#Neural Network Projects Using Matlab
Explore tagged Tumblr posts
Text
Exploring the Potential of Neural Networks In Matlab Projects
At Takeoff Edu Group, we are a team of experts that help beginners to explore the basics details of Neural Network Projects in MATLAB. The core of our curriculum is the combination of the theory with the practice, which allows students to be well-versed in the concept of neural networks. Participants acquire the necessary knowledge through practical projects. This learning process enables them to solve particular problems boldly. Solve the mysteries of Matlab and neural networks together with us to increase your expertise to the next level.
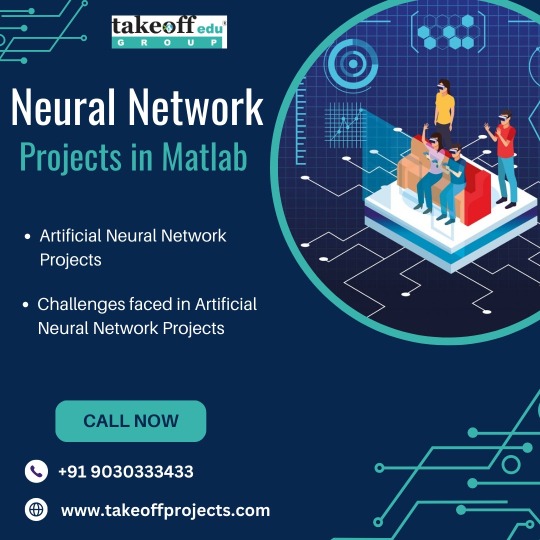
In Matlab Neural Networks Projects, potential users will be taught to use the Matlab programming language for the development and experimentation of artificial neural networks (ANNs). The neural networks are system of computers approved on mimicking the structure and function of the human brain. comprised of connected nodes called neurons, which process and spike the information.
In MATLAB users can develop neural networks in many forms, for example, feedforward neural networks, convolutional neural networks (CNNs), and recurrent neural networks (RNNs). This way they can be made to recognize complex patterns, to classify data, to make predictions and to do this or that.
The user-friendly interface and wide library of tools for the machine learning and deep learning in the Matlab package are one of the key advantages of using it for neural network data science projects. MATLAB functions, and algorithms for designing training and evaluating neural ensembles ensure accessibility to both experienced users and beginners.
Matlab provides a graphical interface through which a user can create models architecture, adjust parameters as well as gather performance level statistics to fine-tune the model. And on the other hand, Matlab assures the modularity of the neural networks with other techniques and algorithms thus allowing for interdisciplinary research and applications development.
Conclusion
In conclusion, Neural Network Projects in Matlab offered by Takeoff hold immense potential to revolutionize various industries. From improving image and speech recognition to aiding in medical diagnosis, financial forecasting, and autonomous systems, these projects span diverse applications. By harnessing the capabilities of Matlab, researchers and practitioners can unlock innovative solutions to complex problems. Takeoff provides a platform for individuals to delve into the realm of neural networks, fostering creativity and driving impactful advancements in their respective fields.
#Neural Network Projects Using Matlab#Matlab Neural Network Projects#Matlab Neural Network Projects Ideas#Matlab Neural Network Online Projects#Matlab Neural Network Projects Help
0 notes
Text
Best AI and Data Science Course College in Tamil Nadu: Where Innovation Meets Education
Choosing the best AI and Data Science course college in Tamil Nadu is no longer just about getting a degree — it’s about aligning yourself with the future of innovation, automation, and global tech opportunities. In an era where Artificial Intelligence is revolutionizing every industry, from healthcare to finance to entertainment, Tamil Nadu has emerged as a hotspot for aspiring AI professionals. Among the rising stars in this space is Mailam Engineering College, where education is more than textbooks — it’s a bridge to cutting-edge careers.
The AI Revolution is Here — Are You Ready?
Artificial Intelligence and Data Science are no longer buzzwords; they’re necessities. Whether it’s an app predicting your shopping behavior or a system detecting early signs of disease, AI is behind it all. India, especially Tamil Nadu, is rapidly adapting to this wave, with institutions evolving to equip students with the skills they need to lead the tech revolution.
But here’s the catch — not all colleges are created equal. Finding an AI and Data Science course college in Tamil Nadu that offers more than just theory — one that focuses on hands-on skills, real-world projects, and global exposure — is the key to staying ahead.
What Makes a College the "Best"?
When evaluating which AI and Data Science course college in Tamil Nadu is best suited for your future, you need to go beyond glossy brochures. Look for:
Updated Curriculum: AI is evolving fast. The syllabus must include Machine Learning, Deep Learning, Natural Language Processing, Computer Vision, and Big Data Analytics.
Practical Experience: Projects, internships, hackathons, and real datasets should be the norm — not the exception.
Strong Faculty: A mix of academic scholars and industry experts brings both depth and relevance to the classroom.
Placement Support: Connections with companies that use AI for real impact, not just IT services.
Research Opportunities: The best colleges empower students to innovate, publish, and patent.
And that’s exactly where Mailam Engineering College stands out.
Why Mailam Engineering College Is a Top Choice in 2025
If you’re searching for the most dynamic and future-forward AI and Data Science course college in Tamil Nadu, Mailam Engineering College deserves a spot at the top of your list. Here’s why:
1. Industry-Centric Curriculum
Mailam’s B.Tech in Artificial Intelligence and Data Science isn’t just about theory. It dives deep into tools like Python, TensorFlow, PowerBI, and MATLAB. From foundational algorithms to advanced neural networks, students learn it all. The curriculum is designed in consultation with AI professionals to ensure industry relevance.
2. Hands-On Learning Environment
At Mailam, labs are buzzing with innovation. Students regularly work on real-time projects like:
Smart traffic monitoring systems
Crop disease prediction tools
AI-powered personal assistants
Data dashboards for business intelligence
These aren’t just academic tasks — they prepare students for real-world challenges.
3. Mentorship from Experts
The faculty includes PhD holders, researchers, and engineers with industry experience. Guest lectures from professionals in Google, TCS, Infosys, and emerging startups ensure students stay current with trends.
4. Career-Ready Training
Every year, Mailam Engineering College’s placement cell ensures students meet recruiters from core AI-driven companies. They also offer:
Resume-building workshops
Mock technical interviews
Coding competitions
Soft skills training
Many graduates have landed roles as Data Scientists, Machine Learning Engineers, AI Analysts, and even R&D interns abroad.
What Students Are Saying
"At Mailam, I didn’t just study AI — I lived it. We built models, handled big data, and presented ideas in front of real engineers from the industry. It shaped the way I think." — Vishnu Priya, Final Year Student
"Getting placed in an AI startup straight out of college was surreal. Thanks to Mailam’s support and continuous motivation from our professors, I had both the knowledge and the confidence to face the world." — Karthik S., Alumni, Class of 2023
A Culture of Innovation
What sets Mailam apart from any other AI and Data Science course college in Tamil Nadu is its culture. Students are encouraged to:
File patents for their innovations
Present papers in national/international conferences
Collaborate on interdisciplinary projects
Compete in global hackathons and AI challenges
This approach doesn’t just make them employable — it turns them into innovators, creators, and leaders.
Affordable and Accessible Excellence
Education in AI shouldn’t be a privilege for a few. Mailam Engineering College keeps its fee structure affordable while providing scholarships, financial aid, and installment plans to ensure every talented student can pursue their dream.
For many families in Tamil Nadu, this balance of affordability and academic excellence makes Mailam the ideal AI and Data Science course college in Tamil Nadu.
Tamil Nadu: A Growing Hub for AI Education
With IT parks in Chennai, Coimbatore, and Madurai expanding rapidly, Tamil Nadu is becoming a preferred destination for AI-driven businesses. Colleges that prepare students with the right mix of technical depth and practical insight are seeing unprecedented growth in demand.
Mailam Engineering College is uniquely positioned to meet this demand with its tailored approach. No wonder it's often listed among the most promising AI and Data Science course colleges in Tamil Nadu by academic reviewers and tech recruiters.
Final Word: Make the Smart Choice
If you're someone who's fascinated by how Netflix recommends your shows, how Alexa answers your questions, or how Tesla drives itself — AI is the field for you. But your success depends on where you learn.
In a sea of options, Mailam Engineering College offers the perfect combination of academic depth, hands-on learning, innovation, and placement support. It doesn’t just promise a degree — it delivers a future.
For those ready to embrace the tech revolution, there’s no better AI and Data Science course college in Tamil Nadu than Mailam Engineering College. Step in today, and step into tomorrow’s technology world with confidence.
0 notes
Text
MCA in AI: High-Paying Job Roles You Can Aim For

Artificial Intelligence (AI) is revolutionizing industries worldwide, creating exciting and lucrative career opportunities for professionals with the right skills. If you’re pursuing an MCA (Master of Computer Applications) with a specialization in AI, you are on a promising path to some of the highest-paying tech jobs.
Here’s a look at some of the top AI-related job roles you can aim for after completing your MCA in AI:
1. AI Engineer
Average Salary: $100,000 - $150,000 per year Role Overview: AI Engineers develop and deploy AI models, machine learning algorithms, and deep learning systems. They work on projects like chatbots, image recognition, and AI-driven automation. Key Skills Required: Machine learning, deep learning, Python, TensorFlow, PyTorch, NLP
2. Machine Learning Engineer
Average Salary: $110,000 - $160,000 per year Role Overview: Machine Learning Engineers build and optimize algorithms that allow machines to learn from data. They work with big data, predictive analytics, and recommendation systems. Key Skills Required: Python, R, NumPy, Pandas, Scikit-learn, cloud computing
3. Data Scientist
Average Salary: $120,000 - $170,000 per year Role Overview: Data Scientists analyze large datasets to extract insights and build predictive models. They help businesses make data-driven decisions using AI and ML techniques. Key Skills Required: Data analysis, statistics, SQL, Python, AI frameworks
4. Computer Vision Engineer
Average Salary: $100,000 - $140,000 per year Role Overview: These professionals work on AI systems that interpret visual data, such as facial recognition, object detection, and autonomous vehicles. Key Skills Required: OpenCV, deep learning, image processing, TensorFlow, Keras
5. Natural Language Processing (NLP) Engineer
Average Salary: $110,000 - $150,000 per year Role Overview: NLP Engineers specialize in building AI models that understand and process human language. They work on virtual assistants, voice recognition, and sentiment analysis. Key Skills Required: NLP techniques, Python, Hugging Face, spaCy, GPT models
6. AI Research Scientist
Average Salary: $130,000 - $200,000 per year Role Overview: AI Research Scientists develop new AI algorithms and conduct cutting-edge research in machine learning, robotics, and neural networks. Key Skills Required: Advanced mathematics, deep learning, AI research, academic writing
7. Robotics Engineer (AI-Based Automation)
Average Salary: $100,000 - $140,000 per year Role Overview: Robotics Engineers design and program intelligent robots for industrial automation, healthcare, and autonomous vehicles. Key Skills Required: Robotics, AI, Python, MATLAB, ROS (Robot Operating System)
8. AI Product Manager
Average Salary: $120,000 - $180,000 per year Role Overview: AI Product Managers oversee the development and deployment of AI-powered products. They work at the intersection of business and technology. Key Skills Required: AI knowledge, project management, business strategy, communication
Final Thoughts
An MCA in AI equips you with specialized technical knowledge, making you eligible for some of the most sought-after jobs in the AI industry. By gaining hands-on experience in machine learning, deep learning, NLP, and big data analytics, you can land high-paying roles in top tech companies, startups, and research institutions.
If you’re looking to maximize your career potential, staying updated with AI advancements, building real-world projects, and obtaining industry certifications can give you a competitive edge.
0 notes
Text
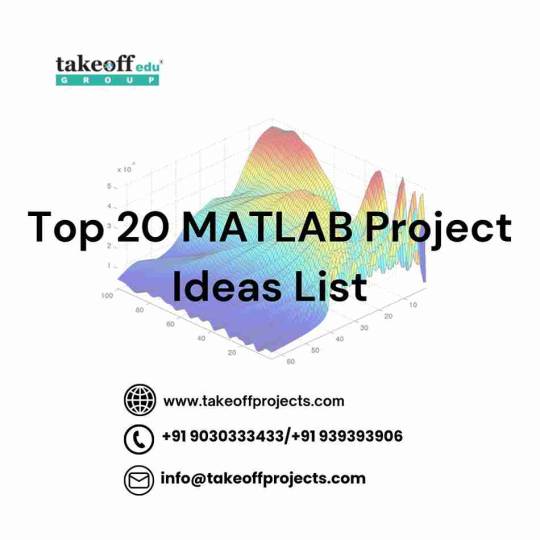
Top 20 MATLAB Project Ideas List
1. Face Recognition System: Establish an approach that identifies faces, and then checks the information provided with image processing techniques.
2. Speech Signal Analysis: Which are then stored for analysis of features such as pitch and intensity as well as individual phonemes.
3. Object Detection Using Deep Learning: Use the machine learning technique to teach the neural network that it is working with to detect objects on images.
4. Simulink for Electric Vehicles: Models and simulates electrical vehicle behaviour.
5. Digital Image Watermarking: Acquire and remove watermarks as a form of protection for material posted on the Internet.
6. Automatic License Plate Recognition: Recognize license plates of the vehicles.
7. Heart Rate Monitoring System: Measure the privacy using ECG signal analysis of the heart rate of a patient.
8. Cryptography System: Further create a mechanism for encryption and decryption.
9. Renewable Energy Modelling: Did or designed systems for the generation of solar or wind energy.
10. Weather Forecasting Tool: Forecast climate conditions from spread market datasets based on time-line records.
11. Edge Detection: The raw imagery requires the creation of new algorithms aimed at detecting edges in images.
12. Signal Compression: Use even some of the technological advance techniques such as wavelet transform for data compression.
13. Stock Market Prediction: Forecast up or down movements in stock prices with artificial intelligence.
14. Lane Detection for Vehicles: Detecting road lanes in videos.
15. Audio Noise Reduction: Remove noise from audio frequency and sounds.
16. Medical Image Analysis: Interpret MRI or CT scan images.
17. Robotics Path Planning: Teach robots how to move in and around with minimal wastage of time.
18. Fingerprint Recognition System: A biometric authentication should be developed.
19. Data Clustering Warning: Use k-means to cluster datasets.
20. Traffic Flow Simulation: Simulate and simulate traffic systems.
These projects cover the basic to the intermediate level and provide numerous ways to implement the use of MATLAB.
#embedded#matlab#projects#engineering#students#imageprocessing#imageanalysis#takeoffedugroup#takeoffprojects
0 notes
Text
The Future of Image Processing Education: AI Integration and Beyond
In the rapidly evolving field of image processing, staying updated with the latest trends is crucial for students aiming to excel in this dynamic discipline. One of the most significant developments in recent years has been the integration of artificial intelligence (AI) techniques into image processing education. This paradigm shift is reshaping how students approach complex tasks such as image classification, object detection, and image enhancement. Let's delve into how these advancements are transforming the educational landscape and what it means for aspiring image processing professionals.
AI Integration in Image Processing Education
AI, particularly machine learning and deep learning algorithms, is revolutionizing image processing curricula across educational institutions worldwide. Traditionally focused on mathematical and algorithmic approaches, modern image processing courses now emphasize practical applications of AI. Students are learning to harness AI tools to automate tasks that were once labor-intensive and time-consuming. Techniques like neural networks are enabling breakthroughs in fields ranging from medical imaging to autonomous vehicle technology.
Cloud-Based Learning Platforms
Another trend gaining traction in image processing education is the adoption of cloud-based learning platforms. These platforms provide students with access to powerful computational resources and specialized software tools without the need for expensive hardware investments. Through cloud computing, students can experiment with large datasets, run complex algorithms, and collaborate on projects seamlessly. This approach not only enhances learning flexibility but also prepares students for real-world applications where cloud-based image processing solutions are increasingly prevalent.
Enhanced Learning Experiences with AR and VR
Augmented Reality (AR) and Virtual Reality (VR) technologies are transforming the classroom experience in image processing. These immersive technologies allow students to visualize complex algorithms in 3D, interact with virtual models of imaging systems, and simulate realistic scenarios. By bridging the gap between theory and practice, AR and VR enhance comprehension and retention of image processing concepts. Educators are leveraging these tools to create engaging learning environments that foster creativity and deeper understanding among students.
Ethical Considerations in Image Processing Education
As image processing technologies become more powerful, addressing ethical considerations is paramount. Educators are incorporating discussions on privacy, bias in algorithms, and societal impacts into the curriculum. By raising awareness about these issues, students are better equipped to navigate the ethical challenges associated with deploying image processing solutions responsibly.
How Our Service Can Help
Navigating through the complexities of image processing assignments can be challenging. At matlabassignmentexperts.com, we understand the importance of mastering these concepts while balancing academic responsibilities. Our team of experts is dedicated to providing comprehensive assistance tailored to your specific needs. Whether you need help with understanding AI algorithms for image classification or completing a challenging assignment on object detection, our experienced tutors are here to support you. Let us help you excel in your studies and confidently do your image processing assignment.
Conclusion
In conclusion, the future of image processing education is bright with innovations like AI integration, cloud-based learning, and immersive technologies reshaping the learning landscape. As students, embracing these advancements not only enhances your skillset but also prepares you for a rewarding career in fields where image processing plays a pivotal role. Stay informed, explore new technologies, and leverage resources like MATLAB Assignment Experts to achieve your academic and professional goals in image processing.
0 notes
Text
Artificial Neural Network Projects for Engineering Students
With a dedicated department of trainers and professional experts in AI/ML Deep Learning, Takeoff projects have helped successfully execute hundreds of projects in Artificial Neural Networks. We can successfully build your Artificial Neural Network Project from the ground-up, and execute and deliver it on time. Or we can also provide assistance and guidance for your current Neural Network Project to ensure its success. You can select one of the ANNs from our database to work with or have your own idea. Feel free to get in contact, and we will deliver your project within your pre-specified timeframe.

Latest Artificial Neural Network Projects:
Emotion Recognition System with Emotion Recognition System and EEG Sensors
Initial catching kidney disease by Electrocardiogram Signals through Machine Learning modalities modelling.
Schematics of a Bio-signal Stress Detection System Using Machine Learning Techniques Based on the Concept.
Trending Artificial Neural Network Projects:
Face Expression Recognition Approach Through Combing Multi-Factor Fusion and High-Order SVD
The role of ANN in stroke image classification like the other neural networks is principally based on learning.
The Particle Swarm Optimization comes up with the selection of the most winning features involved in Face Recognition.
Standard Artificial Neural Network Projects:
Tomato Classification Using Machine Learning Algorithms K-NN, MLP and the K-means algorithm (Coloration Mysticism Organelles)
Cancer of Brain Tumours Classification from MR Images based upon a Neural Network with the use of Central Moments
Artificial Neural Network Projects:
Have you even thought of a concept, deep learning, on which currently many data process without any kind of human intervention? Interestingly, an observable advancement is seen in the field of Artificial Neural Networks that also help the object recognition reach such a high accuracy. An artificial neural network which works just like the biological neurons in our nervous system is called a computational model of the real decision-making neuron network. It deals with those that are many and having the layers with the nodes that trick the one's functioning to the neuron in the brain.
Challenges faced in Artificial Neural Network Projects:
Thus, the existence of such an apparent contradiction that is between the beauty of technology and the fear of losing our people the way they are is the reason why the area of applications of Artificial Intelligence and other machine learning fields are implemented in our real lives. The greats and the concerns are a package deal. At the core, we have the powerful Artificial Neural Networks doing the calculations. If you are a student intending to works on Artificial Neural Network Projects and below is what you should do; visit Takeoff Projects for a practical exposure and development of your skill-set. Visit More information: https://takeoffprojects.com/neural-network-projects-in-matlab
#final year students projects#engineering students projects#academic projects#ai projects#Artificial Neural Network Projects#Academic Students Projects
0 notes
Text
Utilizing Machine Learning in MATLAB Projects: From Theory to Application
Machine learning is a rapidly growing field in the world of technology, with numerous applications in various industries such as healthcare, finance, and manufacturing. It is a subset of artificial intelligence that focuses on teaching machines to learn from data, identify patterns, and make predictions or decisions without explicit instructions. MATLAB, a popular programming language and environment for scientific computing, has become a go-to tool for many machine learning projects due to its powerful algorithms and user-friendly interface. In this article, we will discuss the basics of machine learning in MATLAB and how it can be utilized in various projects.
Understanding Machine Learning in MATLAB MATLAB offers a comprehensive set of tools and functions for machine learning, making it an ideal platform for both beginners and experts in the field. The first step in utilizing machine learning in MATLAB is to understand the different types of learning algorithms. There are three main types of machine learning: supervised learning, unsupervised learning, and reinforcement learning.
Supervised learning involves training a model using a labeled dataset, where the input and output variables are known. It is used for tasks such as classification and regression, where the goal is to predict a categorical or continuous variable, respectively. Unsupervised learning, on the other hand, deals with finding patterns and relationships within an unlabeled dataset. It is often used for tasks like clustering and anomaly detection. Lastly, reinforcement learning involves training a model to make decisions based on rewards and punishments, similar to how humans learn.
Implementing Machine Learning in MATLAB Projects One of the main advantages of using MATLAB for machine learning projects is its extensive library of built-in functions and algorithms. These algorithms cover a wide range of techniques, from simple linear regression to complex deep learning models. This makes it easier for users to implement machine learning models without having to write code from scratch.
Furthermore, MATLAB also offers a range of visualization tools, allowing users to easily visualize their data and model performance. This is particularly useful for understanding the relationships and patterns within the data, as well as identifying any potential issues with the model.
Real-world Applications of Machine Learning in MATLAB MATLAB is widely used in various industries for its machine learning capabilities. In healthcare, it is used for tasks such as disease diagnosis, drug discovery, and medical imaging analysis. In finance, it is used for stock market prediction, fraud detection, and credit risk assessment. In manufacturing, it is used for predictive maintenance, quality control, and supply chain optimization.
One practical example of utilizing machine learning in MATLAB is in the field of image recognition. MATLAB has a built-in function called “trainNetwork” that allows users to train a convolutional neural network (CNN) for image classification. This can be used for tasks such as identifying objects in images or detecting abnormalities in medical scans.
Challenges in Implementing Machine Learning in MATLAB While MATLAB offers a user-friendly interface and a wide range of tools for machine learning, there are still challenges that users may face when implementing it in their projects. One challenge is choosing the right algorithm for a particular task. With so many options available, it can be overwhelming to determine which algorithm is best suited for a specific problem.
Another challenge is the need for a large and diverse dataset. Machine learning models require a significant amount of data to train effectively, and the quality and diversity of the dataset can greatly impact the performance of the model. This can be difficult to obtain in certain industries, especially in healthcare where patient data is highly sensitive.
In conclusion, MATLAB is a powerful tool for implementing machine learning in various projects. Its user-friendly interface, extensive library of algorithms, and visualization tools make it a popular choice among researchers and professionals. As technology continues to advance, we can expect to see even more innovative applications of machine learning in MATLAB. As such, it is essential for those in the field to continuously learn and adapt to stay ahead of the curve.
0 notes
Text
The Best Machine Learning Courses to Accelerate Your Career
Introduction
In the rapidly evolving world of technology, machine learning has emerged as a transformative force, revolutionizing industries and changing the way we interact with information. As businesses strive to harness the power of data-driven insights, the demand for skilled machine learning professionals is skyrocketing. To embark on a successful career in this field, enrolling in a high-quality machine learning course is crucial. In this article, we will explore some of the best machine learning courses available today.
"Machine Learning" by Stanford University (Coursera) Taught by the legendary Andrew Ng, this online course from Stanford University is widely regarded as a definitive introduction to machine learning. It covers the fundamental concepts, algorithms, and practical applications, making it an ideal starting point for beginners. The course employs a hands-on approach, with programming assignments in Octave or MATLAB, enabling students to implement their learning directly.
"Deep Learning Specialization" by deeplearning.ai (Coursera) Also led by Andrew Ng, this specialization is a must for those seeking to dive deep into the world of deep learning. It comprises five courses, covering neural networks, convolutional networks, recurrent networks, and more. This specialization offers a comprehensive understanding of state-of-the-art deep learning techniques and their applications in computer vision, natural language processing, and other fields.
"Machine Learning A-Z™: Hands-On Python & R In Data Science" (Udemy) This highly popular Udemy course provides an all-encompassing curriculum, catering to both Python and R users. With practical examples and real-world projects, learners gain valuable experience in applying machine learning algorithms to solve data science problems. The course covers regression, classification, clustering, and more, making it an excellent choice for individuals with diverse programming backgrounds.
"Applied Machine Learning" by Columbia University (edX) For those seeking a rigorous academic experience, this edX course from Columbia University is a perfect fit. It delves into advanced topics such as support vector machines, ensemble methods, and deep learning. The course includes hands-on labs using Python and scikit-learn, ensuring that learners develop a strong grasp of both theory and implementation.
"Machine Learning with TensorFlow on Google Cloud Platform" by Google Cloud (Coursera) This specialized course focuses on leveraging Google Cloud's machine learning services and TensorFlow to build powerful models. It covers topics like data preparation, feature engineering, model training, and hyperparameter tuning. Ideal for individuals looking to harness cloud-based machine learning solutions, this course equips learners with practical skills applicable to real-world projects.
"Fast.ai Practical Deep Learning for Coders" (fast.ai) Fast.ai is known for its unique approach to teaching deep learning, emphasizing a hands-on, practical methodology. This course empowers learners to quickly build and deploy cutting-edge deep learning models without extensive prerequisites. It covers topics like image classification, natural language processing, and collaborative filtering, among others, using popular libraries like PyTorch.
Conclusion
Machine learning continues to be at the forefront of technological innovation, shaping various industries and paving the way for a data-driven future. To excel in this dynamic field, choosing the right machine learning course is crucial. Whether you're a beginner or an experienced professional, the courses mentioned above offer top-notch instruction and hands-on experience to bolster your expertise and propel your career forward. Invest in your skills, embark on the journey of continuous learning, and unlock the limitless possibilities that machine learning has to offer.
1 note
·
View note
Note
an api for checking skins is at https://sessionserver.mojang.com/session/minecraft/profile/<UUID>
for example: https://sessionserver.mojang.com/session/minecraft/profile/68319ca7-db9d-4044-b142-f08580ca5c99
you could store that as a variable and check every so often. might go make that. i can feel the pull of my weird focus stuff
ohhh ok yeah here's what another anon sent:
that would make it a lot easier honestly. if either of you guys make a bot or script for this, please let me know!! i think it'd be very useful, especially if you're able to either make it a discord bot ppl could add or just link it to a tumblr account that makes a new post every time a skin is updated.
i know tumblr user @/timedeo has 2 tumblr accounts that’re run by a python bot to randomly generate posts based off of tubbo and timedeo’s tweets (@/tubbobot and @/deobot).
#anon#oakstar519#ty for the askkk :)#i can help? but idk how useful i'd be given the last time i had to code#was for a data science final project#made a fucking two layer neural network for sepsis prediction in like 4 days#slept maybe 6 hours during that period and i havent touched python since then#matlab my beloved#honestly itd be more useful than those twt accounts that track cc's spotify accts to see what they're listening to which is a little weird
17 notes
·
View notes
Text
Part 1 2
Jaune: I had a chat with Dr. Polendina earlier.
Penny: My father? What about?
Jaune: Your neural network. He was supposed to be the guy who understands consciousness is more than computation. Instead he told me he just guessed and it just sort of worked.
Penny: What does this mean?
Jaune: Well... Are you... Are you okay? Like it'd be one thing if you volunteered for this project. But you sort of got dragged into it and made by somebody who didn't really know what he was doing. And I guess none of us really know what we are doing. But that doesn't make it okay. So... Are you okay with that? Are you alright? Are you in agony?
Penny: I suppose... I don't think so. But I appreciate this concern. I feel that it's coming from an empathetic place.
Jaune: Right. I guess. It's just that your modality of consciousness could be totally different and alien to ours. And that's scary and it makes me worried about you. I just wanted to know if you were really alright and not in unbearable agony of something else horrific.
Penny: Do you understand neural networks and machine learning programs?
Jaune: A little. There were some computer science classes at Beacon I did alright in. And I understand some computational methods and statistics for modeling. And I did alright in physics. I don't have three doctorates like your dad does so maybe I'm shooting the dark and totally misunderstanding.
Penny: I didn't know that about you. Do you know programing languages?
Jaune: Some. C++, R, Matlab, Python, Latex, Bash, Batch and a little bit of a few others.
Penny: Is that a lot?
Jaune: Not really. I'm not very good at most of those and usually experts have two to three more. Do you not know any computer science?
Penny: Well, I... I guess not really. It wasn't what I was made for.
Jaune: But you run on the stuff.
Penny: Well do you understand how your eyes work and brain works?
Jaune: A little. I know a little about neurotransmitters and I know how the eye evolved to take in an image. From an open canvas of light sensitive cells to a pin hole to focus light to a pin hole with a lens. I understand how it received images upside down and flips them. Like I'm no expert but I know a little.
Penny: Oh. I guess maybe I took it for granted that my father knew exactly what he was doing.
Jaune: And... And how do you feel about that?
Penny: I... I don't know. But I'm grateful you came to talk to me. You're a good friend.
29 notes
·
View notes
Text
Top 10 Python Libraries for Machine Learning
With the increase in the markets for smart products, auto-pilot cars and other smart products, the ML industry is on a rise. Machine Learning is also one of the most prominent tools of cost-cutting in almost every sector of industry nowadays.
ML libraries are available in many programming languages, but python being the most user-friendly and easy to manage language, and having a large developer community, is best suited for machine learning purposes and that's why many ML libraries are being written in Python.
Also, the python works seamlessly with C and C++ and so, the already written libraries in C/C++ can be easily extended to Python. In this tutorial, we will be discussing the most useful and best machine-learning libraries in the Python programming language.
1. TensorFlow :
Website: https://www.tensorflow.org/ GitHub Repository: https://github.com/tensorflow/tensorflow Developed By: Google Brain Team Primary Purpose: Deep Neural Networks TensorFlow is a library developed by the Google Brain team for the primary purpose of Deep Learning and Neural Networks. It allows easy distribution of work onto multiple CPU cores or GPU cores, and can even distribute the work to multiple GPUs. TensorFlow uses Tensors for this purpose.
Tensors can be defined as a container that can store N-dimensional data along with its linear operations. Although it is production-ready and does support reinforcement learning along with Neural networks, it is not commercially supported which means any bug or defect can be resolved only by community help.
2. Numpy:
Website: https://numpy.org/
Github Repository: https://github.com/numpy/numpy
Developed By: Community Project (originally authored by Travis Oliphant)
Primary purpose: General Purpose Array Processing
Created on the top of an older library Numeric, the Numpy is used for handling multi-dimensional data and intricate mathematical functions. Numpy is a fast computational library that can handle tasks and functions ranging from basic algebra to even Fourier transforms, random simulations, and shape manipulations. This library is written in C language, which gives it an edge over standard python built-in sequencing.
Numpy arrays are better than pandas series in the term of indexing and Numpy works better if the number of records is less than 50k. The NumPy arrays are loaded into a single CPU which can cause slowness in processing over the new alternatives like Tensorflow, Dask, or JAX, but still, the learning of Numpy is very easy and it is one of the most popular libraries to enter into the Machine Learning world.
3. Natural Language Toolkit (NLTK):
Website:
https://www.nltk.org/
Github Repository:https://github.com/nltk/nltk
Developed By: Team NLTK
Primary Purpose: Natural Language Processing
NLTK is the widely used library for Text Classification and Natural Language Processing. It performs word Stemming, Lemmatizing, Tokenization, and searching a keyword in documents. The library can be further used for sentiment analysis, understanding movie reviews, food reviews, text-classifier, checking and censoring the vulgarised words from comments, text mining, and many other human language-related operations.
The wider scope of its uses includes AI-powered chatbots which need text processing to train their models to identify and also create sentences important for machine and human interaction in the upcoming future.
4.Pandas
Website: https://pandas.pydata.org/ Github Repository: https://github.com/pandas-dev/pandas Developed By: Community Developed (Originally Authored by Wes McKinney) Primary Purpose: Data Analysis and Manipulation The Library is written in Python Web Framework and is used for data manipulation for numerical data and time series. It uses data frames and series to define three-dimensional and two-dimensional data respectively. It also provides options for indexing large data for quick search in large datasets. It is well known for the capabilities of data reshaping, pivoting on user-defined axis, handling missing data, merging and joining datasets, and the options for data filtrations. Pandas is very useful and very fast with large datasets. Its speed exceeds that of Numpy when the records are more than 50k.
It is the best library when it comes to data cleaning because it provides interactiveness like excel and speed like Numpy. It is also one of the few ML libraries that can deal with DateTime without any help from any external libraries and also with a bare minimum code with python code quality. As we all know the most significant part of data analysis and ML is the data cleaning, processing, and analyzing where Pandas helps very effectively.
5. Scikit-Learn:
Website: https://scikit-learn.org/
Github Repository: https://github.com/scikit-learn/scikit-learn
Developed By: SkLearn.org
Primary Purpose: Predictive Data Analysis and Data Modeling
Scikit-learn is mostly focused on various data modeling concepts like regression, classification, clustering, model selections, etc. The library is written on the top of Numpy, Scipy, and matplotlib. It is an open-source and commercially usable library that is also very easy to understand.
It has easy integrability which other ML libraries like Numpy and Pandas for analysis and Plotly for plotting the data in a graphical format for visualization purposes. This library helps both in supervised as well as unsupervised learnings.
6. Keras:
Website: https://keras.io/
Github Repository: https://github.com/keras-team/keras
Developed By: various Developers, initially by Francois Chollet
Primary purpose: Focused on Neural Networks
Keras provides a Python interface of Tensorflow Library especially focused on AI neural networks. The earlier versions also included many other backends like Theano, Microsoft cognitive platform, and PlaidMl.
Keras contains standard blocks of commonly used neural networks, and also the tools to make image and text processing faster and smoother. Apart from standard blocks of neural networks, it also provides re-occurring neural networks.
7. PyTorch:
Website: https://pytorch.org/
Github Repository: https://github.com/pytorch/pytorch
Developed By: Facebook AI Research lab (FAIR)
Primary purpose: Deep learning, Natural language Processing, and Computer Vision
Pytorch is a Facebook-developed ML library that is based on the Torch Library (an open-source ML library written in Lua Programming language). The project is written in
Python Web Development, C++, and CUDA languages. Along with Python, PyTorch has extensions in both C and C++ languages. It is a competitor to Tensorflow as both of these libraries use tensors but it is easier to learn and has better integrability with Python. Although it supports NLP, but the main focus of the library is only on developing and training deep learning models only.
8. MlPack:
Github Repository: https://github.com/mlpack/mlpack
Developed By: Community, supported by Georgia Institute of technology
Primary purpose: Multiple ML Models and Algorithms
MlPack is mostly C++-based ML library that has bindings to Python other languages including R programming, Julia, and GO. It is designed to support almost all famous ML algorithms and models like GMMs, K-means, least angle regression, Linear regression, etc. The main emphasis while developing this library was on making it a fast, scalable, and easy-to-understand as well as an easy-to-use library so that even a coder new to programming can understand and use it without any problem. It comes under a BSD license making it approachable as both open source and also proprietary software as per the need.
9. OpenCV:
Website: https://opencv.org/
Github Repository: https://github.com/opencv/opencv
Developed By: initially by Intel Corporation
Primary purpose: Only focuses on Computer Vision
OpenCV is an open-source platform dedicated to computer vision and image processing. This library has more than 2500 algorithms dedicated to computer vision and ML. It can track human movements, detect moving objects, extract 3d models, stitch images together to create a high-resolution image, exploring the AR possibilities.
It is used in various CCTV monitoring activities by many governments, especially in China and Israel. Also, the major camera companies in the world use OpenCv for making their technology smart and user-friendly.
10. Matplotlib:
Website: https://matplotlib.org/
Github Repository: https://github.com/matplotlib/matplotlib
Developed By: Micheal Droettboom, Community
Primary purpose: Data Visualization
Matplotlib is a library used in Python for graphical representation to understand the data before moving it to data-processing and training it for Machine learning purposes. It uses python GUI toolkits to produce graphs and plots using object-oriented APIs.
The Matplotlib also provides a MATLAB-like interface so that a user can do similar tasks as MATLAB. This library is free and open-source and has many extension interfaces that extend matplotlib API to various other libraries.
Conclusion:
In this blog, you learned about the best Python libraries for machine learning. Every library has its own positives and negatives. These aspects should be taken into account before selecting a library for the purpose of machine learning and the model’s accuracy should also be checked after training and testing the models so as to select the best model in the best library to do your task.
Also Read:
Unit Testing Frameworks in Python
0 notes
Text
Learn About Different Tools Used in Data Science
Data Science is a very broad spectrum and all its domains need data handling in unique way which get many analysts and data scientists into confusion. If you want to be pro-active in finding the solution to these issues, then you must be quick in making decision in choosing the right tools for your business as it will have a long-term impact.
This article will help you have a clear idea while choosing the best tool as per your requirements.
Let's start with the tools which helps in reporting and doing all types of analysis of data analytic and getting over to dashboarding. Some of the most common tools used in reporting and business intelligence (BI) are as follows:
- Excel: In this you get wide range of options which includes Pivot table and charts, with which you can do the analysis more quickly and easily.
- Tableau: This is one of the most popular visualization tools which is also capable of handling large amounts of data. This tool provides an easy way to calculate functions and parameters, along-with a very neat way to present it in a story interface.
- PowerBI: Microsoft offers this tool in its Business Intelligence (BI) Space, which helps in integrations of Microsoft technologies.
- QlikView: This is also a very popular tool because it’s easy to learn and is also a very intuitive tool. With this, one can integrate and merge, search, visualize and analyse all the sources of data very easily.
- Microstrategy: This BI tool also supports dashboards, key data analytics tasks like other tools and automated distributions as well.
Apart from all these tools, there is one more which you cannot exclude from this tool's list, and that tool is
- Google Analytics: With google analytics, you can easily track all your digital efforts and what role they are playing. This will help in improvising your strategy.
Now let's get to the part where most of the data scientists deal with. The following predictive analytics and machine learning tools will help you solve forecasting, statistical modelling, neural networks and deep learning.
- R: It is very commonly used language in data science. You can access its libraries and packages as they are easily available. R has also a very strong community which will you if you got with something.
- Python: This is also one of the most common language for data science, or you can also say that this is one the most used language for data science. It is an open-source language which makes it favourite among data scientists. It has gained a good place because of its ease and flexibility.
- Spark: After becoming open source, it has become one of the largest communities in the world of data. It holds its place in data analytics as it offers features of flexibility, computational power, speed, etc.
- Julia: This is a new and emerging language which is very similar to Python along-with some extra features.
- Jupyter Notebooks: This is an open-source web application widely used in Python for coding. It is mainly used in Python, but it also supports R, Julia etc.
Apart from all these widely used tools, there are some other tools of the same category that are recognized as industry leaders.
- SAS
- SPSS
- MATLAB
Now let's discuss about the data science tools for Big Data. But to truly understand the basic principles of big data, we will categorize the tools by 3 V's of big data:
· Volume
· Variety
· Velocity
Firstly, let's list the tools as per the volume of the data.
Following tools are used if data range from 1GB to 10GB approx.:
- Microsoft Excel: Excel is most popular tool for handling data, but which are in small amounts. It has limitations of handling up to 16,380 columns at a time. This is not a good choice when you have big data in hand to deal with.
- Microsoft Access: This is also another tool from Microsoft in which you handle databases up to 2 Gb, but beyond that it will not be able to handle.
- SQL: It has been the primary database solution from last few decades. It is a good option and is most popular data management system but, it still has some drawbacks and become difficult to handle when database continues to grow.
- Hadoop: If your data accounts for more than 10Gb then Hadoop is the tool for you. It is an open-source framework that manages data processing for big data. It will help you build a machine learning project from starting.
- Hive: It has a SQL-like interface built on Hadoop. It helps in query the data which has been stored in various databases.
Secondly, let's discuss about the tools for handling Variety
In Variety, different types of data are considered. In all, data are categorized as Structured and Unstructured data.
Structured data are those with specified field names like the employee details of a company or a school database or the bank account details.
Unstructured data are those type of data which do not follow any trend or pattern. They are not stored in a structured format. For example, the customer feedbacks, image feed, video fee, emails etc.
It becomes really a difficult task while handling these types of data. Two most common databases used in managing these data are SQL and NoSQL.
SQL has been a dominant market leader from a long time. But with the emergence of NoSQL, it has gained a lot of attention and many users have started adopting NoSQL because of its ability to scale and handle dynamic data.
Thirdly, there are tools for handling velocity.
It basically means the velocity at which the data is captured. Data could be both real time and non-real time.
A lot of major businesses are based on real-time data. For example, Stock trading, CCTV surveillance, GPS etc.
Other options include the sensors which are used in cars. Many tech companies have launched the self-driven cars and there are many high-tech prototypes in cue to be launched. Now these sensors need to be in real-time and very quick to dynamically collect and process data. The data could be regarding the lane, it could be regarding the GPS location, it could be regarding the distance from other vehicles, etc. All these data need to be collected and processed at the same time.
So, for these types of data following tools are helping in managing them:
- Apache Kafka: This is an open-source tool by Apache and is quick. One good feature of this tool is that this is fault-tolerant because of which this is used in production in many organisations.
- Apache Storm: This is another tool from Apache which can used with most of the programming language. It is considered very fast and good option for high data velocity as it can process up to 1 Million tuples/second.
- Apache Flink: This tool from Apache is also used to process real-time data. Some of its advantages are fault-tolerance, high performance and memory management.
- Amazon Kinesis: This tool from Amazon is a very powerful option for organizations which provides a lot of options, but it comes with a cost.
We have discussed about almost all the popular tools available in the market. But it’s always advisable to contact some data science consulting services to better understand the requirements and which tool will be best suitable for you.
Look for the best data science consulting company which would best suit in your requirements list.
#data science#data science consulting services#best data science consulting company#data science consultant
5 notes
·
View notes
Text
How to Find a Perfect Deep Learning Framework
Many courses and tutorials offer to guide you through building a deep learning project. Of course, from the educational point of view, it is worthwhile: try to implement a neural network from scratch, and you’ll understand a lot of things. However, such an approach does not prepare us for real life, where you are not supposed to spare weeks waiting for your new model to build. At this point, you can look for a deep learning framework to help you.
A deep learning framework, like a machine learning framework, is an interface, library or a tool which allows building deep learning models easily and quickly, without getting into the details of underlying algorithms. They provide a clear and concise way for defining models with the help of a collection of pre-built and optimized components.
Briefly speaking, instead of writing hundreds of lines of code, you can choose a suitable framework that will do most of the work for you.
Most popular DL frameworks
The state-of-the-art frameworks are quite new; most of them were released after 2014. They are open-source and are still undergoing active development. They vary in the number of examples available, the frequency of updates and the number of contributors. Besides, though you can build most types of networks in any deep learning framework, they still have a specialization and usually differ in the way they expose functionality through its APIs.
Here were collected the most popular frameworks
TensorFlow
The framework that we mention all the time, TensorFlow, is a deep learning framework created in 2015 by the Google Brain team. It has a comprehensive and flexible ecosystem of tools, libraries and community resources. TensorFlow has pre-written codes for most of the complex deep learning models you’ll come across, such as Recurrent Neural Networks and Convolutional Neural Networks.
The most popular use cases of TensorFlow are the following:
NLP applications, such as language detection, text summarization and other text processing tasks;
Image recognition, including image captioning, face recognition and object detection;
Sound recognition
Time series analysis
Video analysis, and much more.
TensorFlow is extremely popular within the community because it supports multiple languages, such as Python, C++ and R, has extensive documentation and walkthroughs for guidance and updates regularly. Its flexible architecture also lets developers deploy deep learning models on one or more CPUs (as well as GPUs).
For inference, developers can either use TensorFlow-TensorRT integration to optimize models within TensorFlow, or export TensorFlow models, then use NVIDIA TensorRT’s built-in TensorFlow model importer to optimize in TensorRT.
Installing TensorFlow is also a pretty straightforward task.
For CPU-only:
pip install tensorflow
For CUDA-enabled GPU cards:
pip install tensorflow-gpu
Learn more:
An Introduction to Implementing Neural Networks using TensorFlow
TensorFlow tutorials
PyTorch
PyTorch
Facebook introduced PyTorch in 2017 as a successor to Torch, a popular deep learning framework released in 2011, based on the programming language Lua. In its essence, PyTorch took Torch features and implemented them in Python. Its flexibility and coverage of multiple tasks have pushed PyTorch to the foreground, making it a competitor to TensorFlow.
PyTorch covers all sorts of deep learning tasks, including:
Images, including detection, classification, etc.;
NLP-related tasks;
Reinforcement learning.
Instead of predefined graphs with specific functionalities, PyTorch allows developers to build computational graphs on the go, and even change them during runtime. PyTorch provides Tensor computations and uses dynamic computation graphs. Autograd package of PyTorch, for instance, builds computation graphs from tensors and automatically computes gradients.
For inference, developers can export to ONNX, then optimize and deploy with NVIDIA TensorRT.
The drawback of PyTorch is the dependence of its installation process on the operating system, the package you want to use to install PyTorch, the tool/language you’re working with, CUDA and others.
Learn more:
Learn How to Build Quick & Accurate Neural Networks using PyTorch — 4 Awesome Case Studies
PyTorch tutorials
Keras
Keras was created in 2014 by researcher François Chollet with an emphasis on ease of use through a unified and often abstracted API. It is an interface that can run on top of multiple frameworks such as MXNet, TensorFlow, Theano and Microsoft Cognitive Toolkit using a high-level Python API. Unlike TensorFlow, Keras is a high-level API that enables fast experimentation and quick results with minimum user actions.
Keras has multiple architectures for solving a wide variety of problems, the most popular are
image recognition, including image classification, object detection and face recognition;
NLP tasks, including chatbot creation
Keras models can be classified into two categories:
Sequential: The layers of the model are defined in a sequential manner, so when a deep learning model is trained, these layers are implemented sequentially.
Keras functional API: This is used for defining complex models, such as multi-output models or models with shared layers.
Keras is installed easily with just one line of code:
pip install keras
Learn more:
The Ultimate Beginner’s Guide to Deep Learning in Python
Keras Tutorial: Deep Learning in Python
Optimizing Neural Networks using Keras
Caffe
The Caffe deep learning framework created by Yangqing Jia at the University of California, Berkeley in 2014, and has led to forks like NVCaffe and new frameworks like Facebook’s Caffe2 (which is already merged with PyTorch). It is geared towards image processing and, unlike the previous frameworks, its support for recurrent networks and language modeling is not as great. However, Caffe shows the highest speed of processing and learning from images.
The pre-trained networks, models and weights that can be applied to solve deep learning problems collected in the Caffe Model Zoo framework work on the below tasks:
Simple regression
Large-scale visual classification
Siamese networks for image similarity
Speech and robotics applications
Besides, Caffe provides solid support for interfaces like C, C++, Python, MATLAB as well as the traditional command line.
To optimize and deploy models for inference, developers can leverage NVIDIA TensorRT’s built-in Caffe model importer.
The installation process for Caffe is rather complicated and requires performing a number of steps and meeting such requirements, as having CUDA, BLAF and Boost. The complete guide for installation of Caffe can be found here.
Learn more:
Caffe Tutorial
Choosing a deep learning framework
You can choose a framework based on many factors you find important: the task you are going to perform, the language of your project, or your confidence and skillset. However, there are a number of features any good deep learning framework should have:
Optimization for performance
Clarity and ease of understanding and coding
Good community support
Parallelization of processes to reduce computations
Automatic computation of gradients
Model migration between deep learning frameworks
In real life, it sometimes happens that you build and train a model using one framework, then re-train or deploy it for inference using a different framework. Enabling such interoperability makes it possible to get great ideas into production faster.
The Open Neural Network Exchange, or ONNX, is a format for deep learning models that allows developers to move models between frameworks. ONNX models are currently supported in Caffe2, Microsoft Cognitive Toolkit, MXNet, and PyTorch, and there are connectors for many other popular frameworks and libraries.
New deep learning frameworks are being created all the time, a reflection of the widespread adoption of neural networks by developers. It is always tempting to choose one of the most common one (even we offer you those that we find the best and the most popular). However, to achieve the best results, it is important to choose what is best for your project and be always curious and open to new frameworks.
10 notes
·
View notes
Text
Top 8 Python Libraries for Data Science
Python language is popular and most commonly used by developers in creating mobile apps, games and other applications. A Python library is nothing but a collection of functions and methods which helps in solving complex data science-related functions. Python also helps in saving an amount of time while completing specific tasks.
Python has more than 130,000 libraries that are intended for different uses. Like python imaging library is used for image manipulation whereas Tensorflow is used for the development of Deep Learning models using python.
There are multiple python libraries available for data science and some of them are already popular, remaining are improving day-by-day to reach their acceptance level by developers
Read: HOW TO SHAPE YOUR CAREER WITH DATA SCIENCE COURSE IN BANGALORE?
Here we are discussing some Python libraries which are used for Data Science:
1. Numpy
NumPy is the most popular library among developers working on data science. It is used for performing scientific computations like a random number, linear algebra and Fourier transformation. It can also be used for binary operations and for creating images. If you are in the field of Machine Learning or Data Science, you must have good knowledge of NumPy to process your real-time data sets. It is a perfect tool for basic and advanced array operations.
2. Pandas
PANDAS is an open-source library developed over Numpy and it contains Data Frame as its main data structure. It is used in high-performance data structures and analysis tools. With Data Frame, we can manage and store data from tables by performing manipulation over rows and columns. Panda library makes it easier for a developer to work with relational data. Panda offers fast, expressive and flexible data structures.
Translating complex data operations using mere one or two commands is one of the most powerful features of pandas and it also features time-series functionality.
3. Matplotlib
This is a two-dimensional plotting library of Python a programming language that is very famous among data scientists. Matplotlib is capable of producing data visualizations such as plots, bar charts, scatterplots, and non-Cartesian coordinate graphs.
It is one of the important plotting libraries’ useful in data science projects. This is one of the important library because of which Python can compete with scientific tools like MatLab or Mathematica.
4. SciPy
SciPy library is based on the NumPy concept to solve complex mathematical problems. It comes with multiple modules for statistics, integration, linear algebra and optimization. This library also allows data scientist and engineers to deal with image processing, signal processing, Fourier transforms etc.
If you are going to start your career in the data science field, SciPy will be very helpful to guide you for the whole numerical computations thing.
5. Scikit Learn
Scikit-Learn is open-sourced and most rapidly developing Python libraries. It is used as a tool for data analysis and data mining. Mainly it is used by developers and data scientists for classification, regression and clustering, stock pricing, image recognition, model selection and pre-processing, drug response, customer segmentation and many more.
6. TensorFlow
It is a popular python framework used in deep learning and machine learning and it is developed by Google. It is an open-source math library for mathematical computations. Tensorflow allows python developers to install computations to multiple CPU or GPU in desktop, or server without rewriting the code. Some popular Google products like Google Voice Search and Google Photos are built using the Tensorflow library.
7. Keras
Keras is one of the most expressive and flexible python libraries for research. It is considered as one of the coolest machine learning Python libraries offer the easiest mechanism for expressing neural networks and having all portable models. Keras is written in python and it has the ability to run on top of Theano and TensorFlow.
Compared to other Python libraries, Keras is a bit slow, as it creates a computational graph using the backend structure and then performs operations.
8. Seaborn
It is a data visualization library for a python based on Matplotlib is also integrated with pandas data structures. Seaborn offers a high-level interface for drawing statistical graphs. In simple words, Seaborn is an extension of Matplotlib with advanced features.
Matplotlib is used for basic plotting such as bars, pies, lines, scatter plots and Seaborn is used for a variety of visualization patterns with few syntaxes and less complexity.
With the development of data science and machine learning, Python data science libraries are also advancing day by day. If you are interested in learning python libraries in-depth, get NearLearn’s Best Python Training in Bangalore with real-time projects and live case studies. Other than python, we provide training on Data Science, Machine learning, Blockchain and React JS course. Contact us to get to know about our upcoming training batches and fees.
Call: +91-80-41700110
Mail: [email protected]
#Top Python Training in Bangalore#Best Python Course in Bangalore#Best Python Course Training in Bangalore#Best Python Training Course in Bangalore#Python Course Fees in Bangalore
1 note
·
View note
Text
Machine Learning Training in Noida
Apache MXNet on AWS- For quick training machine learning applications
Need a quick and scalable training and inference framework. Try Apache MXNet. With its straightforward and concise API for machine learning, it will fulfill your need without a doubt.
MXNet includes the extremely vital Gluon the interface that lets developers of all skill levels to get easily started with deep learning on the cloud, on mobile apps, and on edge devices. Few lines of Gluon code can enable you to build recurrent LSTMs, linear regression and convolutional networks for speech recognition, recommendation, object detection and personalization.
Amazon SageMaker, a top rate platform to build, train, and deploy machine learning models at scale can be relied on to get started with MxNet on AWS with a fully-managed exceptional experience. AWS Deep Learning AMIs can also be used to build custom environments and workflows not only with MxNet but other frameworks as well. These frameworks are TensorFlow, Caffe2, Keras, Caffe, PyTorch, Chainer, and Microsoft Cognitive Toolkit.
Benefits of deep learning using MXNet
Ease-of-Use with Gluon
You don’t have to sacrifice training speed in your endeavor to prototype, train, and deploy deep learning models by employing MXNet’s Gluon library that provides a high-level interface. For loss functions, predefined layers, and optimizers, Gluon offers high-level abstractions. Easy to debug and intuitive to work with flexible structure is provided by Gluon.
Greater Performance
Tremendously large projects can be handled in less time simply because of distribution of deep learning workloads across multiple GPUs with almost linear scalability is possible. Depending on the number of GPUs in a cluster, scaling is automatic. Developers increase productivity by saving precious time by running serverless and batch-based inferencing.
For IoT & the Edge
MXNet produces lightweight neural network model representations besides handling multi-GPU training and deployment of complex models in the cloud. This lightweight neural network model representations can run on lower-powered edge devices like a Raspberry Pi, laptop, or smartphone and process data in real-time remotely.
Flexibility & Choice
A wide array of programming languages like C++, R, Clojure, Matlab, Julia, JavaScript, Python, Scala, and Perl, etc. is supported by MXNet. So, you can easily get started with languages that are already known to you. However, on the backend, all code is compiled in C++ for the best performance regardless of language used to build the models.
1 note
·
View note
Text
Top 5 Python Libraries You Must Know
If there’s one dynamic programming language that taking the lead role in disruptive technology applications, it’s Python. One of the basic and important reasons for the programming language’s popularity is its code readability.
Python is increasingly finding applications in disruptive technologies such as data analytics, machine learning, and artificial intelligence. By learning and mastering the programming language, you can get an edge on your career.
Credit: https://stackoverflow.blog/2017/09/06/incredible-growth-python/
Here are the top 5 python libraries:
Matplotlib
Matplotlib is a plotting library for python; it is extensively used for data visualisation as it is designed to produce graphs and plots. The object-oriented API of the library can be used to embed the plots into applications.
Matplotlib makes a great alternative to MATLAB. It is compatible with all types of operating systems as it supports several backends and output types.
Keras
Keras is one of the popular machine learning libraries in Python that eases graph visualisation, data-set processing, and model compiling. Keras is designed to allow ease of neural network expression.
One of the biggest advantages of using Keras is the python library runs smoothly on CPU as well as GPU. Also, Keras supports different types of neural network models, including fully connected, embedding, recurrent, pooling, and convolutional.
NumPy
NumPy is another popular machine learning library in Python. NumPy is one of the libraries TensorFlow uses in order to perform operations on Tensors. NumPy eases coding as therefore is beginner-friendly. Also, the interactive design of the library makes it easy to use.
NumPy Array Object
Pandas
Pandas is the most popular data science library in python that is widely used for data analysis and cleaning. The library’s high-level abstraction makes it a popular choice. WIth Pandas, you can create your own function and run it on data sets.
Growth of Panda as per google search trends
Also, Pandas is highly suitable for time-series-specific functionality such as date shifting, linear regression, moving window, and date range generation. Finance and statistics are the two commercial areas where Pandas come to use.
Theano
Theano is a commonly-used computational framework machine learning library in Python. Theano is similar to TensorFlow in terms of functionality; it is widely used as a standard library for research and development for Deep Learning.
The library was initially designed for computation for large neural network algorithms. The rising number of multiple neural network projects has led to an increase in the popularity of the machine learning library.
Attend a bootcamp for data science and get an edge on your career Attend a data science bootcamp and understand the potential of the disruptive technology. Attending the bootcamp is also helpful for choosing the right programming languages that are used in disruptive technologies.
1 note
·
View note