#Document AI Solutions
Explore tagged Tumblr posts
Text
Unlocking Potential: How Document AI is Shaping Industry Landscapes?
Many businesses are witnessing slow progress as a lot of effort is required to understand the extensive business data. Each company creates many documents across departments to make critical business decisions. This huge volume of data available in the enterprise is called unstructured data, and it isn't easy to extract the information and use it for business processes. As organizations use a variety of documents to proliferate, it is vital to understand the documents more than Optical Character Recognition (OCR). You need technology that can detect and recognize various structural elements in the business documents. Document AI turns the survivor at the juncture.
Document AI is the process of structuring unstructured data for effective business processes. It is no surprise that many industries, from financial services to health care, are unlocking the potential of Artificial Intelligence (AI) and making the most of their investments. The Document AI services leverage the power of Natural Language Processing (NLP) and Machine Learning (ML) technologies to train systems to simulate humans while reviewing documents. It is the need of the hour as it can help derive valuable information from the papers, giving vital insights for business decisions.

Real-world applications of document AI across multiple industries.
Health Care:
Medical Record Management: Document AI PCG can automate patient data extraction and organize the unstructured data for easy access to patient information
Billing and Coding: Document AI solutions assign appropriate codes for medical diagnosis and procedures for error-free billing and streamline insurance and claim processing.
Clinical Documentation: Assist the health care providers in real-time with notetaking and minimize the chances of errors in clinical documentation.
Patient Onboarding: Document AI technology assists in extracting patient data from the forms in the database and verifies them against their identity and insurance details, speeding up the reimbursement process.
Document AI intelligent services will help streamline workflow in the healthcare sector by automating repetitive tasks and reducing the time spent on manual data entry activities. It aids in lowering administrative costs by automating tasks performed by manual labor.
Financial Services:
Document AI PCG is revolutionizing the way financial institutions interact with information. By incorporating automation technology into document processing and extracting hidden insights, financial institutions can perform with the utmost efficiency.
A few of the use cases of document AI include
Loan Processing: Document AI solutions extract information from the loan application and related documents, facilitating a quick verification and approval process.
Accounts Payable and Receivables: Document Artificial intelligence will automate invoice processing and receipt management, improving the accuracy of financial transactions. All the documents are scanned to identify anomalies that indicate fraudulent activities.
Customer Onboarding: Document AI helps streamline KYC by verifying customer documents
Regulatory compliance: The technology helps in extracting data from financial documents and analysing for regulatory compliance.
Fraud detection: The artificially intelligent solutions will analyse transaction patterns to verify document authenticity and any suspicious activities in financial transactions
Document AI solutions can transform the way documents are processed in the organization. It helps in enhancing your risk assessment capabilities and fosters better decision-making. The AI solutions can be tailored to the specific financial needs of the business and can easily integrate into its financial systems.
Contract and Analysis Management :
Artificial intelligence can help legal professionals review legal contracts quickly, identify critical information and risks involved, and draft standard contracts aligned with predefined guidelines.
Legal Research: Artificial intelligence can easily extract information from huge legal databases, provide information to support legal documents, and identify applicable statutes and regulations. The lengthy legal documents can be easily drafted into concise summaries with the technology.
E-Discovery: Legal proceedings can be complex; AI works to make the process hassle-free by automating the collection of relevant documents. AI can further help in analysing large databases to identify relevant patterns. It helps in the easy finding of pertinent information by categorizing documents.
Due Diligence: Legal advisory and verification turn vital during mergers and acquisitions. AI helps legal professionals easily review documents during diligence processes. The technology helps you identify potential risks in related business deals and transactions. It also automates compliance checks.
Litigation Support: AI uses machine learning technology to predict relevant documents in litigation and organize them for trial preparation. It also helps make the legal path easy for professionals analysing and managing evidence.
By incorporating document AI services into legal services, professionals can witness enhanced efficiency and productivity in their jobs. It automates many legal operations, reducing the need for manual labour. When you use AI in legal services, you can zero in on the cost associated with human errors in document processing.
Conclusion.
Document AI PCG is transforming the way industries work by automating repetitive tasks, minimizing errors, and freeing up human resources to focus on higher-value activities. This not only enhances operational efficiency but also significantly improves the customer and employee experience.
0 notes
Text
Revolutionizing Document Management: Document AI Solutions with Piazza Consulting Group
Discover how Piazza Consulting Group is leveraging PCG's cutting-edge Document AI Solutions to transform the landscape of document management. This comprehensive guide explores the intricacies and benefits of implementing AI-driven technologies in streamlining document processing tasks. With a deep dive into the capabilities of Document AI, we will show you how it enhances accuracy, increases efficiency, and reduces operational costs. Learn about real-world applications, client success stories, and the technical underpinnings that make PCG's solutions a game-changer in various industries. Join us in understanding how these innovative technologies are not just reshaping data handling but are also setting new standards for business intelligence and compliance in the digital age. This 1000-word exploration provides insights into the future of document management, powered by artificial intelligence.
Explore the future of document management with "Revolutionizing Document Management: PCG's Document AI Solutions with Piazza Consulting Group." This detailed 1000-word article delves into how Piazza Consulting Group is harnessing the power of PCG's advanced Document AI technologies to redefine traditional document handling processes across various sectors.
In this blog, we'll unpack the sophisticated features of Document AI, such as optical character recognition (OCR), natural language processing (NLP), and machine learning algorithms that enable businesses to extract, process, and analyze data from documents with unprecedented precision and speed. Understand how these technologies are eliminating human error, automating repetitive tasks, and facilitating faster decision-making processes.
We'll showcase real-life case studies demonstrating the transformative impacts of Document AI in industries like finance, healthcare, and legal, where accuracy and efficiency are paramount. From automating data entry and enhancing security protocols to providing actionable insights and improving compliance, the applications are vast and varied.
Additionally, this blog will cover the strategic partnership between PCG and Piazza Consulting Group, highlighting how their collaborative approach has led to the development and implementation of customized solutions that cater specifically to the unique needs of their clients.
Discover the competitive advantages businesses gain by adopting these AI solutions, including cost reductions, improved customer experiences, and enhanced scalability. We'll also touch upon the ethical considerations and challenges of implementing AI in document management, ensuring a balanced view.
Join us to learn how PCG's Document AI Solutions are not just revolutionizing document management but also driving the digital transformation of enterprises worldwide, making them smarter, faster, and more connected. This is your ultimate guide to understanding the role of artificial intelligence in shaping the future of document interactions.
#Document AI Solutions#PCG Document Management#AI in Business#Piazza Consulting AI Technology#AI Document Processing#Intelligent Document Solutions#Machine Learning in Documents#AI OCR Technology#Business Automation AI#AI Compliance and Security
0 notes
Text
AI Solutions in Document AI Solutions
The AI Solutions in Document AI Solutions is the robust AI solution automating and accelerating business processes that matters the most for business growth. Connect with us for more requirements.
0 notes
Text
once again i am being subjected to "educational courses on generative AI" (lengthy advertisements that the higher ups want us to watch so they can say that we are trained in AI)
#it's a contact year we need to show that we spend a lot of tiem not only maintaining this stuff but also learning and improving the produc#we provide#they never define what they mean by AI or how the AI actually works its driving me insane#whoah this adobe ai can generate an image for you and insert it into the image you have have without learning photoshop#yeah but HOW. where are these images being pulled from? what methods are used to produce this shit#HOLY SHIT: most programmers dont actually spend that much time programming. they actually spend a lot of time in meetings. helping coworker#reading emails. reading documentation. HELLO???? YES??? THOSE ARE NORMAL THINGS TO DO???#yes attending meetings is annoying but the solution is to fucking reduce the amount of meetings and ensuring that meetings are efficient#NOT TO ADD AI????#the stupid fucking AI building half ur code isnt gonna reduce the time spent looking at documentation!!!! u can't trust the AI to be accura#to be accurate so ur gonna have to go to the documentation anyway!!!#“u can just code not worrying about syntax blah blah” so writing psuedocode??? doing a top down approach to get the big idea#and then write the little stuff later???#im so fucking livid this is SO DUMB#literally all the shit they mentioned in passing sounds actually useful instead of the generative AI bs#no i dont need a little guy to write my code for me#but a guy who checks my syntax? that suggests i look at a particular function from the library? that sounds useful!!!#“if i ask this thing how to do X it will tell me how with steps!”#Okay so will the documentation???? hello????#omfg this guy conviently skipped over the part where the AI gave a WRONG ANSWER#bro i can read the screen it did NOT accurately describe the game#“have it generate the game for you” the point of the little shit is to learn how to do stuff so you can apply it to the big shit#god im just so enraged#mr supervisor is this a good use of company resources?#you are billing t he client for ME learning ai bullshit#sir you having me sit through hours of learning the newest buzzword concepts. is this a good use of 8 hrs the client pays for me to be here#chit chat
4 notes
·
View notes
Text
im currently working with an intern who does EVERYTHING by asking chatgpt. he knows its not perfect and will tell you random bullshit sometimes. but hes allergic to looking up freely available documentation i guess.
#tütensuppe#worst is when he asks something and gets a vague/unhelpful/nonsense answer#and then he just. leaves it there.#there is literally documentation on this i can find the information within 10 seconds. argh#also this might be just me but personally i enjoy reading 10 tangentially related questions on stackoverflow#and piecing together the exact solution i need from that#he wanted to open hdf5 files in matlab. ai gave a bullshit answer that produced garbled data garbage.#he just went 'ah i guess it doesnt work then'#meanwhile one (1) search i did produced the matlab docu with the 3 lines of code needed to do that.
2 notes
·
View notes
Text
Dive In: How to extract tabular data from PDFs
Fei-Fei Li, a leading AI researcher and co-director of the Stanford Human-Centered AI Institute, once said that “to truly innovate, you must understand the essence of what you’re working with”. This insight is particularly relevant to the sophisticated task of extracting tabular data from PDF documents. We’re not just talking about pulling numbers from well-structured cells. To truly dissect this task, we need to engage with the first principles that govern PDF structuring, deciphering the language it speaks, and reconstructing that data with razor-sharp precision.
And what about those pesky footnotes that seem to follow tables around? Or merged cells that complicate the structure? Headings that stretch across multiple columns, can those be handled too? The answer is a resounding yes, yes, and yes.
Let’s dive in and explore how every aspect of a tabular structure can be meticulously managed, and how today’s AI, particularly large language models, is leading the charge in making this process smarter and more efficient.
Decoding the Components of Tabular Data
The Architectural Elements of Tabular Data
A table’s structure in a PDF document can be dissected into several fundamental components:
Multi-Level Headers: These headers span multiple rows or columns, often representing hierarchical data. Multi-level headers are critical in understanding the organization of the data, and their accurate extraction is paramount to maintaining the integrity of the information.
Vacant or Empty Headers: These elements, while seemingly trivial, serve to align and structure the table. They must be accurately identified to avoid misalignment of data during extraction.
Multi-Line Cells: Cells that span multiple lines introduce additional complexity, as they require the extraction process to correctly identify and aggregate the contents across these lines without losing context.
Stubs and Spanning Cells: Stubs (the spaces between columns) and spanning cells (which extend across multiple columns or rows) present unique challenges in terms of accurately mapping and extracting the data they contain.
Footnotes: Often associated with specific data points, footnotes can easily be misinterpreted as part of the main tabular data.
Merged Cells: These can disrupt the uniformity of tabular data, leading to misalignment and inaccuracies in the extracted output.
Understanding these elements is essential for any extraction methodology, as they dictate the task’s complexity and influence the choice of extraction technique.
Wang’s Notation for Table Interpretation
To better understand the structure of tables, let’s look at Wang’s notation, a canonical approach to interpreting tables:
(
( Header 1 , R1C1 ) ,
( Header 2 . Header 2a , R1C2 ) ,
( Header 2 . Header 2b , R1C3 ) ,
( , R1C4 ) ,
( Header 4 with a long string , R1C5 ) ,
( Header 5 , R1C6 ) ,
. . .
Fig 1. Table Elements and Terminology. Elements in the table are: a) two-level headers or multi-level header, where level I is Header 2 and level II is Header 2a and Header 2b on the same and consecutive row, b) empty header or vacant header cell, c) multi-line header spanning to three levels, d) first or base header row of the table, e) columns of a table, f) multi-line cell in a row spanning to 5 levels, g) stub or white space between columns, h) spanning cells through two columns of a row, i) empty column in a table, similarly can have an empty row, k) rows or tuples of a table
This notation provides a syntactical framework for understanding the hierarchical and positional relationships within a table, serving as the foundation for more advanced extraction techniques that must go beyond mere positional mapping to include semantic interpretation.
Evolving Methods of Table Data Extraction
Extraction methods have evolved significantly, ranging from heuristic rule-based approaches to advanced machine learning models. Each method comes with its own set of advantages and limitations, and understanding these is crucial for selecting the appropriate tool for a given task.
1. Heuristic Methods (Plug-in Libraries):
Heuristic methods are among the most traditional approaches to PDF data extraction. They rely on pre-defined rules and libraries, typically implemented in languages like Python or Java, to extract data based on positional and structural cues.
Key Characteristics:
Positional Accuracy: These methods are highly effective in documents with consistent formatting. They extract data by identifying positional relationships within the PDF, such as coordinates of text blocks, and converting these into structured outputs (e.g., XML, HTML).
Limitations: The primary drawback of heuristic methods is their rigidity. They struggle with documents that deviate from the expected format or include complex structures such as nested tables or multi-level headers. The reliance on positional data alone often leads to errors when the document’s layout changes or when elements like merged cells or footnotes are present.
Output: The extracted data typically includes not just the textual content but also the positional information. This includes coordinates and bounding boxes describing where the text is located within the document. This information is used by applications that need to reconstruct the visual appearance of the table or perform further analysis based on the text’s position.
2. UI Frameworks:
UI frameworks offer a more user-friendly approach to PDF data extraction. These commercial or open-source tools, such as Tabula, ABBYY Finereader, and Adobe Reader, provide graphical interfaces that allow users to visually select and extract table data.
Key Characteristics:
Accessibility: UI frameworks are accessible to a broader audience, including those without programming expertise. They enable users to manually adjust and fine-tune the extraction process, which can be beneficial for handling irregular or complex tables.
Limitations: Despite their ease of use, UI frameworks often lack the depth of customization and precision required for highly complex documents. The extraction is typically manual, which can be time-consuming and prone to human error, especially when dealing with large datasets.
Output: The extracted data is usually outputted in formats like CSV, Excel, or HTML, making it easy to integrate into other data processing workflows. However, the precision and completeness of the extracted data can vary depending on the user’s manual adjustments during the extraction process.
3. Machine Learning Approaches:
Machine learning (ML) approaches represent a significant advancement in the field of PDF data extraction. By leveraging models such as Deep Learning and Convolutional Neural Networks (CNNs), these approaches are capable of learning and adapting to a wide variety of document formats.
Key Characteristics:
Pattern Recognition: ML models excel at recognizing patterns in data, making them highly effective for extracting information from complex or unstructured tables. Unlike heuristic methods, which rely on predefined rules, ML models learn from the data itself, enabling them to handle variations in table structure and layout.
Contextual Awareness: One of the key advantages of ML approaches is their ability to understand context. For example, a CNN might not only identify a table’s cells but also infer the relationships between those cells, such as recognizing that a certain header spans multiple columns.
Limitations: Despite their strengths, ML models require large amounts of labeled data for training, which can be a significant investment in terms of both time and resources. Moreover, the complexity of these models can make them difficult to implement and fine-tune without specialized knowledge.
Output: The outputs from ML-based extraction can include not just the extracted text but also feature maps and vectors that describe the relationships between different parts of the table. This data can be used to reconstruct the table in a way that preserves its original structure and meaning, making it highly valuable for downstream applications.
4. In-house Developed Tools:
In-house tools are custom solutions developed to address specific challenges in PDF data extraction. These tools often combine heuristic methods with machine learning to create hybrid approaches that offer greater precision and flexibility.
Key Characteristics:
Customization: In-house tools are tailored to the specific needs of an organization, allowing for highly customized extraction processes that can handle unique document formats and structures.
Precision: By combining the strengths of heuristic and machine learning approaches, these tools can achieve a higher level of precision and accuracy than either method alone.
Limitations: The development and maintenance of in-house tools require significant expertise and resources. Moreover, the scalability of these solutions can be limited, as they are often designed for specific use cases rather than general applicability.
Output: The extracted data is typically outputted in formats that are directly usable by the organization, such as XML or JSON. The precision of the extraction, combined with the customization of the tool, ensures that the data is ready for immediate integration into the organization’s workflows.
Challenges Affecting Data Quality
Even with advanced extraction methodologies, several challenges continue to impact the quality of the extracted data.
Merged Cells: Merged cells can disrupt the uniformity of tabular data, leading to misalignment and inaccuracies in the extracted output. Proper handling of merged cells requires sophisticated parsing techniques that can accurately identify and separate the merged data into its constituent parts.
Footnotes: Footnotes, particularly those that are closely associated with tables, pose a significant challenge. They can easily be misinterpreted as part of the tabular data, leading to data corruption. Advanced contextual analysis is required to differentiate between main data and supplementary information.
Complex Headers: Multi-level headers, especially those spanning multiple columns or rows, complicate the alignment of data with the correct categories. Extracting data from such headers requires a deep understanding of the table’s structural hierarchy and the ability to accurately map each data point to its corresponding header.
Empty Columns and Rows: Empty columns or rows can lead to the loss of data or incorrect merging of adjacent columns. Identifying and managing these elements is crucial for maintaining the integrity of the extracted information.
Selecting the Optimal Extraction Method
Selecting the appropriate method for extracting tabular data from PDFs is not a one-size-fits-all decision. It requires a careful evaluation of the document’s complexity, the quality of the data required, and the available resources.
For straightforward tasks involving well-structured documents, heuristic methods or UI frameworks may be sufficient. These methods are quick to implement and provide reliable results for documents that conform to expected formats.
However, for more complex documents, particularly those with irregular structures or embedded metadata, machine learning approaches are often the preferred choice. These methods offer the flexibility and adaptability needed to handle a wide range of document formats and data types. Moreover, they can improve over time, learning from the data they process to enhance their accuracy and reliability.
The Role of Multi-Modal Approaches: In some cases, a multi-modal approach that combines text, images, and even audio or video data, may be necessary to fully capture the richness of the data. Multi-modal models are particularly effective in situations where context from multiple sources is required to accurately interpret the information. By integrating different types of data, these models can provide a more holistic view of the document, enabling more precise and meaningful extraction.MethodKey CharacteristicsCost & SubscriptionTemplating & CustomizationLearning CurveCompatibility & ScalabilityHeuristic Methods– Rule-based, effective for well-structured documents
– Extracts positional information (coordinates, etc.)– Generally low-cost
– Often open-source or low-cost libraries– Relies on predefined templates
– Limited flexibility for complex documents– Moderate
– Requires basic programming knowledge– Compatible with standard formats
– May struggle with complex layouts
– Scalability depends on document uniformityUI Frameworks– User-friendly interfaces
– Manual adjustments possible– Subscription- based
– Costs can accumulate over time– Limited customization
– Suitable for basic extraction tasks– Low to Moderate
– Easy to learn but may require manual tweaking– Generally compatible
– Limited scalability for large-scale operationsMachine Learning– Adapts to diverse document formats
– Recognizes patterns and contextual relationships– High initial setup cost
– Requires computational resources
– Possible subscription fees for advanced platforms– Flexible, can handle unstructured documents
– Custom models can be developed– High
– Requires expertise in ML and data science– High compatibility
– Integration challenges possible
– Scalable with proper infrastructureIn-house Developed Tools– Custom-built for specific needs
– Combines heuristic and ML approaches– High development cost
– Ongoing maintenance expenses– Highly customizable
– Tailored to organization’s specific document types– High
– Requires in-depth knowledge of both the tool and the documents– High compatibility
– Scalability may be limited and require further developmentMulti-Modal & LLMs– Processes diverse data types (text, images, tables)
– Context-aware and flexible– High cost for computational resources
– Licensing fees for advanced models– Flexible and adaptable
– Can perform schemaless and borderless data extraction– High
– Requires NLP and ML expertise– High compatibility
– Scalability requires significant infrastructure and integration effort
Large Language Models Taking the Reins
Large Language Models (LLMs) are rapidly becoming the cornerstone of advanced data extraction techniques. Built on deep learning architectures, these models offer a level of contextual understanding and semantic parsing that traditional methods cannot match. Their capabilities are further enhanced by their ability to operate in multi-modal environments and support data annotation, addressing many of the challenges that have long plagued the field of PDF data extraction.
Contextual Understanding and Semantic Parsing
LLMs are designed to acknowledge the broader context in which data appears, allowing them to extract information accurately, even from complex and irregular tables. Unlike traditional extraction methods that often struggle with ambiguity or non-standard layouts, LLMs parse the semantic relationships between different elements of a document. This nuanced understanding enables LLMs to reconstruct data in a way that preserves its original meaning and structure, making them particularly effective for documents with complex tabular formats, multi-level headers, and intricate footnotes.
Example Use Case: In a financial report with nested tables and cross-referenced data, an LLM can understand the contextual relevance of each data point, ensuring that the extracted data maintains its relational integrity when transferred to a structured database.
Borderless and Schemaless Interpretation
One of the most significant advantages of LLMs is their ability to perform borderless and schemaless interpretation. Traditional methods often rely on predefined schemas or templates, which can be limiting when dealing with documents that deviate from standard formats. LLMs, however, can interpret data without being confined to rigid schemas, making them highly adaptable to unconventional layouts where the relationships between data points are not immediately obvious.
This capability is especially valuable for extracting information from documents with complex or non-standardized structures. Such as legal contracts, research papers, or technical manuals, where data may be spread across multiple tables, sections, or even embedded within paragraphs of text.
Multi-Modal Approaches: Expanding the Horizon
The future of data extraction lies in the integration of multi-modal approaches, where LLMs are leveraged alongside other data types such as images, charts, and even audio or video content. Multi-modal LLMs can process and interpret different types of data in a unified manner, providing a more holistic understanding of the document’s content.
Example Use Case: Consider a scientific paper where experimental data is presented in tables, supplemented by images of the experimental setup, and discussed in the text. A multi-modal LLM can extract the data, interpret the images, and link this information to the relevant sections of text, providing a complete and accurate representation of the research findings.
Enhancing Data Annotation with LLMs
Data annotation, a critical step in training machine learning models, has traditionally been a labor-intensive process requiring human oversight. However, LLMs are now playing a significant role in automating and enhancing this process. By understanding the context and relationships within data, LLMs can generate high-quality annotations that are both accurate and consistent, reducing the need for manual intervention.
Key Benefits:
Automated Labeling: LLMs can automatically label data points based on context, significantly speeding up the annotation process while maintaining a high level of accuracy.
Consistency and Accuracy: The ability of LLMs to understand context ensures that annotations are consistent across large datasets, reducing errors that can arise from manual annotation processes.
Example Use Case: In an e-discovery process, where large volumes of legal documents need to be annotated for relevance, LLMs can automatically identify and label key sections of text, such as contract clauses, parties involved, and legal references, thereby streamlining the review process.
Navigating the Complexities of LLM-Based Approaches
While Large Language Models (LLMs) offer unprecedented capabilities in PDF data extraction, they also introduce new complexities that require careful management. Understanding the core of these challenges will help implement robust and trusted strategies.
Hallucinations: The Mirage of Accuracy
Hallucinations in LLMs refer to the generation of plausible but factually incorrect information. In the context of tabular data extraction from PDFs, this means:
Data Fabrication: LLMs may invent data points when encountering incomplete tables or ambiguous content.
Relational Misinterpretation: Complex table structures can lead LLMs to infer non-existent relationships between data points.
Unwarranted Contextualization: LLMs might generate explanatory text or footnotes not present in the original document.
Cross-Document Contamination: When processing multiple documents, LLMs may mistakenly mix information from different sources.
Time-Related Inconsistencies: LLMs can struggle with accurately representing data from different time periods within a single table.
Context Length Limitations: The Truncation Dilemma
LLMs have a finite capacity for processing input, known as the context length. How this affects tabular data extraction from PDFs:
Incomplete Processing: Large tables or documents exceeding the context length may be truncated, leading to partial data extraction.
Loss of Contextual Information: Critical context from earlier parts of a document may be lost when processing later sections.
Reduced Accuracy in Long Documents: As the model approaches its context limit, the quality of extraction can degrade.
Difficulty with Cross-Referencing: Tables that reference information outside the current context window may be misinterpreted.
Challenges in Document Segmentation: Dividing large documents into processable chunks without losing table integrity can be complex.
Precision Control: Balancing Flexibility and Structure
LLMs’ flexibility in interpretation can lead to inconsistencies in output structure and format, challenging the balance between adaptability and standardization in data extraction.
Inconsistent Formatting: LLMs may produce varying output formats across different runs.
Extraneous Information: Models might include unrequested information in the extraction.
Ambiguity Handling: LLMs can struggle with making definitive choices in ambiguous scenarios.
Structural Preservation: Maintaining the original table structure while allowing for flexibility can be challenging.
Output Standardization: Ensuring consistent, structured outputs across diverse table types is complex.
Rendering Challenges: Bridging Visual and Textual Elements
LLMs may struggle to accurately interpret the visual layout of PDFs, potentially misaligning text or misinterpreting non-textual elements crucial for complete tabular data extraction.
Visual-Textual Misalignment: LLMs may incorrectly associate text with its position on the page.
Non-Textual Element Interpretation: Charts, graphs, and images can be misinterpreted or ignored.
Font and Formatting Issues: Unusual fonts or complex formatting may lead to incorrect text recognition.
Layout Preservation: Maintaining the original layout while extracting data can be difficult.
Multi-Column Confusion: LLMs may misinterpret data in multi-column layouts.
Data Privacy: Ensuring Trust and Compliance
The use of LLMs for data extraction raises concerns about data privacy, confidentiality, and regulatory compliance, particularly when processing sensitive or regulated information.
Sensitive Information Exposure: Confidential data might be transmitted to external servers for processing.
Regulatory Compliance: Certain industries have strict data handling requirements that cloud-based LLMs might violate.
Model Retention Concerns: There’s a risk that sensitive information could be incorporated into the model’s knowledge base.
Data Residency Issues: Processing data across geographical boundaries may violate data sovereignty laws.
Audit Trail Challenges: Maintaining a compliant audit trail of data processing can be complex with LLMs.
Computational Demands: Balancing Power and Efficiency
LLMs often require significant computational resources, posing challenges in scalability, real-time processing, and cost-effectiveness for large-scale tabular data extraction tasks.
Scalability Challenges: Handling large volumes of documents efficiently can be resource-intensive.
Real-Time Processing Limitations: The computational demands may hinder real-time or near-real-time extraction capabilities.
Cost Implications: The hardware and energy requirements can lead to significant operational costs.
Model Transparency: Unveiling the Black Box
The opaque nature of LLMs’ decision-making processes complicates efforts to explain, audit, and validate the accuracy and reliability of extracted tabular data.
Decision Explanation Difficulty: It’s often challenging to explain how LLMs arrive at specific extraction decisions.
Bias Detection: Identifying and mitigating biases in the extraction process can be complex.
Regulatory Compliance: Lack of transparency can pose challenges in regulated industries requiring explainable AI.
Trust Issues: The “black box” nature of LLMs can erode trust in the extraction results.
Versioning and Reproducibility: Ensuring Consistency
As LLMs evolve, maintaining consistent extraction results over time and across different model versions becomes a significant challenge, impacting long-term data analysis and comparability.
Model Evolution Impact: As LLMs are updated, maintaining consistent extraction results over time can be challenging.
Reproducibility Concerns: Achieving the same results across different model versions or runs may be difficult.
Backwards Compatibility: Ensuring newer model versions can accurately process historical data formats doesn’t always stand true.
It’s becoming increasingly evident that harnessing the power of AI for tabular data extraction requires a nuanced and strategic approach. So the question naturally arises: How can we leverage AI’s capabilities in a controlled and conscious manner, maximizing its benefits while mitigating its risks?
The answer lies in adopting a comprehensive, multifaceted strategy that addresses these challenges head-on.
Optimizing Tabular Data Extraction with AI: A Holistic Approach
Effective tabular data extraction from PDFs demands a holistic approach that channels AI’s strengths while systematically addressing its limitations. This strategy integrates multiple elements to create a robust, efficient, and reliable extraction process:
Hybrid Model Integration: Combine rule-based systems with AI models to create robust extraction pipelines that benefit from both deterministic accuracy and AI flexibility.
Continuous Learning Ecosystems: Implement feedback loops and incremental learning processes to refine extraction accuracy over time, adapting to new document types and edge cases.
Industry-Specific Customization: Recognize and address the unique requirements of different sectors, from financial services to healthcare, ensuring compliance and accuracy.
Scalable Architecture Design: Develop modular, cloud-native architectures that can efficiently handle varying workloads and seamlessly integrate emerging technologies.
Rigorous Quality Assurance: Establish comprehensive QA protocols, including automated testing suites and confidence scoring mechanisms, to maintain high data integrity.
Even though there are complexities of AI-driven tabular data extraction, adopting AI is the key to unlocking new levels of efficiency and insight. The journey doesn’t end here. As the field of AI and data extraction continues to evolve rapidly, staying at the forefront requires continuous learning, expertise, and innovation.
Addressing Traditional Challenges with LLMs
Custom LLMs trained on specific data and needs in tag team with multi-modal approaches are uniquely positioned to address several of the traditional challenges identified in PDF data extraction:
Merged Cells: LLMs can interpret the relationships between merged cells and accurately separate the data, preserving the integrity of the table.
Footnotes: By understanding the contextual relevance of footnotes, LLMs can correctly associate them with the appropriate data points in the table, ensuring that supplementary information is not misclassified.
Complex Headers: LLMs’ ability to parse multi-level headers and align them with the corresponding data ensures that even the most complex tables are accurately extracted and reconstructed.
Empty Columns and Rows: LLMs can identify and manage empty columns or rows, ensuring that they do not lead to data misalignment or loss, thus maintaining the integrity of the extracted data.
Conclusion
The extraction of tabular data from PDFs is a complex task that requires a deep understanding of both document structure and extraction methodologies. Our exploration has revealed a diverse array of tools and techniques, each with its own strengths and limitations. The integration of Large Language Models and multi-modal approaches promises to revolutionize this field, potentially enhancing accuracy, flexibility, and contextual understanding. However, our analysis has highlighted significant challenges, particularly hallucinations and context limitations, which demand deeper expertise and robust mitigation strategies.
Forage AI addresses these challenges through a rigorous, research-driven approach. Our team actively pursues R&D initiatives, continuously refining our models and techniques to balance cutting-edge AI capabilities with the precision demanded by real-world applications. For instance, our proprietary algorithms for handling merged cells and complex headers have significantly improved extraction accuracy in financial documents.
By combining domain expertise with advanced AI capabilities, we deliver solutions that meet the highest standards of accuracy and contextual understanding across various sectors. Our adaptive learning systems enable us to rapidly respond to emerging challenges, translating complex AI advancements into efficient, practical solutions. This approach has proven particularly effective in highly regulated industries where data privacy and compliance are paramount.
Our unwavering dedication to excellence empowers our clients to unlock the full potential of their critical data embedded in PDF documents – that’s often inaccessible. We transform raw information into actionable insights, driving informed decision-making and operational efficiency.
Experience the difference that Forage AI can make in your data extraction processes. Contact us today to learn how our tailored solutions can address your specific industry needs and challenges, and take the first step towards revolutionizing your approach to tabular data extraction.
#intelligent document processing#idp solutions#IDP#artificial intelligence#AI Document Processing#pdf table extraction#document extraction
0 notes
Text
Is the Future of Localization Up for Grabs? From the beaches of Hawaii to the boardrooms of the global language industry, Andrew Smart has seen it all. In my latest blog post, we dive into his candid reflections on why he’s selling his shares in Slator and how his latest venture, AcudocX, is quietly transforming the certified document translation space. Whether you’re a language industry veteran, a rising entrepreneur, or just curious about where the next big opportunity lies, this is a must-read! 📚 Read the full blog here: https://www.robinayoub.blog 🎙 Watch the full interview on YouTube: https://youtu.be/rE2IdDLr1pc 📹 Prefer bite-sized insights? Watch the 12 YouTube Shorts here: https://www.youtube.com/@L10NFiresideChat/shorts #Localization #Translation #LanguageServices #Slator #AcudocX #Entrepreneurship #LanguageIndustry #AIinTranslation #L10NFiresideChat
#AcudocX#AI in Translation#Andrew Smart#B2B Localization#B2C Translation Solutions#Certified Document Translation#Document Translation Platforms#entrepreneurship#Freelance Translators#Global Language Market#Investor Opportunities#Language Industry News#Language Service Providers#language services#Localization Business Strategy#localization industry#Localization Innovation#Localization Trends#Machine Translation#Media and M&A#Slator#Slator Shares for Sale#Translation Automation#Translation Startups#Translation Technology
0 notes
Text
Trusted Document Verification software| Zionitai
Zioshield is an advanced online document verification software that ensures the authenticity of identity documents like Aadhar, PAN, and passports.
online document verification Software
document verification company in india
Document Security Solutions
Identity Verfication AI
document verification service
id document verification
#online document verification Software#document verification company in india#Document Security Solutions#Identity Verfication AI#document verification service#id document verification
0 notes
Text
Why is writing taking up so much time?!
Why can't I just mindlessly smash my keyboard for a while and all my ideas are on paper? Why do I have to formulate sentences and structure things and look up words? Why do I have to think about how to put what I have in my mind into writing?
#why can't writing be like hacking in movies?#no. ai is not the solution#ai only produces a mess#i want to upload my thoughts into a document#not make a crappy algorithm spit out what it thinks i have in mind#writing#it's a struggle
1 note
·
View note
Text

At Argos Labs, we're committed to helping organizations like yours unlock the full potential of Intelligent Document Processing (IDP). However, we've noticed that several misconceptions about IDP are holding businesses back from realizing its benefits. 𝐌𝐢𝐬𝐜𝐨𝐧𝐜𝐞𝐩𝐭𝐢𝐨𝐧𝐬 𝐥𝐢𝐤𝐞 ❌ Myth #1: Only simple tasks can be automated with IDP. ❌ Myth #2: IDP solutions are only suitable for large organizations. ❌ Myth #3: Traditional automation & AI-powered automation offer equal value. ❌ Myth #4: IDP replaces human workers. ❌ Myth #5: Implementing IDP solutions is a complex process. ❌ Myth #6: AI-powered IDP is a fleeting trend.
0 notes
Text
AI and Document Insights: Simplifying Complex Research problems with Photon Insights
As research is an inexact science, keeping track of vast amounts of data can be daunting. Complicated projects often include reviewing multiple documents, extracting relevant insights from them, synthesizing findings from various sources and synthesizing these into one cohesive research report. Unfortunately, this process can be time consuming and subject to human error, making accuracy and efficiency an ongoing struggle for researchers. Thanks to Artificial Intelligence (AI), platforms like Photon Insights are revolutionizing how researchers handle document insights; streamlining complex projects more efficiently while increasing productivity — this article explores how AI improves document insights while Photon Insights helps researchers navigate projects more successfully than ever before!
Researching Document Insights to Gain New Insights
Documenting insights is vital for researchers across disciplines for multiple reasons, including:
1. Information Overload: Researchers often face an overwhelming amount of information from academic articles, reports, and studies that needs to be processed efficiently to obtain valuable insights for meaningful analysis. Extracting key insights efficiently is paramount.
2. Improved Understanding: Accurate insights help researchers grasp complex topics, identify trends and understand the repercussions of their findings.
3. Evidence-Based Decision Making: Documented insights enable researchers to support their conclusions with solid evidence, which is key for maintaining credibility within academic and corporate environments.
4. Streamlined Collaboration: When conducting multidisciplinary research projects, sharing insights among team members is paramount for cohesive progress and informed decision-making.
Challenges Involve Traditional Document Analysis
Traditional methods for document analysis present several hurdles.
1. Time-Consuming Processes: Reviewing and extracting information from numerous documents manually can take considerable time, limiting research progress.
2. Risk of Human Error: Manual analysis can lead to inaccuracies due to human interpretation, leading to discrepancies and discrepancies within data.
3. Difficulties with Handling Unstructured Data: Research data often contains unstructured content that makes analysis and derivation of insights difficult without using specialist software tools.
4. Limited Collaboration: Sharing insights between team members can be cumbersome when using static documents and manual processes as means for sharing insight.
How AI Is Transforming Document Insights
Document analysis with artificial intelligence (AI) offers several significant advantages for researchers looking to simplify complex projects:
Automated Data Extraction Processes (ADEPs)
AI algorithms can automatically extract relevant data from documents, significantly shortening manual analysis time and freeing researchers up to focus on interpreting their findings rather than collecting information.
Keyword Focus: Automated Data Extraction and Time Efficiency
Photon Insights employs advanced data extraction techniques that enable researchers to quickly gather insights from various documents, streamlining their workflow.
2. Natural Language Processing (NLP)
Natural Language Processing (NLP) allows AI to understand human language, providing insights from unstructured sources like articles and reports. NLP identifies key themes, concepts, and sentiments that make complex texts easier for researchers to grasp the main points.
Keyword Focus: Natural Language Processing and Text Analysis
Researchers can leverage Photon Insights’ NLP capabilities to extract meaningful insights from large volumes of documents, deepening their understanding of complex subjects.
Enhance Search Capabilities
AI-powered search functions allow researchers to query documents using natural language, and return results that are contextual rather than simply keyword matching. This feature improves accuracy and efficiency of research processes.
Keyword Focus: Improve Search, Contextual Queries
Photon Insights provides advanced search functionalities that enable users to quickly locate the information they require, creating smoother research workflows.
Intelligent Summarization (ISS)
AI can produce concise summaries of lengthy documents, outlining only the key information. This allows researchers to quickly assess which documents warrant further study and make informed decisions.
Keyword Focus: Intelligent Summarization, Rapid Insights
Photon Insights provides intelligent summarization tools to enable researchers to gain quick and immediate insights from large amounts of text, saving both time and effort in the process.
5. Collaborative Features
AI-driven platforms can enhance collaboration by allowing team members to easily share insights, comments, and annotations in real time — an indispensable feature that ensures all team members stay informed throughout the research process.
Keyword Focus: Collaborative Features, Real-Time Sharing
Photon Insights encourages collaboration among researchers by enabling them to engage with each other’s findings and insights seamlessly — thus creating a more productive research environment.
Photon Insights Advantage
Photon Insights stands out as an invaluable tool for researchers seeking to leverage AI for document insights. Here’s how it enhances research experiences:
1. Comprehensive Document Management system.
Photon Insights allows users to efficiently organize and manage their documents, providing easy access to relevant materials — an essential step in maintaining an efficient research workflow.
2. User-Friendly Interface
The platform’s intuitive user interface makes navigating documents and extracting insights much simpler, making it ideal for researchers of all skill levels.
3. Customizable Dashboards
Researchers can create customized dashboards that represent their specific research interests and priorities, providing for more focused data analysis and insights.
Integration of Other Tools
Photon Insights provides users with seamless integration between various research tools and databases, enabling them to streamline their workflows and maximize research capabilities.
5. Continuous Development and Learning
Photon Insights’ AI algorithms learn from user interactions, continually honing in on relevance for each researcher to ensure they get the most relevant and up-to-date results possible. This ensures they receive relevant and valuable data.
Case Studies of Success With Photon Insights
Consider these case studies as examples of AI’s effectiveness in document insights:
Case Study 1: Academic Research
Academic researchers investigating climate change made use of Photon Insights to rapidly review hundreds of scientific articles. With its automated data extraction and intelligent summarization features, this team was able to synthesize critical findings more quickly for publication as an extensive review paper with wide appeal.
Case Study 2: Corporate Analysis
Photon Insights helped a corporate research department streamline their market analysis process. Utilizing its NLP capabilities, the team were able to extract sentiment data from industry reports and news articles, providing real-time market intelligence insights for informed strategic decisions.
Case Study 3 — Healthcare Research
Photon Insights was used by a healthcare research group to analyze patient data and clinical studies. With its automated extraction of relevant insights, the team were able to quickly identify trends in treatment outcomes which ultimately resulted in improved care strategies and protocols.
Future Photon Insights and Document Insights
As AI technology develops further, its role in document insights may grow increasingly significant. A number of trends may determine its development:
1. Greater Automation & Designing : Automating document analysis will further increase efficiency, enabling researchers to focus on interpretation and application instead.
2. Advancement in AI Capabilities: Advancements in artificial intelligence algorithms will increase both accuracy and depth of insights drawn from complex documents.
3. Emerging Technologies: When combined, AI and emerging technologies such as blockchain and augmented reality could create new avenues for document insights and analysis.
4. Emphasis on Ethical AI: As AI becomes more integrated into research, attention to ethical considerations will become ever more essential to ensure fairness, transparency, and accountability.
AI is revolutionizing how researchers manage document insights, streamlining complex projects and improving overall efficiency. From automating data extraction and natural language processing to intelligent summarization capabilities, AI enables researchers to navigate large volumes of information with ease.
Photon Insights stands at the forefront of this transformation, offering an AI-powered suite of tools designed to optimize document analysis and foster collaboration. As research requirements increase, adopting solutions like Photon Insights will become essential in meeting those demands while increasing productivity and gaining insights. With so much data out there already available online, AI solutions such as Photon Insights offer key differentiators that will lead to success both academically and corporately alike.
0 notes
Text
Discover AiMunshi, an AI-powered data extraction tool designed to automate and streamline document processing. Enhance efficiency, reduce manual work, and unlock valuable insights with advanced machine learning technology for your business. . For more: https://aimunshi.ai/
#data extraction#data extraction tool#document management#business solutions#ai data extraction tool#document automation
0 notes
Text
Documents Management in ALZERP Cloud ERP Software
In today’s fast-paced business environment, managing and organizing documents effectively is crucial for operational efficiency. ALZERP Cloud ERP Software offers a robust Documents Library or File Storage feature, designed to streamline document management and ensure your business remains agile, compliant, and efficient. This article delves into the comprehensive capabilities of the Documents…
#Affordable Letter Printing Solutions#AI-powered Document Management#Audit Trails#AuditTrail#Automated Letter Generation with Merge Fields#Automated Letter Printing ERP#Best Cloud Document Management Systems#Best Letter Printing System for ERP#Business Letter Automation#Centralized Document Storage ERP#Cloud Document Storage#Cloud ERP Compliance Document Management#Cloud ERP Document Management#Cloud-Based Document Audit Trail#Cloud-Based Document Collaboration#Cloud-Based Document Management#Cloud-based File Management ERP#Cloud-based Letter Printing for ERP#CloudDocumentManagement#Compliance Management#Custom Letter Printing ERP#Customizable Letter Templates in ERP#Digital Document Management#Digital Document Management ERP#Document Generation ERP#Document Lifecycle Management Cloud#Document Management System (DMS)#Document Management System for Finance#Document Management System for Healthcare#Document Process Automation Cloud
0 notes
Text
Best Document Processing Solution
The AI gold rush is on. Many are leading the charge, chief among them OpenAI, Anthropic, Google, Mistral, and DeepSeek. While numerous players race to scale operations and address infrastructure demands with multi-million-dollar investments, companies like DeepSeek are making waves by achieving breakthroughs in cost-efficient AI model deployment—minimizing costs without compromising innovation.
As AI models grow more competent and specialized, businesses are eager for solutions that can tackle the elephant in the room: how can we seamlessly integrate these rapidly evolving models into existing systems? And where do we even begin?
In the document intelligence space, success hinges on model performance, stability, and LLM-agnostic solutions. AI-driven Intelligent Document Processing (IDP) solutions now leverage the full ensemble of Generative AI. This includes Large Language Models (LLMs), Retrieval-Augmented Generation (RAG), Computer Vision, Visual Language Models (VLMs), and Agentic AI frameworks. These technologies work together to extract, analyze, and structure data with remarkable accuracy.
If you would like to learn more about end-to-end intelligent document processing (IDP) solutions for your business, reach out to us to understand the full capacity of our services.
In this, we’ll explore how you can stay ahead of the curve, leverage strategic advantages, and transform your business metrics—starting now.
The Need for Next-Gen Intelligent Document Processing (IDP)
The exponential growth of data across industries has led to inefficiencies in traditional document processing. Major challenges businesses face:
High-volume document processing bottlenecks: Traditional and legacy systems are unable to keep up with the influx of data.
Inconsistent data extraction accuracy: Traditional OCR and rule-based systems struggle with complex layouts, visual data interpretation, and diverse document formats.
Compliance and security risks: Regulatory requirements demand precision in data handling, making automation a necessity rather than an option.
Operational inefficiencies and rising costs: Enterprises need a cost-effective solution that eliminates human intervention while improving data accuracy and speed.
The need for a scalable, AI-powered, and fully automated Intelligent Document Processing solution is now inevitable.
Key Trends Driving Intelligent Document Processing (IDP) in 2025
1. Large Language Models (LLMs) for Contextual Understanding
Integrating LLMs into document processing solutions allows for a deeper contextual understanding of documents, improving data extraction from complex document structures like legal contracts, financial statements, and regulatory filings. Advanced LLMs enable sophisticated text summarization, question-answering, and content classification with human-like comprehension.
2. Visual Language Models (VLMs) for Enhanced Document Parsing
Traditional OCR methods struggle with complex document layouts, but VLMs bridge the gap by integrating image recognition with textual comprehension. These models understand the structure of invoices, receipts, forms, and technical diagrams, ensuring higher precision in data extraction.
3. AI Agents for Autonomous Document Processing
Autonomous AI Agents take IDP beyond mere extraction. These agents can:
Continuously refine document parsing models based on real-time feedback.
Automate decision-making by classifying and routing documents dynamically.
Detect anomalies and discrepancies in extracted data for compliance and auditing.
Reiterate through errors, logs, and self-generated inputs until the desired results are achieved.
4. Multi-Modal AI Processing for Diverse Document Types
IDP solutions now process multiple data formats, including text, images, tables, and multimedia elements. Multi-modal AI models combine textual, visual, and contextual cues to extract meaningful insights from complex and varied document sources.
5. Human-in-the-Loop (HITL) for Continuous Improvement
To maximize accuracy, Human-in-the-Loop (HITL) models refine AI outputs. This ensures:
Reinforcement learning from human feedback (RLHF).
Continuous model updates to address new document structures.
Increased confidence in high-stakes data processing environments.
6. RAG-Based Document Retrieval for Context-Aware Processing
By incorporating Retrieval-Augmented Generation (RAG), IDP systems can reference external and internal data sources to enhance extraction accuracy. This enables:
Intelligent cross-referencing of extracted data.
Enriched insights through supplementary knowledge bases.
Improved contextualization in decision-support workflows.
7. Intelligent Data Governance and Security
With regulatory compliance being a significant concern, IDP solutions now include:
On-premise and private cloud deployments for secure data handling.
AI-driven anomaly detection to prevent fraud and compliance risks.
Automated audit trails for full transparency and traceability.
Making the right decision
Choosing the right Intelligent Document Processing solution can be overwhelming. With so many options on the market, businesses must consider factors like accuracy, scalability, privacy & security, integration capabilities, and long-term reliability. Companies must find a solution that not only automates document extraction but also enhances operational efficiency and decision-making, providing 10x the ROI.
With these trends reshaping the IDP landscape, enterprises need a solution that not only meets today’s demands but is built for the future. This is where Forage AI excels. Unlike traditional IDP solutions that require rigid configurations, Forage AI dynamically adapts, ensuring future-proof automation.
Among the myriad of IDP solutions, Forage AI stands out as the most comprehensive, scalable, and intelligent document processing solution of 2025. Built with state-of-the-art AI and extensive domain expertise, Forage AI transforms document automation with unmatched precision and efficiency.
Comparing the AI-Powered Document Extraction Capabilities
FeatureTraditional OCRRPA-Based IDPAI-Powered IDP (2025)Accuracy~80%~90%99%+ with AI & HITLScalabilityLimitedMediumHigh (Handles millions of docs daily)Complex Data HandlingNoLimitedYes (Multimodal AI, VLMs)Real-Time AdaptationNoNoYes (Agentic AI & RAG)Integration FlexibilityLowMediumHigh (LLM-Agnostic)
Why Forage AI is the Best Document Processing Solution
Forage AI’s AI-powered document processing sets the benchmark for enterprise-grade IDP with cutting-edge automation, accuracy, and scalability.
AI & ML-driven Parsing – Multi-layer AI models handle complex layouts, handwritten text, and tables with 99% accuracy using NLP, ML, and Reinforcement Learning.
RAG & Agentic AI for Context-Aware Extraction – Combines Retrieval-Augmented Generation (RAG) with Agentic AI for real-time, context-aware document processing.
Seamless Data Integration – API-first design, RPA-enabled document fetching, and real-time anomaly detection for smooth enterprise workflow integration.
LLM-Agnostic & Customizable Workflows – Works with any enterprise AI framework, offering industry-specific, adaptable document processing.
Unmatched Scalability & Speed – Processes millions of documents monthly with self-learning models that enhance accuracy while reducing manual intervention.
Enterprise-Grade Security & Compliance – End-to-end encryption, full data ownership, and adherence to HIPAA and GDPR standards.
Best-in-Class QA & Human-in-the-Loop Validation – Multi-layer AI-powered validation with expert human review ensures near-perfect accuracy.
Why Enterprises Choose Forage AI Over Competitors
As organizations look for the best Intelligent Document Processing solution in 2025, Forage AI stands out with its strategic automation, superior accuracy, and innovative scalability.
Forage AI vs Traditional OCR: OCR tools struggle with complex layouts and require extensive rule-based adjustments. Forage AI’s ML models continuously improve extraction accuracy without manual configuration.
Forage AI vs Generic IDP Platforms: Many IDP platforms offer generic solutions with limited customization. Forage AI provides tailor-fit automation with custom data pipelines, document-specific AI models, and flexible deployment options.
Forage AI vs In-House Data Teams: Internal data teams often lack the tools and scalability required for real-time document processing. Forage AI takes full ownership of the data pipeline, delivering speed, accuracy, and compliance without the overhead costs.
Forage AI Document Processing Features Checklist
✅ LLM-Powered Contextual Extraction
✅ VLM-Based Image & Text Processing
✅ AI-Powered Document Classification
✅ 99%+ Data Accuracy
✅ Multi-Layer QA (AI + Human)
✅ On-Prem & Cloud Deployments
✅ RAG-Driven Knowledge Integration
The Future of Intelligent Document Processing
The evolution of IDP is far from over. IDP solutions will become even more adaptable and intuitive with the increasing adoption of Autonomous AI Agents, GenAI-powered search, and contextual AI workflows.
Forage AI is at the forefront of this revolution, combining cutting-edge machine learning, generative AI, and deep domain expertise to offer the most advanced, scalable, and customizable IDP solution on the market.
Ready to Future-Proof Your Document Automation?
Explore Forage AI’s industry-leading document extraction technology today. Talk to us to see how we can transform your document workflows.
#artificial intelligence#Document Processing#IDP#accurate table extraction#idp solutions#ai based document processing#document processing companies
0 notes
Text
MT Post-Editing | Machine Translation Post Editing
MT Post-Editing: An Essential Process for Flawless Communication Effective communication is more crucial than ever. Businesses operate across borders, and cultures blend effortlessly. Yet, language barriers can still create significant challenges. Ensuring clear and accurate communication can make or break international relationships. Therefore, many organizations turn to Machine Translation (MT)…
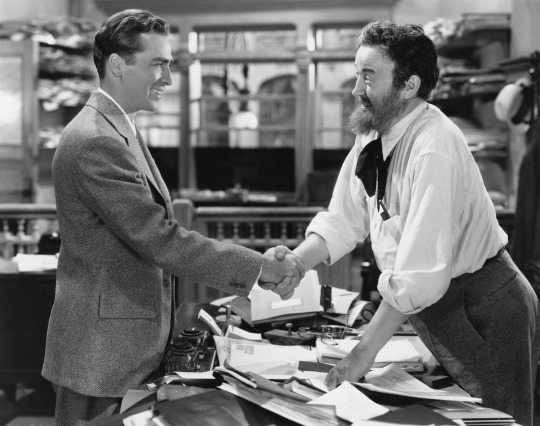
View On WordPress
#AI translation post-editing#cost-effective MT post-editing#fast MT post-editing#fast transcription companies#fast translation companies#high-quality MT post-editing#interpretation services#interpreting companies#linguistic services#machine translation post-editing#minute taking companies#Minute Taking Services#MT post-editing experts#MT post-editing for businesses#MT post-editing for legal documents#MT post-editing for marketing content#MT post-editing for medical texts#MT post-editing for technical documents#MT post-editing services#MT post-editing solutions#MTPE services#note taking companies#note taking services#post-editing machine translations#professional MT post-editing#subtitling companies#subtitling services#transcription companies#transcription service#transcription services
0 notes
Text
Trusted Document Verification software| Zionitai
Zioshield is an advanced online document verification software that ensures the authenticity of identity documents like Aadhar, PAN, and passports.
online document verification Software
document verification company in india
Document Security Solutions
Identity Verfication AI
document verification service
id document verification
#online document verification Software#document verification company in india#Document Security Solutions#Identity Verfication AI#document verification service#id document verification
0 notes