#Analytics API
Explore tagged Tumblr posts
Text
Gemini Looker: AI-Driven Insights & Streamlined Development

Conversational, visual, AI-powered data discovery and integration are in Gemini Looker.
Google's trusted semantic model underpins accurate, reliable insights in the AI era, and today at Google Cloud Next '25, it's announcing a major step towards making Looker the most powerful data analysis and exploration platform by adding powerful AI capabilities and a new reporting experience.
Conversational analytics using Google's latest Gemini models and natural language is now available to all platform users. Looker has a redesigned reporting experience to better data storytelling and exploration. All Gemini Looker clients can use both technologies.
Modern organisations require AI to find patterns, predict trends, and inspire intelligent action. Looker reports and Gemini make business intelligence easier and more accessible. This frees analysts to focus on more impactful work, empowers enterprise users, and reduces data team labour.
Looker's semantic layer ensures everyone uses a single truth source. Gemini Looker and Google's AI now automate analysis and give intelligent insights, speeding up data-driven organisational decisions.
All Looker users can now utilise Gemini
To make sophisticated AI-powered business intelligence (BI) accessible, you introduced Gemini in Looker at Google Cloud Next ’24. This collection of assistants lets customers ask their data queries in plain language and accelerates data modelling, chart and presentation building, and more.
Since then, those capabilities have been in preview, and now that the product is more accurate and mature, they should be available to all platform users. Conversational Analytics leverages natural language queries to deliver data insights, while Visualisation Assistant makes it easy to configure charts and visualisations for dashboards using natural language.
Formula Assistant provides powerful on-the-fly calculated fields and instant ad hoc analysis; Automated Slide Generation creates insightful and instantaneous text summaries of your data to create impactful presentations; and LookML Code Assistant simplifies code creation by suggesting dimensions, groups, measures, and more.
Business users may perform complex procedures and get projections using the Code Interpreter for Conversational insights in preview.
Chat Analytics API
We also released the Conversational Analytics API to expand conversational analytics beyond Gemini Looker. Developers may now immediately add natural language query capabilities into bespoke apps, internal tools, or workflows due to scalable, reliable data modelling that can adapt to changing requirements and secure data access.
This API enables you develop unique BI agent experiences using Google's advanced AI models (NL2SQL, RAG, and VizGen) and Looker's semantic model for accuracy. Developers may easily leverage this functionality in Gemini Looker to build user-friendly data experiences, ease complex natural language analysis, and share insights from these talks.
Introduce Looker reports
Self-service analysis empowers line-of-business users and fosters teamwork. Looker reports integrate Looker Studio's powerful visualisation and reporting tools into the main Looker platform, boosting its appeal and use.
Looker reports, which feature native Looker content, direct linkages to Google Sheets and Microsoft Excel data, first-party connectors, and ad hoc access to various data sources, increase data storytelling, discovery, and connectivity.
Interactive reports are easier than ever to make. Looker reports includes a huge collection of templates and visualisations, extensive design options, real-time collaboration features, and the popular drag-and-drop interface.
New reporting environment coexists with Looker Dashboards and Explores in Gemini Looker's regulated framework. Importantly, Gemini in Looker readily integrates with Looker Reports, allowing conversational analytics in this new reporting environment.
Continuous integration ensures faster, more dependable development
Google Cloud is automating SQL and LookML testing and validation by purchasing Spectacles.dev, enabling faster and more reliable development cycles. Strong CI/CD methods build data confidence by ensuring semantic model precision and consistency, which is critical for AI-powered BI.
Looker reports, the Conversational Analytics API, Gemini, and native Continuous Integration features promote an AI-for-BI platform. Nous make powerful AI, accurate insights, and a data-driven culture easier than ever.
Attend Google Cloud Next to see Gemini Looker and hear how complete AI for BI can convert your data into a competitive advantage. After the event, Gemini Looker offers AI for BI lecture.
#technology#technews#govindhtech#news#technologynews#AI#artifical intelligence#Gemini Looker#Gemini#Looker reports#Google cloud Looker#Gemini in Looker#Analytics API
0 notes
Text
How to Integrate Analytics APIs into Your Business Workflow

Nowadays, businesses depend on data to make better decisions and work more efficiently. Using analytics API in your business workflow can greatly enhance how you collect, analyze, and report data. Integrating these tools helps you understand your data better and make informed choices that can lead to improved performance and growth. This blog outlines key steps to seamlessly integrate Analytics APIs into your existing systems, ensuring you harness their full potential.
1. Understand Your Analytics Needs
Before integrating an analytics API, it’s crucial to identify your specific analytics requirements. Consider what data you need to track, such as user behaviour, sales performance, or marketing effectiveness. Understanding your goals will help you select the right API and establish metrics that match your business objectives.
2. Choose the Right Analytics API
With various analytics APIs available, selecting the right one for your needs is essential. Popular options include Google Analytics, Adobe Analytics, and Mixpanel. Evaluate these APIs based on features, ease of integration, cost, and compatibility with your existing systems. Additionally, consider factors such as scalability and customer support to ensure long-term success.
3. Plan Your Integration Strategy
Once you’ve chosen an API, it’s time to develop a clear integration strategy. Outline the steps involved in the integration process, including the required resources, timelines, and potential challenges. Consider whether you will integrate the API directly into your application or use middleware to facilitate the connection. Creating a detailed plan will help streamline the integration process and minimize disruptions.
4. Set Up Authentication and Access Controls
Most analytics API require authentication to ensure secure access to data. Implement OAuth or API keys for authentication, following best practices for managing access controls. Assign permissions based on user roles within your organization so that only authorized personnel can access sensitive data.
5. Develop Data Collection Methods
Integrating an Analytics API involves developing methods for data collection. Depending on the API, this may require adding tracking codes to your website or application to configure event tracking, or setting up data pipelines. Ensure that your data collection methods are accurately capturing the information you need for analysis.
6. Test the Integration
Before fully deploying the integration, conduct thorough testing to ensure everything functions correctly. Check that data is collected accurately and that reports are generated as expected. Address any issues identified during testing to avoid complications once the API is live.
7. Train Your Team
To maximize the benefits of your Analytics API, it’s essential to train your team on how to use the new tools effectively. Provide training sessions and resources to help employees understand the features of the API and how to interpret the data. Empowering your team with the right knowledge will enhance their ability to make data-driven decisions.
8. Monitor and Optimize
After the integration is complete, continuously monitor the performance of the Analytics API within your workflow. Analyze the collected data to identify trends, patterns, and areas for improvement. Regularly review your analytics strategy and make adjustments as needed to ensure you are leveraging the full capabilities of the API.
Conclusion
Integrating analytics API into your business workflow can transform the way you gather, analyze, and utilize data. By following these steps—understanding your needs, choosing the right API, planning your integration, and training your team—you can ensure a successful implementation. With the right analytics, your business can make informed decisions that drive growth and enhance overall performance.
Transform your data insights with AllThingsDev for seamless Analytics API integration!
0 notes
Text
I will setup facebook pixel google analytics 4 migration conversion API with GTM
Do you plan for Facebook pixel setup, conversion API, product catalogs, enhanced e-commerce tracking, or UTM? Google Tag Manager and Google Analytics 4. Ready to boost your business?
What is Facebook Pixel, UTM, Google Analytics 4, and GTM?.
The Facebook Pixel, UTM, Google Analytics, and tag manager are codes that are placed on your website, page, business page, and service related website. It collects and gathers data that converts your ads, optimizes ads, and builds targeted traffic and strong demographics for future ads.
What will I give for your business, service, and targeted audience?
Setup Facebook pixel.
Setup conversion API With GTM.
Conversion API Setup and integration.
Automatic shop catalog and optimization.
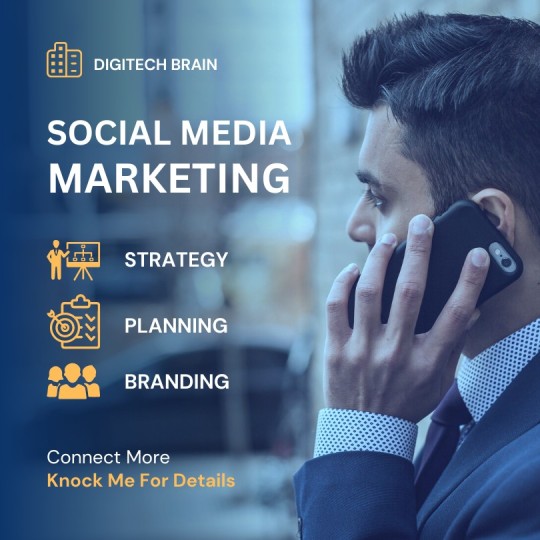
#facebookpixelsetup#facebookads#facebook#gtm#google analytics#conversion api#facebook marketing#facebook pages
13 notes
·
View notes
Text
How Real-Time Sports Data is Powering the Future of the Global Sports Industry
In an era where speed, precision, and interactivity define the sports experience, real-time sports data has emerged as the cornerstone of transformation across the global sports industry. From enhancing fan engagement and enabling smarter coaching decisions to powering next-gen fantasy platforms and fueling sports betting insights, real-time data is revolutionizing how sports are played, watched, and monetized.
As a leader in delivering accurate, real-time, and customizable data feeds, Data Sports Group (DSG) stands at the forefront of this data-driven evolution. Let’s explore how real-time sports data is shaping the future of sports and why it’s becoming indispensable for every stakeholder in the ecosystem.
1. The Rise of Real-Time Sports Data
Not too long ago, post-match statistics were the norm. Coaches relied on after-game reviews, fans waited for highlight reels, and fantasy players depended on next-day updates. Fast forward to 2025, and the demand for live, up-to-the-second insights has become the industry standard.
With the adoption of IoT devices, high-speed connectivity, and AI-powered analytics, data is now collected, processed, and delivered in real time—covering everything from player positioning and ball possession to biometric stats and predictive performance metrics.
2. Transforming Fan Engagement
Today’s fans are no longer passive spectators. They demand interactivity, insights, and personalized experiences.
How Real-Time Data Enhances Fan Experience:
Live Stats & Visualizations: Fans enjoy instant access to stats like speed, distance covered, shot accuracy, and player heatmaps during the match.
Second-Screen Experiences: Apps and streaming platforms now integrate real-time dashboards for fans to analyze gameplay as it unfolds.
Gamification & Fantasy Integration: Real-time data fuels fantasy sports and prediction games, increasing engagement through competition.
With DSG’s real-time sports data API, platforms can deliver immersive, data-rich experiences that keep fans engaged before, during, and after the match.
3. Empowering Coaching & Player Performance
For teams and coaches, real-time data is more than just a tool—it’s a tactical weapon. Modern coaching staffs now rely on real-time analytics to:
Track player fatigue, heart rate, and stress levels.
Adjust tactics based on opponent behavior and momentum shifts.
Make split-second decisions on substitutions and positioning.
Using wearable tech and AI, real-time performance tracking helps teams optimize training, reduce injury risks, and strategize more effectively.
4. Enabling Smarter Fantasy Sports Platforms
Fantasy sports has evolved into a billion-dollar industry. Real-time data is the engine that drives user engagement, satisfaction, and retention in this space.
Key Benefits for Fantasy Platforms:
Live Scoring Updates: Users can track performance points as players perform on the field.
Instant Lineup Adjustments: Real-time injury updates and performance alerts empower users to make timely changes.
AI-Driven Recommendations: Predictive analytics suggest smart picks based on historical and current data.
Platforms that integrate DSG’s fantasy-ready data feeds enjoy higher user interaction, more time spent on apps, and lower churn rates.
5. Driving Precision in Sports Betting
In sports betting, information is currency. Real-time data gives sportsbooks the edge to:
Offer live odds and micro-betting options (e.g., next goal, next serve winner).
Adjust spreads and betting lines dynamically.
Detect suspicious betting patterns using real-time anomaly detection.
With DSG’s secure, fast, and reliable data feeds, betting operators gain access to real-time event data, injury updates, player form, and even environmental conditions.
6. Powering Sports Media & Broadcasting
Real-time data is redefining storytelling in sports broadcasting. Commentators and analysts now have:
Instant Access to Deep Stats: Enriching match narratives with advanced stats.
Predictive Tools: Forecasting player substitutions or game outcomes using AI models.
On-Screen Visuals: Delivering interactive overlays and dynamic charts to the viewers.
Whether it’s OTT platforms or traditional broadcasters, real-time data enhances the viewing experience with interactive, insightful, and engaging content.
7. Data Integrity & Security: A Non-Negotiable
As sports data becomes more central to high-stakes decisions, the integrity and security of that data are critical. DSG ensures:
Blockchain-Backed Data Integrity
End-to-End Encryption
Scalable APIs with 99.99% Uptime
Clients trust DSG for accuracy, speed, and reliability, ensuring their platforms never miss a beat.
8. Future-Proofing the Sports Ecosystem
Looking ahead, real-time data will continue to evolve with technologies like:
5G & Edge Computing: Reducing latency for instantaneous data delivery.
Augmented Reality (AR): A
Enriching live experiences with data overlays.
AI-Powered Insights: Automating deeper, predictive analysis.
As the industry adapts, those who leverage real-time data will stay ahead—be it in performance, engagement, or monetization.
Why Choose Data Sports Group?
At DSG, we’re not just delivering data. We’re powering the future of sports. Here’s what sets us apart:
Global Coverage: From football and cricket to esports and American football.
Customizable Solutions: Tailored APIs for fantasy, betting, broadcasting, and coaching.
Real-Time Accuracy: Instant updates that match the speed of the game.
Actionable Insights: From raw data to predictive modeling—all in one place.
Final Thoughts
The global sports industry is undergoing a massive shift—from reactive analysis to proactive, real-time intelligence. Whether you’re a coach, fantasy app developer, betting operator, or media executive, one thing is clear: real-time sports data is your competitive edge.
Partner with Data Sports Group to unlock the full potential of data in sports.
📩 Ready to power your platform with real-time insights? Contact DSG and experience the future of sports data today.
0 notes
Text
Are you struggling with inaccurate data, missing conversions, or ineffective tracking? Accurate tracking is the backbone of high-performing ad campaigns, and I specialize in Google Analytics 4 (GA4), Google Tag Manager (GTM), Facebook Pixel, Conversion API (CAPI), and Server-Side Tracking to ensure precise data collection and campaign optimization.
0 notes
Text

Why is Data Critical in Life Sciences and the Emerging Trends in 2024
The life sciences industry covers sectors such as pharmaceuticals, biotechnology, healthcare, and medical devices, and the sector has been rapidly evolving in the last few years based on the interaction of data analytics, AI, and other corresponding digital technologies. The growth of precision medicine, a desire for better efficiency in drug development, and a growing focus on patient outcomes are part of the reason why data has become so critical to innovation in the life sciences industry today.
#pharmaceutical companies in south Africa#pharmacy products#pharmaceutical online#pharmaceutical research#third party medicine manufacturer#drug manufacturing process#pharma advertising#API manufacturing process#pharma analytics
1 note
·
View note
Text
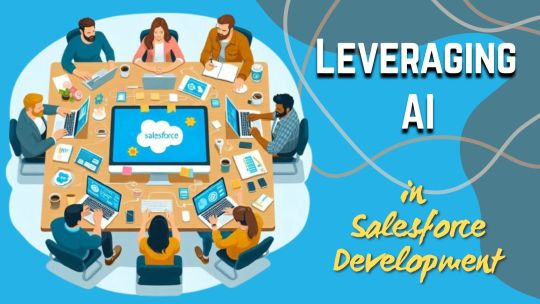
Leveraging AI in Salesforce Development
Artificial Intelligence (AI) is revolutionizing Salesforce development by infusing intelligence into traditional CRM processes. AI allows Salesforce to evolve from a static data management platform into a dynamic, insight-driven system that can predict, recommend, and automate key functions. With AI, Salesforce development is now more about building solutions that are not just reactive but proactive.
Know more at: https://www.cyberswift.com/blog/leveraging-ai-in-salesforce-development/
#generative ai in salesforce#salesforce ai cloud#salesforce einstein gpt#ai-powered crm solutions#bill cipher#salesforce automation with ai#predictive analytics in salesforce#ai-driven customer insights#ai-enhanced salesforce workflows#salesforce lightning and ai integration#chatbots and salesforce crm#salesforce consulting services#salesforce crm solutions#custom salesforce development#salesforce lightning development#salesforce app development#salesforce api integration#salesforce cloud services#salesforce automation solutions#salesforce migration services#salesforce support and maintenance#low-code salesforce development#ai-powered salesforce solutions#iot integration with salesforce#salesforce blockchain integration
1 note
·
View note
Text
Top 10 AI Tools for Embedded Analytics and Reporting (May 2025)
New Post has been published on https://thedigitalinsider.com/top-10-ai-tools-for-embedded-analytics-and-reporting-may-2025/
Top 10 AI Tools for Embedded Analytics and Reporting (May 2025)
Embedded analytics refers to integrating interactive dashboards, reports, and AI-driven data insights directly into applications or workflows. This approach lets users access analytics in context without switching to a separate BI tool. It’s a rapidly growing market – valued around $20 billion in 2024 and projected to reach $75 billion by 2032 (18% CAGR).
Organizations are embracing embedded analytics to empower end-users with real-time information. These trends are fueled by demand for self-service data access and AI features like natural language queries and automated insights, which make analytics more accessible.
Below we review top tools that provide AI-powered embedded analytics and reporting. Each tool includes an overview, key pros and cons, and a breakdown of pricing tiers.
AI Tools for Embedded Analytics and Reporting (Comparison Table)
AI Tool Best For Price Features Explo Turnkey, white-label SaaS dashboards Free internal · embed from $795/mo No-code builder, Explo AI NLQ, SOC 2/HIPAA ThoughtSpot Google-style NL search for data in apps Dev trial free · usage-based quote SpotIQ AI insights, search & Liveboards embed Tableau Embedded Pixel-perfect visuals & broad connectors $12–70/user/mo Pulse AI summaries, drag-drop viz, JS API Power BI Embedded Azure-centric, cost-efficient scaling A1 capacity from ~$735/mo NL Q&A, AutoML visuals, REST/JS SDK Looker Governed metrics & Google Cloud synergy Custom (≈$120k+/yr) LookML model, secure embed SDK, BigQuery native Sisense OEMs needing deep white-label control Starter ≈$10k/yr · Cloud ≈$21k/yr ElastiCube in-chip, NLQ, full REST/JS APIs Qlik Associative, real-time data exploration $200–2,750/mo (capacity-based) Associative engine, Insight Advisor AI, Nebula.js Domo Everywhere Cloud BI with built-in ETL & sharing From ~$3k/mo (quote) 500+ connectors, alerts, credit-based scaling Yellowfin BI Data storytelling & flexible OEM pricing Custom (≈$15k+/yr) Stories, Signals AI alerts, multi-tenant Mode Analytics SQL/Python notebooks to embedded reports Free · Pro ≈$6k/yr Notebooks, API embed, Visual Explorer
(Source: Explo)
Explo is an embedded analytics platform designed for product and engineering teams to quickly add customer-facing dashboards and reports to their apps. It offers a no-code interface for creating interactive charts and supports white-labeled embedding, so the analytics blend into your product’s UI.
Explo focuses on self-service: end-users can explore data and even build ad hoc reports without needing developer intervention. A standout feature is Explo AI, a generative AI capability that lets users ask free-form questions and get back relevant charts automatically.
This makes data exploration as easy as typing a query in natural language. Explo integrates with many databases and is built to scale from startup use cases to enterprise deployments (it’s SOC II, GDPR, and HIPAA compliant for security).
Pros and Cons
Drag-and-drop dashboards—embed in minutes
Generative AI (Explo AI) for NLQ insights
Full white-label + SOC 2 / HIPAA compliance
Young platform; smaller community
Costs rise with large end-user counts
Cloud-only; no on-prem deployment
Pricing: (Monthly subscriptions – USD)
Launch – Free: Internal BI use only; unlimited internal users/dashboards.
Growth – from $795/month: For embedding in apps; includes 3 embedded dashboards, 25 customer accounts.
Pro – from $2,195/month: Advanced embedding; unlimited dashboards, full white-label, scales with usage.
Enterprise – Custom: Custom pricing for large scale deployments; includes priority support, SSO, custom features.
Visit Explo →
ThoughtSpot is an AI-driven analytics platform renowned for its search-based interface. With ThoughtSpot’s embedded analytics, users can type natural language queries (or use voice) to explore data and instantly get visual answers.
This makes analytics accessible to non-technical users – essentially a Google-like experience for your business data. ThoughtSpot’s in-memory engine handles large data volumes, and its AI engine (SpotIQ) automatically finds insights and anomalies.
For embedding, ThoughtSpot provides low-code components and robust REST APIs/SDKs to integrate interactive Liveboards (dashboards) or even just the search bar into applications. It’s popular for customer-facing analytics in apps where end-users need ad-hoc querying ability.
Businesses in retail, finance, and healthcare use ThoughtSpot to let frontline employees and customers ask data questions on the fly. The platform emphasizes ease-of-use and fast deployment, though it also offers enterprise features like row-level security and scalability across cloud data warehouses.
Pros and Cons
Google-style NL search for data
SpotIQ AI auto-surfaces trends
Embeds dashboards, charts, or just the search bar
Enterprise-grade pricing for SMBs
Limited advanced data modeling
Setup needs schema indexing expertise
Pricing: (Tiered, with consumption-based licensing – USD)
Essentials – $1,250/month (billed annually): For larger deployments; increased data capacity and features.
ThoughtSpot Pro: Custom quote. Full embedding capabilities for customer-facing apps (up to ~500 million data rows).
ThoughtSpot Enterprise: Custom quote. Unlimited data scale and enterprise SLA. Includes multi-tenant support, advanced security, etc.
Visit ThoughtSpot →
Tableau (part of Salesforce) is a leading BI platform known for its powerful visualization and dashboarding capabilities. Tableau Embedded Analytics allows organizations to integrate Tableau’s interactive charts and reports into their own applications or websites.
Developers can embed Tableau dashboards via iFrames or using the JavaScript API, enabling rich data visuals and filtering in-app. Tableau’s strength lies in its breadth of out-of-the-box visuals, drag-and-drop ease for creating dashboards, and a large user community.
It also has introduced AI features – for example, in 2024 Salesforce announced Tableau Pulse, which uses generative AI to deliver automated insights and natural language summaries to users. This augments embedded dashboards with proactive explanations.
Tableau works with a wide range of data sources and offers live or in-memory data connectivity, ensuring that embedded content can display up-to-date info. It’s well-suited for both internal embedded use (e.g. within an enterprise portal) and external customer-facing analytics, though licensing cost and infrastructure must be planned accordingly.
Pros and Cons
Market-leading visual library
New “Pulse” AI summaries & NLQ
Broad data connectors + massive community
License cost balloons at scale
Requires Tableau Server/Cloud infrastructure
Styling customization via JS API only
Pricing: (Subscription per user, with role-based tiers – USD)
Creator – $70 per user/month: Full authoring license (data prep, dashboard creation). Needed for developers building embedded dashboards.
Explorer – $35 per user/month: For users who explore and edit limited content. Suitable for internal power users interacting with embedded reports.
Viewer – $12 per user/month: Read-only access to view dashboards. For end viewers of embedded analytics.
Visit Tableau →
Microsoft Power BI is a widely-used BI suite, and Power BI Embedded refers to the Azure service and APIs that let you embed Power BI visuals into custom applications. This is attractive for developers building customer-facing analytics, as it combines Power BI’s robust features (interactive reports, AI visuals, natural language Q&A, etc.) with flexible embedding options.
You can embed full reports or individual tiles, control them via REST API, and apply row-level security for multi-tenant scenarios. Power BI’s strengths include tight integration with the Microsoft ecosystem (Azure, Office 365), strong data modeling (via Power BI Desktop), and growing AI capabilities (e.g. the Q&A visual that allows users to ask questions in plain English).
Pros and Cons
Rich BI + AI visuals (NL Q&A, AutoML)
Azure capacity pricing scales to any user base
Deep Microsoft ecosystem integration
Initial setup can be complex (capacities, RLS)
Devs need Power BI Pro licenses
Some portal features absent in embeds
Pricing: (Azure capacity-based or per-user – USD)
Power BI Pro – $14/user/month: Enables creating and sharing reports. Required for developers and any internal users of embedded content.
Power BI Premium Per User – $24/user/month: Enhanced features (AI, larger datasets) on a per-user basis. Useful if a small number of users need premium capabilities instead of a full capacity.
Power BI Embedded (A SKUs) – From ~$735/month for A1 capacity (3 GB RAM, 1 v-core). Scales up to ~$23,500/month for A6 (100 GB, 32 cores) for high-end needs. Billed hourly via Azure, with scale-out options.
Visit Power BI →
Looker is a modern analytics platform now part of Google Cloud. It is known for its unique data modeling layer, LookML, which lets data teams define business metrics and logic centrally.
For embedded analytics, Looker provides a robust solution: you can embed interactive dashboards or exploratory data tables in applications, leveraging the same Looker backend. One of Looker’s core strengths is consistency – because of LookML, all users (and embedded views) use trusted data definitions, avoiding mismatched metrics.
Looker also excels at integrations: it connects natively to cloud databases (BigQuery, Snowflake, etc.), and because it’s in the Google ecosystem, it integrates with Google Cloud services (permissions, AI/ML via BigQuery, etc.).
Pros and Cons
LookML enforces single source of truth
Secure embed SDK + full theming
Tight BigQuery & Google AI integration
Premium six-figure pricing common
Steep LookML learning curve
Visuals less flashy than Tableau/Power BI
Pricing: (Custom quotes via sales; example figures)
Visit Looker →
Sisense is a full-stack BI and analytics platform with a strong focus on embedded analytics use cases. It enables companies to infuse analytics into their products via flexible APIs or web components, and even allows building custom analytic apps.
Sisense is known for its ElastiCube in-chip memory technology, which can mash up data from multiple sources and deliver fast performance for dashboards. In recent years, Sisense has incorporated AI features (e.g. NLQ, automated insights) to stay competitive.
A key advantage of Sisense is its ability to be fully white-labeled and its OEM-friendly licensing, which is why many SaaS providers choose it to power their in-app analytics. It offers both cloud and on-premises deployment options, catering to different security requirements.
Sisense also provides a range of customization options: you can embed entire dashboards or individual widgets, and use their JavaScript library to deeply customize look and feel. It’s suited for organizations that need an end-to-end solution – from data preparation to visualization – specifically tailored for embedding in external applications.
Pros and Cons
ElastiCube fuses data fast in-memory
White-label OEM-friendly APIs
AI alerts & NLQ for end-users
UI learning curve for new users
Quote-based pricing can be steep
Advanced setup often needs dev resources
Pricing: (Annual license, quote-based – USD)
Starter (Self-Hosted) – Starts around $10,000/year for a small deployment (few users, basic features). This would typically be an on-prem license for internal BI or limited OEM use.
Cloud (SaaS) Starter – ~$21,000/year for ~5 users on Sisense Cloud (cloud hosting carries ~2× premium over self-host).
Growth/Enterprise OEM – Costs scale significantly with usage; mid-range deployments often range $50K-$100K+ per year. Large enterprise deals can reach several hundred thousand or more if there are very high numbers of end-users.
Visit Sisense →
Qlik is a long-time leader in BI, offering Qlik Sense as its modern analytics platform. Qlik’s embedded analytics capabilities allow you to integrate its associative data engine and rich visuals into other applications.
Qlik’s differentiator is its Associative Engine: users can freely explore data associations (making selections across any fields) and the engine instantly updates all charts to reflect those selections, revealing hidden insights.
In an embedded scenario, this means end-users can get powerful interactive exploration, not just static filtered views. Qlik provides APIs (Capability API, Nebula.js library, etc.) to embed charts or even build fully custom analytics experiences on top of its engine. It also supports standard embed via iframes or mashups.
Qlik has incorporated AI as well – the Insight Advisor can generate insights or chart suggestions automatically. For developers, Qlik’s platform is quite robust: you can script data transformations in its load script, use its security rules for multi-tenant setups, and even embed Qlik into mobile apps.
Pros and Cons
Associative engine enables free exploration
Fast in-memory performance for big data
Robust APIs + Insight Advisor AI
Unique scripting → higher learning curve
Enterprise-level pricing
UI can feel dated without theming
Pricing: (USD)
Starter – $200 / month (billed annually): Includes 10 users + 25 GB “data for analysis.” No extra data add-ons available.
Standard – $825 / month: Starts with 25 GB; buy more capacity in 25 GB blocks. Unlimited user access.
Premium – $2,750 / month: Starts with 50 GB, adds AI/ML, public/anonymous access, larger app sizes (10 GB).
Enterprise – Custom quote: Begins at 250 GB; supports larger app sizes (up to 40 GB), multi-region tenants, expanded AI/automation quotas.
Visit Qlik →
Domo is a cloud-first business intelligence platform, and Domo Everywhere is its embedded analytics solution aimed at sharing Domo’s dashboards outside the core Domo environment. With Domo Everywhere, companies can distribute interactive dashboards to customers or partners via embed codes or public links, while still managing everything from the central Domo instance.
Domo is known for its end-to-end capabilities in the cloud – from data integration (500+ connectors, built-in ETL called Magic ETL) to data visualization and even a built-in data science layer.
For embedding, Domo emphasizes ease of use: non-technical users can create dashboards in Domo’s drag-and-drop interface, then simply embed them with minimal coding. It also offers robust governance so you can control what external viewers see.
Pros and Cons
End-to-end cloud BI with 500+ connectors
Simple drag-and-embed workflow
Real-time alerts & collaboration tools
Credit-based pricing tricky to budget
Cloud-only; no on-prem option
Deeper custom UI needs dev work
Pricing: (Subscription, contact Domo for quote – USD)
Basic Embedded Package – roughly $3,000 per month for a limited-user, limited-data scenario. This might include a handful of dashboards and a moderate number of external viewers.
Mid-size Deployment – approximately $20k–$50k per year for mid-sized businesses. This would cover more users and data; e.g., a few hundred external users with regular usage.
Enterprise – $100k+/year for large-scale deployments. Enterprises with thousands of external users or very high data volumes can expect costs in six figures. (Domo often structures enterprise deals as unlimited-user but metered by data/query credits.)
Visit Domo →
Yellowfin is a BI platform that has carved a niche in embedded analytics and data storytelling. It offers a cohesive solution with modules for dashboards, data discovery, automated signals (alerts on changes), and even a unique Story feature for narrative reporting.
For embedding, Yellowfin Embedded Analytics provides OEM partners a flexible licensing model and technical capabilities to integrate Yellowfin content into their applications. Yellowfin’s strength lies in its balanced focus: it’s powerful enough for enterprise BI but also streamlined for embedding, with features like multi-tenant support and white-labeling.
It also has NLP query (natural language querying) and AI-driven insights, aligning with modern trends. A notable feature is Yellowfin’s data storytelling – you can create slide-show style narratives with charts and text, which can be embedded to give end-users contextual analysis, not just raw dashboards.
Yellowfin is often praised for its collaborative features (annotations, discussion threads on charts) which can be beneficial in an embedded context where you want users to engage with the analytics.
Pros and Cons
Built-in Stories & Signals for narratives
OEM pricing adaptable (fixed or revenue-share)
Multi-tenant + full white-label support
Lower brand recognition vs. “big three”
Some UI elements feel legacy
Advanced features require training
Pricing: (Custom – Yellowfin offers flexible models)
Visit Yellowfin →
Mode is a platform geared towards advanced analysts and data scientists, combining BI with notebooks. It’s now part of ThoughtSpot (acquired in 2023) but still offered as a standalone solution.
Mode’s appeal in an embedded context is its flexibility: analysts can use SQL, Python, and R in one environment to craft analyses, then publish interactive visualizations or dashboards that can be embedded into web apps. This means if your application’s analytics require heavy custom analysis or statistical work, Mode is well-suited.
It has a modern HTML5 dashboarding system and recently introduced “Visual Explorer” for drag-and-drop charting, plus AI assist features for query suggestions. Companies often use Mode to build rich, bespoke analytics for their customers – for example, a software company might use Mode to develop a complex report, and then embed that report in their product for each customer with the data filtered appropriately.
Mode supports white-label embedding, and you can control it via their API (to provision users, run queries, etc.). It’s popular with data teams due to the seamless workflow from coding to sharing insights.
Pros and Cons
Unified SQL, Python, R notebooks → dashboards
Strong API for automated embedding
Generous free tier for prototyping
Analyst skills (SQL/Python) required
Fewer NLQ/AI features for end-users
Visualization options less extensive than Tableau
Pricing: (USD)
Studio (Free) – $0 forever for up to 3 users. This includes core SQL/Python/R analytics, private data connections, 10MB query limit, etc. Good for initial development and testing of embedded ideas.
Pro (Business) – Starts around ~$6,000/year (estimated). Mode doesn’t list fixed prices, but third-party sources indicate pro plans in the mid four-figure range annually for small teams.
Enterprise – Custom pricing, typically five-figure annually up to ~$50k for large orgs. Includes all Pro features plus enterprise security (SSO, advanced permissions), custom compute for heavy workloads, and premium support.
Visit Mode →
How to Choose the Right Embedded Analytics Tool
Selecting an embedded analytics solution requires balancing your company’s needs with each tool’s strengths. Start with your use case and audience: Consider who will be using the analytics and their technical level. If you’re embedding dashboards for non-technical business users or customers, a tool with an easy UI could be important. Conversely, if your application demands highly custom analyses or you have a strong data science team, a more flexible code-first tool might be better.
Also evaluate whether you need a fully managed solution (more plug-and-play, e.g. Explo or Domo) or are willing to manage more infrastructure for a potentially more powerful platform (e.g. self-hosting Qlik or Sisense for complete control). The size of your company (and engineering resources) will influence this trade-off – startups often lean towards turnkey cloud services, while larger enterprises might integrate a platform into their existing tech stack.
Integration and scalability are critical factors. Look at how well the tool will integrate with your current systems and future architecture. Finally, weigh pricing and total cost of ownership against your budget and revenue model. Embedded analytics tools vary from per-user pricing to usage-based and fixed OEM licenses. Map out a rough projection of costs for 1 year and 3 years as your user count grows.
FAQs (Embedded Analytics and Reporting)
1. What are the main differences between Tableau and Power BI?
Tableau focuses on advanced visual design, cross-platform deployment (on-prem or any cloud), and a large viz library, but it costs more per user. Power BI is cheaper, tightly integrated with Microsoft 365/Azure, and great for Excel users, though some features require an Azure capacity and Windows-centric stack.
2. How does Sisense handle large datasets compared to other tools?
Sisense’s proprietary ElastiCube “in-chip” engine compresses data in memory, letting a single node serve millions of rows while maintaining fast query response; benchmarks show 500 GB cubes on 128 GB RAM. Competing BI tools often rely on external warehouses or slower in-memory engines for similar workloads.
3. Which embedded analytics tool offers the best customization options?
Sisense and Qlik are stand-outs: both expose full REST/JavaScript APIs, support deep white-labeling, and let dev teams build bespoke visual components or mashups—ideal when you need analytics to look and feel 100 % native in your app.
4. Are there any free alternatives to Tableau and Sisense?
Yes—open-source BI platforms like Apache Superset, Metabase, Redash, and Google’s free Looker Studio deliver dashboarding and basic embedded options at zero cost (self-hosted or SaaS tiers), making them good entry-level substitutes for smaller teams or tight budgets.
#000#2023#2024#2025#250#Accounts#ADD#ai#ai tools#AI-powered#AI/ML#alerts#amp#analyses#Analysis#Analytics#anomalies#Apache#API#APIs#app#applications#approach#apps#architecture#automation#autoML#azure#benchmarks#Best Of
0 notes
Text
#advertising#advertising agency#conversion rate optimization#cro#digital marketing agency#facebook ads#google ads#google advertise#marketing#ppc agency#web analytics#google analytics#GTM#fb pixel & conversion api#server side tracking
1 note
·
View note
Text
Leveraging Django for Machine Learning Projects: A Comprehensive Guide
Using Django for Machine Learning Projects: Integration, Deployment, and Best Practices
Introduction:As machine learning (ML) continues to revolutionize various industries, integrating ML models into web applications has become increasingly important. Django, a robust Python web framework, provides an excellent foundation for deploying and managing machine learning projects. Whether you’re looking to build a web-based machine learning application, deploy models, or create APIs for…
0 notes
Text
Ranking Drop? Chill Out Google's Silent, But We Got You!

Ever see your website ranking plummet in search results, leaving you feeling ♀️♂️ and searching for answers?
Here's the truth: Google keeps its ranking algorithm a closely guarded secret. So, minor fluctuations or mysterious updates can cause ranking drops without a detailed explanation.
But don't despair! Focusing on these key areas will put you on the path to long-term ranking success:
High-Quality Content ✍️: Create informative, engaging content that solves problems and keeps users glued to your site.
Strong Technical SEO ⚙️: Ensure your website is mobile-friendly, loads fast, and has a clear structure.
Positive User Experience (UX): Make your website easy to navigate, visually appealing, and a joy to use.
By focusing on these fundamentals, you'll be well on your way to ranking high, even if Google stays silent on every drop.
#serpapi#SERPHouse#google#ranking#success#API#market research#business#analytics#software#bing#yahoo
1 note
·
View note
Text
Nemolizumab in the USA | Breakthrough in Targeted Therapy
Itchy skin disease with nodules: Prurigo nodularis is a chronic skin disease defined by itchy skin and hard itchy nodules on skin surface. This impacts approx. 181, 000 residents in the United States and has a great influence over the quality of life of sufferers because of sleep loss and other forms of stigmatization1. Specifically, Nemolizumab seems to have a promising efficacy in clinical modalities since it relieves itching and decreases the size of the nodules for patients.
#analytical development#product formulation#generic medicine company list#api suppliers in India#biologics and biosimilars#pharmaceutical labs near me
1 note
·
View note
Text
#cloud call center#cloud calling#real time analytics#voip technology#business voip#voip solutions#voice broadcasting#voice message#entrepreneur#b2b#whatsapp business api#bulk sms#sms api
0 notes
Text
What Is a Computer Vision Engineer? Unlocking the Power of Sight in Machines
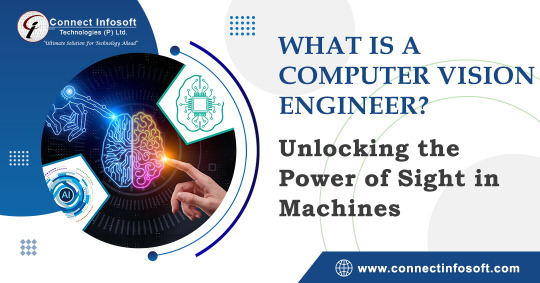
In today's rapidly evolving technological landscape, one of the most remarkable areas of development is computer vision. As humans, our ability to perceive and understand the visual world around us is a fundamental aspect of our daily lives. Similarly, the field of computer vision aims to enable machines to interpret and understand visual data. At the heart of this fascinating domain lies the computer vision engineer, an expert who plays a crucial role in unlocking the power of sight in machines.
In this article, we will delve into the world of computer vision engineering, exploring the responsibilities, skills, and potential applications of this field.
Understanding Computer Vision Engineering:
Computer vision engineering encompasses the design, development, and deployment of systems that enable machines to gain an understanding of visual data. The primary goal of computer vision engineers is to create algorithms and models that can accurately interpret images and videos, replicating human visual perception to some extent. By using artificial intelligence (AI) techniques such as machine learning and deep learning, computer vision engineers equip machines with the ability to analyze, recognize, and make decisions based on visual information.
Responsibilities of a Computer Vision Engineer:
The responsibilities of a computer vision engineer are diverse and demanding. They involve working with large datasets, developing and fine-tuning complex algorithms, and collaborating with cross-functional teams to implement computer vision solutions.
Some key responsibilities include:
1. Data Collection and Preprocessing: Computer vision engineers gather large volumes of visual data and preprocess it to enhance the accuracy of subsequent analysis. This often involves tasks such as data labeling, augmentation, and cleaning.
2. Algorithm Development: Computer vision engineers develop and optimize algorithms that can detect and recognize objects, people, gestures, and other visual cues. They leverage machine learning techniques, such as convolutional neural networks (CNNs), to train models on labeled data and improve their ability to make accurate predictions.
3. Model Evaluation and Optimization: Computer vision engineers evaluate the performance of trained models, fine-tuning them to achieve higher accuracy and robustness. They employ techniques like transfer learning and regularization to enhance the models' generalization capabilities.
4. Integration and Deployment: Once the computer vision systems are developed, engineers work on integrating them into real-world applications. This involves optimizing the models for efficiency, scalability, and compatibility with hardware and software frameworks.
Skills Required:
Becoming a proficient computer vision engineer requires a combination of technical skills and domain knowledge. Some essential skills include:
1. Programming: Proficiency in programming languages such as Python, C++, or MATLAB is crucial for implementing computer vision algorithms and working with relevant libraries and frameworks like OpenCV, TensorFlow, or PyTorch.
2. Mathematics and Statistics: A solid foundation in linear algebra, calculus, probability, and statistics is necessary to understand the mathematical underpinnings of computer vision algorithms and models.
3. Machine Learning: Familiarity with machine learning concepts and techniques is vital for training and fine-tuning models. Understanding topics like supervised and unsupervised learning, neural networks, and optimization algorithms is essential.
4. Image Processing: Knowledge of image processing techniques, such as filtering, segmentation, and feature extraction, allows computer vision engineers to manipulate and enhance visual data before feeding it into models.
5. Problem-Solving and Critical Thinking: Strong problem-solving and critical thinking skills enable computer vision engineers to tackle complex challenges and devise innovative solutions.
Applications of Computer Vision:
Computer vision has many uses in numerous industries. Some famous examples are:
1. Autonomous Vehicles: Computer vision enables self-driving cars to perceive and understand their surroundings, identifying objects, pedestrians, traffic signs, and lane markings to make informed decisions.
2. Healthcare: Computer vision aids in medical imaging analysis, assisting with tasks like tumor detection, disease diagnosis, and surgical planning. It also facilitates remote patient monitoring and analysis of vital signs.
3. Retail and E-commerce: Computer vision is used for product recognition and visual search, allowing customers to find similar products based on images. It also enables automated inventory management and checkout processes.
4. Security and Surveillance: Computer vision systems enhance security by detecting and tracking suspicious activities, recognizing faces, and analyzing video footage for real-time threat detection.
5. Augmented Reality (AR) and Virtual Reality (VR): Computer vision is instrumental in AR and VR applications, overlaying digital information in the real world or creating immersive virtual environments.
Conclusion:
Computer vision engineering is an exciting and rapidly evolving field that empowers machines with the ability to perceive and understand the visual world. By leveraging AI and machine learning techniques, computer vision engineers unlock the potential for machines to analyze, interpret, and make decisions based on visual data. With applications spanning industries like automotive, healthcare, retail, security, and entertainment, the impact of computer vision is revolutionizing our lives. As technology continues to advance, computer vision engineers will play an increasingly vital role in shaping the future of intelligent machines.
#Hire Machine Learning Developer#Machine Learning Development in India#Looking For Machine Learning Developer#Looking For Machine Learning Dev Team#Data Analytics Company#Vision AI Solution#Vision AI Development#Vision AI Software#Vision API#Vertex AI Vision#computer vision engineers#Computer Vision
0 notes
Text
Alentris Research Private Limited
At Alentris Research Private Limited, we are dedicated to advancing the field of impurity science since our founding in 2019. Our experienced team provides solutions for pharmaceutical, biopharmaceutical, and chemical companies seeking expertise in API Impurities, Working Standards, Analytical Standards, Degradation Impurities, Genotoxic Impurities, Organic Impurities, and satisfying Regulatory Requirements for impurity testing and identification. Leveraging state-of-the-art analytical instrumentation and methodologies, we specialize in the Isolation and Characterisation of both known and Unknown Impurities, enabling the Discovery of Impurities to support development, improve quality, and ensure safety. Get in touch to learn more about our end-to-end impurity management services.
With expertise in Impurity Research, our scientific team specializes in the custom Synthesis of Impurity standards including Nitrosamines Impurities, Oncology Impurities, and Peptide Impurities. We are dedicated to supporting pharmaceutical companies through expert Impurity services for drug development, quality control, and regulatory compliance. Contact us to learn more about our capabilities in impurity management.
We offer Force Degradation studies to assess impurity profiles and meet EP and USP Impurities guidelines. Our capabilities include Photolytic Degradation Impurity, Thermal Degradation Impurity, Oxidative Degradation Impurity, and Acid-Alkaline Degradation Impurity testing. For pharmaceutical companies, we provide impurity analysis and identification in drug substances, Formulation Impurities, degradation products, and more. Contact us to learn about our impurity management services for API and drug development.
1 note
·
View note
Text
Ifood Restaurant Data Scraping | Scrape Ifood Restaurant Data
Foodspark provides the Best Ifood Restaurant Data Scraping services in the USA, UK, Spain and China to extract or Scrape Ifood restaurant menu competitive pricing. Get the Best Ifood Restaurant Data Scraping API at affordable prices
#ifood restaurant data scraping#ifoods data scraping#web scraping tools#food data scraping#food data scraping services#zomato api#restaurant data scraping#grocerydatascrapingapi#fooddatascrapingservices#web scraping services#restaurant data analytics#seamless api#data scraping#data scraping services#restaurantdataextraction
0 notes