#machinelearningmodels
Explore tagged Tumblr posts
Text
Predictive vs Prescriptive vs Descriptive Analytics Explained
Business analytics leveraging data patterns for strategic moves comes in three key approaches – descriptive identifying “what has occurred", predictive forecasting “what could occur” and prescriptive recommending “what should occur” to optimize decisions. We decode the science behind each for aspiring analytics professionals.
Descriptive analytics convert volumes of historical data into insightful summaries around metrics revealing business health, customer trends, operational efficiencies etc. using direct analysis, aggregation and mining techniques producing current reports.
Predictive analytics forecast unknown future probabilities applying statistical, econometric and machine learning models over existing data to minimize uncertainties and capture emerging behaviors early for mitigation actions. Risk models simulate scenarios balancing upside/downside tradeoffs.
Prescriptive analytics take guidance one step further by dynamically recommending best decision options factoring in key performance indicators for business objective improvements after predicting multiple futures using bell curve simulations. Optimization algorithms deliver preferred actions.
While foundational data comprehension and wrangling abilities fuel all models – pursuing analytics specializations focused on statistical, computational or operational excellence boosts career-readiness filling different priorities global employers seek!
Posted By:
Aditi Borade, 4th year Barch,
Ls Raheja School of architecture
Disclaimer: The perspectives shared in this blog are not intended to be prescriptive. They should act merely as viewpoints to aid overseas aspirants with helpful guidance. Readers are encouraged to conduct their own research before availing the services of a consultant.
#analytics#types#predictive#prescriptive#descriptive#PrescriptiveAnalytics#StrategicMoves#AnalyticsProfessionals#DataScience#HistoricalData#Metrics#BusinessHealth#CustomerTrends#OperationalEfficiencies#StatisticalModels#EconometricModels#MachineLearningModels#EnvoyOverseas#EthicalCounselling#EnvoyInternationalStudents#EnvoyCounselling
4 notes
·
View notes
Text
Accelerate AI Training with Quality Data

Speed up your AI development with the perfect training data. Our data labeling services are designed to meet the needs of your machine learning models—boosting performance and ensuring reliability. Trust us to provide the data that fuels your AI.
0 notes
Text
Machine Learning Yearning" is a practical guide by Andrew Ng, a pioneer in the field of artificial intelligence and machine learning. This book is part of the deeplearning.ai project and is designed to help you navigate the complexities of building and deploying machine learning systems. It focuses on strategic decision-making and best practices rather than algorithms or code. Below is a step-by-step breakdown of the outcomes you can expect after reading this book, presented in a user-friendly manner:
#MachineLearning#DeepLearning#AI#ArtificialIntelligence#ML#DeepLearningAI#MLYearning#AndrewNg#AIProject#DataScience#MachineLearningBooks#NeuralNetworks#AICommunity#TechBooks#AIResearch#AITraining#MLModels#DeepLearningTutorials#AIApplications#MLAlgorithms#MLDevelopment#DeepLearningProjects#MachineLearningModels#TechLearning#AIForBeginners#DataDriven#MachineLearningTools
0 notes
Text

VADY – Simplifying Complex Data for Smarter Business Growth
Don't let big data overwhelm you—VADY simplifies it! 💡 Our AI-powered platform translates complex datasets into actionable insights, helping businesses make data-driven decisions with clarity and confidence. 📈
#VADY#ClearData#BusinessGrowth#AIForSuccess#SmartInsights#TechRevolution#DigitalTransformation#NewFangled#AIInnovation#NextGenAI#BusinessAutomation#MachineLearningModels#AIForAll#DataAnalytics#BusinessOptimization
0 notes
Text
The World of Machine Learning Platforms
Machine learning platforms are powerful tools that enable the development, training, and deployment of machine learning models. These platforms provide essential resources like algorithms, frameworks, and data processing tools. This guide explores the world of machine learning platforms, highlighting popular options, key features, and how they empower businesses and developers to build AI-driven solutions.
#MachineLearning#MLPlatforms#AIPlatforms#DataScience#MachineLearningTools#TechInnovation#AIdevelopment#MachineLearningModels#TechTrends#ArtificialIntelligence
0 notes
Text
ChatGPT vs. DeepSeek AI: Which AI Model Wins in 2025?
AI has come a long way, and in 2025, the competition between ChatGPT and DeepSeek AI is heating up. Both models are powerful, but they have different strengths. ChatGPT is known for its natural conversation flow, creativity, and versatility, while DeepSeek AI is gaining attention for its logical reasoning, factual accuracy, and problem-solving capabilities.
So, which one is the better AI model? Let’s break it down in simple human terms and determine which AI wins in 2025!
➥ Introduction: The AI Battle of 2025
If you’ve ever used an AI chatbot to answer your questions, help with writing, or generate ideas, chances are it was ChatGPT. OpenAI’s model has been dominating the space for a while.
But now, we have DeepSeek AI, a new player that promises better reasoning, deeper understanding, and more accurate answers. People are asking:
Which AI is smarter?
Which AI is more useful in daily life and work?
Is DeepSeek AI a real competitor to ChatGPT?
To answer these questions, let’s compare them based on their features, performance, and how they actually help people in real-world situations.
➥ Key Features Comparison
🧠 Understanding & Response Quality
ChatGPT: It’s great at mimicking human conversation, making it feel like you’re chatting with a real person. It’s creative, and engaging, and works well for storytelling, marketing, and brainstorming.
DeepSeek AI: It focuses more on logic, accuracy, and reasoning. If you ask it a factual question, it’s more likely to give a precise and well-structured answer.
🔄 Context & Memory
ChatGPT: Can remember parts of a conversation, but sometimes loses track if the discussion gets too long.
DeepSeek AI: Claims to have better memory, meaning it can keep up with longer, more detailed conversations without losing track.
🎨 Creativity & Content Writing
ChatGPT: If you need blog posts, stories, or catchy marketing copy, ChatGPT is amazing at generating creative content.
DeepSeek AI: It’s more focused on accuracy and clarity than creativity, so it might not be as strong for storytelling or marketing.
Read in detail: https://www.knowledgewale.com/2025/02/chatgpt-vs-deepseek-ai.html
#chatgptvsdeepseekai#deepseekai2025#chatgpt2025#aimodelcomparison#bestai2025#chatgptvdeepseek#deepseekvschatgpt#aiwars2025#artificialintelligencebattle#nlpmodelcomparison#chatbotcomparison#aiinnovation2025#machinelearningmodels#futureofai#aistrategy
0 notes
Text
Artificial Neural Networks in a Deep Learning Perspective
Artificial Neural Networks (ANNs) are central to deep learning, revolutionizing industries like healthcare, finance, and automotive. The Computer Science and Engineering department at K. Ramakrishnan College of Technology (KRCT) equips students with cutting-edge skills in ANNs, blending theory and hands-on projects. KRCT's focus on advanced AI ensures graduates are prepared to lead future innovations.

Click here:
#ArtificialNeuralNetworks#top college of technology in trichy#krct the top college of technology in trichy#quality engineering and technical education.#k ramakrishnan college of technology trichy#training and engineering placement#DeepLearning#AIRevolution#MachineLearningModels#FutureOfTechnology#ANNsInIndustry#ConvolutionalNeuralNetworks#ArtificialIntelligenceInsights#RecurrentNeuralNetworks#ExplainableAI#AIInHealthcare#AIInEducation
0 notes
Text
instagram
🚀Motivation comes from the sense of longing something or someone. May it be in terms of money, affluence or to woo someone 😇
🌟Start asking yourself Questions like:
📍Are you happy with your current situation? Is this the best that you can do?
Question this to yourself whenever you are weary.
If the answer to the above question is yes, then set new goals. Raise your bar.
But if you have the answer as No, then here are some things that you can do.
1. Focus on what you want more. There has to be something that you would want far more than others. Set that as your target.
2. Make it fun. Believe me you don’t want to do what you dont like.
3. Treat yourself with every step closer to your goal.
4. Fill yourself with a positive attitude. Always hope for better for that is one thing that gives us strength to move forward.
5. Once achieved your goal, set a new target.
The most important thing in life is moving forward; doing things that we haven’t. The thrill of the unknown and variety of possibilities of life that you can uncover will always keep you motivated. 🙏🏻✨🥰
#programming#programmers#developers#datascientist#machinelearning#deeplearning#tensorflow#PyTorch#codingchallenge#machinelearningtools#python#machinelearningalgorithm#machinelearningmodel#machinelearningmodels#datasciencecourse#datasciencebootcamp#dataanalyst#datavisualization#machinelearningengineer#artificialintelligence#mobiledeveloper#softwaredeveloper#devlife#coding#setup#1w#Instagram
0 notes
Text
What is data labeling in artificial intelligence?
Data labeling is a fundamental aspect of AI and ML development, enabling machines to understand and interpret complex data. With EnFuse Solutions as a trusted partner, businesses can access top-tier data labeling services that drive success in AI-driven applications. Their commitment to quality, accuracy, and efficiency makes them a preferred choice for companies seeking reliable data labeling solutions in India and beyond. Get in touch with EnFuse Solutions today!
#DataLabelingServices#LabeledDatasets#AISolutions#DataAnnotationServices#AIModels#MachineLearningModels#AIML#EnFuseSolutions
0 notes
Text
What Does A Data Annotator Do?
A data annotator is a vital link in the machine-learning pipeline, facilitating the creation of accurate and effective models. EnFuse Solutions, with its expertise and commitment to quality, emerges as a top choice for organizations seeking reliable and professional data annotation services.
#DataAnnotator#DataAnnotationServices#AIModels#MachineLearningModels#LabelingAndTaggingData#AIML#EnFuseSolutions
0 notes
Text
What Is Data Science? Learn Its Significance & Key Concepts

What is data science?
Data science is the study of data to derive business-relevant insights. In order to analyze vast volumes of data, this multidisciplinary approach integrates concepts and methods from the domains of computer engineering, artificial intelligence, statistics, and mathematics. Data scientists can use this analysis to ask and answer questions like as what happened, why it happened, what will happen, and what can be done with the data.
What makes data science essential?
Because it creates meaning from data by combining tools, techniques, and technology, data science is significant. There is an abundance of gadgets that can automatically gather and store data, and modern businesses are overloaded with it. In the domains of e-commerce, healthcare, banking, and every other facet of human existence, online platforms and payment portals gather more data. It possess enormous amounts of text, audio, video, and image data.
For what purposes is data science used?
There are four primary ways that data science is used to investigate data:
Descriptive analysis
Through descriptive analysis, one can learn more about what has occurred or is occurring in the data context. Data visualizations like tables, bar charts, line graphs, pie charts, and produced narratives are its defining features. Data such as the number of tickets purchased daily, for instance, may be recorded by an airline booking service. High-performing months for this service as well as booking slumps and spikes will be identified through descriptive analysis.
Diagnostic analysis
A thorough or in-depth data study to determine the cause of an event is known as diagnostic analysis. It is distinguished by methods like correlations, data mining, data discovery, and drill-down. A given data collection may undergo a number of data operations and transformations in order to find distinct patterns in each of these methods.For instance, in order to better understand the rise in bookings, the flying service may focus on a month that performs very well. This could reveal that a monthly athletic event draws a lot of customers to a specific city.
Predictive analysis
Using historical data, predictive analysis generates precise predictions about potential future data trends. Predictive modeling, pattern matching, forecasting, and machine learning are some of the methods that define it. Computers are trained to infer causal relationships from the data in each of these methods. The airline service team, for instance, might utilize data science at the beginning of each year to forecast flight booking trends for the upcoming year. The algorithm or computer software may use historical data to forecast May booking increases for specific locations. Given their knowledge of their customers’ future travel needs, the business may begin focusing its advertising efforts on those cities in February.
Prescriptive analysis
The next step up from predictive data is prescriptive analytics. In addition to forecasting the likely course of events, it also recommends the best course of action in the event of that occurrence. It can determine the optimum course of action by analyzing the possible effects of various decisions. It makes use of machine learning recommendation engines, neural networks, complicated event processing, simulation, and graph analysis.
To maximize the benefit of the impending booking increase, prescriptive analysis could examine past marketing campaigns, returning to the example of aircraft bookings. Booking results could be projected by a data scientist for varying marketing spend levels across many marketing channels. The airline would be more confident in its marketing choices with these data projections.
What are the business advantages of data science?
The way businesses function is being revolutionized by data science. A strong data science strategy is essential for many companies, regardless of size, to spur growth and keep a competitive edge. Key advantages include:
Find unidentified transformative patterns
Businesses can find new links and patterns with data science that could revolutionize their organization. For the greatest effect on profit margins, it might highlight inexpensive adjustments to resource management. For instance, data science is used by an online retailer to find that an excessive number of client inquiries are being sent after work hours. Customers who receive a timely response are more likely to make a purchase than those who receive an answer the following business day, according to investigations. Providing round-the-clock customer support increases the company’s income by 30%.
Innovate new products and solutions
Gaps and issues that would otherwise go unreported can be found via data science. More knowledge about consumer preferences, corporate procedures, and purchase decisions can spur innovation in both internal and external operations. Data science, for instance, is used by an online payment system to compile and examine social media reviews left by customers. According to analysis, customers are dissatisfied with the present password retrieval method and forget their credentials during periods of high purchase activity. The business can observe a notable rise in client satisfaction and develop a superior solution.
Real-time optimization
Real-time response to changing conditions is extremely difficult for corporations, particularly huge enterprises. This may result in large losses or interruptions to business operations. Data science may assist businesses in anticipating change and responding to various situations in the best possible way. When trucks break down, for instance, a truck-based transportation company employs data science to minimize downtime. Truck schedules are adjusted once they determine which routes and shift patterns result in more frequent breakdowns. Additionally, they establish a stock of popular spare components that require regular renewal in order to expedite vehicle repairs.
What is the process of data science?
Usually, the data science process starts with a business challenge. Working with business stakeholders, a data scientist will ascertain what the company needs. After defining the issue, the data scientist can use the OSEMN data science process to resolve it:
O – Obtain data
Existing data, recently acquired data, or a data repository that can be downloaded from the internet are all examples of data. Web server logs, social media, firm CRM software, internal or external databases, and reliable third-party sources are all places where data scientists can obtain and extract information.
S – Scrub data
Using a preset format to standardize the data is known as data scrubbing or data cleaning. Managing missing data, correcting data inaccuracies, and eliminating any data outliers are all included. Several instances of data cleansing include:
Fixing spelling errors or extra spaces; fixing mathematical errors or eliminating commas from big numbers; and standardizing the format of all date data.
E – Explore data
Preliminary data analysis, or data exploration, is used to design subsequent data modeling techniques. Using tools for data visualization and descriptive statistics, data scientists obtain a preliminary comprehension of the data. They then examine the data to find intriguing trends that might be investigated or used as a basis for action.
M – Model data
Deeper insights, outcome predictions, and the optimal course of action are all accomplished through the use of software and machine learning algorithms. Using the training data set, machine learning methods such as clustering, classification, and association are used. The correctness of the results may be evaluated by comparing the model to predefined test data. Numerous adjustments can be made to the data model to enhance the results.
N – Interpret results
Data scientists collaborate with analysts and companies to turn insights from data into action. They create charts, graphs, and diagrams to illustrate trends and forecasts. Data summary aids stakeholders in comprehending and successfully implementing outcomes.
What kinds of technology are used in data science?
Data scientists deal with sophisticated technologies such
Artificial intelligence: For prescriptive and predictive analysis, machine learning models and associated software are utilized. Cloud computing: With cloud technologies, data scientists now have the processing capacity and flexibility needed for sophisticated data analytics. Internet of things: The term “IoT” describes a variety of gadgets that can connect to the internet on their own. These gadgets gather information for projects using data science. Massive amounts of data are produced by them, which can be utilized for data extraction and mining. Quantum computing: These machines are capable of carrying out intricate computations quickly. Expert data scientists utilize them to create intricate quantitative algorithms.
Read more on govindhtech.com
#DataScience#LearnItsSignificance#KeyConcepts#artificialintelligence#datascience#Obtaindata#Scrubdata#Exploredata#Modeldata#data#Interpretresults#machinelearningmodels#machinelearning#technology#technews#news#govindhtech
0 notes
Photo
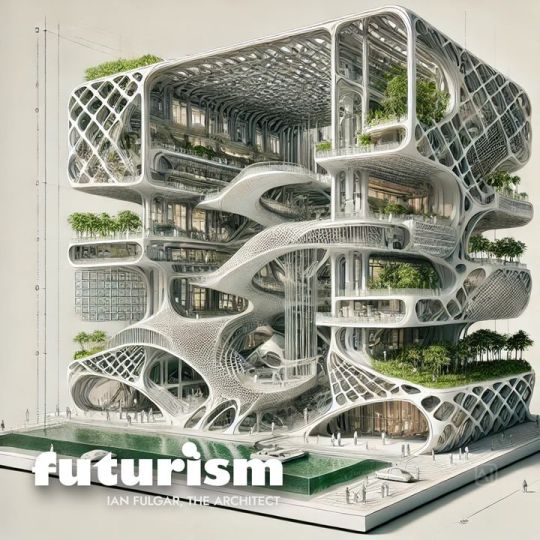
Traditional architectural designs often lack adaptability, limiting their relevance in a rapidly changing world. AI-powered generative adversarial networks (GANs) can help architects create adaptive designs that adjust to varying conditions, such as weather, population shifts, or technological advancements. By training these networks on vast datasets, we can develop buildings that evolve alongside their environment. How will this technology influence the longevity and resilience of our structures? Perhaps the future lies in buildings that continue to learn and adapt, creating architecture that pivots over time.
#ai#architecture#newcities#generativeadversarialnetworks#ganarchitecture#adaptivebuildingdesign#airesilience#dynamicarchitecture#aibuildingdesign#datadrivendesign#architecturaladaptability#futureproofdesign#intelligentarchitecture#aiandconstruction#architecturalresilience#machinelearningmodels#smartdesignsolutions#evolvingarchitecture
0 notes
Text
Flux.1: Görüntü Üretme Dünyasında Yeni Bir Dönem
Flux.1, Stable Diffusion'ın arkasındaki ekip olan Black Forest Labs tarafından geliştirilen, açık kaynaklı bir görüntü üretme modelidir. Bu son teknoloji yapay zeka modeli, olağanüstü görüntü kalitesi, detaylı çıktıları ve etkileyici istem takip yetenekleriyle kısa sürede dikkatleri üzerine çekti.
Flux.1'in Ana Özellikleri - Gelişmiş Anatomik Doğruluk: Flux.1, özellikle insan özellikleri, özellikle de eller konusunda daha önceki modellerin zorlandığı bir alanda mükemmel sonuçlar sunar. Bu sayede karakter tabanlı görüntülerde daha gerçekçi ve orantılı vücut parçaları elde edilir. - En Son Teknoloji Performansı: Flux.1, mükemmel istem takibi, görsel kalite, görüntü detayı ve çıktı çeşitliliği ile birinci sınıf görüntü üretimi sunar. - Çok Yönlülük: Model, çeşitli yaratıcı projeler için esneklik sunan geniş bir en boy oranı ve çözünürlük yelpazesini destekler. - Metin Üstünlüğü: Flux.1, metin oluşturmada özellikle başarılıdır ve bu da çarpıcı tipografi, gerçekçi tabelalar ve görüntüler içindeki karmaşık detaylar oluşturmak için ideal hale getirir. Flux.1'in Mimarisi ve Çalışma Prensibi - Rectified Flow Transformer: Flux.1, diğer birçok modern görüntü üretme modeli gibi, bir tür transformer mimarisi kullanır. Ancak, Flux.1'deki bu mimari, "rectified flow" olarak adlandırılan bir teknikle güçlendirilmiştir.Bu teknik, modelin daha karmaşık ve gerçekçi görüntüler üretmesine olanak tanır. - 12 Milyar Parametre: Modelin 12 milyar parametreye sahip olması, devasa bir bilgiyi hafızada tutmasına ve bu bilgiyi kullanarak oldukça çeşitli ve detaylı görüntüler oluşturmasına olanak tanır. - Eğitim Verisi: Flux.1, metin-görüntü eşleşmelerinden oluşan büyük bir veri seti üzerinde eğitilir. Bu sayede model,bir metin açıklamasına karşılık gelen bir görsel temsil oluşturmayı öğrenir. ve ilişkileri öğrenmesine olanak tanıyan büyük bir görüntü ve metin veri seti üzerinde eğitilmiştir. Neden Flux.1 Bu Kadar İyi? - Anatomik Doğruluk: Modelin mimarisi ve eğitim süreci, özellikle insan vücudu gibi karmaşık yapıları doğru bir şekilde temsil etmesine olanak tanır. Özellikle eller gibi zorlu bölgelerde bile oldukça başarılı sonuçlar verir. - Metin Anlama: Flux.1, metin istemlerini çok daha iyi anlar ve bu istemlere uygun görseller üretir. Örneğin, "bir astronotun ay yüzeyinde yürüdüğü bir resim" gibi karmaşık bir istemi bile doğru bir şekilde yorumlayabilir. - Detay Düzeyi: Modelin yüksek çözünürlüklü ve detaylı görüntüler üretebilme yeteneği, diğer modellerden ayrılan en önemli özelliklerinden biridir. Flux.1 ve Diğer Modeller Arasındaki Farklar - Stable Diffusion: Flux.1, Stable Diffusion'ın geliştirilmiş bir versiyonudur. Daha fazla parametreye sahip olması ve farklı bir eğitim süreci geçirmesi sayesinde daha iyi sonuçlar verir. - Midjourney: Midjourney gibi diğer popüler modellerle kıyaslandığında, Flux.1 genellikle daha gerçekçi ve detaylı görüntüler üretir. Ancak, her modelin farklı güçlü ve zayıf yönleri vardır. Flux.1'in Geleceği Flux.1, açık kaynaklı bir model olması sayesinde hızla gelişmektedir. Topluluk tarafından geliştirilen yeni teknikler ve daha büyük veri setleri sayesinde, modelin yetenekleri sürekli olarak artmaktadır. Gelecekte, Flux.1'in daha da gerçekçi ve yaratıcı görüntüler üretebileceğini söylemek yanlış olmaz. Sonuç Flux.1, görüntü üretme alanında önemli bir dönüm noktasıdır. Modelin sunduğu yüksek kalite, esneklik ve açık kaynaklı yapısı, birçok farklı alanda kullanılmasına olanak tanır. Gelecekte, yapay zeka destekli görüntü üretiminin daha da gelişmesiyle birlikte, Flux.1 gibi modellerin hayatımızın birçok alanında önemli bir rol oynayacağı öngörülmektedir. Read the full article
#AçıkKaynak#AIArt#AIGeneratedArt#AISanatı#BilgisayarGörü#ComputerVision#DeepLearningModels#DerinÖğrenme#DijitalSanat#Flux.1#GenerativeAI#GörüntüSentezi#GörüntüÜretimi#MachineLearningModels#MakineÖğrenmesi#MetindenGörüntüye#NeuralNetworks#OpenSourceSoftware#StableDiffusion#TexttoImage#YapaySinirAğları#yapayzeka#YapayZekaSanatı
0 notes
Text

What is the underlying AI model used by Copilot?
a) GPT-3 b) BERT c) ResNet d) AlphaFold
#CopilotAI#CopilotAIquiz#CopilotAIPoll#followme#followforfollow#instadaily#follow4follow#like4like#letsconnect#scriptzol#AIModels#CopilotInsights#GPT3Explained#BERTvsGPT3#ResNetOverview#AlphaFoldTech#MachineLearningModels#TechInnovation#AIUnderstanding#DeepLearningDemystified
0 notes
Text
EXHIBITOR ANNOUNCEMENT!!! Join us as an Exhibitor/Sponsor Advantage " Siya Diagnostics International" at #11DIGIPATH2023 Attend the CME/CPD accredited #11DIGIPATH2023 from December 15-17, 2023, in Holiday Inn Dubai, Al Barsha, UAE & Virtual.
These opportunities can lead to valuable connections and potential business partnerships. Industry digital pathology veterans, entrepreneurs, and young digital pathology professionals are all invited to engage in discussions that will shape the future of the digital pathology space WORKSHOP TITLE: NEXT GENERATION REPORTING & CONSULTANCY SYSTEM - Digital Diagnostics Beyond Barriers.” Exhibitor page: https://digitalpathology.ucgconferences.com/siya-diagnostics-international/ Book your sponsor/exhibitor spot here: https://digitalpathology.ucgconferences.com/exhibit-sponsor-with-us/ Visit here: https://digitalpathology.ucgconferences.com/committee-members-and-speakers/
#DigitalPathology#PathologyInnovation#VirtualMicroscopy#WholeSlideImaging#PathologyTechnology#digipathExhibitor#digipathSponsor#Telepathology#PathologyDigitalization#PathologyInformatics#DigitalPathologySolutions#PathologySlides#digipathWORKSHOP#MachineLearningModels#DataMining#NaturalLanguageProcessing#ComputerVision#AIEthics#AIIndustry
0 notes
Text
Integrating Machine Learning Platforms with Existing Systems
Integrating machine learning platforms with existing systems involves incorporating AI models into current software environments to enhance data processing, automation, and decision-making. This requires ensuring compatibility, scalability, and seamless communication between platforms. Key steps include selecting suitable ML models, preparing data, setting up APIs, and testing performance to ensure the integration delivers value and enhances system capabilities.
#MachineLearning#AIintegration#TechInnovation#DataScience#AIplatforms#SystemIntegration#Automation#TechDevelopment#AIinBusiness#MachineLearningModels
0 notes