, and Data Engineering.
Key Big Data Concepts
Some key Big Data concepts include:
Volume, Velocity, and Variety: Large volume of data, generated rapidly in various formats.
Structured vs. Unstructured Data: Organized data in databases versus raw, unstructured data.
Tools like Hadoop and Spark are crucial in handling Big Data efficiently.
Data Engineering: The Backbone of Big Data
Data Engineering is the infrastructure behind Big Data. Data Engineering basics involve creating pipelines and processing systems to store and manage massive datasets. Learning these fundamentals is critical for those aspiring to Data Engineering jobs.
Big Data Applications Across Industries
Big Data applications span across industries, from healthcare and finance to marketing and manufacturing. In healthcare, Big Data is used for predictive analytics and improving patient care. In finance, it helps detect fraud, optimize investment strategies, and manage risks. Marketing teams use Big Data to understand customer preferences, personalize experiences, and create targeted campaigns. The possibilities are endless, making Big Data one of the most exciting fields to be a part of today.
As a beginner, you might wonder how Big Data fits into everyday life. Think of online streaming services like Netflix, which recommend shows based on your previous viewing patterns, or retailers who send personalized offers based on your shopping habits. These are just a couple of ways Big Data is being applied in the real world.
Building a Career in Big Data
The demand for Big Data professionals is on the rise, and there are a variety of career paths you can choose from:
Data Science Career: As a Data Scientist, you'll focus on predictive modeling, machine learning, and advanced analytics. This career often involves a strong background in mathematics, statistics, and coding.
Data Analysis Jobs: As a Data Analyst, you’ll extract meaningful insights from data to support business decisions. This role emphasizes skills in statistics, communication, and data visualization.
Data Engineering Jobs: As a Data Engineer, you’ll build the infrastructure that supports data processing and analysis, working closely with Data Scientists and Analysts to ensure that data is clean and ready for use.
Whether you're interested in Data Science, Data Analysis, or Data Engineering, now is the perfect time to jumpstart your career. Each role has its own unique challenges and rewards, so finding the right fit will depend on your strengths and interests.
Career Opportunities in Big Data and Their Salaries
As the importance of Big Data continues to grow, so does the demand for professionals skilled in handling large data sets. Let’s check the different career paths in Big Data, their responsibilities, and average salaries:
Data Scientist
Role: Data Scientists develop models and algorithms to extract insights from large data sets. They work on predictive analytics, machine learning, and statistical modeling.
Average Salary: $120,000 to $150,000 per year in the U.S.
Skills Needed: Strong background in math, statistics, programming (Python, R), and machine learning.
Data Analyst
Role: Data Analysts interpret data to provide actionable insights for decision-making. They focus on generating reports, dashboards, and business insights.
Average Salary: $60,000 to $90,000 per year in the U.S.
Skills Needed: Proficiency in SQL, Excel, Python, data visualization tools like Tableau or Power BI, and statistical analysis.
Data Engineer
Role: Data Engineers build and maintain the architecture (databases, pipelines, etc.) necessary for data collection, storage, and analysis.
Average Salary: $100,000 to $140,000 per year in the U.S.
Skills Needed: Knowledge of cloud platforms (AWS, Google Cloud), database management, ETL tools, and programming languages like Python, Scala, or Java.
Big Data Architect
Role: Big Data Architects design the infrastructure that supports Big Data solutions, ensuring scalability and performance.
Average Salary: $140,000 to $180,000 per year in the U.S.
Skills Needed: Expertise in cloud computing, distributed systems, database architecture, and technologies like Hadoop, Spark, and Kafka.
Machine Learning Engineer
Role: Machine Learning Engineers create algorithms that allow systems to automatically improve from experience, which is key in processing and analyzing large data sets.
Average Salary: $110,000 to $160,000 per year in the U.S.
Skills Needed: Proficiency in machine learning libraries (TensorFlow, PyTorch), programming (Python, R), and experience with large datasets.
Learn Big Data with Guruface
Guruface, an online learning platform, offers different Big Data courses. Whether you’re looking for an Introduction to Big Data, a Data Science tutorial, or Data Engineering basics, Guruface provides beginner-friendly resources to guide your learning. Their courses are ideal for those looking to learn Big Data concepts and practical applications in Data Science, Data Analysis, and Data Engineering.
Conclusion
With data being the driving force in today’s society, understanding the Big Data concepts, tools as well as applications, is a key step towards an exciting Big Data Career. Platforms like Guruface provide the ideal starting point for beginners interested in Big Data, Data Science, Data Analysis, or Data Engineering. Start your journey today and explore the vast potential of Big Data.
0 notes
Text
*leaves a comment on every single chapter of a fic as I read it* *author replies to every single comment with increasing giddiness and excitement*
This is good. I’m getting a good grade in fic reader. This is both something that is normal to want and possible to achieve.
#ra speaks#personal#fanfiction#fanfic#ao3#archive of our own#I’m not going to lie: then replying incentivizes me to keep commenting#and like I get it Big Fics make it unrealistic for an author to reply to every comment#but boy that email gives me the same dopamine rush as the emails abt ppl leaving comments on MY fics#who gives a shit of ‘oh but I don’t want to inflate my comment count - ‘ shut up and let me kiss you. with tongue.#or bake you your favorite kind of muffin if it’s best we keep this professional/platonic#in other news: o7 survived my first day as a TA. I have class in a few hours for my data science course and it probably won’t kill me.#probably.
10 notes
·
View notes
Text
"Unleashing the Power of Big Data: Fueling AI and Machine Learning with Massive Amounts of Training Data"
Introduction:
The symbiotic relationship between big data, artificial intelligence (AI), and machine learning (ML) has become the catalyst for groundbreaking advancements in today's data-driven world. Big data, which consists of massive amounts of diverse and complex information, is the fuel that propels AI and ML forward. Big data enables AI algorithms to learn, adapt, and make intelligent decisions by providing the necessary training data. In this article, we will look at how big data acts as a fuel for AI and ML, allowing them to reach their full potential.
The Importance of Training Data: At the heart of AI and ML is the concept of training data. These algorithms learn from patterns and examples, and their performance improves as they are exposed to more relevant data. Big data plays an important role in training AI and ML models by providing massive amounts of training data. With such a wealth of data at their disposal, AI algorithms gain a broader understanding of the problem space and become more capable of making accurate predictions and informed decisions.
Unleashing the Potential of Big Data:
Unprecedented Insights: Big data provides an unprecedented opportunity to extract valuable insights. AI and ML algorithms can uncover hidden patterns, correlations, and trends in massive amounts of structured and unstructured data by analyzing massive amounts of structured and unstructured data. This enables businesses and organizations to make data-driven decisions, optimize processes, and gain a competitive advantage.
Enhanced Personalization: Using big data, AI and ML models can personalize user experiences by providing tailored recommendations, targeted advertisements, and customized services. These algorithms can deliver personalized and relevant content by analyzing vast amounts of user data, such as preferences, behavior, and demographics, resulting in increased customer satisfaction and engagement.
Predictive Analytics: Big data powers predictive analytics, allowing organizations to forecast future trends, behaviors, and outcomes. AI and ML models can make accurate predictions by analyzing historical data patterns, allowing businesses to optimize inventory management, anticipate customer demands, prevent fraud, and optimize marketing campaigns.
Automation and Efficiency: AI and ML powered by big data drive automation and efficiency across industries. From self-driving cars to smart manufacturing processes, these technologies use big data to learn from real-time data streams, optimize operations, and make quick decisions, resulting in increased productivity and cost savings.
Challenges and Considerations: While big data offers tremendous opportunities, it also poses significant challenges. Handling and processing massive amounts of data necessitates a strong infrastructure, scalable storage systems, and effective data management strategies. Furthermore, privacy, security, and ethical concerns about the use of big data must be addressed in order to maintain trust and compliance.
Conclusion:
Big data acts as the fuel that powers AI and ML by providing immense volumes of training data. With this abundant resource, AI algorithms gain the ability to learn, adapt, and deliver transformative insights and capabilities. From personalized experiences to predictive analytics and automation, big data unleashes the full potential of AI and ML, propelling us into a future where data-driven decision-making and innovation become the norm. Embracing the power of big data in conjunction with AI and ML is not only a competitive advantage but also a pathway to unlocking new frontiers of knowledge and possibilities.
Are you ready to explore the world of Big Data? Join CACMS today to realise your full potential! Enroll in our comprehensive Big Data course today and begin an exciting learning journey. Don't miss out on this fantastic opportunity; Sign up Now
#cacms institute#big data analytics#big data course#big data course in amritsar#big data training course#AI#Machine Learning#Training data#R#R Training#learn Big Data#sign up now
0 notes
Text
Navigating the Data Landscape: A Deep Dive into ScholarNest's Corporate Training
In the ever-evolving realm of data, mastering the intricacies of data engineering and PySpark is paramount for professionals seeking a competitive edge. ScholarNest's Corporate Training offers an immersive experience, providing a deep dive into the dynamic world of data engineering and PySpark.
Unlocking Data Engineering Excellence
Embark on a journey to become a proficient data engineer with ScholarNest's specialized courses. Our Data Engineering Certification program is meticulously crafted to equip you with the skills needed to design, build, and maintain scalable data systems. From understanding data architecture to implementing robust solutions, our curriculum covers the entire spectrum of data engineering.
Pioneering PySpark Proficiency
Navigate the complexities of data processing with PySpark, a powerful Apache Spark library. ScholarNest's PySpark course, hailed as one of the best online, caters to both beginners and advanced learners. Explore the full potential of PySpark through hands-on projects, gaining practical insights that can be applied directly in real-world scenarios.
Azure Databricks Mastery
As part of our commitment to offering the best, our courses delve into Azure Databricks learning. Azure Databricks, seamlessly integrated with Azure services, is a pivotal tool in the modern data landscape. ScholarNest ensures that you not only understand its functionalities but also leverage it effectively to solve complex data challenges.
Tailored for Corporate Success
ScholarNest's Corporate Training goes beyond generic courses. We tailor our programs to meet the specific needs of corporate environments, ensuring that the skills acquired align with industry demands. Whether you are aiming for data engineering excellence or mastering PySpark, our courses provide a roadmap for success.
Why Choose ScholarNest?
Best PySpark Course Online: Our PySpark courses are recognized for their quality and depth.
Expert Instructors: Learn from industry professionals with hands-on experience.
Comprehensive Curriculum: Covering everything from fundamentals to advanced techniques.
Real-world Application: Practical projects and case studies for hands-on experience.
Flexibility: Choose courses that suit your level, from beginner to advanced.
Navigate the data landscape with confidence through ScholarNest's Corporate Training. Enrol now to embark on a learning journey that not only enhances your skills but also propels your career forward in the rapidly evolving field of data engineering and PySpark.
#data engineering#pyspark#databricks#azure data engineer training#apache spark#databricks cloud#big data#dataanalytics#data engineer#pyspark course#databricks course training#pyspark training
3 notes
·
View notes
Text
my early childhood education class is really driving home the developmental in developmental disability.... like i knew thats what adhd is classified as but they just keep dropping skill after skill that are standard for Small Children that i do not have D:
#attention span. is of course the one that is most noted but even like#theres that one adhd sway thing and i was like huh i do that guess theres another data point. not a big deal though it doesnt cause any iss#es#right. well. apparently one of the skills youre supposed to get is spatial awareness. and it specifically mentioned going around things#which technically ! i do but also sometimes. quite often even#i do not manage fully around. meanwhile its expected for Small Children to get able to do that. huh what HUH#beeep#like again not getting fully around isnt a Big deal i just get caught on doors and then uncatch myself its not Disabling like other aspects#and if i hadnt seen the youtube (the youtube referencing a study not just a youtube)#i wouldve kept on assuming its just a itty bitty side effect of weird eye#but like......... man. in nearly every area i was like wow i suck at this one wow i suck at this one w#its a good thing im an adult algebra is much easier than counting to one hundred . u can write it down and when u lose your place u just#look at the paper#although i havent gotten algebra in a while i miss it............ but i could go do algebra. if i had time. and thats what is nice#anywayyyyyyy ahaha
3 notes
·
View notes