#Multimodal AI
Explore tagged Tumblr posts
Text
How Google Generative AI and Multimodal AI Are Transforming Industries?
Google Generative AI creates content like text, images, and music using advanced models like GANs and transformers. Meanwhile, Multimodal AI integrates multiple data types—text, images, and audio—for better decision-making in healthcare, self-driving vehicles, and virtual assistants. Together, they enhance creativity and analytics, revolutionizing industries like marketing, education, and entertainment. Stay ahead in the AI revolution—explore the latest trends and insights on RobotsIntelli today! 🚀For more info. visit: https://robotsintelli.com/what-is-the-difference-between-generative-ai-and-multimodal-ai/

2 notes
·
View notes
Text
Global Multimodal AI Market Forecast: Growth Trends and Projections (2024–2034)
Global Multimodal AI Market: Growth, Trends, and Forecasts for 2024-2034
The Global Multimodal AI Market is witnessing explosive growth, driven by advancements in artificial intelligence (AI) technologies and the increasing demand for systems capable of processing and interpreting diverse data types.
The Multimodal AI market is projected to grow at a compound annual growth rate (CAGR) of 35.8% from 2024 to 2034, reaching an estimated value of USD 8,976.43 million by 2034. In 2024, the market size is expected to be USD 1,442.69 million, signaling a promising future for this cutting-edge technology. In this blog, we will explore the key components, data modalities, industry applications, and regional trends that are shaping the growth of the Multimodal AI market.
Request Sample PDF Copy :https://wemarketresearch.com/reports/request-free-sample-pdf/multimodal-ai-market/1573
Key Components of the Multimodal AI Market
Software: The software segment of the multimodal AI market includes tools, platforms, and applications that enable the integration of different data types and processing techniques. This software can handle complex tasks like natural language processing (NLP), image recognition, and speech synthesis. As AI software continues to evolve, it is becoming more accessible to organizations across various industries.
Services: The services segment encompasses consulting, system integration, and maintenance services. These services help businesses deploy and optimize multimodal AI solutions. As organizations seek to leverage AI capabilities for competitive advantage, the demand for expert services in AI implementation and support is growing rapidly.
Multimodal AI Market by Data Modality
Image Data: The ability to process and understand image data is critical for sectors such as healthcare (medical imaging), retail (visual search), and automotive (autonomous vehicles). The integration of image data into multimodal AI systems is expected to drive significant market growth in the coming years.
Text Data: Text data is one of the most common data types used in AI systems, especially in applications involving natural language processing (NLP). Multimodal AI systems that combine text data with other modalities, such as speech or image data, are enabling advanced search engines, chatbots, and automated content generation tools.
Speech & Voice Data: The ability to process speech and voice data is a critical component of many AI applications, including virtual assistants, customer service bots, and voice-controlled devices. Multimodal AI systems that combine voice recognition with other modalities can create more accurate and interactive experiences.
Multimodal AI Market by Enterprise Size
Large Enterprises: Large enterprises are increasingly adopting multimodal AI technologies to streamline operations, improve customer interactions, and enhance decision-making. These companies often have the resources to invest in advanced AI systems and are well-positioned to leverage the benefits of integrating multiple data types into their processes.
Small and Medium Enterprises (SMEs): SMEs are gradually adopting multimodal AI as well, driven by the affordability of AI tools and the increasing availability of AI-as-a-service platforms. SMEs are using AI to enhance their customer service, optimize marketing strategies, and gain insights from diverse data sources without the need for extensive infrastructure.
Key Applications of Multimodal AI
Media & Entertainment: In the media and entertainment industry, multimodal AI is revolutionizing content creation, recommendation engines, and personalized marketing. AI systems that can process text, images, and video simultaneously allow for better content discovery, while AI-driven video editing tools are streamlining production processes.
Banking, Financial Services, and Insurance (BFSI): The BFSI sector is increasingly utilizing multimodal AI to improve customer service, detect fraud, and streamline operations. AI-powered chatbots, fraud detection systems, and risk management tools that combine speech, text, and image data are becoming integral to financial institutions’ strategies.
Automotive & Transportation: Autonomous vehicles are perhaps the most high-profile application of multimodal AI. These vehicles combine data from cameras, sensors, radar, and voice commands to make real-time driving decisions. Multimodal AI systems are also improving logistics and fleet management by optimizing routes and analyzing traffic patterns.
Gaming: The gaming industry is benefiting from multimodal AI in areas like player behavior prediction, personalized content recommendations, and interactive experiences. AI systems are enhancing immersive gameplay by combining visual, auditory, and textual data to create more realistic and engaging environments.
Regional Insights
North America: North America is a dominant player in the multimodal AI market, particularly in the U.S., which leads in AI research and innovation. The demand for multimodal AI is growing across industries such as healthcare, automotive, and IT, with major companies and startups investing heavily in AI technologies.
Europe: Europe is also seeing significant growth in the adoption of multimodal AI, driven by its strong automotive, healthcare, and financial sectors. The region is focused on ethical AI development and regulations, which is shaping how AI technologies are deployed.
Asia-Pacific: Asia-Pacific is expected to experience the highest growth rate in the multimodal AI market, fueled by rapid technological advancements in countries like China, Japan, and South Korea. The region’s strong focus on AI research and development, coupled with growing demand from industries such as automotive and gaming, is propelling market expansion.
Key Drivers of the Multimodal AI Market
Technological Advancements: Ongoing innovations in AI algorithms and hardware are enabling more efficient processing of multimodal data, driving the adoption of multimodal AI solutions across various sectors.
Demand for Automation: Companies are increasingly looking to automate processes, enhance customer experiences, and gain insights from diverse data sources, fueling demand for multimodal AI technologies.
Personalization and Customer Experience: Multimodal AI is enabling highly personalized experiences, particularly in media, healthcare, and retail. By analyzing multiple types of data, businesses can tailor products and services to individual preferences.
Conclusion
The Global Multimodal AI Market is set for Tremendous growth in the coming decade, with applications spanning industries like healthcare, automotive, entertainment, and finance. As AI technology continues to evolve, multimodal AI systems will become increasingly vital for businesses aiming to harness the full potential of data and automation. With a projected CAGR of 35.8%, the market will see a sharp rise in adoption, driven by advancements in AI software and services, as well as the growing demand for smarter, more efficient solutions across various sectors.
1 note
·
View note
Text
AI GEMINI
youtube
#google gemini#google gemini ai#future of ai#artificial intelligence#gemini#gemini google#google ai gemini#what is google gemini#google ai#gemini ai#google#multimodal ai#gemini ai google#google gemini demo#gemini google ai#will google gemini be free#new google ai gemini#google gemini revolutionizing tech review#multimodal#the future of ai#google gemini explained#multimodal intelligence#multimodal intelligence network#Youtube
0 notes
Text
Emerging Trends in AI in 2024

Artificial Intelligence (AI) is not just a buzzword anymore; it’s a driving force behind the digital transformation across industries. As we move into 2024, AI continues to evolve rapidly, introducing new possibilities and challenges. From enhancing business processes to reshaping entire sectors, AI's influence is expanding. Here, we explore the emerging AI trends in 2024 that are set to redefine how we live, work, and interact with technology.
Emerging trends in Artificial Intelligence (AI) in 2024
AI-Driven Creativity: Expanding the Horizons of Innovation One of the most exciting trends in AI for 2024 is its growing role in creative processes. AI is no longer limited to analyzing data or automating tasks; it is now actively contributing to creative fields. AI-driven creativity refers to the use of AI to generate new ideas, designs, and even art. This trend is particularly prominent in industries such as fashion, entertainment, and design, where AI algorithms are being used to create novel designs, suggest creative concepts, and even compose music. For example, AI can analyze vast amounts of data to identify emerging design trends, which can then be used to create new products that align with consumer preferences. In the entertainment industry, AI is being used to generate scripts, compose music, and even create digital art. This trend is pushing the boundaries of creativity, enabling human creators to collaborate with AI in unprecedented ways. As AI continues to develop its creative capabilities, we can expect to see more AI-generated content across various media, leading to a fusion of human and machine creativity that will redefine innovation.
AI-Powered Automation: Transforming Business Operations Automation has been a key application of AI for years, but in 2024, AI-powered automation is set to reach new levels of sophistication. AI is increasingly being used to automate complex business processes, from supply chain management to customer service. This trend is driven by advancements in machine learning and natural language processing, which enable AI systems to perform tasks that were previously thought to require human intelligence. One area where AI-powered automation is making a significant impact is in customer service. AI chatbots and virtual assistants are becoming more advanced, capable of understanding and responding to complex customer queries in real-time. This not only improves the customer experience but also reduces the need for human intervention, allowing businesses to operate more efficiently. In addition to customer service, AI-powered automation is also being used in manufacturing, logistics, and finance. For example, AI algorithms can optimize production schedules, predict maintenance needs, and even automate financial transactions. As businesses continue to adopt AI-powered automation, they can expect to see increased efficiency, reduced costs, and improved decision-making capabilities.
AI and Sustainability: Driving Environmental Innovation As the world grapples with the challenges of climate change, AI is emerging as a powerful tool for driving sustainability. In 2024, AI is being used to develop innovative solutions that reduce environmental impact and promote sustainability across various sectors. This trend is particularly evident in areas such as energy management, agriculture, and transportation. One of the most promising applications of AI in sustainability is in energy management. AI algorithms can analyze energy consumption patterns and optimize the use of renewable energy sources, such as solar and wind power. This not only reduces carbon emissions but also lowers energy costs for businesses and consumers. In agriculture, AI is being used to optimize farming practices, from precision irrigation to crop monitoring. By analyzing data from sensors and satellites, AI can help farmers make more informed decisions, leading to increased crop yields and reduced resource use. This trend is critical for addressing the global challenges of food security and environmental sustainability. Moreover, AI is playing a crucial role in the development of smart cities, where it is used to optimize transportation systems, reduce traffic congestion, and minimize pollution. As AI continues to drive sustainability, it will play a pivotal role in creating a more sustainable and resilient future.
AI Ethics and Responsible AI: Ensuring Trust and Transparency As AI becomes more integrated into our daily lives, concerns about its ethical implications are growing. In 2024, AI ethics and responsible AI development are emerging as critical areas of focus for businesses, governments, and researchers. Ensuring that AI is developed and used responsibly is essential for maintaining public trust and preventing unintended consequences. One of the key ethical concerns surrounding AI is bias in decision-making algorithms. AI systems are often trained on historical data, which may contain biases that can lead to unfair outcomes. For example, AI algorithms used in hiring or lending decisions may inadvertently discriminate against certain groups. To address this issue, researchers and companies are developing techniques to detect and mitigate bias in AI systems. Another important aspect of AI ethics is transparency. Users need to understand how AI systems make decisions, especially when those decisions have significant impacts on their lives. This has led to a push for explainable AI, where the decision-making process is clear and understandable to humans. Additionally, there is a growing emphasis on AI governance, where organizations are establishing frameworks and guidelines for responsible AI development. This includes ensuring that AI systems are used in ways that align with ethical principles, such as fairness, accountability, and transparency. As AI continues to evolve, addressing its ethical challenges will be critical to ensuring that it benefits society as a whole.
AI in Healthcare: Revolutionizing Patient Care The integration of AI in healthcare is not a new trend, but in 2024, it is set to revolutionize patient care in unprecedented ways. AI is being used to improve diagnostics, treatment planning, and patient outcomes, making healthcare more efficient and accessible. One of the most significant applications of AI in healthcare is in medical imaging. AI algorithms can analyze medical images, such as X-rays and MRIs, with incredible accuracy, often detecting abnormalities that might be missed by human doctors. This can lead to earlier diagnosis and treatment of diseases like cancer, ultimately saving lives. In addition to diagnostics, AI is also being used to develop personalized treatment plans. By analyzing a patient's genetic information, medical history, and lifestyle, AI can recommend treatments that are most likely to be effective for that individual. This personalized approach not only improves patient outcomes but also reduces the likelihood of adverse reactions to treatments. Moreover, AI is playing a crucial role in drug discovery. AI algorithms can analyze vast amounts of data to identify potential new drugs and predict how they will interact with the human body. This accelerates the drug development process, bringing new treatments to market faster. As AI continues to advance in healthcare, it will lead to better patient outcomes, more efficient healthcare systems, and ultimately, a healthier population. Conclusion The year 2024 is set to be a transformative one for AI, with emerging trends that will shape the future of technology, business, and society. From AI-driven creativity and automation to sustainability and ethics, these trends highlight the growing influence of AI in our lives. As we navigate this rapidly evolving landscape, it is essential to stay informed and prepared for the changes that lie ahead. By embracing these emerging AI trends, businesses and individuals can harness the power of AI to drive innovation, improve outcomes, and create a better future.
0 notes
Text
Gemini: Google Stirs Controversy Again with Generative AI Product Announcement
(Image credit : Google, Google DeepMind) Google announced its new AI model, Gemini, on December 6, 2023. In this blog, we will delve into the controversy surrounding this announcement and outline the steps the company should take to avoid similar setbacks in future product launches. Table of Contents The AnnouncementThe ControversyRecommendationsAdditional Readings The Announcement On…

View On WordPress
0 notes
Text
#Gemini AI#Google DeepMind#Artificial Intelligence#Multimodal AI#Human-like communication#AI creativity#Data analysis#AI applications#Future of AI
0 notes
Text
Google Gen AI SDK, Gemini Developer API, and Python 3.13
A Technical Overview and Compatibility Analysis 🧠 TL;DR – Google Gen AI SDK + Gemini API + Python 3.13 Integration 🚀 🔍 Overview Google’s Gen AI SDK and Gemini Developer API provide cutting-edge tools for working with generative AI across text, images, code, audio, and video. The SDK offers a unified interface to interact with Gemini models via both Developer API and Vertex AI 🌐. 🧰 SDK…
#AI development#AI SDK#AI tools#cloud AI#code generation#deep learning#function calling#Gemini API#generative AI#Google AI#Google Gen AI SDK#LLM integration#multimodal AI#Python 3.13#Vertex AI
0 notes
Text
ChatGPT’s First Year: The AI-mpressive Journey from Bytes to Insights
The Genesis of a Digital GiantChatGPT’s story is a testament to human ingenuity. Birthed by OpenAI, a company co-founded by the visionary Sam Altman, ChatGPT is the offspring of years of groundbreaking work in AI. OpenAI, once a non-profit, evolved into a capped-profit entity, striking a balance between ethical AI development and the need for sustainable growth. Altman, a figure both admired and…

View On WordPress
#AI#AI Development#AI Experts#AI in Business#AI Integration#Artificial Intelligence#ChatGPT#content creation#Machine Learning#Multimodal AI#technology
0 notes
Text
Pegasus 1.2: High-Performance Video Language Model

Pegasus 1.2 revolutionises long-form video AI with high accuracy and low latency. Scalable video querying is supported by this commercial tool.
TwelveLabs and Amazon Web Services (AWS) announced that Amazon Bedrock will soon provide Marengo and Pegasus, TwelveLabs' cutting-edge multimodal foundation models. Amazon Bedrock, a managed service, lets developers access top AI models from leading organisations via a single API. With seamless access to TwelveLabs' comprehensive video comprehension capabilities, developers and companies can revolutionise how they search for, assess, and derive insights from video content using AWS's security, privacy, and performance. TwelveLabs models were initially offered by AWS.
Introducing Pegasus 1.2
Unlike many academic contexts, real-world video applications face two challenges:
Real-world videos might be seconds or hours lengthy.
Proper temporal understanding is needed.
TwelveLabs is announcing Pegasus 1.2, a substantial industry-grade video language model upgrade, to meet commercial demands. Pegasus 1.2 interprets long films at cutting-edge levels. With low latency, low cost, and best-in-class accuracy, model can handle hour-long videos. Their embedded storage ingeniously caches movies, making it faster and cheaper to query the same film repeatedly.
Pegasus 1.2 is a cutting-edge technology that delivers corporate value through its intelligent, focused system architecture and excels in production-grade video processing pipelines.
Superior video language model for extended videos
Business requires handling long films, yet processing time and time-to-value are important concerns. As input films increase longer, a standard video processing/inference system cannot handle orders of magnitude more frames, making it unsuitable for general adoption and commercial use. A commercial system must also answer input prompts and enquiries accurately across larger time periods.
Latency
To evaluate Pegasus 1.2's speed, it compares time-to-first-token (TTFT) for 3–60-minute videos utilising frontier model APIs GPT-4o and Gemini 1.5 Pro. Pegasus 1.2 consistently displays time-to-first-token latency for films up to 15 minutes and responds faster to lengthier material because to its video-focused model design and optimised inference engine.
Performance
Pegasus 1.2 is compared to frontier model APIs using VideoMME-Long, a subset of Video-MME that contains films longer than 30 minutes. Pegasus 1.2 excels above all flagship APIs, displaying cutting-edge performance.
Pricing
Cost Pegasus 1.2 provides best-in-class commercial video processing at low cost. TwelveLabs focusses on long videos and accurate temporal information rather than everything. Its highly optimised system performs well at a competitive price with a focused approach.
Better still, system can generate many video-to-text without costing much. Pegasus 1.2 produces rich video embeddings from indexed movies and saves them in the database for future API queries, allowing clients to build continually at little cost. Google Gemini 1.5 Pro's cache cost is $4.5 per hour of storage, or 1 million tokens, which is around the token count for an hour of video. However, integrated storage costs $0.09 per video hour per month, x36,000 less. Concept benefits customers with large video archives that need to understand everything cheaply.
Model Overview & Limitations
Architecture
Pegasus 1.2's encoder-decoder architecture for video understanding includes a video encoder, tokeniser, and big language model. Though efficient, its design allows for full textual and visual data analysis.
These pieces provide a cohesive system that can understand long-term contextual information and fine-grained specifics. It architecture illustrates that tiny models may interpret video by making careful design decisions and solving fundamental multimodal processing difficulties creatively.
Restrictions
Safety and bias
Pegasus 1.2 contains safety protections, but like any AI model, it might produce objectionable or hazardous material without enough oversight and control. Video foundation model safety and ethics are being studied. It will provide a complete assessment and ethics report after more testing and input.
Hallucinations
Occasionally, Pegasus 1.2 may produce incorrect findings. Despite advances since Pegasus 1.1 to reduce hallucinations, users should be aware of this constraint, especially for precise and factual tasks.
#technology#technews#govindhtech#news#technologynews#AI#artificial intelligence#Pegasus 1.2#TwelveLabs#Amazon Bedrock#Gemini 1.5 Pro#multimodal#API
2 notes
·
View notes
Text
Más allá del chatbot: construyendo agentes reales con IA
Vengo escuchando desde hace un tiempo que un modelo de lenguaje al que, usando ChatGPT o Copilot, le subes archivos y le haces preguntas sobre estos artículos, es un “agente”. A simple vista, parece solo una herramienta que responde preguntas usando texto. Eso no tiene pinta de ser un agente. Pero, ¿lo es?
Tras ver este video sobre los diferentes tipos de agentes de IA que existen, creo que ya sé por qué estamos llamando "agentes" a ese uso concreto de los modelos.
Los 5 tipos de agentes de IA
Según la teoría clásica (ver “Artificial Intelligence: A Modern Approach”, 4th edition, de Stuart Russell y Peter Norvig, sección 2.4, "The Structure of Agents"), los agentes se clasifican así:
Reflexivo simple: responde con reglas fijas.
Basado en modelos: tiene una representación del entorno y memoria.
Basado en objetivos: toma decisiones según metas.
Basado en utilidad: evalúa opciones según preferencia/valor.
De aprendizaje: mejora con la experiencia.
¿Dónde encaja el caso que estamos analizando, ese modelo al que le subimos unos documentos y le hacemos preguntas sobre ellos? Eso que OpenAI llama GPTs y que Microsoft llama "agentes" en el Copilot Studio, ¿con cuál de los anteriores tipos de agentes se corresponde?
Si lo usamos solo para responder una pregunta directa → se parece al reflexivo simple.
Si analiza archivos cargados y extrae conclusiones dispersas → actúa como basado en modelos.
Si le damos tareas claras (resumir, estructurar, comparar) → parece el basado en objetivos.
Si optimiza claridad o formato según instrucciones → podría ser el basado en utilidad.
Si el sistema aprende de nosotros y mejora con el tiempo → sería un agente de aprendizaje.
Por lo tanto, GPT (o el mismo caso hecho en Copilot) por sí mismo no es un agente completo, pero integrado con sistemas (nosotros mismos, por ejemplo) que le dan contexto, metas, memoria y feedback, claramente se convierte en uno.
Entonces, ¿cómo sería un agente “de verdad”? Un agente de verdad es uno que actúa como un sistema autónomo inteligente, no solo uno que responde preguntas.
Para aclarar qué es un agente en términos más prácticos, vamos a intentar comprender la arquitectura MCP (Model Context Processing), propuesta por Anthropic para construir agentes y que está siendo adoptada por la industria.
MCP: Conectando agentes de IA con el mundo real
MCP (Model Context Protocol) es una infraestructura para que modelos de lenguaje puedan interactuar de forma segura y estructurada con herramientas externas, APIs, bases de datos y otros sistemas disponibles dentro de la organización.
Aunque no es una arquitectura cognitiva completa, puede servir como la “capa de integración” que permite a un agente cognitivo acceder a información en tiempo real, ejecutar acciones y trabajar sobre entornos reales. MCP es la “puerta al mundo real” para agentes que necesitan trabajar con datos y sistemas externos.
Ejemplo práctico: Un agente que resuelve problemas en una organización
Imaginemos un asistente corporativo inteligente que:
a) hemos diseñado con una arquitectura cognitiva basada en módulos (percepción, cognición, acción) y que, además,
b) se conecta al ecosistema de la empresa mediante el protocolo MCP (Model Context Protocol) de Anthropic.
Veamos qué funciones contendría cada uno de los tres módulos cognitivos que compondrían dicho asistente y cómo interactuaría con el mundo a su alrededor mediante MCP:
1. Percepción
Lee bases de datos, informes, logs, emails, APIs internas.
Recibe consultas humanas o detecta anomalías automáticamente.
2. Cognición
Usa uno o varios GPTs para interpretar texto, combinar datos y razonar.
Planea pasos: “analizar gastos”, “comparar con presupuestos”, “detectar desviaciones”.
Mantiene memoria de su contexto de trabajo, objetivos y estados intermedios.
3. Acción
Consulta sistemas, genera informes, dispara flujos de trabajo.
Toma decisiones o propone acciones con justificación.
Aprende de feedback: mejora sus planes con el tiempo.
Veamos ahora a ese agente en funcionamiento en un caso concreto:
Percibe: detecta aumento de costes en logística.
Razona: analiza contratos, identifica rutas ineficientes, predice impacto.
Actúa: propone cambios, notifica a compras, inicia seguimiento.
¿Por qué queremos construir este tipo de agentes?
Porque van más allá de un chatbot con el que conversamos, como ChatGPT.
Porque automatizan la resolución de problemas reales.
Porque combinan todos los datos de la organización, eliminándose así los silos de información aislados.
Porque actúan con propósito, objetivo. No se limitan a responder preguntas.
La IA no es solo generar texto en respuesta a una pregunta. Es una IA estructurada, autónoma y conectada. Y arquitecturas cognitivas combinadas con protocolos como MCP hacen posible que los agentes realmente trabajen con nosotros —y por nosotros— en contextos organizativos complejos. Es comportamiento estructurado, toma de decisiones, acción. Eso es un agente.

#inteligencia artificial#IA#GPT#agentes inteligentes#machine learning#MCP#Anthropic#arquitectura cognitiva#tecnología empresarial#automatización#datos empresariales#sistemas inteligentes#procesamiento multimodal#LLM#AI agents
0 notes
Text
A New Player in the League of LLMs – Mistral Le Chat | Infographic
Learn about the latest player in the world of LLMs – the Mistral’s Le Chat and understand in this infographic its features, and how it compares with leading players.
Read More: https://shorturl.at/N6pIs
Mistral Le Chat, AI assistant, multimodal AI model, AI models, Machine learning algorithms, AI chatbots, large language models, best AI certifications, AI Engineer, AI skills
0 notes
Text
AI-Driven Content Creation Tools for Smarter Marketing | OneAIChat Boost your brand with AI-driven content creation—generate high-quality, engaging content faster and smarter with advanced artificial intelligence tools.
#ai driven content creation#ai powered content generation#multimodal integration#ai powered content creation
0 notes
Text
Ask A Genius 1353: GPT-5, AI Consciousness, and Crossover Country
Scott Douglas Jacobsen: You have been listening to a country song designed for people who do not usually enjoy country music—not the traditional kind aimed at long-time fans, but rather a version that tries to appeal to outsiders. Rick Rosner: There is crossover country, of course. However, in Albuquerque, I could only find stations playing formulaic country music on the radio. There is…
#AGI without consciousness#AI and nuclear war risk#multimodal AI integration#Rick Rosner#Scott Douglas Jacobsen
0 notes
Text
Google Gemini: The Ultimate Guide to the Most Advanced AI Model Ever
We hope you enjoyed this article and found it informative and insightful. We would love to hear your feedback and suggestions, so please feel free to leave a comment below or contact us through our website. Thank you for reading and stay tuned for more
Google Gemini: A Revolutionary AI Model that Can Shape the Future of Technology and Society. Artificial intelligence (AI) is one of the most exciting and rapidly evolving fields of technology today. From personal assistants to self-driving cars, AI is transforming various aspects of our lives and society. However, the current state of AI is still far from achieving human-like intelligence and…
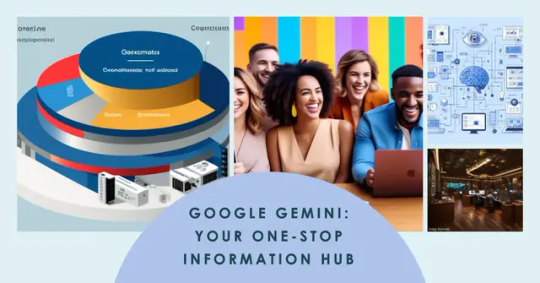
View On WordPress
#AI ethics#AI model#AI research#API integration#artificial intelligence#business#creative content generation#discovery#Education#google gemini#language model#learning#marketing#memory#multimodal AI#personal assistants#planning#productivity tools#scientific research#tool integration
0 notes
Text
Meta presenta la nueva versión de su inteligencia artificial: Llama 4
Lanzamiento de Llama 4: La Nueva Versión de la IA de Meta que Revoluciona WhatsApp, Instagram y Más Fecha de publicación: 5 de abril de 2025 Meta, la empresa matriz de Facebook, Instagram y WhatsApp, ha dado un paso gigante en el mundo de la inteligencia artificial con el lanzamiento de Llama 4, su modelo de IA más avanzado hasta la fecha. Anunciado por Mark Zuckerberg el 5 de abril de 2025, este…
#código abierto#Facebook#IA multimodal#innovación digital#Instagram#inteligencia artificial#Llama 4#LlamaCon#Mark Zuckerberg#Messenger#Meta AI#mezcla de expertos#tecnología 2025#WhatsApp
0 notes