#DataOps Tools
Explore tagged Tumblr posts
Text
#DataOps Platform#Data Collaboration#Data Security#Data Workflow Automation#Cross-Functional Collaboration#DataOps Tools#Data Insights#Data Quality#DevOps#Data Pipelines
1 note
·
View note
Text
DataOps is a set of practices, processes, and technologies that combines an integrated and process-oriented perspective on data with automation and methods from agile software engineering to improve quality, speed, and collaboration and promote a culture of continuous improvement in the area of data analytics.
0 notes
Text
Simplifying Complex Data Operations with Smart Tools: Match Data Pro LLC Leading the Charge
In the data-driven economy, businesses are increasingly relying on accurate, actionable, and streamlined data to make informed decisions. But as the volume of data grows, so do the challenges: mismatched entries, inconsistent formats, manual data handling, and disconnected systems. That’s where Match Data Pro LLC steps in with robust, user-friendly solutions built to simplify the most complex data tasks.
From intuitive point-and-click data tools to enterprise-ready on-premise data software, Match Data Pro LLC offers a full suite of data ops software that helps businesses regain control of their data environments. Let’s explore how our tools are transforming data workflows and improving business intelligence across industries.
The Challenge of Mismatched Data
Modern businesses often operate across multiple platforms — CRMs, ERPs, marketing suites, accounting software, and more. With so many systems exchanging data, inconsistencies and mismatches are inevitable.
Common mismatched data issues include:
Duplicated records with minor variations
Inconsistent formatting across platforms
Incomplete or outdated entries
Data schema conflicts
These mismatches don’t just clutter your systems — they lead to flawed analytics, poor customer experiences, and inefficient operations. Match Data Pro LLC offers specialized mismatched data solutions that identify, resolve, and prevent these inconsistencies before they impact your business.
Mismatched Data Solutions That Actually Work
Our intelligent matching algorithms use fuzzy logic, pattern recognition, and customizable rules to identify mismatches across large datasets — whether it's customer records, product inventories, or financial transactions.
With our solutions, you can:
Detect and correct field-level mismatches
Merge records with varying structures
Align data formats across multiple systems
Automate reconciliation and cleanup processes
Whether your data is siloed in spreadsheets or flowing through APIs, Match Data Pro LLC helps you achieve consistency and reliability.
Empowering Users with Point-and-Click Data Tools
Not every business has a dedicated IT or data science team. That’s why Match Data Pro LLC designed point-and-click data tools — intuitive interfaces that empower non-technical users to manage, match, and clean data without writing a single line of code.
With our user-friendly dashboard, you can:
Drag and drop datasets for instant processing
Match records with customizable logic
Filter, group, and sort data visually
Schedule automated data operations
Generate real-time reports with one click
These tools are perfect for marketing teams, sales professionals, analysts, and operations managers who need quick results without technical overhead.
Optimize Workflow with Data Ops Software
DataOps, or data operations, is the practice of automating, monitoring, and improving the data pipeline across your organization. Match Data Pro LLC offers scalable data ops software that bridges the gap between IT and business, ensuring that clean, accurate data flows freely across systems.
Our DataOps platform supports:
Data ingestion and transformation
Real-time validation and matching
Workflow automation
Custom pipelines with REST API integration
End-to-end visibility into data flow
By implementing a robust DataOps framework, organizations can break down silos, accelerate decision-making, and reduce the time from data collection to business action.
On-Premise Data Software for Total Control
While cloud-based solutions offer flexibility, some businesses — especially in finance, healthcare, and government — require strict control over their data infrastructure. For these clients, Match Data Pro LLC provides secure, customizable on-premise data software.
With our on-premise solution, you get:
Full ownership of your data and environment
Greater compliance with regulatory standards (GDPR, HIPAA, etc.)
No dependency on third-party cloud providers
Seamless integration with legacy systems
Offline capabilities for remote or secure locations
Whether you're managing sensitive customer data or maintaining a private data warehouse, our on-premise offerings ensure peace of mind and operational integrity.
How Match Data Pro LLC Delivers Value
We understand that every organization’s data landscape is unique. That’s why we offer flexible configurations, expert support, and scalable features that grow with your business.
Key benefits of our platform:
Accurate data matching and cleaning
Automation that saves hours of manual effort
Tools accessible to both technical and non-technical users
API integration for seamless system connectivity
On-premise and cloud deployment options
Whether you’re a startup seeking better customer segmentation or a multinational enterprise trying to unify datasets across geographies, Match Data Pro LLC has a solution that fits.
Real-World Use Cases
Marketing: Clean up and deduplicate lead lists from multiple sources using point-and-click tools
Finance: Reconcile transactions across multiple ledgers with automated workflows
Retail: Align product data across warehouses, stores, and e-commerce platforms
Healthcare: Match patient records across systems while complying with data privacy regulations
Government: Maintain accurate citizen records with secure on-premise deployment
Our flexible software supports use cases across every industry — because clean, reliable data is a universal need.
Match Data Pro LLC: Your Data, Unified and Simplified
In a world driven by data, the ability to unify and streamline your information is what sets top-performing companies apart. Match Data Pro LLC provides the tools, support, and infrastructure to turn messy, mismatched datasets into clean, actionable intelligence.
With point-and-click data tools, mismatched data solutions, data ops software, and on-premise data software, we give you the power to take control of your data — and your business future.
0 notes
Text
The Ultimate Tableau Dashboard Testing Checklist

Ensuring the quality of a Tableau dashboard goes beyond building. It requires thorough testing to validate its reliability and usability. This tableau dashboard testing checklist focuses on essential aspects like verifying data accuracy, evaluating functionality, security testing to protect sensitive data, stress testing for performance under load, and visual testing to maintain clarity and design standards.
Aspects Involved in Testing the Tableau Dashboard

Testing Data Sources:
Ensure the dashboard is connected to the correct data sources and that credentials are set up properly.
Checking Data Accuracy:
1. Check whether the source data is reflected in the dashboard. This involves cross-checking the data on the dashboard with the data from the sources.
2. Verify that the calculated fields, aggregates, and measures are as expected.
Functionality Testing:
1. Report or dashboard design check.
2. Filters and parameters testing to see if they work as expected and do not display incorrect data. Also, if the dynamic changes to data visuals are applied and reflected.
3. Drilldown reports checking.
4. Ease of navigation, interactivity, and responsiveness in terms of usability.
Security Testing:
1. To check the security for report access and Row Level Security Permissions.
2. Integration of Single Sign On (SSO) security.
3. Multi-factor authentication.
Regression Testing:
Any change to the BI Dashboard/Model can impact the existing reports. It is important to perform regression testing so that after updates or modifications, the data/visuals shown in the dashboard remain the same before and after the changes.
youtube
Stress Testing:
To test the load time, run time, filter application time, and to simulate the access of reports and user behavior.
Visual Testing:
To check alignments, layouts, consistencies in terms of texts, visuals, or images to ensure all the elements are properly aligned.
How Does DataOps Suite BI Validator Enable Testing of Tableau Dashboards?

BI Validator is a no-code testing tool to automate the regression, stress, and functional testing of Tableau reports and dashboards.
Checking Data Accuracy:
DataOps Suite allows users to validate the data from the visuals of the report to be compared to the source databases. On connecting to Tableau and selecting a report, the datasets underlying the visuals of the reports are accessible through the suite as well. Each Visual has its own dataset, which can be compared to a source database used to build the tableau report.
This is possible with the help of the Data Compare component in the suite, which can compare the aggregated data from the databases and the datasets of the visuals. The differences are captured, thus allowing users to check the data accuracy between the reports and databases.
Functionality Testing and Visual Testing:
Once the connection is established, the users can access the reports and the exact workspace to work with. The specific workbook/report is accessible. The report will be loaded without any changes, and the visuals and filters of the report can be accessed from the BI Validator itself, thus verifying the responsiveness of the visuals and filters and verifying whether the dynamic changes are reflected.
The BI Validator comes with the Tableau Upgrade component to compare two reports, which can be the same or different from one or different data sources.
A common use case is the comparison of views and worksheets across multiple environments. Comparison can be done in terms of both text and appearance, where the differences are captured and pointed out wherever mismatch occurs in both reports. Also, BI Validator allows the differences in the filters of both reports to be pointed out on enabling “Capture filters after applying.”.
youtube
Security Testing:
BI Validator connects with Tableau Testing through direct trust authentication, default authentication, or personal access token, where the users must provide their respective Tableau credentials, secret key, and secret ID (in direct trust connection) and the necessary web login commands (for default or personal access token connection). Thus, ensuring the authentication is secure so that only the users with valid credentials are accessing their reports to be validated.
BI Validator restricts the users from downloading the testing results when it comes to BI Reports to prevent the sensitive information from being downloaded.
The DataOps suite also has user-based access through roles and containers to prevent access to reports for everyone. The BI Validator can only allow users with defined roles and permissions to access reports.
Regression Testing:
BI Validator supports regression testing of the reports through the Tableau Regression component, which automates the testing of Tableau reports during any in-place upgrades and workbook deployments. This testing happens by comparing a benchmarked/baseline version of the dashboard/report with the live version. The filters can be changed accordingly if needed before/after the benchmarking. These filter changes can be bookmarked as the latest checkpoint before running the test.
Similar to upgrades, regression test runs can validate the differences in terms of appearance or text. Also, differences in the filters can be pointed out on enabling “capture filters after applying.”
Stress Testing:
BI Validator comes with a stress test plan to simulate concurrent users accessing reports to evaluate how reports and dashboards perform under heavy load. The plan typically involves running multiple users through different types of interactions, such as viewing reports, applying filters, refreshing data, and interacting with custom visuals.
The stress test plan allows the users to select the pages/dashboards from required workspaces to perform stress testing. These pages can be either from the same or different report.
Users can run the stress test plan on specific run options like number of parallel users, time spent on each page, total simulation run time, number of seconds to reach parallel users, refresh time, and other options to run the stress test plan.
The runs will result in showing metrics like Average Open Time and Max Open Time, Average Filter Apply Time, SLA Failures.
#datagaps#Tableau#Tableau Testing#Tableau dashboard#Tableau Testing Checklist#BI Validator#BI#Youtube
0 notes
Text
Data Architect
Designing the target architecture for data platform along with tools and processes by evaluating existing platform and future trends. Azure data stack with fabric and Databricks, open source data stack knowledge Exposure to dataops ecosystem and tools would be plus. The strategy outcome would have target architecture, tools to be implemented, design patterns etc., Apply Now
0 notes
Text
Unlocking the Potential of Your Data: A Guide to Modern Data Engineering Services
In today's digital-first world, data has become the backbone of business success. From enhancing decision-making to driving innovation, the value of data cannot be overstated. But how do businesses ensure that their data is ready to deliver on its promise? Enter data engineering services — the bridge between raw data and actionable insights.
This blog explores the latest trends, best practices, and unique strategies in data engineering, helping organizations leverage data to stay ahead of the curve.
1. The Role of Data Engineering in Modern Businesses
Data engineering is more than just managing databases or building pipelines. It's about creating robust frameworks for data collection, transformation, and storage while ensuring quality and accessibility. Businesses today demand real-time analytics, predictive modeling, and seamless integrations — all of which hinge on well-engineered data systems.
2. Top Trends Transforming Data Engineering Services
a. Rise of Cloud Data Platforms Cloud-native data engineering solutions like Snowflake, Databricks, and BigQuery are revolutionizing how businesses store and process data. They offer scalability, flexibility, and cost efficiency.
b. DataOps for Agile Data Management DataOps combines DevOps principles with data engineering, enabling faster and more reliable data delivery. Automation and CI/CD pipelines for data workflows are becoming the norm.
c. Focus on Data Observability Ensuring data reliability is critical. Tools like Monte Carlo and Datadog are helping organizations proactively monitor and address data quality issues.
d. Integration of AI and Machine Learning Data engineering services now incorporate AI and ML models to automate routine tasks like data mapping, anomaly detection, and schema generation.
3. Benefits of Investing in Data Engineering Services
Improved Decision-Making: Access to clean, structured, and actionable data enables faster and better decisions.
Cost Efficiency: Efficient data pipelines reduce resource wastage and infrastructure costs.
Enhanced Compliance: Modern solutions ensure adherence to data privacy regulations like GDPR and CCPA.
Scalability: With the right data engineering services, businesses can handle growing data volumes seamlessly.
4. Key Components of Effective Data Engineering Solutions
Data Architecture Design: Structuring data ecosystems for optimal performance.
ETL/ELT Processes: Building automated workflows for data extraction, transformation, and loading.
Real-Time Data Processing: Leveraging tools like Apache Kafka and Spark for instantaneous data insights.
Data Governance and Security: Ensuring data integrity, privacy, and compliance with robust frameworks.
5. How to Choose the Right Data Engineering Service Provider
When selecting a data engineering partner, consider:
Their expertise in modern tools and platforms.
Proven case studies and success stories.
Scalability of their solutions.
Focus on data security and governance.
Conclusion The demand for data engineering services is only set to grow as businesses increasingly rely on data-driven strategies. By embracing cutting-edge trends like DataOps, cloud platforms, and AI-driven automation, organizations can unlock the full potential of their data. Investing in the right data engineering solutions today will pave the way for sustained success tomorrow.
0 notes
Text
IBM Watsonx.data Offers VSCode, DBT & Airflow Dataops Tools
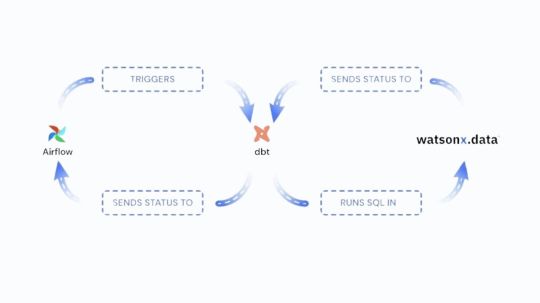
We are happy to inform that VSCode, Apache Airflow, and data-build-tool a potent set of tools for the contemporary dataops stack are now supported by IBM watsonx.data. IBM Watsonx.data delivers a new set of rich capabilities, including data build tool (dbt) compatibility for both Spark and Presto engines, automated orchestration with Apache Airflow, and an integrated development environment via VSCode. These functionalities enable teams to effectively construct, oversee, and coordinate data pipelines.
The difficulty with intricate data pipelines
Building and maintaining complicated data pipelines that depend on several engines and environments is a challenge that organizations must now overcome. Teams must continuously move between different languages and tools, which slows down development and adds complexity.
It can be challenging to coordinate workflows across many platforms, which can result in inefficiencies and bottlenecks. Data delivery slows down in the absence of a smooth orchestration tool, which postpones important decision-making.
A coordinated strategy
Organizations want a unified, efficient solution that manages process orchestration and data transformations in order to meet these issues. Through the implementation of an automated orchestration tool and a single, standardized language for transformations, teams can streamline their workflows, facilitating communication and lowering the difficulty of pipeline maintenance. Here’s where Apache Airflow and DBT come into play.
Teams no longer need to learn more complicated languages like PySpark or Scala because dbt makes it possible to develop modular structured query language (SQL) code for data transformations. The majority of data teams are already familiar with SQL, thus database technology makes it easier to create, manage, and update transformations over time.
Throughout the pipeline, Apache Airflow automates and schedules jobs to minimize manual labor and lower mistake rates. When combined, dbt and Airflow offer a strong framework for easier and more effective management of complicated data pipelines.
Utilizing IBM watsonx.data to tie everything together
Although strong solutions like Apache Airflow and DBT are available, managing a developing data ecosystem calls for more than just a single tool. IBM Watsonx.data adds the scalability, security, and dependability of an enterprise-grade platform to the advantages of these tools. Through the integration of VSCode, Airflow, and DBT within watsonx.data, it has developed a comprehensive solution that makes complex data pipeline management easier:
By making data transformations with SQL simpler, dbt assists teams in avoiding the intricacy of less used languages.
By automating orchestration, Airflow streamlines processes and gets rid of bottlenecks.
VSCode offers developers a comfortable environment that improves teamwork and efficiency.
This combination makes pipeline management easier, freeing your teams to concentrate on what matters most: achieving tangible business results. IBM Watsonx.data‘s integrated solutions enable teams to maintain agility while optimizing data procedures.
Data Build Tool’s Spark adaptor
The data build tool (dbt) adapter dbt-watsonx-spark is intended to link Apache Spark with dbt Core. This adaptor facilitates Spark data model development, testing, and documentation.
FAQs
What is data build tool?
A transformation workflow called dbt enables you to complete more tasks with greater quality. Dbt can help you centralize and modularize your analytics code while giving your data team the kind of checks and balances that are usually seen in software engineering workflows. Before securely delivering data models to production with monitoring and visibility, work together on them, version them, test them, and record your queries.
DBT allows you and your team to work together on a single source of truth for metrics, insights, and business definitions by compiling and running your analytics code against your data platform. Having a single source of truth and the ability to create tests for your data helps to minimize errors when logic shifts and notify you when problems occur.
Read more on govindhtech.com
#IBMWatsonx#dataOffer#VSCode#DBT#data#ApacheSpark#ApacheAirflow#Watsonxdata#DataopsTools#databuildtool#Sparkadaptor#UtilizingIBMwatsonxdata#technology#technews#news#govindhteh
0 notes
Text
Basil Faruqui, BMC Software: How to nail your data and AI strategy - AI News
New Post has been published on https://thedigitalinsider.com/basil-faruqui-bmc-software-how-to-nail-your-data-and-ai-strategy-ai-news/
Basil Faruqui, BMC Software: How to nail your data and AI strategy - AI News
.pp-multiple-authors-boxes-wrapper display:none; img width:100%;
BMC Software’s director of solutions marketing, Basil Faruqui, discusses the importance of DataOps, data orchestration, and the role of AI in optimising complex workflow automation for business success.
What have been the latest developments at BMC?
It’s exciting times at BMC and particularly our Control-M product line, as we are continuing to help some of the largest companies around the world in automating and orchestrating business outcomes that are dependent on complex workflows. A big focus of our strategy has been on DataOps specifically on orchestration within the DataOps practice. During the last twelve months we have delivered over seventy integrations to serverless and PaaS offerings across AWS, Azure and GCP enabling our customers to rapidly bring modern cloud services into their Control-M orchestration patterns. Plus, we are prototyping GenAI based use cases to accelerate workflow development and run-time optimisation.
What are the latest trends you’ve noticed developing in DataOps?
What we are seeing in the Data world in general is continued investment in data and analytics software. Analysts estimate that the spend on Data and Analytics software last year was in the $100 billion plus range. If we look at the Machine Learning, Artificial Intelligence & Data Landscape that Matt Turck at Firstmark publishes every year, its more crowded than ever before. It has 2,011 logos and over five hundred were added since 2023. Given this rapid growth of tools and investment, DataOps is now taking center stage as companies are realising that to successfully operationalise data initiatives, they can no longer just add more engineers. DataOps practices are now becoming the blueprint for scaling these initiatives in production. The recent boom of GenAI is going make this operational model even more important.
What should companies be mindful of when trying to create a data strategy?
As I mentioned earlier that the investment in data initiatives from business executives, CEOs, CMOs, CFOs etc. continues to be strong. This investment is not just for creating incremental efficiencies but for game changing, transformational business outcomes as well. This means that three things become very important. First is clear alignment of the data strategy with the business goals, making sure the technology teams are working on what matters the most to the business. Second, is data quality and accessibility, the quality of the data is critical. Poor data quality will lead to inaccurate insights. Equally important is ensuring data accessibility – making the right data available to the right people at the right time. Democratising data access, while maintaining appropriate controls, empowers teams across the organisation to make data-driven decisions. Third is achieving scale in production. The strategy must ensure that Ops readiness is baked into the data engineering practices so its not something that gets considered after piloting only.
How important is data orchestration as part of a company’s overall strategy?
Data Orchestration is arguably the most important pillar of DataOps. Most organisations have data spread across multiple systems – cloud, on-premises, legacy databases, and third-party applications. The ability to integrate and orchestrate these disparate data sources into a unified system is critical. Proper data orchestration ensures seamless data flow between systems, minimising duplication, latency, and bottlenecks, while supporting timely decision-making.
What do your customers tell you are their biggest difficulties when it comes to data orchestration?
Organisations continue to face the challenge of delivering data products fast and then scaling quickly in production. GenAI is a good example of this. CEOs and boards around the world are asking for quick results as they sense that this could majorly disrupt those who cannot harness its power. GenAI is mainstreaming practices such as prompt engineering, prompt chaining etc. The challenge is how do we take LLMs and vector databases, bots etc and fit them into the larger data pipeline which traverses a very hybrid architecture from multiple-clouds to on-prem including mainframes for many. This just reiterates the need for a strategic approach to orchestration which would allow folding new technologies and practices for scalable automation of data pipelines. One customer described Control-M as a power strip of orchestration where they can plug in new technologies and patterns as they emerge without having to rewire every time they swap older technologies for newer ones.
What are your top tips for ensuring optimum data orchestration?
There can be a number of top tips but I will focus on one, interoperability between application and data workflows which I believe is critical for achieving scale and speed in production. Orchestrating data pipelines is important, but it is vital to keep in mind that these pipelines are part of a larger ecosystem in the enterprise. Let’s consider an ML pipeline is deployed to predict the customers that are likely to switch to a competitor. The data that comes into such a pipeline is a result of workflows that ran in the ERP/CRM and combination of other applications. Successful completion of the application workflows is often a pre-requisite to triggering the data workflows. Once the model identifies customers that are likely to switch, the next step perhaps is to send them a promotional offer which means that we will need to go back to the application layer in the ERP and CRM. Control-M is uniquely positioned to solve this challenge as our customers use it to orchestrate and manage intricate dependencies between the application and the data layer.
What do you see as being the main opportunities and challenges when deploying AI?
AI and specifically GenAI is rapidly increasing the technologies involved in the data ecosystem. Lots of new models, vector databases and new automation patterns around prompt chaining etc. This challenge is not new to the data world, but the pace of change is picking up. From an orchestration perspective we see tremendous opportunities with our customers because we offer a highly adaptable platform for orchestration where they can fold these tools and patterns into their existing workflows versus going back to drawing board.
Do you have any case studies you could share with us of companies successfully utilising AI?
Domino’s Pizza leverages Control-M for orchestrating its vast and complex data pipelines. With over 20,000 stores globally, Domino’s manages more than 3,000 data pipelines that funnel data from diverse sources such as internal supply chain systems, sales data, and third-party integrations. This data from applications needs to go through complex transformation patterns and models before its available for driving decisions related to food quality, customer satisfaction, and operational efficiency across its franchise network.
Control-M plays a crucial role in orchestrating these data workflows, ensuring seamless integration across a wide range of technologies like MicroStrategy, AMQ, Apache Kafka, Confluent, GreenPlum, Couchbase, Talend, SQL Server, and Power BI, to name a few.
Beyond just connecting complex orchestration patterns together Control-M provides them with end-to-end visibility of pipelines, ensuring that they meet strict service-level agreements (SLAs) while handling increasing data volumes. Control-M is helping them generate critical reports faster, deliver insights to franchisees, and scale the roll out new business services.
What can we expect from BMC in the year ahead?
Our strategy for Control-M at BMC will stay focused on a couple of basic principles:
Continue to allow our customers to use Control-M as a single point of control for orchestration as they onboard modern technologies, particularly on the public cloud. This means we will continue to provide new integrations to all major public cloud providers to ensure they can use Control-M to orchestrate workflows across three major cloud infrastructure models of IaaS, Containers and PaaS (Serverless Cloud Services). We plan to continue our strong focus on serverless, and you will see more out-of-the-box integrations from Control-M to support the PaaS model.
We recognise that enterprise orchestration is a team sport, which involves coordination across engineering, operations and business users. And, with this in mind, we plan to bring a user experience and interface that is persona based so that collaboration is frictionless.
Specifically, within DataOps we are looking at the intersection of orchestration and data quality with a specific focus on making data quality a first-class citizen within application and data workflows. Stay tuned for more on this front!
Want to learn more about AI and big data from industry leaders? Check out AI & Big Data Expo taking place in Amsterdam, California, and London. The comprehensive event is co-located with other leading events including Intelligent Automation Conference, BlockX, Digital Transformation Week, and Cyber Security & Cloud Expo.
Explore other upcoming enterprise technology events and webinars powered by TechForge here.
Tags: automation, BMC, data orchestration, DataOps
#000#2023#Accessibility#ADD#ai#ai & big data expo#ai news#AI strategy#amp#Analytics#Apache#apache kafka#application layer#applications#approach#architecture#artificial#Artificial Intelligence#automation#AWS#azure#bi#Big Data#billion#BMC#board#boards#bots#box#Business
0 notes
Text
Data Engineering Services Explained: What Lies Ahead for the Industry
In an era where data shapes every aspect of business decision-making, organizations are turning to data engineering to harness its full potential. As data volumes and complexities escalate, the demand for specialized data engineering services has surged. This article delves into the core components of data engineering services and offers insights into the evolving future of this critical field.
What are Data Engineering Services?
Data engineering involves the design, construction, and maintenance of systems and infrastructure that allow for the collection, storage, processing, and analysis of data. Data engineering services encompass a variety of tasks and functions that ensure data is accessible, reliable, and usable for data scientists, analysts, and business stakeholders. Key components of data engineering services include:
1. Data Architecture
Data engineers are responsible for designing data architectures that define how data is collected, stored, and accessed. This includes selecting appropriate databases, data lakes, and data warehouses to optimize performance and scalability.
2. Data Integration
Data often comes from multiple sources, including transactional systems, external APIs, and sensor data. Data engineering services involve creating ETL (Extract, Transform, Load) processes that integrate data from these various sources into a unified format.
3. Data Quality and Governance
Ensuring data quality is critical for accurate analysis. Data engineers implement data validation and cleansing processes to identify and rectify errors. They also establish governance frameworks to maintain data integrity and compliance with regulations.
4. Data Pipeline Development
Data pipelines automate the flow of data from its source to storage and processing systems. Data engineering services focus on building efficient pipelines that can handle large volumes of data while ensuring minimal latency.
5. Performance Optimization
As organizations scale, performance becomes a crucial consideration. Data engineers optimize databases and pipelines for speed and efficiency, enabling faster data retrieval and processing.
6. Collaboration with Data Teams
Data engineers work closely with data scientists, analysts, and other stakeholders to understand their data needs. This collaboration ensures that the data infrastructure supports analytical initiatives effectively.
The Future of Data Engineering
As the field of data engineering evolves, several trends are shaping its future:
1. Increased Automation
Automation is set to revolutionize data engineering. Tools and platforms are emerging that automate repetitive tasks such as data cleansing, pipeline management, and monitoring. This will allow data engineers to focus on more strategic initiatives rather than manual processes.
2. Real-time Data Processing
With the rise of IoT devices and streaming applications, the demand for real-time data processing is growing. Future data engineering services will increasingly incorporate technologies like Apache Kafka and Apache Flink to facilitate real-time data ingestion and analytics.
3. Cloud-based Solutions
Cloud computing is becoming the norm for data storage and processing. Data engineering services will continue to leverage cloud platforms like AWS, Google Cloud, and Azure, offering greater scalability, flexibility, and cost-effectiveness.
4. DataOps
DataOps is an emerging discipline that applies agile methodologies to data management. It emphasizes collaboration, continuous integration, and automation in data pipelines. As organizations adopt DataOps, the role of data engineers will shift toward ensuring seamless collaboration across data teams.
5. Focus on Data Security and Privacy
With growing concerns about data security and privacy, data engineers will play a vital role in implementing security measures and ensuring compliance with regulations like GDPR and CCPA. Future services will prioritize data protection as a foundational element of data architecture.
6. Integration of AI and Machine Learning
Data engineering will increasingly intersect with artificial intelligence and machine learning. Data engineers will need to build infrastructures that support machine learning models, ensuring they have access to clean, structured data for training and inference.
Conclusion
Data engineering services are essential for organizations seeking to harness the power of data. As technology continues to advance, the future of data engineering promises to be dynamic and transformative. With a focus on automation, real-time processing, cloud solutions, and security, data engineers will be at the forefront of driving data strategy and innovation. Embracing these trends will enable organizations to make informed decisions, optimize operations, and ultimately gain a competitive edge in their respective industries.
#Data Engineering Services#Data Security#Data Privacy#Future of Data Engineering#Data Architecture#Data Governance
0 notes
Text
DataOps is a set of practices, processes, and technologies that combines an integrated and process-oriented perspective on data with automation and methods from agile software engineering to improve quality, speed, and collaboration and promote a culture of continuous improvement in the area of data analytics.
0 notes
Text
Data Science with Generative AI Training Hyderabad | Data Science Training
The Future of Data Science? Key Trends to Watch
Introduction Data Science with Generative AI Course continues to transform industries, driving decision-making, innovation, and efficiency. With the rapid advancement of technology, the field is evolving at a breakneck pace. From automation to ethical AI, data science is entering an exciting new era. This article highlights the key trends shaping the future of data science and what to expect as the discipline continues to grow. Data Science Course in Hyderabad

AI and Machine Learning Integration AI and machine learning (ML) are at the heart of data science advancements. The ability to automate complex tasks and generate insights is driving innovation in various sectors.
Automated Data Processing: AI can streamline data cleaning and preparation, reducing the manual labor required by data scientists.
Predictive Analytics: ML models will become even more sophisticated, leading to better forecasting and real-time decision-making.
AI-Powered Applications: Expect more integration of AI into everyday software and business processes, improving productivity.
Augmented Analytics Augmented analytics leverages AI to enhance data analysis. This trend democratizes data science by making analytics accessible to a broader range of users.
Self-Service Tools: Businesses will see an increase in user-friendly platforms that allow non-technical users to generate insights without needing a data scientist.
AI-Driven Insights: Automation will help uncover hidden patterns in data, speeding up the decision-making process.
Ethical Ai and Responsible Data Usage As AI grows in prominence, ethical concerns around data privacy, bias, and transparency are gaining attention.
Bias Mitigation: Efforts to reduce algorithmic bias will intensify, ensuring AI models are fair and inclusive.
Privacy Protection: Stricter regulations will push companies to prioritize data privacy and security, promoting responsible use of data.
The Rise of DataOps DataOps, the data-focused counterpart to DevOps, will become central to managing data pipelines efficiently.
Automation: Expect greater automation in data workflows, from data integration to deployment.
Collaboration: DataOps encourages better collaboration between data scientists, engineers, and operations teams, improving the speed and quality of data-driven projects.
Real-Time Analytics As businesses demand faster insights, real-time analytics is set to become a significant focus in data science.
Streaming Data: The rise of IoT devices and social media increases the demand for systems that can process and analyze data in real time. Data Science Training Institute in Hyderabad
Faster Decision-Making: Real-time analytics will enable organizations to make more immediate and informed decisions, improving responsiveness to market changes.
Conclusion The future of data science is promising, with trends like AI integration, ethical practices, and real-time analytics reshaping the field. These innovations will empower businesses to harness data's full potential while navigating the challenges that come with responsible and effective data management.
Visualpath is the Leading and Best Institute for learning in Hyderabad. We provide Data Science with Generative AI Training Hyderabad you will get the best course at an affordable cost.
Attend Free Demo
Call on – +91-9989971070
Visit blog: https://visualpathblogs.com/
WhatsApp: https://www.whatsapp.com/catalog/919989971070/
Visit: https://visualpath.in/data-science-with-generative-ai-online-training.html
#Data Science with Generative AI Course#Data Science with Generative AI Training Hyderabad#Data Science Training in Hyderabad#Data Science Training in Ameerpet#Data Science Training Institute in Hyderabad#Data Science Course Training in Hyderabad#Data Science with Generative AI Online Training#Data Science with Generative AI Training#Data Science with Generative AI Course Ameerpet#Data Science with Generative AI Course Hyderabad#Data Science Course in Hyderabad
0 notes
Text
Optimizing Data Management with Mismatched Data Solutions and Data Ops Software
In the fast-paced world of data management, organizations struggle with data inconsistencies, inefficient processing, and mismatched data issues that hinder operations. Businesses need robust mismatched data solutions and advanced data ops software to optimize workflows, ensure data accuracy, and improve decision-making. Match Data Pro LLC provides cutting-edge solutions to handle data challenges effectively, offering seamless integration and automation capabilities for streamlined data processing.
Understanding Mismatched Data Challenges
Data mismatches occur when datasets fail to align correctly due to discrepancies in formats, missing values, or duplicate entries. These inconsistencies can result in poor analytics, incorrect reporting, and flawed decision-making. Organizations dealing with vast amounts of information require mismatched data solutions that can identify, correct, and prevent errors efficiently.
Common Causes of Mismatched Data
Inconsistent Formatting – Variations in date formats, numerical representation, and naming conventions.
Duplicate Records – Repetitive entries causing redundancy and inaccuracy.
Data Entry Errors – Human errors leading to incorrect or incomplete data.
Integration Issues – Discrepancies arising when merging data from multiple sources.
Data Decay – Outdated or obsolete information affecting relevance and accuracy.
The Role of Mismatched Data Solutions
To overcome these challenges, businesses rely on specialized mismatched data solutionsthat offer:
Automated Data Cleansing – Identifying and correcting errors with minimal manual intervention.
Data Matching Algorithms – Ensuring accurate record linkage across different datasets.
Standardization Processes – Unifying data formats to maintain consistency.
Data Validation Techniques – Ensuring the reliability and completeness of datasets.
Match Data Pro LLC delivers state-of-the-art mismatched data solutions, providing businesses with powerful tools to enhance data quality and integrity.
Data Ops Software: Enhancing Operational Efficiency
Data operations, commonly known as DataOps, involve the automation, monitoring, and governance of data workflows. Data ops software plays a crucial role in optimizing data pipelines, reducing errors, and accelerating data-driven decision-making.
Key Features of Data Ops Software
Automated Workflow Orchestration – Streamlining data processing tasks without manual intervention.
Real-Time Monitoring & Alerts – Identifying anomalies and performance issues promptly.
Data Integration Capabilities – Seamlessly connecting various data sources.
Scalability & Flexibility – Adapting to growing data demands.
Security & Compliance – Ensuring data governance and regulatory compliance.
Benefits of Implementing Data Ops Software
Businesses leveraging data ops software gain several advantages, including:
Improved Data Accuracy – Ensuring reliable and consistent datasets.
Increased Productivity – Reducing time spent on manual data processing.
Faster Decision-Making – Providing real-time insights for strategic planning.
Enhanced Collaboration – Facilitating seamless communication between data teams.
Cost Efficiency – Reducing expenses associated with data errors and inefficiencies.
Match Data Pro LLC: Your Trusted Partner for Data Management
As a leading provider of mismatched data solutions and data ops software, Match Data Pro LLC helps businesses streamline data operations, reduce mismatches, and enhance efficiency. Their comprehensive solutions cater to organizations of all sizes, ensuring seamless data management through:
On-Premise & Cloud-Based Solutions – Flexible deployment options to suit business needs.
Bulk Data Processing Capabilities – Handling large datasets efficiently.
Point-and-Click Data Tools – User-friendly interfaces for simplified data management.
REST API Integration – Seamless connectivity with existing systems.
Automated Data Scheduling & Pipelines – Enabling end-to-end automation.
Conclusion
Data inconsistencies and inefficiencies can severely impact business operations. Implementing robust mismatched data solutions and leveraging data ops software can enhance data accuracy, streamline workflows, and boost decision-making. Match Data Pro LLC offers industry-leading solutions that empower businesses with automated, scalable, and efficient data management tools.
0 notes
Text
How to Train Your Team to Use a DataOps Platform Effectively

Organizations are depending more and more on massive volumes of data in today’s data-driven world in order to spur innovation, make wise decisions, and gain a competitive edge. Effectively managing and improving data processes is a major difficulty, though. A DataOps platform can be useful in this situation.
A DataOps Platform: What Is It?
A complete solution created to improve and expedite the data management and analytics processes is called a DataOps platform. DataOps is an approach to data management that takes cues from software development processes known as DevOps. Its objective is to ensure high standards of quality, dependability, and compliance while expediting the production and delivery of data products.
Important attributes and advantages
a. Automated Data Pipelines: The automation of data pipelines is a fundamental component of data operations platforms. This entails reducing manual involvement and error-proneness by automating the extraction, transformation, and loading (ETL) procedures. Faster data processing is made possible by automated pipelines, which facilitate the integration and analysis of data from several sources.
b. Enhanced Data Quality: Accurate analytics and reporting depend on high-quality data. Data validation and cleaning techniques are included into DataOps systems to assist find and fix problems with data quality early in the pipeline. As a result, decisions are made more effectively and with more reliability.
c. Enhanced Collaboration: By dismantling silos between data scientists, business analysts, and data engineers, DataOps platform encourages a collaborative approach. The platform facilitates a more integrated and effective workflow by offering features like version control, shared workspaces, and real-time communication capabilities.
d. Flexibility and Scalability: As businesses expand, so do their data requirements. A data operations platform is made to grow with the company, adapting to changing needs and bigger data volumes. Additionally, it supports a broad range of data integration situations by providing flexibility with regard to data sources and destinations.
e. Governance and Compliance: DataOps systems come with strong governance and compliance tools to assist enterprises in following legal obligations and data security guidelines. These characteristics lower the possibility of compliance problems by guaranteeing that data management procedures are open and auditable.
The DataOps Future
With the integration of cutting-edge technologies like artificial intelligence and machine learning into these platforms, the world of data operations is fast changing. More advanced automation features, improved predictive analytics, and deeper interaction with cloud-based services are possible future advances.
A data operations platform will be crucial in guaranteeing that data processes are effective, dependable, and in line with corporate objectives as companies continue to leverage the power of data. In an increasingly data-centric world, companies may preserve a competitive edge and open up new avenues for innovation by using a DataOps strategy.
0 notes
Text
What are the key trends shaping the future of data science?
Hi,
The field of data science is continuously evolving, and several key trends are shaping its future. Here are some of the most significant trends:
Generative AI: Generative AI involves creating new content, such as text, images, and videos, using machine learning models. Technologies like Generative Adversarial Networks (GANs) are pushing the boundaries of what AI can create, leading to innovative applications in art, design, and media.
Ethical AI: As AI technologies become more prevalent, there is increasing focus on ethical considerations. This includes addressing biases in AI models, ensuring fairness, and implementing practices that promote transparency and accountability in AI systems.
Augmented Analytics: Augmented analytics uses AI and machine learning to enhance data analysis processes. This includes automating data preparation, generating insights, and providing recommendations. Augmented analytics aims to make data analysis more accessible and efficient.
Edge Computing: Edge computing involves processing data closer to the source rather than relying on centralized cloud servers. This trend is driven by the need for faster data processing and reduced latency, especially for applications like IoT and real-time analytics.
DataOps: DataOps is an agile methodology for managing data operations, similar to DevOps in software engineering. It focuses on improving the efficiency and quality of data processes through automation, collaboration, and continuous integration.
Interdisciplinary Collaboration: Data science is increasingly collaborating with other fields, such as business, healthcare, and engineering. This interdisciplinary approach helps in addressing complex problems and developing solutions that are both technically sound and practically relevant.
Self-Service Analytics: There is a growing trend towards self-service analytics, where users can access and analyze data without relying on IT or data experts. This trend is supported by user-friendly tools and platforms that empower business users to perform their own data analysis.
Advanced Privacy Measures: As data privacy concerns grow, there is an emphasis on implementing advanced privacy measures, such as encryption and anonymization techniques. These measures help protect sensitive data while still allowing for valuable insights to be gained.
In summary, key trends shaping the future of data science include advancements in AI, ethical considerations, augmented analytics, edge computing, DataOps, interdisciplinary collaboration, self-service analytics, and privacy measures.
These trends are driving innovation and transforming how data science is applied across various domains.
0 notes
Text
Conquer Your Data Challenges with Web Age Solutions' Data Engineering Courses
Organizations are constantly bombarded with information. The ability to effectively collect, store, transform, and analyze this information is crucial for gaining valuable insights and making informed decisions. This is where data engineering comes in. Data engineers are the architects of the data pipelines that power these insights.
Web Age Solutions empowers you to build a skilled data engineering team with our comprehensive suite of data engineering courses. Led by industry experts, our courses provide a blend of theoretical knowledge and practical application, equipping you with the skills to tackle critical data challenges head-on.
What You'll Gain from Our Data Engineering Courses:
Master Data Wrangling with Python: The "Data Engineering with Python" course delves into the practical application of Python, a widely used programming language in data engineering. You'll gain proficiency in popular Python libraries like NumPy, pandas, and Matplotlib, enabling you to manipulate, analyze, and visualize data effectively.
Bridge the Gap for Data-Driven Leaders: The "Data Engineering for Managers" course is designed for business leaders who want to understand the fundamentals of data engineering and its role in driving business outcomes. This course equips you to make informed decisions about data infrastructure and effectively communicate with your data engineering team.
Harness the Power of Operational Data Analytics: "Operational Data Analytics with Splunk" introduces you to the Splunk platform, a powerful tool for analyzing machine-generated data. Through hands-on exercises, you'll learn to onboard and forward data, monitor systems in real-time, and create informative data visualizations.
Embrace Cloud-Based Data Processing: "Cloud Data Engineering with NiFi on AWS or GCP" equips you with the skills to leverage Apache NiFi, a visual programming tool, for data processing on cloud platforms like AWS and GCP. This course blends theoretical concepts with practical guidance to help you automate data movement and transformation within cloud environments.
Build a Solid Foundation in Data Engineering: "Data Engineering, ETL and DataOps" provides a comprehensive introduction to core data engineering principles. You'll gain hands-on experience with ETL (Extract, Transform, Load) processes, data manipulation with pandas, data visualization using Python, and essential data operations (DataOps) practices. Additionally, the course covers Apache Spark and Spark SQL, further equipping you for success in data engineering and data analytics roles.
Investing in Your Data Engineering Skills:
By empowering your team with data engineering expertise, you unlock a wealth of benefits for your organization. Data engineers can help you:
Optimize Risk and Performance: Analyze data to identify potential risks and opportunities, allowing for proactive decision-making.
Enhance Data Quality: Implement processes and tools to ensure data accuracy and consistency, leading to reliable insights.
Forecast with Precision: Leverage data to predict future trends and customer behavior, enabling informed business strategies.
Streamline Business Processes: Automate data-driven workflows, improving efficiency and productivity across your organization.
Web Age Solutions: Your Partner in Data Engineering Education
Our data engineering courses are designed to cater to a wide range of learners, from beginners seeking a foundational understanding to experienced professionals looking to refine their skillset. We offer flexible delivery options, including on-site and instructor-led virtual classes, to accommodate your team's learning preferences.
Ready to unlock the power of your data? Browse our complete course catalog or contact Web Age Solutions today to discuss your data engineering training needs. With expert-led instruction and a practical approach, we will equip your team with the skills to transform your data into actionable insights.
For more information visit: https://www.webagesolutions.com/courses/data-engineering-training
0 notes
Text
The Future of Data Operations: Trends and Predictions

Introduction
Data is the lifeblood of modern businesses, and the field of data operations (DataOps) is evolving at a rapid pace to meet the growing demands for data-driven decision-making, optimization, and innovation. As organizations increasingly rely on data, staying ahead of the latest trends and predictions in data operations is crucial. This article explores the future of data operations, highlighting emerging technologies, predicted trends, and the challenges that lie ahead.
Emerging Technologies Shaping Data Operations
Artificial Intelligence and Machine Learning Integration
Artificial Intelligence (AI) and Machine Learning (ML) are set to revolutionize data operations. These technologies can automate repetitive tasks such as data cleaning, integration, and analysis, significantly enhancing efficiency and reducing human error. For example, AI-driven tools can automatically detect anomalies in data, ensuring higher data quality. Furthermore, advanced analytics powered by AI can provide deeper insights, enabling businesses to anticipate market trends and make proactive decisions.
Real-Time Data Processing and Analytics
Real-time data handling is becoming crucially significant. Technologies like Apache Kafka and Apache Flink enable real-time data processing, allowing organizations to gain immediate insights. This capability is critical for industries where timely information is essential, such as finance and healthcare. Real-time analytics empower businesses to make informed decisions quickly, improving responsiveness and agility.
Blockchain for Data Integrity and Security
Blockchain technology offers significant potential for ensuring data integrity and security. By creating immutable records of transactions, blockchain can enhance data transparency and traceability. This is particularly beneficial for industries that require high levels of data security, such as supply chain management and financial services. Decentralized data management through blockchain also reduces the risk of data breaches and enhances overall data security.
Predicted Trends in Data Operations
Increased Focus on Data Governance and Privacy
With stricter data privacy regulations like GDPR and CCPA, businesses must enhance their data governance frameworks to ensure compliance. This includes implementing robust data management policies and procedures to protect sensitive information. Additionally, there will be a greater emphasis on data ethics, ensuring that data is used transparently and fairly.
Data Democratization
The rise of self-service analytics tools is empowering non-technical users to access and analyse data independently. This trend, known as data democratization, enables more employees to derive insights and contribute to data-driven decision-making. To support this, companies are investing in data literacy programs, training employees to effectively interpret and utilize data.
Enhanced Data Collaboration and Integration
Integrated data platforms that consolidate data from various sources are becoming more prevalent, facilitating seamless data collaboration across departments and teams. Cross-industry data sharing is also on the rise, leading to more comprehensive datasets and richer insights. This trend promotes a more holistic approach to data analysis and decision-making.
Challenges Ahead
Data Quality Management
As data volume and variety continue to grow, ensuring high-quality data remains a significant challenge. Businesses must invest in advanced data quality management tools and techniques to maintain the accuracy and reliability of their data. This includes implementing automated data cleansing and validation processes to detect and correct errors in real-time.
Scalability and Performance
Maintaining performance as data operations scale is critical. Organizations need to adopt scalable architectures and technologies to handle increasing data loads without compromising on speed and efficiency. Cloud-based solutions and distributed computing models are becoming essential for managing large-scale data operations.
Talent Shortage
The shortage of skilled data professionals persists as demand rises. To address this talent shortage, businesses must focus on developing talent through training and development programs. Additionally, leveraging AI and automation can help mitigate the impact of the talent gap by streamlining data operations and reducing the reliance on manual processes.
Conclusion
The future of data operations is poised for significant advancements, driven by emerging technologies and evolving business needs. As organizations navigate this dynamic landscape, staying informed about the latest trends and predictions is crucial. By embracing innovation, enhancing data governance, and fostering a data-driven culture, businesses can unlock the full potential of their data and maintain a competitive edge in the years to come.
0 notes